Abstract
Breast cancer is one of the deadly cancer types that causes high mortality among women globally. Meanwhile, Deep Learning (DL) emerges as the most frequently utilized and rapidly developing branch of classical machine learning. The study examines a modern Computer-Aided Diagnosis (CAD) framework that uses DL to extract features and classify them for aiding radiologists in breast cancer diagnosis. This is accomplished through four distinct experimentations aimed at identifying the most optimal method of effective classification. Here, the first uses Deep CNNs that are pre-trained, such as AlexNet, GoogleNet, ResNet50, and Dense-Net121. The second is based on experiments using Deep CNNs to extract features and applying them onto a Support Vector Machine algorithm using three different kernels. The next one involves the fusion of different deep features for demonstrating the classification improvement by fusion of these deep features. The final experiment involves Principal Component Analysis (PCA) for reducing the computational cost and for decreasing the larger feature vectors created during fusion. The abovesaid experimentations are carried out in two different mammogram datasets namely MIAS and INbreast. The classification accuracy attained for both datasets through the fuzing of deep features (97.93% for MIAS and 96.646% for INbreast) is the highest compared with the state-of-the-art frameworks. In contrast, the classification performance did not enhance while applying the PCA on combined deep features; but the decrease in execution time provides a reduced computational cost.
Abbreviations: CAD: Computer Aided Diagnosis; CNN: Convolution Neural Network; CSI: Classification Success Index; DCNN: Deep Convolution Neural Network; DICOM: Digital Imaging and Communications in Medicine; DL: Deep Learning; FC layer: Fully Connected layer; FFDM: Full-Field Digital Mammograms; FN: False Negative; FP: False Positive; ICSI: Individual Classification Success Index; MIAS: Mammographic Image Analysis Society; ML: Machine Learning; MLO: Medio-Lateral Oblique; PCA: Principal Component Analysis; PGM: Portable Gray Map; PPV: Positive Predictive Value; RBF: Radial Basis Function; SGDM: Stochastic Gradient Descent with Momentum; SVM: Support Vector Machine; TN: True Negative; TP: True Positive; TPR: True Positive Rate; UK: United Kingdom
DISCLOSURE STATEMENT
No potential conflict of interest was reported by the author(s).
Additional information
Notes on contributors
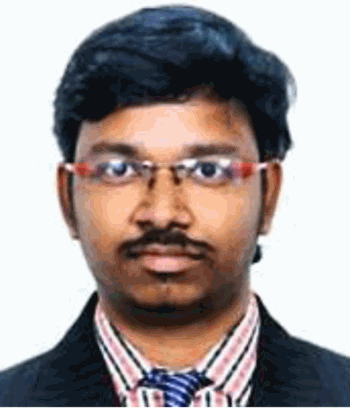
S. R. Sannasi Chakravarthy
Sannasi Chakravarthy S R completed his BE degree in ECE from P T Lee CNCET, Kanchipuram, India, in 2010; ME degree in applied electronics from Thanthai Periyar Institute of Technology, Vellore, India, in 2012, and a PhD degree in machine learning from Anna University, Chennai, in 2020.Currently, he is an assistant professor at Bannari Amman Institute of Technology, Tamilnadu, India. His area of research includes machine learning and deep learning in the biomedical field.
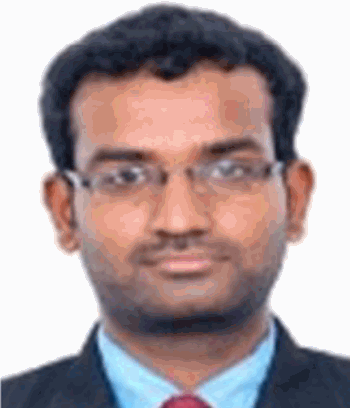
N. Bharanidharan
Bharanidharan N completed his PhD in Machine Learning from Anna University, Chennai in 2020. His area of research includes machine learning and deep learning in the biomedical field. Email: [email protected]

Harikumar Rajaguru
Harikumar R completed his PhD in Bio-Signal Processing from Anna University. His area of research includes machine learning and deep learning in the biomedical field. Email: [email protected]