Abstract
Malicious Network Traffic identification is the major problems in Internet of Things (IoT) infrastructure. From fast growth and popularization of IoT, threats and attacks in these networks are also increasing correspondingly. To detect Malicious Network Traffic on IoT surroundings, accurate scheme is significant to reduce protection risks on IoT. In this manuscript, Weibull distributive feature scaling multivariate censored extreme learning classification is proposed for Malicious IoT Network Traffic Detection (WDGMS- MCP-ELM-AD-IoT). Initially, the input datas are taken from UNSW-NB15 dataset. The datas are fed to pre-processing. During pre-processing, the redundancy eradication together with replacement of missing value is done for removing uncertainties based on local least squares. The pre-processed data is given to feature selection for choosing relevant features. The process of feature scaling is carried out by Weibull distributive generalized multidimensional scaling (WDGMS). Classification is done with the help of Multivariate Censored phi correlative Extreme learning machines classifier (MCP-ELM) depending on optimum features, which classifies normal or anomalous IoT Network Traffic. Among these, anomalous IoT Network Traffic involves fuzzers, analysis, backdoors, DoS, exploits, generic, reconnaissance, shell code, worm attacks. The proposed approach is activated in Python. The performance metrics, such as accuracy, F-Measure, precision specificity, sensitivity, and false positive rate is evaluated. The simulation of the proposed Weibull distributive feature scaling multivariate censored extreme learning classification for attack detection in IoT (WDGMS- MCP-ELM-AD-IoT) achieves better accuracy 39.45%, 34.58%, 28.29, better sensitivity 26.34%, 21.23%, 27.25%, better specificity 33.67%, 22.37%, 24.78% analyzed to the existing XGBoost-AD-IoT, DNN-AD-IoT and DRaNN-AD-IoT models respectively.
DISCLOSURE STATEMENT
No potential conflict of interest was reported by the author(s).
Additional information
Notes on contributors
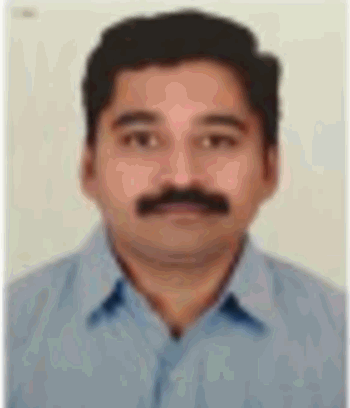
K. Sudhakar
K Sudhakar, is currently pursuing PhD in the Department of Computer Science and Engineering, University College of Engineering, Pattukkottai, Tamil Nadu, India. His research interests includes machine learning, deep learning techniques, artificial intelligence, data science etc. Reviewed and handled many research papers on latest techniques and guided many students in the same technology. Corresponding author. Email: [email protected]
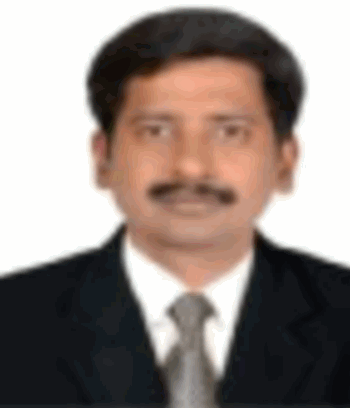
S. Senthilkumar
S Senthilkumar, currently working as assistant professor in the Department of Computer Science and Engineering, University College of Engineering, Pattukkottai, Tamil Nadu, India. He is author of several scholarly research/ review papers, including 50+ reputed and peer reviewed international journal (Scopus/SCI/UGC/IEEE/ Springer/WOS) papers with Citation index. He has won several research paper awards in different national and international conferences and symposia. He has published 1 patent and 3 books. He is member in many leading professional societies and fora. He is reviewer and editorial board member/ advisory board for many reputed/UGC approved international/ national journals and has published 4 technical books in the field of computer engineering. He delivered many keynote speeches and chaired technical sessions in many international,national conferences and Symposia. Email: [email protected]