Abstract
Flooding events can have significant impacts, in terms of both loss of life and property damage. Analyzing floods to understand their processes and patterns improves our ability to predict when floods will occur. Under changing climate conditions, this will become even more important. The utility of an alternative approach to conventional flood analysis was investigated in a regulated river system with a reservoir, the Nechako River Basin located in British Columbia, Canada. Using the 1955–2008 daily discharge measured on the Nechako River discharge and its major tributaries, a random forest model was constructed to evaluate the relative importance of contributing discharges to the main stem during significant flood events in the downstream community of Prince George. Discharges from the unregulated Stellako and Nautley Rivers were found to be the most important variables during a spring flood. Conversely, during a winter flood, the regulated Skins Lake Spillway and the Kemano Powerhouse discharges were found to be the most important variables in the model. During periods of no flood, the main stem Nechako River at Isle Pierre discharge was the most important. This work provides a novel source of evidence that flow regulation on the Nechako River is an important indicator of downstream winter flood conditions in Prince George, potentially exacerbating ice jams and/or causing significant property damage and economic losses.
Les inondations peuvent avoir des répercussions importantes, autant en termes de pertes de vies humaines et des dégâts matériels. Analyser les inondations afin de mieux comprendre leurs processus améliore notre capacité de prédire lorsque les inondations se produiront. Avec les changements climatiques, cela deviendra encore plus important. L'utilité d'une approche alternative à l'analyse des crues a été étudiée dans un système d’une rivière régulée avec un réservoir, le bassin versant de la rivière Nechako situé en Colombie-Britannique, Canada. Utilisant les données de débits quotidiens pour la période 1955-2008 mesurés sur la rivière Nechako et ses principaux affluents, un modèle aléatoire de forêt a été construit pour évaluer l'importance relative des systèmes qui contribuent à la rivière Nechako pendant les événements importants d'inondation dans la communauté en aval de Prince George. Les débits des rivières non-réglementées Stellako et Nautley ont été jugés les variables les plus importantes lors d'une crue printanière. En revanche, lors d'une inondation en hiver, les débits réglementés du réservoir Skins Lake et de la centrale hydroélectrique Kemano ont été jugés les variables les plus importantes dans le modèle. Pendant les périodes d'absence de crues, les débits de la rivière Nechako à Isle Pierre étaient les plus importants. Ce travail fournit une nouvelle source de preuves que la régulation des débits sur la rivière Nechako est importante indication des conditions d'inondation en hiver à Prince George, potentiellement aggravant des embâcles et/ou causant d'importants dégâts matériels et des pertes économiques.
Introduction
Flood events represent significant risks to communities located on or near river floodplains. Predictive and preventative measures are often taken to limit the damage caused by a flood (e.g. flood frequency analysis and forecasting, dikes, diversion canals, etc.), although large floods, driven by significant flow events, often still cause substantial damages. Hydrological indicators, including the timing, magnitude and duration of flow changes, provide quantitative information to evaluate long-term streamflow patterns (Monk et al. Citation2011). Rivers may also serve as reliable indicators of climatic change (e.g. Déry et al. Citation2005, Citation2011 Citation2012). Long-term trends, however, can obscure extreme flow events such as floods and therefore the associated risks. Governments, commercial interests and property owners often take little solace in the identification of decadal changes in flow regimes when extensive property loss occurs as a result of an extreme flood event on a daily time scale. It may be more useful to identify important variables that led to flood conditions and the important variables that prevailed during less destructive times.
An alternative approach to flood analysis is particularly important in systems that are highly regulated. The single largest human impact on hydrologic systems is due to the operation of regulated reservoir systems (Wisser et al. Citation2010). It is, however, sometimes difficult to separate the importance of hydrologic variables when both climatic and regulation factors are at play. Direct relationships between large-scale climatic variables and hydrological regimes are often uncertain (Whitfield and Spence Citation2011), and can be further confounded by non-random flow/reservoir regulation. For example, conventional flood frequency curves are typically assumed to follow a log-Pearson distribution with three parameters – mean, variance and skew (Dunne and Leopold Citation1978). Flood frequency is then evaluated on an n-year basis. This is an appropriate approach in an unregulated system because the underlying assumption is that floods occur on a random basis. However, in a regulated system, flooding and changes in discharge may be due to non-random changes in flow conditions, suggesting a different analysis approach may be useful. The traditional data modeling approach is to develop a relationship by devising and applying a set of parameters to an observed pattern and to make inferences based on the model (Breiman Citation2001a). Recent studies have derived a regulated flow frequency curve model to account for system specific conditions (Ergish Citation2010). These approaches, however, are still reliant upon the development of an accurate data model, which if poor or unrepresentative will likewise be a poor reproduction of the underlying process (Breiman Citation2001a).
One example of a regulated system of significant cultural, socioeconomic and ecological value is the Nechako River Basin (NRB) located in British Columbia (BC), Canada. Since its development in the early 1950s, the 922-km2 Nechako Reservoir has drastically altered the hydrology of the region, impacting local First Nations communities, migrating salmon, lotic ecosystems and downstream flood risks (Mundie and Bell-Irving Citation1986; French and Chambers 1997; Macdonald et al. Citation2012). Since then, downstream communities, such as Prince George, have experienced several destructive floods on the Nechako River in both the winter and spring (Septer Citation2007). In particular, ice jam-related floods in 1996 and 2007/2008 caused significant destruction with damages in the millions of dollars (CAD; Sui et al. Citation2010). Gerard and Davar (Citation1995) estimate an annual cost of CAD $60 million from ice jam floods in Canada. The number is likely even more significant today. Winter flooding caused by ice jams arises when the water level has to rise to overcome the flow resistance created by the ice boundary (Beltaos and Prowse Citation2001). As the ice thickens, the water level, and subsequent flooding, is even greater. Spring flood events driven by snowmelt have also contributed to the flooding problem in Prince George.
The goal of this paper is to examine a highly regulated and flood-prone area, the NRB of northern BC, using a random forest analysis. Using traditional flood recurrence intervals in an ice jam-prone area and a highly regulated watershed may be inappropriate. Ice jams have a known backwater effect that can be the dominant hydrological process during an ice jam flood event (Beltaos and Prowse Citation2001). The objective is to present an alternative modeling approach to identify which upstream rivers are most important during ice jam and spring flood events. To this end, a random forest modeling approach is used; the approach is assumption independent and results in very accurate models (Breiman Citation2001b; Cutler et al. Citation2007).
Study area
The NRB spans 52,000 km2 over ~1400 m of relief and forms the second largest sub-watershed of the Fraser River Basin (Benke and Cushing Citation2005). The eastern slopes of the Coast Mountains of western BC form the source of the headwaters for the NRB and feed the main stem Nechako River and its tributaries (Figure ). From its headwaters, the Nechako River flows northeastward toward the community of Fort Fraser, where it is joined by the Nautley River. Thereafter, the Nechako River flows eastward through Vanderhoof near the confluence with the Stuart River to finally reach its confluence with the Fraser River at Prince George. The Nechako River main stem descends from 853 m to 564 m at Prince George over a stream length of 290 km (Helm et al. Citation1980). The NRB includes several large natural bodies of water including the 254 km2 Francois, 55 km2 Fraser, 359 km2 Stuart, 247 km2 Takla and 116 km2 Trembleur lakes that can attenuate hydrologic signals in downstream rivers. The remainder of the watershed is mainly covered by forests (88%), with some agricultural lands (6%) and a few small communities (0.2%) (Benke and Cushing Citation2005). Annual air temperature in the NRB averages 3.7°C, while mean annual precipitation totals 601 mm with about a third in the form of snow (Danard and Murty Citation1994; Benke and Cushing Citation2005).
Figure 1. Map of the Nechako River Basin. All stations used in this analysis are presented in this figure. The red outline denotes the area upstream of the Kenney Dam and impacted by the Kemano project.
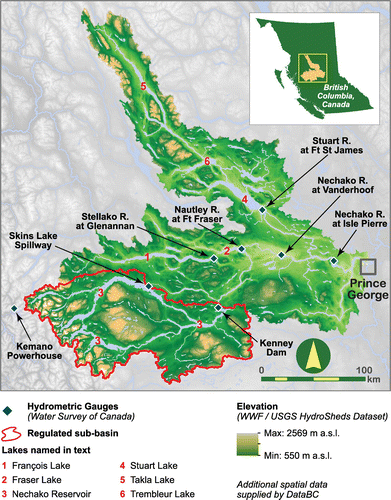
In 1949, the BC provincial government approved what would become the Kemano development, in the upper reaches of the NRB. The Aluminum Company of Canada (ALCAN) was granted a “Conditional Water License” in 1950, paving the way for the construction of the Kenney Dam, the Nechako Reservoir and the Kemano Powerhouse (Hartman Citation1996). Regions in the southwestern part of the basin below 853 m in elevation were all flooded as a result of the dam construction (Helm et al. Citation1980). In 1954, a 16-km tunnel was drilled through the Coast Mountains that would connect westward-diverted water from the Nechako Reservoir through to the Kemano Powerhouse. Hydroelectricity generated at the Kemano Powerhouse then powers an aluminum smelter in Kitimat, with the surplus being transferred to the province-wide BC Hydro grid (Mundie and Bell-Irving Citation1986; Hartman Citation1996). Kenney Dam diverted 15,600 km2 of the southern portion of the NRB, largely flooding the Ootsa, Tahtsa, Tetachuck and Whitesail Rivers in creating the 32.7-km3 Nechako Reservoir (Schiefer and Klinkenberg Citation2004). A spillway was constructed at Skins Lake to manage reservoir levels and flow into the Cheslatta and Nechako Rivers (Northwest Hydraulic Consultants [NHC] Citation2003). The criterion for water release from the spillway has been modified over time such that, since 1980, the regime currently manages for salmon conservation values and also minimum flows from September to June. During periods when the reservoir is near capacity, significant volumes of water are released via the spillway (NHC Citation2003; Macdonald et al. Citation2012). After the creation of the reservoir, the Nechako River and all downstream points in the basin have exhibited evidence of regulated flow and separation from a natural nival regime (NHC, Citation2003; Macdonald et al. Citation2012).
Materials and methods
Hydrometeorology of the Nechako Basin
Hydrometric data
NRB daily mean river discharge measurements were extracted from the Water Survey of Canada's hydrometric database (HYDAT). The criterion for gauge record selection was upstream of and including the Nechako River at Isle Pierre near Prince George. An additional criterion included a continuous record of observations from 1955 to 2008. An initial query to HYDAT extracted 20 gauges upstream of the Isle Pierre gauge within the Nechako system. Six gauges possessing continuous data sets from 1 January 1955 to 31 December 2008 were subsetted from this initial query to form the test data set (Table ). Among these, the Stellako, Nautley and Stuart rivers are streamflow records not influenced by regulation. The sole gauge chosen outside the Nechako system was at Kemano Powerhouse, because of its importance to the reservoir. That gauged drainage is significant because it receives water from the Nechako Reservoir influencing the need for regulation of the Skins Lake Spillway discharge, and thus is plausibly an important variable for the hydrology of the NRB.
Table 1. Gauging stations metadata selected for analysis, and annual streamflow statistics (1955–2008). Coefficient of variation (CV) is computed as the ratio of the standard deviation (SD) to the mean.
Flood date identification
The identification of flooding dates was subject to two criteria. First, the flood needed to be in the vicinity of Prince George and caused by the Nechako River (rather than the Fraser River at Prince George). Second, the flood needed to be severe enough (in terms of property damage, evacuations, etc.) to merit media coverage. Media coverage may not identify all significant floods upstream or downstream of Prince George and thus represents an incomplete record. Nevertheless, floods most important to humans are those that impact daily life. Therefore, it is assumed that this approach captures only the most important floods. This approach forced a focus mostly on the peak-of-flood date, limiting the ability to characterize the entire flood event. Septer (Citation2007) compiled a comprehensive list of flooding events in northern BC from 1820 to 2006. This record is drawn from newspaper articles and recounts the location, the dates and damage caused by the floods. Post-2006 flood records were drawn also from newspaper articles, although these dates and locations were compiled by the present authors (Table ).
Table 2. Dates of flooding in Prince George. Flooding dates based on Septer (Citation2007) and newspaper articles for post-2006 flood events.
Regional precipitation influence
The Nautley River and the Skins Lake Spillway gauges were selected to compare precipitation and discharge data to explore the hydrologic connectivity in one highly regulated site and one unregulated site (Figure ). Meteorological data were extracted online from Environment Canada (Fraser Lake, station ID 109C0LF, and Skins Lake Spillway, station ID 1085836) and compared using Spearman’s rank correlation coefficient. Total daily precipitation and daily discharge were used to evaluate relationships between regional climatic factors and flow patterns.
Random forest model
Water transport calculations
Water transport lag times from individual gauging stations to the Nechako River at Isle Pierre station were calculated using a correlation method. The maximum correlation coefficient between daily discharge measurements at Isle Pierre and a range of daily lag values (0 to 30 days) on discharge for station i was indicative of a transport time at the corresponding lag value. The Kemano Powerhouse was omitted from the lag analysis because the water re-directed to it never reaches the Isle Pierre gauging station despite its indirect influence on the Nechako flow. Spearman's rank correlation test was used to evaluate the strength of lag values. The strongest significant correlation of lag values was taken as the correct lag value.
Random forest background
Random forests (Breiman Citation2001b) represent an alternative to traditional data modeling approaches. Instead of fitting data to a formulated model, random forest is an algorithmic or “black box” approach (Breiman Citation2001a). The familiar elements of this black box are that a set of predictor values is related to a set of response values (Breiman Citation2001a). Computationally intensive, a random forest uses a series of classification trees to evaluate variable importance. The black box approach assumes that systems will generate data in a manner that is too complex and will be inherently beyond the range of human understanding.
Random forests are particularly useful in classification problems where the response variable emanating from the black box is limited to a relatively small number (< 32) of categories. In the context of NRB’s hydrology, the response variable “occurrence of flooding” can be reduced to a binary set of categories “flooding or no flooding”. A random forest analysis then allows for the assessment of the importance of observed variables (Table ) submitted to the black box on the response variables (flooding or no flooding). Regulated hydrological zones that have experienced flooding represent suitable circumstances for the random forest approach. Attempting to define a parametric model that accurately captures the dual complexity of natural and regulated hydrologic systems and the interaction between the two is extremely difficult and problematic.
Random forest training
The goal of a random forest is to explain a categorical response variable using a set of explanatory (or predictor) variables by fitting a predictor–response model to many classification trees. A classification approach to random forests was used because of the discrete nature of flood events. The model used to fit the random forest included the flood status for a given date as a response variable, and the discharge from all stations that satisfied the above criteria and was corrected for lag effects as the predictors (Table ). Each tree was independently created by a bootstrap sample of the larger data set. Each class of a set of observations was predicted for each tree. Trees were then combined by a simple majority vote of the predictions by the trees. In a random forest at each node in a classification tree, a small number of randomly selected variables are used to determine the best split at that node. The error estimate (out-of-bag error) of a random forest consists of predicting the data that were not bootstrap sampled, using the tree grown with that sample. The only two input values required to implement the random forest model are the number of trees (ntree) and the number of variables available to be randomly chosen when splitting at a node (mtry). We used the suggested integer values of the square root of the number of variables (Liaw and Weiner Citation2002) for node splitting classification (mtry = 3), and set the number of trees at 5000. Because the number of samples was so skewed towards the number of observations with flood days, the number of bootstrap samples was reduced to 320 for the “no-flooding” class.
Statistical analysis
All random forest analyses were performed using the R 2.14.2 (R Foundation for Statistical Computing Citation2011) statistical package with the randomForest package (Liaw and Wiener Citation2002) to implement the random forest model. All graphics were created using the ggplot2 package (Wickham Citation2009).
Results
Hydrometeorology of the NRB
Flooding events
Flood events occurred throughout the study period, although the most significant winter flood events have occurred in the past 20 years (1996, 2007/2008; Table ). The monthly Nechako discharge during the 1997 floods (320 m3/s) was 2.5 times the average Nechako discharge (124 m3/s) under the current salmon water management regime over the same month (November 1980–2008). Similarly, the floods in December of 2007 saw approximately the same discharge (267 m3/s) increased over the same average discharge period (108 m3/s). These two events caused considerable property damage in Prince George and were only eventually mitigated by natural conditions. Spring flood events (occasionally stretching into summer) have also occurred, although these events did not cause the same level of damage as the winter floods.
Discharge patterns
Driving flooding events in the NRB are the rivers that met the selection criteria (see section on Hydrometric data), shown in Table . All unregulated rivers (Table ) exhibit strongly nival (unimodal) patterns of discharge (Figure ). The northernmost gauge (Stuart River) reaches maximum flows later in the year than either the Stellako or Nautley Rivers. In contrast, the Nechako River gauges at Isle Pierre and Vanderhoof exhibit variable (multimodal) patterns of peak flows resulting from their location downstream of the Skins Lake Spillway (Figure ). Annual streamflow trends fell into three categories (Table ).
Figure 2. The 1955–2008 hydrologic regimes of the seven selected gauging stations. The Stuart, Nautley and Stellako Rivers exhibit strongly nival regimes while the Nechako River hydrograph demonstrates the strong influence of upstream regulation. Both the Skins Lake Spillway and the Kemano Powerhouse irregular hydrographs are consistent with highly regulated flows.
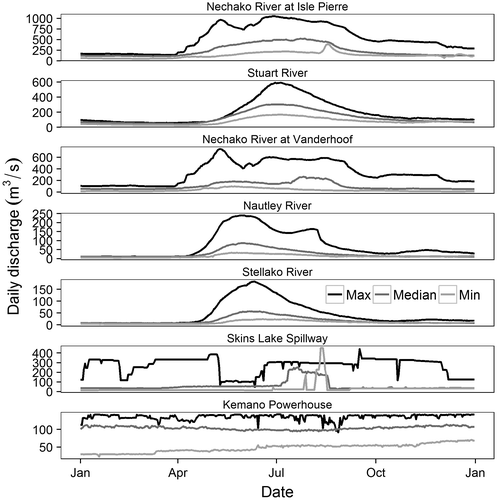
Regional precipitation
Precipitation is not correlated to discharge because of the highly non-linear and lagged relation between rainfall and streamflow. For example, the Nautley discharge was weakly but significantly correlated to total precipitation. In contrast, the Skins Lake Spillway discharge had no significant correlation to regional precipitation patterns (Figure ). Other measures of precipitation (e.g. snow water equivalent measured by snow pillows) were also weakly correlated against discharge and therefore are not presented here.
Random forest model
Model performance assessment
Model performance was assessed using the out-of-bag error. The out-of-bag error aggregates, for each decision tree, observations that are not trained, resulting in no bias for the assessment (Breiman Citation2001b). The out-of-bag error stabilized after 750 trees, resulting in a 0.01095 error rate. This is an unbiased demonstration of good model performance as well as an additional indicator that the model was trained on a suitable number of trees.
Sensitivity analysis
A sensitivity analysis was conducted on the random forest model to evaluate changes in variable importance by excluding the most important variable for each flooding state. For example, for the classification of winter ice jam-related flooding, the Skins Lake Spillway was excluded from the data set and then the model was re-run. There was minimal change in the importance rankings (i.e. some rivers changing rankings by one) and no impact on the interpretation of the model. When the input variable (ntree and mtry) changed iteratively, importance values and error estimates remained mostly stable indicating the model was not sensitive to the lone user inputs. Similar to the above, changing the number of trees occasionally swapped the Stuart River and Kemano Powerhouse rankings in the spring freshet flooding (Figure ). This change had no impact on the interpretation of the model and therefore should not detract from the model. Based on previous favourable validations and comparisons of random forest models in Cutler et al. (Citation2007), the model was not further validated.
Figure 4. Variable importance as estimated by a 5000 tree random forest. The model trained on this random forest included upstream discharge predictors of flooding in Prince George, BC. During spring floods the most important variables in the data models were the Stellako and Nautley Rivers. In contrast, the most important variables during winter floods were Skins Lake Spillway and Kemano Powerhouse discharge. In the absence of floods, the most important predictor was simply the main stem Nechako River discharge at Isle Pierre. Values should not be compared amongst panels but instead within panels. The absolute value on the vertical axis is only relevant as an intra-panel comparison.
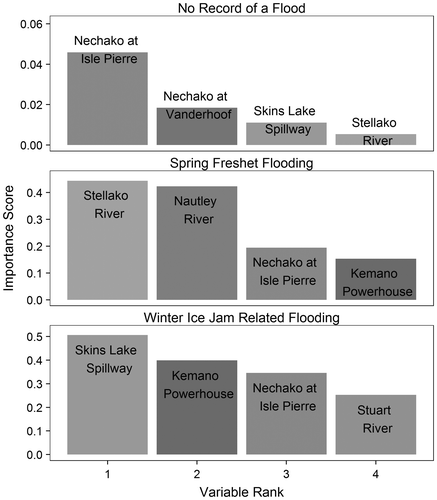
Water transport times
Input variables for the random forest model were lag-corrected discharge values using the results from Figure . Stuart River flows were most highly correlated with those at Isle Pierre with a lag time of zero, indicating that water took less than a day to travel from the Stuart gauge to the one at Isle Pierre. Water took slightly longer to travel from Vanderhoof to Isle Pierre (2 days). Because the Stellako River is nested within the Nautley system, it was assumed that the zero value found for the Stellako was indicative of the hydrologic disconnect between Isle Pierre and the Stellako River. Therefore, for subsequent analysis, a lag value of 6 days, derived from the Nautley River gauge, was used. The Skins Lake Spillway correlation value was maximized at a lag value of 7 days.
Figure 5. Correlation between a range of lagged (0–30 days) daily discharge values of individual gauges and the discharge of the Nechako River at Isle Pierre gauge over 1955–2008. Since the Stellako River is nested within the Nautley system, it was assumed that the lag time associated with the Nautley River was equivalent to the Stellako River and that the calculated lag time of the Stellako River (lag = 0) was a product of that nesting. Maximum values are labeled by the corresponding integer.
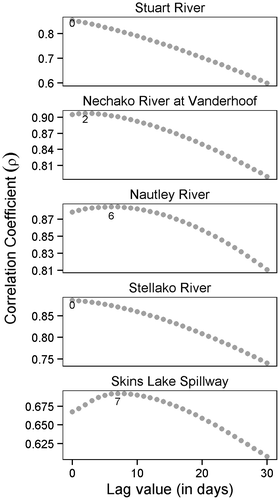
Random forest variable importance
The results of the random forest training were divided into the three flood categories, and variable (gauge) importance can be assessed on a relative basis within each category. During periods of no recorded flooding, the most important variable was the discharge from the Nechako River at the Isle Pierre gauge. Further, this variable was substantially more important than the second most important variable (Figure ). During periods of spring flood, flows from the Stellako and Nautley Rivers become more important. Winter flooding is influenced mostly by the Skins Lake Spillway, with the Kemano Powerhouse gauge being the second most important variable in the model. The presence of the Nechako River at Isle Pierre and Stuart River variables in third and fourth positions, respectively, reveals the complex feedback within the system as well as the large natural contribution of the Stuart River to the overall flows of the Nechako River at Isle Pierre (Figure ).
Figure 6. The contribution of the three major upstream watersheds to the Nechako River at Isle Pierre discharge. The figure highlights that most of the discharge in the basin is driven by the selected variables. Average individual drainage values for the Skins Lake Spillway, the Stuart River and the Nautley River are respectively 32.8, 50.5 and 11.3%. Values exceeding 100% are a single-year aberration and are likely due to instrument accuracy issues.
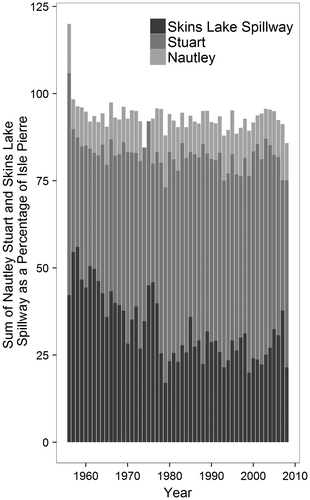
Discussion
To the authors’ knowledge, this is the first application of a random forest approach to evaluating flood events in a regulated watershed. This study demonstrates, as one might expect, that the NRB spring snowmelt floods are driven by high flows in the unregulated Stuart and Nautley Rivers (Figure ). In contrast, the NRB winter ice jam floods appear to be influenced by water releases from the Nechako Reservoir. While daily discharge at the Kemano Powerhouse is variable, there is a consistency over time in the attempts by Alcan (now Rio Tinto Alcan) to provide a continuous supply of hydroelectricity for the aluminum smelter in Kitimat. Releases of water at the Skins Lake Spillway, however, can vary substantially over time, and are largely determined by the level of the Nechako Reservoir and the judgment of the spillway operators. Data from an Environment Canada meteorological station on the north shore of Fraser Lake near the center of the NRB reveal that precipitation in 2007 was exceptionally high, with unusually abundant snowfall and rainfall (not shown). This then led to the release of large amounts of water from the reservoir during the summer and into the fall of 2007 and the winter of 2008 that exacerbated the ice jam flood on the lower reaches of the Nechako River (Figure ). Wintertime water releases from the Nechako Reservoir are to prevent excessive water levels in spring as they compromise reservoir safety; however, these artificial water releases may then lead to flooding at downstream locations such as Prince George, whereas in the past the high precipitation may simply have been stored in the seasonal snowpack for release, perhaps at a slower pace, later in the hydrological year.
Figure 7. Multi-year discharge patterns (2005–2008) for four selected gauging stations within the Nechako River Basin.
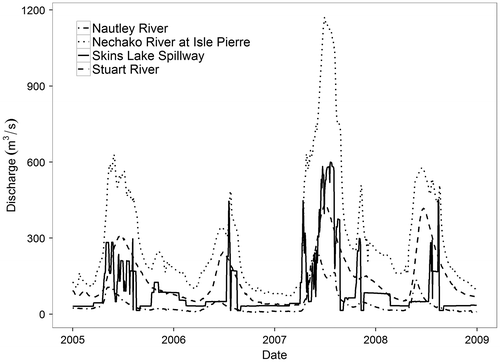
Global and regional climate model simulations suggest that the Fraser River will transition from a nival to a more pluvial regime by the end of the twenty-first century (Morrison et al. Citation2002; Kerkhoven and Gan Citation2011; Poitras et al. Citation2011; Shrestha et al. Citation2012; Kang et al. Citation2014), and will experience more frequent great flood events (Milly et al. Citation2002). These simulations also project the Fraser River Basin, including the NRB, will experience more wintertime precipitation, in the form of both rain and snow. Increasing wintertime precipitation over the NRB suggests the potential for larger and more frequent releases of water from the Nechako Reservoir. This could therefore exacerbate wintertime flood events on the lower reaches of the Nechako River, particularly in the presence of ice jams.
Conclusion
This study examined the 1955–2008 flood events on the Nechako River near Prince George, BC, using a novel application of a random forest model. Events were stratified as winter floods associated with ice jams, snowmelt-driven spring floods, or no floods. The random forest model was then applied to seven hydrometric gauges associated with the NRB including three unregulated tributaries of the Nechako River, two on the river’s main stem and two near flow regulation facilities. Results show that spring floods were influenced mainly by the Stellako and Nautley Rivers, two unregulated tributaries of the Nechako River. Winter floods, in contrast, demonstrated an influence of the regulated Skins Lake Spillway discharge and operations at the Kemano Powerhouse. In the absence of floods, discharge on the main stem Nechako River at Isle Pierre was most important. This study illustrates how seasonal flood events in a highly regulated watershed may be driven by various processes (ice jams, snowmelt, etc.) and by different, upstream portions of a basin. It also demonstrates that operations to control flows on a large reservoir may, in fact, exacerbate downstream flood events depending on management priorities and the timing of water releases. Climate change is projected to result in warmer, wetter conditions in the NRB during the twenty-first century, particularly in winter. Future work should therefore identify the causes of and changes in water releases from the Nechako Reservoir and their potential contributions to winter floods on the Nechako River in the context of climate change. Classification, particularly machine learning options such as random forest analysis, represents exciting new methods of analysis to evaluate hydrology in the context of salmon management strategies and future climate scenarios.
Acknowledgments
Hydrometric data for this paper are available from Environment Canada’s HYDAT database, whereas climate data are available from its Canadian Historical Climate database. Thanks to Phil Owens, Margot Parkes, Carla Mellott, Kara Przeczek and Marco Hernández-Henríquez (UNBC) as well as Lynne Campo (Environment Canada) for assistance with this work. Thanks to Michael Allchin (UNBC) for assistance with the Nechako River basin map. Funding provided by the government of Canada’s CRC program to SJD, NSERC Discovery Grants to SJD and ELP, and the Real Estate Foundation of BC.
References
- Atkinson, C. 2007. Flooded homes, businesses evacuated in Prince George. Globe and Mail. 13 December, S1.
- Atkinson, C. 2008. Warm weather, water start to melt ice jam. Globe and Mail. 7 February, S1.
- Austin, I., and G. Luymes. 2007. Rising rivers wreak havoc: Eight communities declare emergencies, with more alerts coming today. Times–Colonist. 8 June, A3.
- Beltaos, S., and T. D. Prowse. 2001. Climate impacts on extreme ice-jam events in Canadian rivers. Hydrological Sciences Journal 46: 157–181. doi:10.1080/02626660109492807.
- Benke, A. C., and C. E. Cushing (eds.). 2005. Rivers of North America. New York: Elsevier.
- Breiman, L. 2001a. Statistical modeling: The two cultures. Statistical Sciences 16: 199–231.
- Breiman, L. 2001b. Random forests. Machine Learning 45: 5–32. doi:10.1023/A:1010933404324.
- Cutler, D. R., T. C. Edwards, K. H. Beard, A. Cutler, K. T. Hess, J. Gibson, and J. J. Lawler. 2007. Random forest for classification in ecology. Ecology 88: 2783–2792. doi:10.1890/07-0539.1.
- Danard, M., and T. S. Murty. 1994. On recent climate trends in selected salmon-hatching areas of British Columbia. Journal of Climate 7(11): 1803–1812.
- Déry, S. J., M. A. Hernández-Henríquez, P. N. Owens, M. W. Parkes, and E. L. Petticrew. 2012. A century of hydrological variability and trends in the Fraser River Basin. Environmental Research Letters 7: 024019. doi:10.1088/1748-9326/7/2/024019.
- Déry, S. J., T. J. Mlynowski, M. A. Hernández-Henríquez, and F. Straneo. 2011. Interannual variability and interdecadal trends in Hudson Bay streamflow. Journal of Marine Systems 88: 341–351. doi:10.1016/j.jmarsys.2010.12.002.
- Déry, S. J., M. Stieglitz, E. C. McKenna, and E. F. Wood. 2005. Characteristics and trends of river discharge into Hudson, James, and Ungava Bays, 1964–2000. Journal of Climate 18: 2540–2557. doi:10.1175/JCLI3440.1.
- Dunne, T., and L. Leopold. 1978. Water in environmental planning. W.H Freeman and Company. San Francisco, CA.
- Ergish, N. J. 2010. Flood frequency analysis for regulated watersheds, MSc thesis, University of California, Davis.
- French, T. D., and P. A. Chambers. 1997. Reducing flows in the Nechako River (British Columbia, Canada): Potential response of the macrophyte community. Canadian Journal of Fisheries and Aquatic Sciences 54: 2247–2254.
- Gerard, R., and K. S. Davar. 1995. Introduction. Chapter 1 in: River Ice Joins, ed. S. Beltaos, Highlands Ranch, CO: Water Resources.
- Hartman, G. F. 1996. Impacts of growth in resource use and human population on the Nechako River: A major tributary of the Fraser River, British Columbia, Canada. Geojournal 40: 147–164. doi:10.1007/BF00222540.
- Helm, R., D. MacDonald, B. Sinclair, D. Chan, T. Harrington, A. Chalmers, and B. G. Shepherd. 1980. A review of the Nechako River Watershed. Internal Report. Fisheries and Oceans Canada. Vancouver, BC.
- Ivens, A. 2007. Levels of most rivers keep falling; there's optimism scores of communities will escape serious flooding, The Province. 11 June, A4.
- Kang, D. H., X. Shi, H. Gao, and S. J. Déry. 2014. On the changing contribution of snow to the hydrology of the Fraser River Basin. Journal of Hydrometeorology 15: 1344–1365.
- Kerkhoven, E., and T. Gan. 2011. Differences and sensitivities in potential hydrologic impact of climate change to regional-scale Athabasca and Fraser River basins of the leeward and windward sides of the Canadian Rocky Mountains respectively. Climatic Change 106 (4): 583–607. doi: 10.1007s10584-010-9958-7.
- Liaw, A., and M. Wiener. 2002. Classification and regression by randomForest. R News 2: 18–22.
- Macdonald, J. S., J. Morrison, and D. A. Patterson. 2012. The efficacy of reservoir flow regulation for cooling migration temperature for sockeye salmon in the Nechako River watershed of British Columbia. North American Journal of Fisheries Management 32: 415–527. doi:10.1080/02755947.2012.675946.
- Milly, P. C. D., R. T. Wetherald, K. A. Dunne, and T. L. Delworth. 2002. Increasing risk of great floods in a changing climate. Nature 415: 514–517. doi:10.1038/415514a.
- Monk, W. A., D. L. Peters, R. A. Curry, and D. J. Baird. 2011. Quantifying trends in indicator hydroecological variables for regime-based groups of Canadian rivers. Hydrological Processes 25: 3086–3100. doi:10.1002/hyp.8137.
- Morrison, J., M. C. Quick, and M. G. G. Foreman. 2002. Climate change in the Fraser River watershed: Flow and temperature projections. Journal of Hydrology 263(1): 230–244. doi:10.1016/S0022-1694(02)00065-3.
- Mundie, J. H., and R. Bell-Irving. 1986. Predictability of the consequences of the Kemano Hydroelectric proposal for natural salmon populations. Canadian Water Resources Journal 11: 14–25.
- Northwest Hydraulic Consultants (NHC). 2003. Nechako River geomorphic assessment Phase I: Historical analysis of the lower Nechako River. Prepared for the British Columbia Ministry of Water, Land and Air Protection. 31 pp. + app. Victoria, B.C.
- Poitras, V., L. Sushama, F. Seglenieks, M. N. Khaliq, and E. Soulis. 2011. Projected changes to streamflow characteristics over western Canada as simulated by the Canadian RCM. Journal of Hydrometeorology 12: 1395–1413. doi:10.1007/s10584-010-9958-7.
- R Foundation for Statistical Computing. 2011. R 2.14: A language and environment for statistical computing. Vienna, Austria: R Foundation for Statistical Computing. http://www.R-project.org
- Rolfsen, C. 2007. Prince George braces for flooding; a rapidly rising Fraser River also has the Lower Mainland on alert. The Vancouver Sun. 2 June, B2.
- Schiefer, E., and B. Klinkenberg. 2004. The distribution and morphometry of lakes and reservoirs in British Columbia: A provincial inventory. Canadian Geographer 48: 345–355. doi:10.1111/j.0008-3658.2004.00064.x.
- Septer, D. 2007. Flooding and landslide events Northern British Columbia 1820–2006. Ministry of Environment, Province of British Columbia. http://www.env.gov.bc.ca/wsd/public_safety/flood/pdfs_word/floods_landslides_north.pdf (accessed May, 2014).
- Shrestha, R. R., M. A. Schnorbus, A. T. Werner, and A. J. Berland. 2012. Modelling spatial and temporal variability of hydrological impacts of climate change in the Fraser River basin, British Columbia, Canada. Hydrological Processes 26: 1840–1860. doi:10.1002/hyp.9446.
- Sui, J., J. Wang, Y. He, and F. Krol. 2010. Velocity profiles and incipient motion of frazil particles under ice cover. International Journal of Sediment Research 25: 39–51. doi:10.1016/S1001-6279(10)60026-1.
- Whitfield, P. H., and C. Spence. 2011. Estimates of Canadian Pacific Coast runoff from observed streamflow data. Journal of Hydrology 410: 141–149. doi:10.1016/j.jhydrol.2011.05.057.
- Wickham, H. 2009. ggplot2: Elegant graphics for data analysis. New York: Springer. http://had.co.nz/ggplot2/book
- Wisser, D., B. M. Fekete, C. J. Vörösmarty, and A. H. Shumann. 2010. Reconstructing 20th century global hydrography: a contribution to the Global Terrestrial Network- Hydrology (GTN-H). Hydrology and Earth System Sciences 14: 1–24. doi:10.5194/hess-14-1-2010.