Abstract
The standard communication protocols transfer big data over the internet. However, the challenge lies while sharing the massive amount of genomics data quickly over limited bandwidth networks. Managed File Transfer (MFT) that uses File Transfer Protocol Secure (FTPS) or Secure Shell File Transfer Protocol (SFTP) is a scalable and secure alternative for the purpose. This paper proposes a framework consisting of a Variable-length Genomic Binary Encoding (VGBE) scheme and a hybrid Gated Recurrent Unit-Convolutional Neural Network model (GRU-CNN). The Method exploits Managed File Transfer to reduce the bit size before transmission and increases the bit rate during the transmission of genomics data. Consequently, the method ensures a minimum number of bits per word and less latency. The methodology is tested on various communication protocols. It is validated in terms of performance metrics viz. transfer rate and the size of transferred data using diverse benchmark datasets such as protein sequences and human reference genome taken from the University of California Santa Cruz (UCSC) and National Center for Biotechnology Information (NCBI), respectively. Furthermore, simulation and implementation results show that the model is 98% faster, has 96% less data size, and is more secure than standard communication protocols. Thus, the proposed method is an efficient and secure genomics data transfer method using deep learning techniques.
ACKNOWLEDGEMENTS
The authors are grateful to the Director, Sant Longowal Institute of Engineering and Technology, Longowal, India, for providing all the necessary facilities for this research. The authors are highly thankful to Sh Amritpal Singh for providing the idea of the research field and Er. Sarbjeet Singh for his continuous support.
DISCLOSURE STATEMENT
No potential conflict of interest was reported by the author(s).
Additional information
Notes on contributors
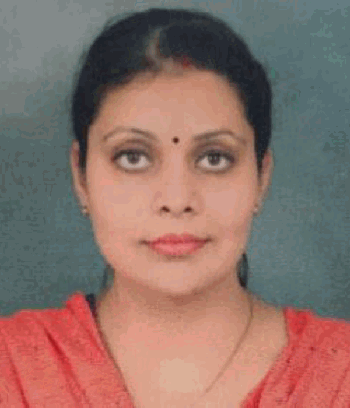
Amandeep Kaur
Amandeep Kaur is a doctoral research scholar in the Department of Electronics and Communication Engineering at Sant Longowal Institute of Engineering and Technology, Longowal, India. She did her BE and Masters from Thapar Institute of Engineering and Technology, Patiala, India. She is a recipient of a fellowship by the Ministry of Education, Government of India. Her research interests are deep learning, medical imaging, and genomics data analysis.
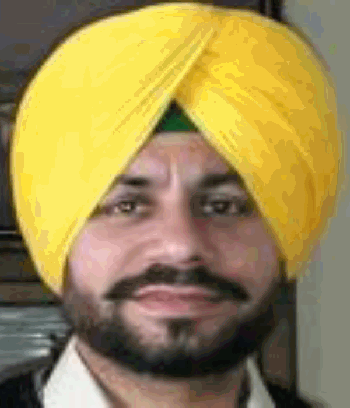
Ajay Pal Singh Chauhan
Ajay Pal Singh Chauhan is working as a professor in the Department of Electronics and Communication Engineering at Sant Longowal Institute of Engineering and Technology, Longowal, India. He did his BE from Gulbarga university, Karnataka, India; masters from Guru Nanak Dev Engineering College, Ludhiana, India, and PhD from Sant Longowal Institute of Engineering and Technology, Longowal, India. His area of interest is computer vision and image processing. Email: [email protected]
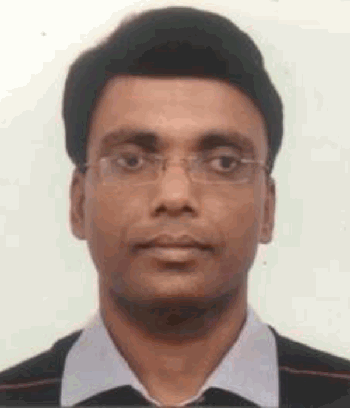
Ashwani Kumar Aggarwal
Ashwani Kumar Aggarwal is working as associate professor in the Department of Electrical and Instrumentation Engineering at Sant Longowal Institute of Engineering and Technology, Longowal, India. He did BE from the College of Engineering and Technology, Bathinda, India; masters from the Indian Institute of Science, Bangalore, India, and PhD from the University of Tokyo, Japan. His area of interest is artificial intelligence and machine learning methods. Email: [email protected]