Abstract
The Coronavirus pandemic devastatingly affects worldwide social prosperity, and general well-being, deadening the human way of life all around the world and undermining our security. Due to the increasing number of confirmed cases associated with COVID-19, it is more important to identify the healthy and infected patients so the control of spread and treatment of infected patients can be done effectively. This work aims to correlate the presence of Covid-19 with the help of both chest X-ray images and CT Scan Images. Deep ensemble learning models take advantage of the different deep learning models, combine them, and produce a model with better performance. The proposed system involves Data augmentation and preprocessing of CT scan images. The same process is applied for Chest X-ray Images, compares the evaluation metrics amongst the models, and suggests the best use of CT scan and Chest X-ray for better Results and accuracy. The features extracted from the Inception V3 model are combined with the features extracted from the Xception model. The inception model convolves the same input tensor with the help of multiple filters, and the results are concatenated. The pre-trained Xception model is capable of depth-wise separable convolutions. The proposed framework works in Covid-19 diagnosis with an accuracy of 96% in Xception and 98% while combining Xception and InceptionV3 models. The final results showed that the Convolutional Neural Network Classifier built with the ensemble of Inception and Xception models that use X-ray images efficiently collects the essential features related to the infections of COVID-19.
Disclosure statement
No potential conflict of interest was reported by the author(s).
Additional information
Notes on contributors
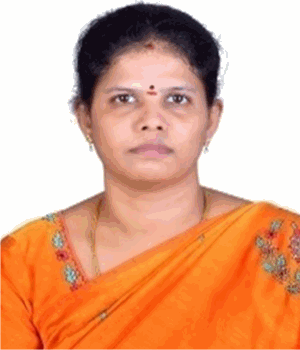
K.V. Uma
KV Uma is currently working as assistant professor, Faculty of Information Technology department in Thiagarajar College of Engineering. She has a total teaching experience of 13 yrs and her area of research is deep learning. She has completed UG in computer science and engineering in the year 2002 from Periyar University. Completed her PG in computer science and engineering in the year 2010 from Thiagarajar College of engineering in Tamilnadu. She has completed PhD in the year 2021 under Anna University in Chennai. Corresponding author. Email: [email protected]
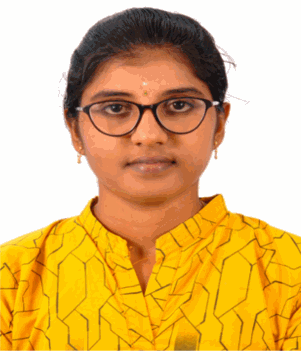
C. Sakthi Birundha
C Sakthi Birundha recently completed an undergraduate B Tech degree in the department of Information Technology from Thiagarajar College of Engineering, Madurai and Tamil Nadu, India. Her areas of specializations are database management systems, machine learning. Email: [email protected]

S. Subasri
S Subasri recently completed an undergraduate BTech Degree in the department of Information Technology from Thiagarajar College of Engineering, Madurai, Tamil Nadu, India. Her areas of specializations are database management systems, Java, machine learning. Email: [email protected]
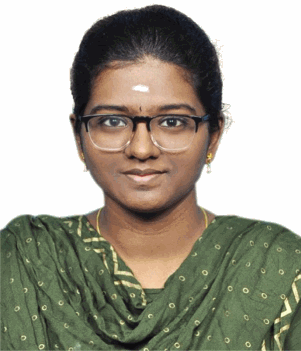
V. A. Harini
VA Harini recently completed an undergraduate B Tech degree in the department of information technology from Thiagarajar College of Engineering, Madurai, Tamil Nadu, India. Her areas of specializations are database management systems, artificial intelligence, machine learning. Email: [email protected]