Abstract
Alcohol is considered to be a psychoactive substance that has dependence-producing properties. The early prediction of the degree of intake of alcohol can be helpful to get early intervention through counseling, and other preventive measures can be employed. Existing works related to alcohol use prediction have only focused on identifying whether an individual is an alcohol user or a non-user. There is no remarkable efficient model for predicting the time of use of alcohol to date. In this work, novel approaches have been proposed to predict the time of use of alcohol consumption by individuals. It predicts that the alcohol is consumed on just the previous day or previous week or previous month, or a previous year or not at all consumed. Ultimately, the risk of becoming an alcohol addict has been identified. The input features used in this study are demographic features and personality traits. SMOTE with Tomek link has been used to make the samples balanced in all the classes. Machine learning algorithms, such as K-nearest neighbour (k-NN), random forest and gradient boosting, have been used to predict the time of use based on input features. The highest accuracy of 77% is obtained in the random forest algorithm using the feature set comprising of demographic features and personality traits. The obtained sensitivity, specificity, precision and F-score are 76.198%, 94.19%, 75.53% and 75.86%, respectively. The novelty of the work is that it can predict the time of use of alcohol without a clinical test with acceptable accuracy.
DISCLOSURE STATEMENT
No potential conflict of interest was reported by the author(s).
Additional information
Notes on contributors
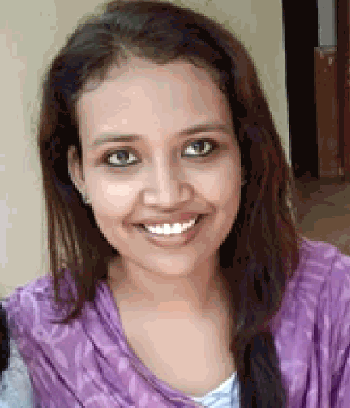
Divya Kumari
Divya Kumari is currently working as an assistant professor in the School of Computer Engineering, Kalinga Institute of Industrial Technology, Bhubaneswar. She has over 6 years of experience in teaching, research and industry. Her research focuses on machine learning and information security. Email: [email protected]
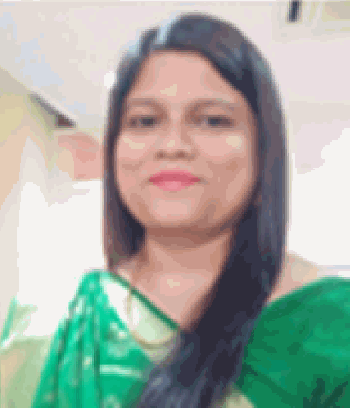
Aleena Swetapadma
Aleena Swetapadma received the BTech degree from CET, Bhubaneswar, India (2011) and MTech degree from NIT, Raipur, India (2013). She received the PhD degree in electrical engineering from NIT, Raipur, India, in 2016. She is currently working as assistant professor in KIT University, Bhubaneswar, India. Her research interest includes power system protection, FACTS, HVDC, artificial intelligence, and signal and image processing. She is a member of IEEE, IE India and Institute of Scholars, India. She got a POSOCO Power System Award from PGCIL, India, for her MTech thesis (2014) and PhD thesis (2014) and Ph.D. thesis (2017). She is conferred with the Young Achiever Award 2021 from the Institute of Scholars. She has published papers in refereed journals such as IEEE, IET, Elsevier, Springer, Wiley, etc.