Abstract
Medical research has focused on improving diagnosis through medical imaging in recent decades. Computer Assisted Diagnosis (CAD) systems have been developed to help doctors identify suspicious areas of interest, particularly those with cancer-like characteristics. CAD systems employ various algorithms and techniques to extract important numerical measurements from medical images that clinicians can use to evaluate patient conditions. This study proposes a statistical classification-based approach to efficient brain cancer detection. The proposed approach operates in three stages: first, Gradient Vector Flow (GVF) Snake models and mathematical morphology techniques retrieve regions of interest. The second stage characterizes these regions using morphological and textural parameters. Finally, a Bayesian network uses this description as input to identify malignant and benign cancer classes. We also compared the performance of the Bayesian network with other popular classification algorithms, including SVM, MLP, KNN, Random Forest, Decision Tree, XGBoost, LGBM, Gaussian Process, and RBF SVM. The results showed the superiority of the Bayesian network for the task of brain tumor classification. The proposed approach has been experimentally validated, with a sensitivity of 100% and a classification accuracy of over 98% for tumors, demonstrating the high efficiency of cancer cell segmentation.
Disclosure statement
No potential conflict of interest was reported by the author(s).
Additional information
Notes on contributors
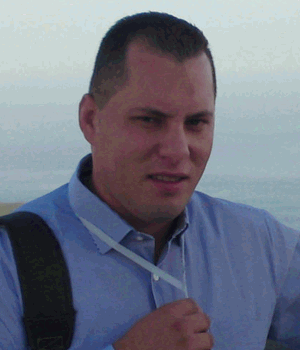
Mohamed Amine Guerroudji
Mohamed Amine Guerroudji obtained PhD degree at UMMTO, Tizi ouzou, Algeria, in 2017 and master in information processing and computer system at USDB, Blida, Algeria, in 2013. He works as a permanent researcher at CDTA with the IRVA team. main research includes: augmented and virtual reality, and computer vision. Corresponding author. Email: [email protected].
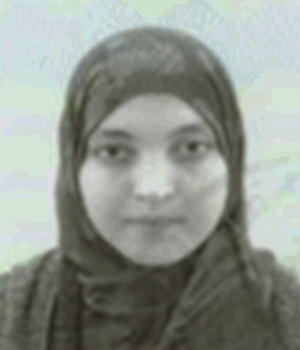
Zineb Hadjadj
Zineb Hadjadj received the PhD degree in electronic from the University of Saad Dahleb, Blida, Algeria, in 2019; and currently she is working on virtual and augmented reality at CERIST. Email: [email protected].
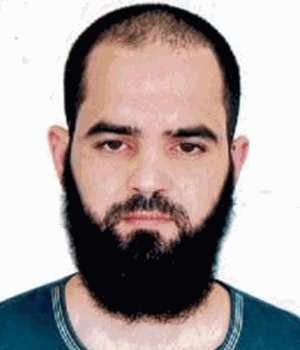
Mohamed Lichouri
Mohamed Lichouri obtained his PhD and Magister degree at USTHB, Algiers, Algeria, in 2021 and 2012, respectively in Electronics. He works as an assistant professor at USTHB University in Electronics. Email: [email protected].
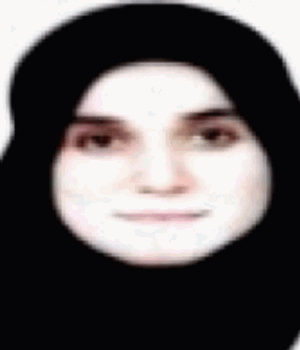
Kahina Amara
Kahina Amara obtained her PhD degree at USTHB, Algiers, Algeria, in 2018 and her master in control and robotics at USTHB in 2011. She works as a permanent researcher at CDTA with the IRVA team. Email: [email protected].
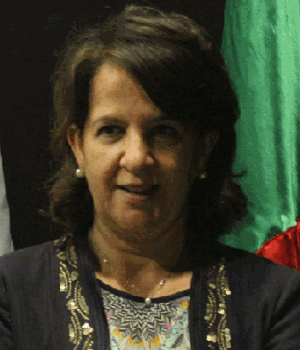
Nadia Zenati
Nadia Zenati received her PhD degree in process control and robotics from the University of Franche-Comté, France, in 2008. Now, she works as a researcher at CDTA. Email: [email protected].