Abstract
In the world of detection and classification, You Only Look Once (YOLO)-v3 has been maintaining its position as the best classifier and detector and is due to its fastness, accuracy, less complex structure, continuous modifications, improvements, etc. Several approaches have been proposed in this paper to modify improved YOLO-v3. Our main contributions are (1) increasing the number of anchor boxes; (2) replacement of K-means clustering with density-based spatial clustering of applications with noise (DBSCAN); (3) introducing final bounding box alignment (FBBA) technique; and (4) replacement of multi-scaling with a depth calculation algorithm. At first, we increased the number of anchor boxes from 9 to 11 which resulted in a higher performance of 6% for both visual object classes and common objects in context datasets, where increment in computational cost was negligible. Similarly, it has been analyzed that a true number of anchor boxes can increase efficiency. So, instead of generating a static number of anchor boxes with k-means clustering, DBSCAN has been proposed for generating a dynamic and accurate number of anchor boxes. For minimizing the localization error, a new FBBA method has been introduced which would exactly overlap the object. In the same way, depth calculation algorithm can solve the small and large size object detection and classification problem without doing multi-scaling.
Additional information
Notes on contributors
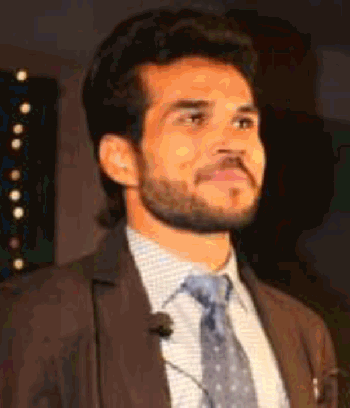
Muhammad Nawaz
Muhammad Nawaz has done his graduation with a Bachelor of Science degree in computer science from Namal College Mianwali, an Associate College of the University of Bradford UK (2019). Currently, he is pursuing a master’s degree in data science at NUST School of Electrical Engineering and Computer Science, Islamabad, Pakistan. His research interest and command lie in data science, machine learning, artificial intelligence, computer vision, image and video processing, etc.
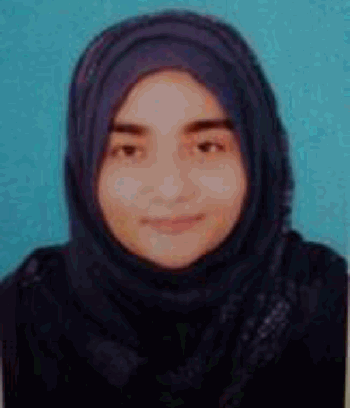
Mahrukh Khalil
Mahrukh Khalil received the BS degree in computer system engineering from the Islamia University of Bahawalpur, Pakistan (2019). Currently, she is doing MS in data science from the School of Electrical Engineering and Computer Science, National University of Science and Technology, Pakistan. Her research interests are computer vision, machine learning, and data science. Email: [email protected]
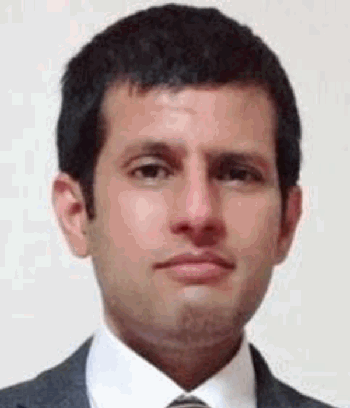
Muhammad Khuram Shehzad
Muhammad Khuram Shehzad received the BE and MS degrees in information technology from The University of Lahore (2004) and National University of Science and Technology (NUST), Islamabad, Pakistan (2007), respectively. He earned a PhD degree in computer engineering from Sungkyunkwan University (SKKU), Suwon, South Korea. He joined MONET Lab in SKKU as postdoctoral researcher in 2016. Later, he worked as assistant professor with the Electrical Energy Engineering Department from 2017 to 2018 at Keimyung University, Daegu, South Korea. Currently, he is working as an assistant professor at the Department of Computing, School of Electrical Engineering and Computer Science, NUST, Islamabad, Pakistan. His responsibilities include PG CS Coordinator, PG Data Science Coordinator, PG CS Interviews, and also worked as QS Ranking Rubrics lead. His research interests include artificial intelligence, data science, and graph theory. Email: [email protected]