Abstract
Excessive phosphorus (TP) and nitrogen (TN) inputs from the Red–Assiniboine River Basin (RARB) have been linked to eutrophication of Lake Winnipeg; therefore, it is important for the management of water resources to understand where and from what sources these nutrients originate. The RARB straddles the Canada–United States border and includes portions of two provinces and three states. This study represents the first binationally focused application of SPAtially Referenced Regressions on Watershed attributes (SPARROW) models to estimate loads and sources of TP and TN by jurisdiction and basin at multiple spatial scales. Major hurdles overcome to develop these models included: (1) harmonization of geospatial data sets, particularly construction of a contiguous stream network; and (2) use of novel calibration steps to accommodate limitations in spatial variability across the model extent and in the number of calibration sites. Using nutrient inputs for a 2002 base year, a RARB TP SPARROW model was calibrated that included inputs from agriculture, forests and wetlands, wastewater treatment plants (WWTPs) and stream channels, and a TN model was calibrated that included inputs from agriculture, WWTPs and atmospheric deposition. At the RARB outlet, downstream from Winnipeg, Manitoba, the majority of the delivered TP and TN came from the Red River Basin (90%), followed by the Upper Assiniboine River and Souris River basins. Agriculture was the single most important TP and TN source for each major basin, province and state. In general, stream channels (historically deposited nutrients and from bank erosion) were the second most important source of TP. Performance metrics for the RARB SPARROW model are similarly robust compared to other, larger US SPARROW models making it a potentially useful tool to address questions of where nutrients originate and their relative contributions to loads delivered to Lake Winnipeg.
Les apports excessifs en phosphore (PT) et en azote (AT) en provenance des bassins de la rivière Rouge et de la rivière Assiniboine (BRRA) ont été liés à l’eutrophisation du lac Winnipeg; il est par conséquent important pour la gestion de comprendre où et de quelles sources proviennent les apports. Les BRRA chevauchent la frontière canado-américaine et englobent partiellement deux provinces et trois États. La présente étude représente la première application binationale des modèles SPARROW (SPAtially Referenced Regressions On Watershed attributes, ou régressions par coordonnées spatiales appliquées aux bassins versants) pour estimer les charges et les sources de PT et d’AT de chaque territoire et bassin versant à de multiples échelles spatiales. Des obstacles majeurs ont dû être contournés pour mettre au point ces modèles, dont: (1) l’harmonisation d’ensembles de données géospatiales, en particulier la construction d’un réseau hydrographique contigu; et (2) l’utilisation de nouvelles étapes d’étalonnage pour surmonter les limites de la variabilité spatiale de l’étendue du modèle et du nombre de sites d’étalonnage. En utilisant des apports d’éléments nutritifs sur la base de l’année de référence 2002, un modèle SPARROW pour le PT dans les BRRA a été étalonné avec les apports de l’agriculture, les forêts et les milieux humides, les stations d’épuration des eaux usées (SEEU) et les chenaux de cours d’eau, et un modèle pour l’AT a été étalonné avec les apports de l’agriculture, les SEEU sources ponctuelles et le dépôt atmosphérique. À la décharge des BRRA, en aval de Winnipeg (Manitoba), la majorité du PT et de l’AT provenaient du bassin versant de la rivière Rouge (90 %), suivi du bassin versant du cours supérieur de la rivière Assiniboine et de la rivière Souris. L’agriculture était la source la plus importante de PT et d’AT pour chaque bassin versant majeur, province ou État. En général, les chenaux de cours d’eau (éléments nutritifs accumulés au fil du temps et par l’érosion des rives) étaient la deuxième plus importante source de PT. Les paramètres de performance pour le modèle SPARROW pour les BRRA sont aussi robustes que ceux des modèles de plus grande échelle SPARROW pour les États-Unis, ce qui en fait un outil potentiellement utile pour répondre aux questions sur le lieu d’origine des éléments nutritifs et sur leurs contributions relatives aux charges apportées dans le lac Winnipeg.
Introduction
The Red—Assiniboine River Basin (RARB) spans portions of Canada (Manitoba and Saskatchewan) and the United States (US, North Dakota, South Dakota and Minnesota) (Figure ). Via the Red River, this international basin discharges into Lake Winnipeg, a lake that has experienced a proliferation of severe algal blooms and release of cyanotoxins over recent decades (Kling et al. Citation2011; McCullough et al. Citation2012). The putative cause of eutrophication in prairie environments is excessive loading of nutrients (namely phosphorus and nitrogen) from streams, which are eventually routed into larger rivers and downstream receiving waters (Schindler et al. Citation2012). To most effectively reduce nutrient loading, it would be useful to understand from where and from what sources this loading originates.
Figure 1. Spatial domain of the Red—Assiniboine River Basin (RARB) SPARROW total phosphorus (TP) and total nitrogen (TN) models.
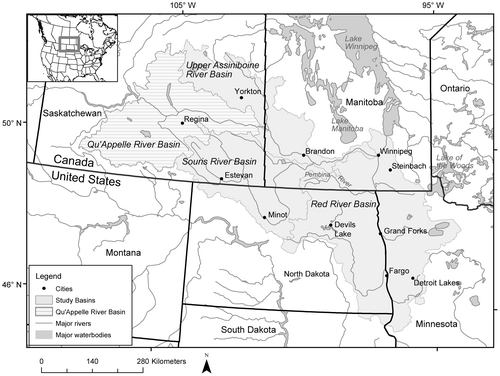
From the 1960s until the early 1990s, midsummer total phosphorus (TP) concentrations in Lake Winnipeg were relatively stable between 20 and 30 μg/L; however, over the past 20 years, the concentrations have almost doubled (McCullough et al. Citation2012). During this time, TP concentrations in the Red River have increased by about 20% and annual loads have increased by about 130% as a result of higher flows (McCullough et al. Citation2012). The southern portion of the lake suffers from algal blooms that have degraded the aquatic food web structure, negatively affected tourism and recreation through fouled beaches and infested nearshore zones, and threatened drinking water supplies for coastal communities. Two of the dominant anthropogenic factors considered responsible for the condition of the lake are the intensification of agriculture, through increased livestock production and use of synthetic fertilizers, and an increase in the frequency and intensity of spring floods as a result of climate change and altered prairie hydrology (Schindler et al. Citation2012).
Remediation of eutrophication and its symptoms requires reduction of nutrients from surface waters, but not all parts of most watersheds and sources of nutrients contribute equally (University of Wisconsin-Madison, College of Agricultural and Life Sciences [UW-CALS] Citation2005; Robertson et al. Citation2014). To support the development of water quality objectives and nutrient management policies, credible estimates of the distribution of nutrient loads from across the RARB are needed as well as robust methods for apportionment of nutrient sources. For Lake Winnipeg as a whole, the average annual TP load for the 1999 to 2007 period was estimated to be 7660 metric tonnes (MT) and the average annual total nitrogen (TN) load for the 1994 to 2007 period was 90,700 MT (Armstrong and McCullough Citation2011). It was further estimated that 68% (5380 MT) of the annual TP load and 34% (31,500 MT) of the annual TN load to the lake comes from the RARB. Upstream of the confluence of the Red and Assiniboine rivers in the City of Winnipeg, it was estimated that 68% of the TP and 70% of the TN to Lake Winnipeg comes from the Red River and 14% of the TP and 12% of the TN comes from the Assiniboine River, with the remainder entering the system between Winnipeg and the outlet (Armstrong and McCullough Citation2011).
For the portion of the Red River Basin just upstream from the City of Winnipeg, Armstrong and McCullough (Citation2011) estimated that 70% of the TP and 78% of the TN comes from south of the Canada–US border. Smaller amounts of TP and TN come from the Souris River system, which spans portions of Saskatchewan, North Dakota and Manitoba, as it traverses the border before it joins the Assiniboine River in southwestern Manitoba. These load estimates reflect nutrient contributions from key areas in the river network, but to develop policy recommendations, better spatial resolution is needed as well as a basin-wide perspective to understand the nutrient sources and processes that affect their delivery. In a transboundary context, this involves the attribution of nutrient sources by jurisdiction and by basin.
Water quality modelling represents a method by which nutrient loads can be related to measured and mapped attributes of a watershed or landscape. Modelling results can facilitate spatial targeting of nutrient abatement strategies and beneficial management practices (BMPs) to more efficiently reduce loads. Monitoring and modelling studies within the RARB have identified specific nutrient sources and biogeochemical processes that have impaired local water quality and degraded downstream aquatic ecosystems (Li et al. Citation2011; Shrestha et al. Citation2012; Yang et al. Citation2014). A challenge in aquatic landscape ecology is the interpretation of local studies at larger spatial scales due to variation in climate, physiography, soils, vegetative cover and the morphology and morphometry of rivers, reservoirs and lakes. For regional or larger spatial extents, regression-based empirical models have typically been used to predict regional variation in water quality (Mueller et al. Citation1997). However, simple regression models tend to suffer from several weaknesses, such as: (1) the lack of information on where long-term storage/removal of nutrients occurs during downstream transport, (2) the lack of separation between processes that occur on land and those that occur in streams, and (3) difficulty separating the effects of individual variables because they may act as surrogates and potentially mask the actual sources (Robertson and Saad Citation2011).
To better understand and effectively manage nutrient loading from a multi-jurisdictional and binational system the size of the RARB, analytical tools are needed that can handle complex hydrological networks, geographical heterogeneity and water quality data that vary spatially and temporally. To address these issues, the SPARROW (SPAtially Referenced Regressions On Watershed attributes) model developed by the United States Geological Survey (USGS) was used to simulate TP and TN loads from throughout the RARB and identify and quantify key sources and processes that may account for variation in loads across the basin. SPARROW uses a process-based, mass-balance approach built on a spatially defined digital network of streams and reservoirs to track the attenuation of nutrients during their downstream transport from each source, and is calibrated using various statistical techniques (Schwarz et al. Citation2006).
SPARROW has been applied extensively in large basins in the US (Alexander et al. Citation2008; Preston et al. Citation2011) and New Zealand (Elliot et al. Citation2005). US portions of the Red and Assiniboine river basins were previously included in other SPARROW applications, including those at the national (i.e. US) scale representing nutrient inputs in the late 1980s (Smith et al. Citation1997) and in the early 1990s (Alexander et al. Citation2008), and regional scales of the Upper Midwest part of the US (referred to as Major River Basin 3; MRB3 SPARROW models) representing nutrient inputs in the early 2000s (Robertson and Saad Citation2011). This study represents the first binationally focused application of the model.
Application of any water quality model to geographical extents that span multiple countries comes with its own set of unique challenges. A common precursor to calibrating a water quality model is the establishment of a stream network that can route water and its chemical constituents from headwater regions to downstream drainage basin outlets. Though both Canada and the US have well-developed hydrographic networks, they have largely been developed separately. Similarly, any geospatial data layers used to generate source and delivery variables and any data used to estimate the nutrient loads at monitoring stations must be comparable across the international border. Only after all of this information is harmonized can calibration of a binational SPARROW model proceed.
There are four objectives of this paper. Objective 1 is to summarize the processes used to develop harmonized Canada–US binational data sets for use in the SPARROW models. Objective 2 is to describe how binational SPARROW models for TP and TN were developed for the RARB. The RARB is relatively small in terms of SPARROW modelling with limited data and limited spatial variability, which led to a few novel approaches being required to develop the models. Objective 3 is to apply the SPARROW models to: (1) estimate TP and TN loads throughout the RARB; (2) compare the relative importance of the various nutrient sources; (3) spatially apportion nutrient sources by jurisdiction and by major river basin; and (4) compare these results with those found in previous studies. Objective 4 is to make recommendations on the use of SPARROW models for future water quality modelling applications in binational basins and in basins with data constraints similar to those in the RARB.
Methods
Study area
The multi-jurisdictional RARB includes the entire international Red and Souris river basins and the rest of the Assiniboine River Basin, which is completely in Canada (Figure ). The total area of the RARB is ~220,000 km2: 47% in Canada and 53% in the US (Table ). The system is complex in that the Souris River Basin is a sub-basin of the Assiniboine River Basin, which in turn is a sub-basin of the Red River Basin. The terminus of this SPARROW application is where the Red River discharges into the South Basin of Lake Winnipeg at Netley-Libau Marsh, ~20 km downstream from Selkirk, Manitoba. Although the Qu’Appelle River Basin, contained within the Assiniboine River Basin, is part of the overall drainage system, data limitations restricted its inclusion in this model. Consequently, the Qu’Appelle River contribution to the Upper Assiniboine River is treated as a single point source of nutrients, which was estimated based on loading computed at a monitoring station near the Saskatchewan–Manitoba border. Although the Qu’Appelle River Basin at this location represents over 50% of the entire Assiniboine River Basin (Table ), the measured loads only represent about 10% of the TP and 14% of the TN near the outlet of the Assiniboine River Basin.
Table 1. Drainage areas and major land coverages for the areal extent of Red—Assiniboine River Basin (RARB) SPARROW models.
Most of the RARB can be characterized by two physiographic regions typical of prairie environments. The first is the very flat lowland region of the Red River Basin, commonly known as the Red River Valley, which formed the southern extent of Glacial Lake Agassiz. Along the western edge of the basin, the Manitoba Escarpment marks the transition between the lowland region and the second region, where the landscape has greater relief and is characterized by rolling topography and a mosaic of prairie wetlands. Agriculture is the predominant land use in both regions (Table ). In addition, forested land occurs along the eastern edge of the Red River Basin with mainly coniferous forest in Manitoba and a mix of coniferous and deciduous forest in Minnesota. Accordingly, river morphology varies widely across the RARB; rivers in the Red River Basin and lower reaches of the Assiniboine River Basin tend be sinuous while rivers elsewhere tend to follow major landforms. In the Red River Basin, particularly in Manitoba, many stream reaches have been engineered into linear drains to facilitate drainage of lowland agricultural lands. Across the RARB, the continental climate is, on average, relatively drier and cooler towards the northwest and warmer and wetter towards the southeast. Mean annual temperatures range from 3 to 5°C, with normal summer highs between 25 and 30°C and normal winter lows between −20 and −15°C (ECCC Citation2016a, NCEI Citation2016). Annual precipitation ranges from less than 400 mm in the west to more than 550 mm to the east (Environment and Climate Change Canada [ECCC] Citation2016a; National Centers for Environmental Information [NCEI] Citation2016).
Though the RARB is mostly rural, there are several populated urban centres including Winnipeg and Brandon (Manitoba), Grand Forks–East Grand Forks and Fargo–Morehead (North Dakota–Minnesota), and Minot (North Dakota).
SPARROW model – overview
SPARROW is a spatially explicit watershed model that uses a mass-balance approach to estimate nutrient sources, and non-conservative transport and transformation (i.e. losses) of nutrients throughout watersheds under long-term steady-state conditions (see Schwarz et al. Citation2006 and Smith et al. Citation1997 for more detailed descriptions). There are four major components of a SPARROW model: (1) a stream network and its catchments throughout the basin; (2) nutrient sources that are input to each of the catchments; (3) the land-to-water delivery and instream decay factors that describe the variability in the amount of the nutrients input to each catchment that is delivered to each stream and then lost during instream transport; and (4) measured estimates of long-term average annual nutrient loads, detrended to a specific year, at locations in the network for model calibration (dependent variables). In this RARB application, most of the data sets and information used in the development of SPARROW models needed to be harmonized across jurisdictions, both within Canada (between the provinces of Manitoba and Saskatchewan) and between Canada and the US, prior to model calibration (described in Jenkinson and Benoy Citation2015). SPARROW models are developed for a specific base year. The base year represents the year for which the most recently available explanatory geospatial (source inputs and land-to-water delivery) data are available. The 2002 base year was selected for this application to coincide with the most recently available data and it was the year used in previous US SPARROW models for part of this basin (MRB3; Robertson and Saad Citation2011). Although a specific base year is used for model inputs, long-term average annual loads detrended to the base year are used in model calibration in order to incorporate the aggregate effect of floods, droughts and extreme seasonality that have occurred over an extended period, in this case from the early 1970s to the late 2000s.
Stream network and catchment delineation
The stream network describes the geographic path and connectivity of water bodies throughout the RARB. Stream delineations were acquired using mapped stream network products from several national and binational data sources. The National Hydro Network (NHN) was used for most of the Canadian portion of the model (Natural Resources Canada [NRCan] Citation2009), the National Hydrography Dataset (NHD) was used for most of the US portion Moore and Dewald (Citation2016), and a third product provided by the International Joint Commission (IJC) Data Harmonization project included the harmonized stream segment data set across the border between Canada and the US (Laitta Citation2010). This last data set was available for the Souris River Basin, but was not fully completed for the Red River Basin at the time of this study; therefore, a few manual adjustments were required at the border to connect some streams. This harmonization process is described in Jenkinson and Benoy (Citation2015). Water bodies, such as lakes and reservoirs, which are hydraulically connected to the stream network require explicit definition in a SPARROW model. For each of these lakes or reservoirs, the “hydraulic load” was estimated from the ratio of the average streamflow to lake or reservoir area – a surrogate for hydraulic residence time.
To describe nutrient inputs to each stream reach in a SPARROW model, the contributing catchments for each stream reach must be delineated. These catchments were delineated using digital elevation model (DEM) data products (Canadian Digital Elevation Dataset [CDED] for Canada, and National Elevation Dataset [NED] for the US) that were harmonized over the domain using flow-direction accumulations derived from DEM slope data (Jenkinson and Benoy Citation2015). The resulting DEM had a 30-m horizontal resolution. Due to the relatively limited topographic relief in much of the RARB, the catchments could not be reliably delineated by DEM data alone. The catchments were further defined using techniques developed by the USGS with available hydrographic data sets, including the stream network itself and previously delineated sub-basin boundaries (Johnston et al. Citation2009). A total of 74,219 catchments were identified within the RARB.
Not all areas of the RARB contribute runoff to the river network. Two sources of information were used to define these areas. The first was the non-contributing area map developed by Agriculture and Agri-Food Canada (AAFC; Prairie Farm Rehabilitation Agency [PFRA] Citation2008). The second was a list of isolated lakes presumed to be non-contributing, and the regionally labelled “prairie potholes.” These areas were removed from the RARB river network as they do not contribute to loading estimates in the SPARROW models.
Time of travel in each reach is used to describe removal (loss) in streams. Time of travel was estimated from stream-reach length and average flow velocity of channel segments. Average flow velocity estimated was from average annual flow and channel slope. Flow estimates were derived from a 900-m USGS runoff grid, following an approach similar to that used in the MRB3 model (Robertson and Saad Citation2011). The inverse of hydraulic loading was used to estimate retention time and describe the removal of nutrients in reservoirs (Alexander et al. Citation2008).
Nutrient sources
SPARROW models should include all of the important sources of the parameter being modelled. After evaluating a variety of model specifications, using knowledge of the key sources in the region and from prior modelling efforts, the following nutrient sources were included in the RARB models. For the TP model, input from wastewater treatment plants (WWTPs), farm fertilizers and total manure (collectively referred to as agricultural inputs), forests and wetlands, and medium-sized stream channels (referred to as stream channels) were included. For the TN model, inputs from WWTPs, agricultural inputs and atmospheric deposition were included. A brief description of each source is given below, and described in more detail along with spatial coverages in Jenkinson and Benoy (Citation2015).
Inputs from WWTP effluent in Canada were estimated by population, and discharge locations were provided by Manitoba Conservation and Water Stewardship (MCWS) and the Saskatchewan Water Security Agency (SWSA). Inputs from WWTP effluent in the US were directly estimated from nutrient concentrations and discharges in the USEPA Permit Compliance System, and supplemented with data obtained from some state agencies (Maupin and Ivahnenko Citation2011). In total, 351 catchments with WWTP input were identified in the RARB. Fertilizer inputs for the Canadian part of the RARB were estimated from the AAFC agricultural census of 2001 reports and Canadian Fertilizer Institute (CFI) expenditure reports (Jenkinson and Benoy Citation2015). Fertilizer inputs for US catchments were based on county-level estimates made by Ruddy et al. (Citation2006). Fertilizer input was then applied to each catchment as a nutrient source based on its relative amount of agricultural land (not including pasture) in the sub-sub-drainage area (SSDA) or the county. Nutrients from livestock wastes (manure) were estimated from the Statistics Canada Census of Agriculture and United States Department of Agriculture (USDA) Census of Agriculture. Canadian data included livestock headcounts by SSDA boundaries (NRCan Citation2003), which were available for 2001 (AAFC Citation2001). US data were estimated from total livestock headcounts by county for 2002. Nutrients from each type of animal were calculated by determining head counts in each reporting area and applying a livestock-specific multiplier (AAFC Citation2001). Nutrient inputs from manure were then apportioned to each catchment based on its relative amount of agricultural land (including pasture). For both TP and TN, due to the correlation between fertilizer and manure inputs, it was not possible to statistically separate the effects of these two sources; therefore, they were combined into a single agricultural input term and assumed to be exported at a similar rate.
Some general land-use-related inputs, such as from forested and urban areas, were estimated based on the area of each land-use type in a catchment, which was computed from Land Cover data circa 2000 from the Natural Resources Canada Geobase (Centre for Topographic Information [CTI] Citation2009) and US National Land Cover Database 2001 (NLCD; Homer et al. Citation2007). These two land use data products were harmonized using a set of cross-walk tables (Figure ). Forest and wetland areas were then combined into one source variable during model calibration. Sediment mobilized from channel erosion and streambank slumping can be a source of P (Sekely et al. Citation2002); therefore, “stream channels” was used as a source variable (Robertson and Saad Citation2013). The specific discharge values were found during the model calibration process (i.e. medium-sized streams with discharge between 0.03 and 0.21 m3/s or 1 and 7.5 cfs). Atmospheric deposition of TN was obtained from the Community Multi-scale Air Quality Model (CMAQ; Schwede et al. Citation2009; Hong et al. Citation2011; USEPA Citation2012), which includes volatilized N from natural, agricultural and urban sources.
Figure 2. Major land coverages of the Red—Assiniboine River Basin (RARB) and the locations of calibration sites. Land cover data for Canada circa 2000 is derived from Natural Resources Canada Geobase, and for the US it is from 2001 National Land Cover Data. Red N (nitrogen) circles and orange P (phosphorus) circles show which parameters were sampled from particular water quality stations.
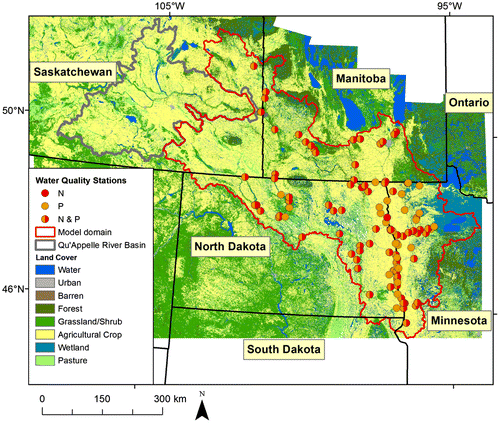
Land-to-water delivery variables
Land-to-water delivery variables in SPARROW models simulate specific landscape properties that affect nutrient transport from the sources into streams, such as climatic variables and soil characteristics. From the many possible variables, the specific ones that are typically included in SPARROW models are those that are usually statistically significant in explaining variability in TP and TN delivery to streams. The environmental variables examined in this study were the same as those included in previous SPARROW models for the area (Robertson and Saad Citation2011, 2013). Final land-to-water delivery variables for the RARB models included mean annual precipitation rates and air temperature. Precipitation and temperature data were obtained from the Precipitation-Elevation Regressions on Independent Slopes Model (PRISM; Daly et al. Citation1994), the Canadian Forest Service, and the Canadian Precipitation Analysis (CaPA; Mahfouf et al. Citation2007). Complete coverages of various other potential landscape variables, including soil characteristics, were developed (Jenkinson and Benoy Citation2015), but were not found to be important for these models.
Nutrient load estimation
Long-term mean annual TP and TN loads for monitoring sites throughout the RARB with sufficient stream flow and water quality data were computed with the regression-based rating curve procedure in the Fluxmaster load estimation program (see Jenkinson and Benoy Citation2015 and Saad et al. Citation2011 for assembling and screening information). This procedure combines the nutrient concentration data with daily streamflow measurements to provide more accurate load estimates than can be obtained using individual water-quality measurements alone (Walker Jr. Citation1981). Daily flow data for Canadian sites for the period 1971–2010 were obtained from the Water Survey of Canada (ECCC Citation2016b) and US sites for the period 1971–2006 from the National Water Information System (NWIS) database (USGS Citation2016). A slightly longer period of data was used for the Canadian sites in order to take advantage of the more recently collected data in Canada, and obtain additional sites. For monitoring sites without a co-located hydrometric station, flow was estimated by area-ratio transfer functions from nearby stations.
Several databases were accessed for water quality data for the Canadian sites, including the Environment Canada Aquatic Chemistry and Biological Information System (ACBIS) database, the water quality database of MCWS, Saskatchewan Environment’s Environmental Management System (SEEMS), and the City of Winnipeg’s Water and Wastewater Department. Water quality data for US sites were obtained from the USEPA STOret and RETrieval (STORET) database, the USGS NWIS database, the Minnesota Pollution Control Agency and the North Dakota Department of Health. Only data from agencies that routinely collect water-quality data and use standardized sample collection and laboratory analysis protocols were used in this analysis. For a monitoring site to be considered for inclusion in the SPARROW models it had to have at least 25 samples over at least 2 years (including data near the base year of 2002), although most sites had much more data than this.
Regression models that related the logarithm of concentration to the logarithm of daily flow, day of the year (to compensate for seasonality) and decimal time (to compensate for trends) were fit to data from each potential load site. Each regression model was then used to estimate a long-term mean annual load, and detrended to the 2002 base year, by first calibrating the flux model using data from 1971–2010 or 1971–2006 and then re-estimating using detrended daily flows for the entire period (see Schwarz et al. Citation2006 for a complete description of the detrending process). All daily loads were then adjusted for log re-transformation biases. Average detrended (to 2002) annual loads were then computed by aggregating the daily detrended loads for all years with complete records of daily flow, and averaging over all such years between 1971–2010 or 1971–2006. The use of detrended mean annual loads helps compensate for differences in the length of record and frequency of monitoring data among sites. It also minimizes the inherent variability introduced by year-to-year variation in precipitation, facilitating the identification of factors that affect loading over long periods. To ensure that load estimations used in SPARROW calibration were as accurate as possible, sites for which the regression relation performed poorly (i.e. standard error [SE] greater than 50% or a ratio of the total estimated load to the total observed load for days with water quality measurement greater than 1.5 or less than 0.667) were omitted.
For the Canadian portion of the RARB, 19 TP and 17 TN sites had sufficient data to compute long-term average loads. For the US portion of the RARB, 85 TP stations and 58 TN stations were used (Robertson and Saad Citation2011). When combined into one transboundary water-quality monitoring network, there were 104 TP load sites and 75 TN load sites (Figure ).
SPARROW model calibration in areas with limited data
In general, SPARROW models are calibrated by minimizing the error between observed and estimated loads in natural log units using nonlinear regression techniques. Individual source variables are typically included in the model only if they are statically significant (p ≤ 0.05) in explaining variation in TP and TN loads. Increasing the range of observations within source variables and land-to-water delivery variables increases the likelihood of significance; however, this may not always be achievable in relatively smaller basins with spatially limited variation and areas with limited numbers of monitoring sites. In instances where specific source variables known to be important are not significant, they are usually combined with other similar variables to create composite variables through a series of calibration runs until an acceptable level of model fit is achieved, as measured by root mean square error (RMSE), coefficient of determination (R2), variance inflation factors (VIF), model-estimated coefficients and distributions in residual errors (Robertson and Saad Citation2011). To demonstrate the robustness of the final models, mean coefficient values and confidence intervals are determined using a non-parametric bootstrap procedure (Schwarz et al. Citation2006; Robertson et al. Citation2009).
When using statistical techniques to determine which variables are important in a watershed model, it is important to have calibration sites that represent the ranges of stream orders, landscape characteristics and nutrient sources that occur in the study area. However, the final sites available to calibrate the RARB model were primarily in areas dominated by agriculture and located on mainstem rivers of the network (Figure ). Without having sites representing the full range of conditions for which the models are being applied, it can be difficult to incorporate specific source terms even if the spatial distribution of loads is predicted well. This, as will be shown, resulted in the need for a few unique approaches to develop the final SPARROW models. Incorporating all of the known source terms into the RARB models was a challenge, especially for inputs from WWTPs for both the TP and TN models. Additionally, factors that have limited geographic variation across the study area (such as atmospheric deposition in the RARB) may be hard to model statistically, even if they are known to be important sources. These circumstances made it difficult to use regression techniques to include a few of the important non-agricultural sources to these SPARROW models. To resolve these issues, coefficients for these types of variables were manually set based on theoretical estimates and prior modelling experience (Robertson and Saad Citation2013) and do not have a p-value to describe their statistical significance. In addition, some sources of nutrients, such as atmospheric sources of TP, TN from legume crops, or other background contributions, were not explicitly included in the models; therefore, their contributions were incorporated into other source terms.
Similarly, model calibration is generally improved as the number of calibration sites increases. The effect of increasing the sample size, while holding other factors constant, is to reduce the standard errors of the estimated coefficients. This results in a reduction in the p-values of the coefficients, meaning that more coefficients will achieve statistical significance as the sample size increases even if the value of the estimated coefficient remains the same. More sites tend to enable inclusion of additional terms in the model and thus allow SPARROW to better describe the spatial variability in yields or loads and individual sources. Trying to distinguish the effects of several source terms, land-to-water delivery terms, and instream decay terms is difficult without a large number of calibration sites.
Results
Calibration of SPARROW models
Various trial calibration runs were examined in the development of the SPARROW models for the RARB using traditional techniques. However, some challenges stemmed from the aforementioned lack of spatial variability in the domain and the limited number and location of monitoring stations. For example, the coefficient associated with WWTPs was not statistically significant, when it is known that WWTPs should be an important source. WWTPs deliver their effluent directly to most streams; therefore, if our estimated WWTP loads were accurate, we would expect its coefficient to be ~1.0 (100% delivery). The coefficients for WWTPs in the MRB3 TP model (Robertson and Saad Citation2011) and the Mississippi/Atchafalaya River Basin (MARB) TP model (Robertson and Saad Citation2013) were estimated to be very near 1.0 (1.07 and 1.05, respectively). As a result, the coefficient for WWTPs was manually set to 1.0, followed by the use of routine calibration procedures to develop the model. The final RARB TP SPARROW model had four source terms (WWTPs, agricultural inputs, forests and wetlands, and stream channels), one land-to-water delivery term (precipitation), and loss or decay in reservoirs (Table ). Instream decay was not found to be statistically significant, possibly due to stream channels being a source of nutrients, and therefore was left out of the model. Coefficients for all final sources, with the exception of WWTPs, and delivery variables were highly significant (p ≤ 0.05), indicating that each is important in describing the measured loads. The coefficients were also robust, with narrow 90% confidence intervals. The TP model had an overall RMSE of 0.489, and it explained approximately 92% of monitored loads and 82% of monitored yields (all in natural log units).
Table 2. Summary of Red—Assiniboine River Basin (RARB) calibration results for total phosphorus (TP) and total nitrogen (TN) SPARROW models. RMSE is root-mean square error.
The ability of the SPARROW TP model to simulate the observed loads is shown through the depiction of errors calculated as the difference between observed and predicted loads (Figure a). Prediction errors are reported on a log base 2 scale because positive errors can become very large, but negative errors are constrained by an estimated load of zero. Base 2 was chosen so that each unit of 1 is equivalent to a doubling or halving of the measured values. Negative errors indicate over-predictions by the model, whereas positive errors indicate model under-predictions. For example, over-predictions of −1 and −2 indicate that the predicted value is 2× and 4× the measured value, respectively. Examination of the spatial distribution of residuals for the TP SPARROW model revealed limited regional biases, with loads in the Souris River Basin being slightly over-predicted and, by comparison, under-predicted for the Pembina River Basin, a tributary of the Red River along the border between Manitoba and North Dakota (Figure a).
Figure 3. Predictability of Red—Assiniboine River Basin SPARROW models for (a) phosphorus (TP) and (b) nitrogen (TN). Predictability is the difference between observed and predicted loads expressed in units of log 2, which represents the number of doublings of the measured loads at each site (i.e. over-predicted – negative values, upward pointing triangles; under-predicted – positive values, downward pointing triangles).
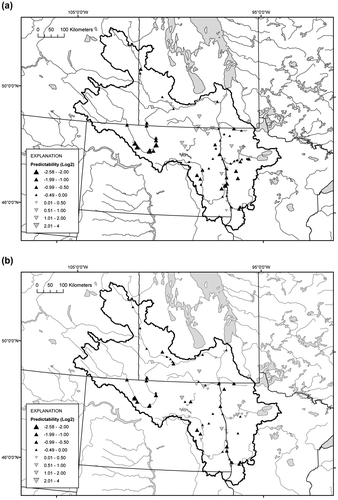
The coefficient associated with the agricultural inputs variable (0.011) in the RARB TP model (Table ) indicate that ~1% of the TP in agricultural inputs is transferred to streams, which is less than that estimated (~3–9%) for the MRB3 (Robertson and Saad Citation2011) and MARB (Robertson and Saad Citation2013). On average, forested areas of the RARB are estimated to contribute ~6 kg/km2/yr. This is less than the contribution estimated for MRB3 (~15 kg/km2/yr), but similar to that of the MARB (4.2 kg/km2/yr), and within the range of published annual TP exports from forested areas throughout the US (2–83 kg/km2, Reckhow et al. Citation1980). Additionally, TP was contributed by stream channels. Only variability in precipitation was important in describing the variability in delivery of these sources to streams; the positive coefficient indicates increased precipitation enhanced the delivery of TP. The last significant term included in the model was losses of TP in reservoirs.
Development of a TN SPARROW model for the RARB was more difficult than for TP because there were ~25% fewer monitoring stations and there was limited variability in some sources. Initial standard calibration techniques resulted in the coefficients associated with WWTPs and atmospheric deposition being negative and not statistically significant. Therefore, the coefficient for WWTPs was again manually set to 1.0, which is slightly higher than that found for MRB3 and the MARB (~0.8). Atmospheric deposition has been shown to be a significant source of TN in most previous regional (Robertson and Saad Citation2011, 2013) and national SPARROW models (Smith et al. Citation1997; Alexander et al. 2011); however, its calibrated coefficient was negative and not statistically significant. This may have resulted from the input from atmospheric deposition being strongly correlated with agricultural inputs. Past studies that have used CMAQ to quantify atmospheric deposition have found that its rate of delivery was close to that of fertilizers and manure (Robertson and Saad Citation2013); therefore, it was manually set to equal that estimated for those sources. The final RARB TN SPARROW model had three source terms (WWTPs, agricultural inputs and atmospheric deposition), two land-to-water delivery terms (precipitation and air temperature), and in-stream and in-reservoir decay (Table ). Instream decay and reservoir losses were not statistically significant at p ≤ 0.05, but were retained in the final model. These variables represent known processes, such as denitrification, that have consistently been found to be significant in other models for the US portion of this area (Robertson and Saad Citation2011, 2013). The final TN model had an overall RMSE of 0.440 and it explained 93% of monitored loads (all in natural log units). Examination of residuals for the TN SPARROW model revealed limited regional biases, with loads in the Souris River Basin being slightly over-predicted (Figure b).
Some of the TN model sources described here were not statistically estimated, as in other SPARROW models. The exception is the term for agricultural inputs, for which it was found that only ~3% of the TN reaches streams, which is less than that found for other published SPARROW models for areas near the RARB (Robertson and Saad Citation2011, 2013). Compared to other nearby areas simulated with SPARROW models, average precipitation in the RARB is less, which should result in less runoff and less nutrient delivery of TP and TN to the streams.
As noted previously, SPARROW models work best in areas with diverse nutrient inputs and environmental characteristics, and where calibration sites span a wide range of potential nutrient inputs. Most of the calibration sites in the RARB are in areas dominated by agriculture. As a result, other nutrient sources, such as WWTPs, are not well represented by the calibration targets and could not be added to the model using traditional statistical methods. These sources were added manually, using coefficients based on other, nearby and overlapping SPARROW models. These models can still be used to evaluate where and from what sources the TP and TN in RARB streams originate; however, the coefficients of the manually added sources cannot be evaluated statistically for robustness or significance.
Nutrient loads and yields
SPARROW models simulate long-term annual loads at various spatial scales. Loads generated within a catchment and delivered to local streams are referred to as incremental loads. SPARROW models keep track of changes to these incremental loads as they are delivered downstream and are subject to instream and reservoir loss decay processes (referred to as delivered incremental loads). Delivered incremental loads are referenced to a downstream target, which, for the RARB models, is Lake Winnipeg. Incremental and delivered incremental loads can be summed for specified areas (these are referred to as aggregated or delivered aggregated loads, respectively) to evaluate nutrient loading at various watershed scales or political boundaries. Aggregated loads, also called non-decayed loads (i.e. no losses in transport beyond the original reach) are useful for evaluating upstream contributions to local water-quality conditions. Delivered aggregated loads are useful for evaluating local contributions to water-quality conditions at a specified downstream location such as a lake or estuary. Nutrient yields are calculated as loads divided by the corresponding drainage area. Similar to loads, there are incremental, delivered incremental, aggregated and delivered aggregated yields.
For the entire RARB, the aggregated yield for TP to streams was 30.2 kg/km2, and for TN it was 240 kg/km2 (Table ). Subdivided into sub-drainage area (SDA) in Canada (NRCan Citation2003) or Hydrologic Unit Code 4 (HUC4) in the US (USGS and USEPA 2006), hereafter referred to as “major basin,” the highest aggregated yields for both TP and TN were for the Red River major basin, 3 to 7 times higher than for the Souris River and Upper Assiniboine River major basins. Catchments including WWTPs tended to have especially high yields, though areas dominated by intensive agriculture, such as in the Red River and Upper Assiniboine River major basins, also had relatively high yields. Aggregated TP and TN yields were relatively low for the western portion of the Souris River major basin, reflecting relatively lower rates of precipitation compared to eastern and southern portions. The aggregated nutrient loads in all streams throughout the RARB were 6640 MT for TP and 52,700 MT for TN.
Table 3. Estimated annual loads and yields of total phosphorus (TP) and total nitrogen (TN) from Red—Assiniboine River Basin (RARB) SPARROW models, normalized to 2002. MT is metric tonnes. (Note: loads do not necessarily add up due to rounding.)
At the outlet of the RARB to Lake Winnipeg, the delivered aggregated load was 4970 MT for TP and 33,300 MT for TN (Table ). The delivered aggregated load at the outlet represents a 25% decrease in TP and a 37% decrease in TN from the total non-decayed aggregated load (i.e. loss during downstream transport). When the delivered aggregated TP and TN loads are subdivided into major basins, 90% came from the Red River for both nutrients. For delivered aggregated yield by major basin, TP and TN yields were 5 to 15 times higher for the Red River compared to the other major basins. Substantial differences observed between the Red River major basin and the other major basins in both TP and TN delivered loads and yields are reflected in the higher delivery fractions calculated for the Red River (~2.5 times greater).
When subdivided by province or state, the delivered aggregated load for TP was similar for Manitoba, North Dakota and Minnesota, and much lower for Saskatchewan and South Dakota. The total delivered load for Canada was 1900 MT, compared to 3060 MT for the US However, when adjusted for differences in jurisdictional area, a different ranking results. The highest delivered aggregated yields for TP were similar among Minnesota, South Dakota and Manitoba, followed by North Dakota and Saskatchewan. The average TP yield for Canada was 18.4 kg/km2 compared to 26.3 kg/km2 for the US For TN, the delivered aggregated load was highest for Manitoba, followed by Minnesota and North Dakota. The total delivered TN load from Canada was 14,100, compared to 19,200 MT for the US. When comparing delivered aggregated loads, the switch in rank between North Dakota and Minnesota is because a larger portion of the drainage area is in Minnesota. Delivered aggregated TN yields by jurisdictional area were similar between Manitoba and Minnesota, followed by North Dakota, South Dakota and Saskatchewan. The average delivered TN yield for Canada was 136 kg/km2, compared to 165 kg/km2 for the US.
Midway between major basin and catchment scales, at the HUC8 (USGS and USEPA 2006) or SSDA scale, there are 54 geospatial units. For TP, aggregated yields were highest in portions of the Red River and lowest in the upper reaches of the Souris River and Upper Assiniboine River basins (Figure a). When expressed as delivered aggregated yields, the contribution from the Red River to TP at the outlet of the RARB was much greater than that from the other major basins. For TN, a similar pattern for aggregated yields was observed across the RARB except that more of the Souris River Basin had a relatively lower yield (Figure b). When expressed as delivered aggregated yields to Lake Winnipeg, it was the Red River that contributed more TN to total load at the outlet.
Sources of nutrients
Only a fraction of the nutrient input to the landscape is delivered to streams; further losses are associated with instream decay and reservoir sequestration, which are assumed to be similar for all nutrient sources. Based on results from the RARB TP SPARROW model, ~65% of the TP reaching Lake Winnipeg came from agricultural inputs, ~16% from stream channels, ~12% from WWTPs, ~6% from forests and wetlands, and less than 1% from the Qu’Appelle River (Figure a). Based on results from the TN model, ~75% came from agricultural inputs, ~12% from each of atmosphere deposition and WWTPs, and less than 1% from the Qu’Appelle River (Figure b).
Figure 5. Delivered aggregated loads (and delivered aggregated yields) to Lake Winnipeg by geographic unit of the Red—Assiniboine River Basin (RARB) for (a) phosphorus (TP) and (b) nitrogen (TN). Loads are subdivided by source and shown as percentages.
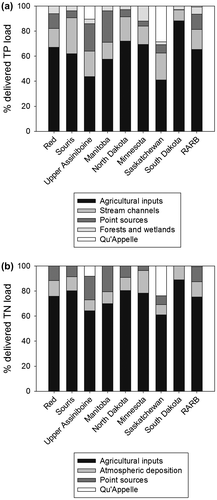
For the Red River and Souris River major basins, the greatest source of TP was from agricultural inputs, followed by channels, WWTPs, and forests and wetlands. The relative importance of agricultural inputs appears to be smaller for the Upper Assiniboine River because of the relatively higher input from WWTPs and the Qu’Appelle River. However, given that much of the Qu’Appelle River Basin is agricultural, a majority of that portion would be expected to be from agricultural inputs. The relative importance of stream channels was greatest in the Souris River Basin. A similar pattern was observed for TN. Agricultural input was the dominant source for the Red River and Souris River major basins, followed by atmospheric deposition and WWTPs. For the Upper Assiniboine River, WWTPs and the Qu’Appelle River comprised ~25% of the delivered aggregated TN load.
For all provinces and states, agricultural input was the largest source for both TP and TN. For TP, the relative contribution of agriculture in Manitoba was less than other jurisdictions because WWTPs represent ~25% of the load, mainly due to inputs from the City of Winnipeg. Contributions from agriculture inputs, stream channels and WWTPs were relatively similar for North Dakota and Minnesota. The contribution from forests and wetlands was greatest for Minnesota, owing to the forested area along the eastern portion of the Red River. When considered together, the contributions from WWTPs and the Qu’Appelle River are ~35% of the total delivered aggregated TP load from Saskatchewan. As noted, since much of the Qu’Appelle River Basin is agricultural, a majority of that portion would be expected to be from agricultural inputs, so the allocation is similar to that of other areas. For TN from Manitoba, WWTPs were the second most important source. In the other jurisdictions, atmospheric input was the second most important source of TN, especially in Minnesota where it accounted for ~20% of the TN. Collectively, WWTPs and the Qu’Appelle River contribute ~30% of the TN from Saskatchewan.
Discussion
Comparison of SPARROW loads and yields with previous studies
Nutrient loads estimated by SPARROW can be compared with those of other studies. For the period 1999 to 2007 (roughly the same as in this study), Armstrong and McCullough (Citation2011) estimated that the average annual TP load at the outlet of the Red River was 5380 MT/year and the TN load was 31,500 MT/year, which compares well with the SPARROW model estimates of 4960 MT/year for TP and 33,300 MT/year for TN. For the earlier time period of 1994 to 2001, Bourne et al. (Citation2002) estimated that the average TP load was 4270 MT/yr and the TN load was 29,100 MT/yr, suggesting that net delivered nutrient loads (especially TP) have been increasing.
For both TP and TN, ~90% of the delivered load to the outlet of the RARB came from the Red River. Upstream of the City of Winnipeg, before the confluence of the Red River with the Assiniboine River, the SPARROW model estimated delivered load was 3980 MT/year for TP and 26,200 MT/yr for TN, similar to values calculated by Armstrong and McCullough (Citation2011; 3680 MT/yr for TP and 22,000 MT/yr for TN). Based on SPARROW results, the US portion of the Red River Basin contributed 76% of the TP and 70% of the TN. In comparison, Armstrong and McCullough (Citation2011) estimated that 70% of the TP and 78% of the TN came from the US portion.
For their respective areas, TP yields estimated from RARB models were one half to one third of those calculated for MRB3 and MARB models, and TN yields were one third to one tenth (Robertson and Saad Citation2011, 2013). Although not directly comparable due to differences in the data sets used for calibration, these coarse-scale differences between modelling results may be primarily attributable to less precipitation causing less runoff to be generated in the northwestern portions of the Great Plains region in the RARB. When just northern sections of the MARB are considered, the delivered yields for the RARB are intermediate between the Upper Missouri River Basin to the west and the Upper Mississippi River Basin to the southeast (i.e. for TP: RARB – 22.6 kg/km2, Upper Missouri – 11.1 kg/km2 and Upper Mississippi – 34.4 kg/km2; for TN: RARB – 152 kg/km2, Upper Missouri – 77.0 kg/km2 and Upper Mississippi – 580 kg/km2). Average annual nutrient exports (i.e. yields) reported by Armstrong and McCullough (Citation2011) for the Red–Assiniboine Basin of the Lake Winnipeg watershed after removing the Qu’Appelle River drainage area were 22.8 kg/km2 for TP and 133.3 kg/km2 for TN, values which are similar to those estimated in this study. For the Qu’Appelle River Basin alone, nutrient exports found by Armstrong and McCullough (Citation2011) were 15 kg/km2 for TP and 103 kg/km2 for TN. In addition, Armstrong and McCullough (Citation2011) estimated that the Qu’Appelle River contributed 77 MT/yr of TP (same as the value used in this study) and 525 MT/yr of TN (compared to 473 MT/yr used in this study). Overall, the pattern in nutrient yields and delivered nutrients from this study and that of Armstrong and McCullough (Citation2011) is consistent with the continental hydroclimatic gradient that extends from relatively drier conditions in the northwest part of the basin grading to relatively wetter conditions in the southeast.
Of the major basins identified in this study, the predictability of loads in the Souris River Basin by SPARROW was biased high for both TP and TN. The sinuosity of the river across the border suggests that the bias exists in both countries. When adjusted for basin area, SPARROW RARB delivered loads are ~20% higher for TP and ~25% higher for TN compared to loads reported by Armstrong and McCullough (Citation2011). Therefore, the importance of the Souris River Basin is slightly overestimated compared to that of the other basins (Table ). To a lesser extent, the converse is observed for the Pembina River Basin, where a bias towards under-prediction exists for TP. These patterns in load discrepancies are at least partially attributable to the relatively limited number and spatial variability of sources that were included in the RARB SPARROW models. More extensive SPARROW models that cover a larger spatial domain will hopefully reduce these biases.
Comparison of sources with previous studies
At the national, provincial and state, and major basin scales, the combined fertilizer and manure term was the largest source of both TP and TN in the SPARROW RARB models (i.e. 44–88% for TP and 61–89% for TN). When compared with the summed fertilizer and manure (and legume crops for just TN) terms described in the MARB SPARROW models (Robertson and Saad Citation2013), the TP and TN agricultural sources for the RARB are more important than those for the Upper Mississippi River Basin (i.e. 65.4% vs. 53.2% for TP and 75.2% vs. 68.5% for TN), but quite similar to those for the Upper Missouri River Basin (i.e. 65.4% vs. 66.8% for TP and 75.2 vs. 73.6%).
Input from WWTPs was a more important source of TP and TN in the Province of Manitoba than in other parts of the RARB, partially because of contributions from the City of Winnipeg. Although forest cover is the dominant land cover along the eastern side of the Red River major basin, forests and wetlands played a minor role as a source of TP in the RARB. Inputs from stream channels reflect the importance of TP that was either eroded from channels and streambanks or previously deposited from upstream sources. Based on results from the RARB TP model, stream channels accounted for ~16% of the delivered aggregated load, which is close to the value of 14% for the MARB TP SPARROW model (Robertson and Saad Citation2013) and a field-based estimate of ~10% for the Blue Earth River, Minnesota (Sekely et al. Citation2002). Compared with stream channel contributions estimated from the Upper Missouri River Basin and the Upper Mississippi River Basin, the contribution from stream channels in the Red River and Upper Assiniboine River major basins was 15% and 20%, respectively. Stream channels in the Souris River major basin were relatively more important at 29%, suggesting that streambanks and streambeds may be more important in the export of TP from catchments in the western portions of the RARB. Similar proportions of channel-based erosion have been observed in other predominantly agricultural watersheds in Denmark (Kronvang et al. Citation2012) and Vermont (DeWolfe et al. Citation2004), suggesting that this is a common source of TP.
Calibration of spatially explicit models in areas with limited variation and data
Some areas may not have all of the information typically needed to develop a spatially explicit watershed model like SPARROW, which is calibrated using statistically derived coefficients. Such constraints may include limited spatial variability in nutrient sources and environmental characteristics, strong correlations among input sources, limited number of calibration sites especially in specific land-use and land-cover areas, or a combination thereof. Many of these difficulties exist in the RARB; therefore, unique approaches were used to develop the RARB SPARROW models, such as manually setting specific coefficient values based on previous knowledge of these sources and prior modelling efforts, and lessening the restrictions on statistical significance. The net effect of these factors was that it was a challenge to identify many watershed attributes that could more finely resolve the observed spatial variation that exists across the RARB.
When compared with calibration statistics for other SPARROW models in the contiguous US and New Zealand, the performance of the binational RARB models is very similar based on various statistical summary values, such as RMSEs (Elliott et al. Citation2005; Robertson and Saad Citation2011, 2013). The major difference is that the total number of explanatory variables is greater for these other models than for the RARB models. For the New Zealand TP and TN models, while the number of calibration sites was similar to that of the RARB (i.e. 77 sites in New Zealand for both TP and TN), greater definition of the inputs from various sources was achieved through a wider range in environmental conditions and distribution of nutrient load calibration stations that better reflect the wide ranges of land use and land cover (Elliott et al. Citation2005). For the MARB and MRB3 SPARROW models (Robertson and Saad Citation2011, 2013), the combination of much larger spatial extents (i.e. > 10×) and a greater number of calibration sites (i.e. 5–10×) meant that more fully resolved sources could be included than the relatively coarser ones in the RARB models (i.e. agricultural sources could be subdivided into inputs from fertilizers, manure and legume crops). Accordingly, RARB SPARROW models could be improved by either: (1) increasing monitoring efforts within the spatial extent of the stream network, especially in areas not dominated by agriculture; or (2) geographically expanding the model to capture larger variability in nutrient loads, and larger variability in the sources and delivery factors across a larger region, such as incorporating the RARB into either the MRB3 or MARB models. However, while greater resolution of data sets describing TP and TN variables may be available at larger spatial scales in either Canada or the US, the creation of harmonized binational data sets means that data are sometimes lost due to re-sampling at a common resolution.
Conclusions
Meaningful understanding and effective management of boundary lakes and rivers requires binational applications of analytical approaches and tools. Water-quality modelling represents one option for gaining an integrated perspective on the sources and fates of nutrients across these shared drainage areas. However, noticeably absent from published SPARROW models are the Canadian portions of transboundary watersheds (Moore et al. Citation2011; Robertson and Saad Citation2011). The TP and TN models reported on here for the RARB represent the first binationally focused application of the SPARROW model. Though modest in spatial extent when compared with SPARROW models developed for the US, the performance metrics for the RARB model were similarly robust, and the estimated average annual loads of TP and TN at the outlet of the entire basin were close to those of published reports. Therefore, RARB SPARROW models should be applicable to regional-scale questions of nutrient loads by watershed or by jurisdiction.
Reducing nutrient loadings to streams throughout the entire RARB would provide a comprehensive way to reduce loading to Lake Winnipeg and remediate eutrophication; however, it may not be the most efficient strategy because not all areas contribute equal quantities of nutrients. As shown here, SPARROW results can be used to target where reduction efforts would provide the largest potential local and downstream benefit (i.e. areas with the largest relative contributions or highest contributing areas). Therefore, contributions from sub-basins at various scales (e.g. catchments, river basins or provinces/states) can be ranked on the basis of their respective loads, yields or delivered incremental yields. SPARROW model results also demonstrated the dominant sources of TP and TN at each of these various scales. Information from these SPARROW models may assist managers in guiding efforts to reduce nutrient loading from large catchments by describing where efforts could have the largest effects (based on ranking results) and what types of actions would have the largest impact (based on source type allocation results).
Through this RARB binational application, the SPARROW model has been shown to be useful for estimating nutrient loads, particularly at larger spatial aggregations, and for coarse characterization of key source terms. The RARB models benefitted from data sets generated in support of the MRB3 SPARROW model in the US portion of the basin. However, two key hurdles had to be overcome to develop the models. The first hurdle was harmonization of the many geospatial data sets between Canada and the US. The second hurdle involved developing novel calibration approaches to address limited spatial variability in nutrient sources and environmental characteristics, strong correlations among input sources, and a limited number of calibration sites, especially in some land-use and land-cover types.
When SPARROW models are developed in relatively small areas of the Great Plains of North America, such as the RARB, or other areas that are largely agricultural, it is not surprising that only a few terms could be incorporated into the models to explain variability in the nutrient sources and their delivery. There are basically two options for RARB model improvement. The first option involves working with the existing spatial extent of the model by increasing the number of nutrient load calibration stations through relaxation of screening criteria, and refining or developing new source and delivery variables that may be potentially influential. The second option involves developing SPARROW models with a greater geographic extent that encompass the RARB, thereby increasing the spatial variability and climatic gradients across the landscape and the number of nutrient load calibration stations. This would result in an increased likelihood that source terms could be more specific and that additional attributes could be included in the models. Based on the lessons learned through the development of the RARB SPARROW models, efforts are underway to develop binational SPARROW models that include the entire Red–Assiniboine, Great Lakes and Rainy–Lake of the Woods basins. When completed, mid-continent models would stretch from the Qu’Appelle River in the west to the downstream outlet of the St. Lawrence River in the east, and will serve to strengthen results for all component watersheds, including the RARB.
Funding
This work was supported by the International Joint Commission.
Supplemental material
The RARB SPARROW models described here, including Fluxmaster and SPARROW model output, can be accessed through an online Red–Assiniboine SPARROW mapping tool at http://wim.usgs.gov/SparrowRAmapper/SparrowRAmapper.html#.
Acknowledgements
The authors thank the IJC and its International Watersheds Initiative for providing the major funding for this project. Many federal and provincial agencies generously contributed expertise and data: Craig Johnston and Donna Myers (USGS), Richard Burcher and Martin Serrer (National Research Council), Erika Klyszejko and Craig McCrimmon (ECCC), Jason Vanrobaeys (AAFC), Elaine Page and Justin Shead (MCWS) and Pam Minifie and Ondiveerapan Thirunavukkarasu (SWSA). Michael Laitta and Ted Yuzyk set the context for this binational model application through the IJC’s Hydrographic Data Harmonization Task Force. The lead author acknowledges the support of ECCC and AAFC with whom he was affiliated when the project was initiated. Sarah Lobrichon of the IJC is thanked for translating the abstract. Finally, we thank the International Red River Board and the International Souris River Board for encouraging us to undertake this project.
References
- Agriculture and Agri-Food Canada (AAFC). 2001. Interpolated Census of Agriculture to Soil Landscapes, Ecological Frameworks, and Drainage Areas of Canada. Technical Report, Agriculture and Agri-Food Canada. Ottawa, ON.
- Alexander, R. B., R. A. Smith, G. E. Schwarz, E. W. Boyer, J. V. Nolan, and J. W. Brakebill. 2008. Differences in phosphorus and nitrogen delivery to the Gulf of Mexico from the Mississippi River Basin. Environmental Science and Technology 42(3): 822–830.
- Armstrong, N., and G. K. McCullough. 2011. 7.0 Nutrient loading to Lake Winnipeg. In State of Lake Winnipeg: 1999–2007, ed. L. Lévesque and E. Page, 81–103. Environment Canada and Manitoba Water Stewardship. [Winnipeg, MB]
- Bourne, A., N. Armstrong, and G. Jones. 2002. A preliminary estimate of total nitrogen and total phosphorus loading to streams in Manitoba, Canada. Manitoba Conservation Report No. 2002–04. Winnipeg, MB: Manitoba Conservation Water Quality Management Section, 49 pp.
- Centre for Topographic Information (CTI). 2009. Land cover, circa 2000 – Vector data product, edition 1.0. Technical report. Natural Resources Canada. Ottawa, ON.
- Daly, C., R. P. Neilson, and D. L. Phillips. 1994. A statistical-topographic model for mapping climatological precipitation over mountainous terrain. Journal of Applied Meteorology 33(2): 140–158.
- DeWolfe, M. N., W. C. Hession, and M. C. Watzin. 2004. Sediment and phosphorus loads from streambank erosion in Vermont, USA. In Critical transitions in water and environmental resources management, ed. G. Sehlke, D.F. Hayes and D.K. Stevens. Reston, VA: American Society of Civil Engineers. 10 pp.
- Elliott, A. H., R. B. Alexander, G. E. Schwarz, U. Shankar, J. P. S. Sukias, and G. B. McBride. 2005. Estimation of nutrient sources and transport for New Zealand using the hybrid mechanistic-statistical model SPARROW. Journal of Hydrology (New Zealand) 44 (1): 1–27.
- Environment and Climate Change Canada (ECCC). 2016a. Historical climate data. http://climate.weather.gc.ca/index_e.html (accessed February, 2016).
- Environment and Climate Change Canada (ECCC). 2016b. HYDAT database: National water data archive. https://ec.gc.ca/rhc-wsc/default.asp?lang=En&n=9018B5EC-1 (accessed February, 2016).
- Homer, C., J. Dewitz, J. Fry, M. Coan, N. Hossain, C. Larson, N. Herold, A. McKerrow, J. N. VanDriel, and J. Wickham. 2007. Completion of the 2001 National Land Cover Database for the conterminous United States. Photogrammetric Engineering and Remote Sensing 73 (4): 337–341.
- Hong, B., D. P. Swaney, and R. W. Howarth. 2011. A toolbox for calculating net anthropogenic nitrogen inputs (NANI). Environmental Modelling & Software 26(5): 623–633.
- Jenkinson, W. J., and G. A. Benoy. 2015. Red—Assiniboine Basin SPARROW model development technical document. Ottawa, ON: National Research Council of Canada, 65 pp. https://www.ijc.org/en_IWI_Publications (accessed August, 2015).
- Johnston, C.M., T. G. Dewald, T. R. Bondelid, B. B. Worstell, L. D. McKay, A. Rea, R. B. Moore, and J. L. Goodall. 2009. Evaluation of catchment delineation methods for the medium-resolution national hydrography dataset. US Geological Survey Scientific Investigations Report 2009–5233, 88 pp. http://pubs.usgs.gov/sir/2009/5233/ (accessed July, 2015).
- Kling, H. J., S. B. Watson, G. K. McCullough, and M. P. Stainton. 2011. Bloom development and phytoplankton succession in Lake Winnipeg: A comparison of historical records with recent data. Aquatic Ecosystem Health and Management 14(2): 219–224.
- Kronvang, B., J. Audet, A. Baattup-Pedersen, H. S. Jensen, and S. E. Larsen. 2012. Phosphorus loads to surface water from bank erosion in a Danish lowland river basin. Journal of Environmental Quality 41 (2): 304–313.
- Laitta, M. 2010. Canada–US transboundary hydrographic data harmonization efforts gain momentum. http://nhd.usgs.gov/Canada-US_Hydro_Harmonization.pdf (accessed July, 2015).
- Li, S., J. A. Elliott, K. H. D. Tiessen, J. Yarotski, D. A. Lobb, and D. N. Flaten. 2011. The effects of multiple beneficial management practices on hydrology and nutrient losses in a small watershed in the Canadian Prairies. Journal of Environmental Quality 40(5): 1627–1642.
- Mahfouf, J.-F., B. Brasnett, and S. Gagnon. 2007. A Canadian precipitation analysis (CaPa) project: Description and preliminary results. Atmosphere-Ocean 45(1): 1–17.
- Maupin, M. A., and T. Ivahnenko. 2011. Nutrient loadings to streams of the continental United States from municipal and industrial effluent. Journal of the American Water Resources Association 47(5): 950–964.
- McCullough, G. K., S. J. Page, R. H. Hesslein, M. P. Stainton, H. J. Kling, A. G. Salki, and D. G. Barber. 2012. Hydrological forcing of a recent trophic surge in Lake Winnipeg. Journal of Great Lakes Research 38 : 95–105.
- Moore, R. B., C. M. Johnston, R. A. Smith, and B. Milstead. 2011. Source and delivery of nutrients to receiving waters in the Northeastern and Mid-Atlantic regions of the United States. Journal of the American Water Resources Association 47(5): 965–990.
- Moore, R. B., and T. G. Dewald, 2016. The road to NHDPlus — Advancements in digital stream networks and associated catchments. Journal of the American Water Resources Association 1–10. DOI: 10.1111/1752-1688.12389.
- Mueller, D. K., B. C. Ruddy, and W. A. Battaglin. 1997. Logistic model of nitrate in streams in the upper-midwestern United States. Journal of Environmental Quality 26(5): 1223–1230.
- National Centers for Environmental Information (NCEI). 2016. Climate of the US. http://www.ncdc.noaa.gov/ (accessed February, 2016).
- Natural Resources Canada (NRCan). 2003. Atlas of Canada 1,000,000 national frameworks data, hydrology – drainage areas). http://www.geogratics.gc.ca/ (accessed July, 2015).
- Natural Resources Canada (NRCan). 2009. National Hydro Network (NHN). http://www.geobase.ca/ (accessed July, 2015).
- Prairie Farm Rehabilitation Agency (PFRA). 2008. PFRA watershed project – Areas of non-contributing drainage. Regina, SK: Agriculture and Agri-Food Canada.
- Preston, S. D., R. B. Alexander, and D. M. Wolock. 2011. SPARROW modeling to understand water-quality conditions in major regions of the United States: A featured collection introduction. Journal of the American Water Resources Association 47(5): 887–890.
- Reckhow, K. H., M. N. Beaulac, and J. T. Simpson. 1980. Modeling phosphorus loading and lake response under uncertainty: A manual and compilation of export coefficients. USEPA Report EPA-440/5-80-011. Office of Water Regulation, Criteria and Standards Division. Washington, DC: USEPA.
- Robertson, D. M., and D. A. Saad. 2011. Nutrient inputs to the Laurentian Great Lakes by source and watershed estimated using SPARROW watershed models. Journal of the American Water Resources Association 47 (5): 1011–1033.
- Robertson, D. M., and D. A. Saad. 2013. SPARROW models used to understand nutrient sources in the Mississippi/Atchafalaya river basin. Journal of Environmental Quality 42(5): 1422–1440.
- Robertson, D. M., D. A. Saad, and G. E. Schwarz. 2014. Spatial variability in nutrient transport by HUC8, state, and subbasin based on Mississippi/Atchafalaya River Basin SPARROW models. Journal of the American Water Resources Association 50 (4): 988–1009.
- Robertson, D. M., G. E. Schwarz, D. A. Saad, and R. B. Alexander. 2009. Incorporating uncertainty into the ranking of SPARROW model nutrient yields from Mississippi/Atchafalaya River Basin watersheds. Journal of the American Water Resources Association 45(2): 534–549.
- Ruddy, B. C., D. L. Lorenz, and D. K. Mueller. 2006. County-level estimates of nutrient inputs to the land surface of the conterminous United States, 1982–2001. US Department of the Interior, US Geological Survey.http://pubs.usgs.gov/sir/2006/5012/. Accessed 29 May 2016.
- Saad, D. A., G. E. Schwarz, D. M. Robertson, and N. L. Booth. 2011. A multi-agency nutrient dataset used to estimate loads, improve monitoring design, and calibrate regional nutrient SPARROW models. Journal of the American Water Resources Association 47(5): 933–949.
- Schindler, D. W., R. E. Hecky, and G. K. McCullough. 2012. The rapid eutrophication of Lake Winnipeg: Greening under global change. Journal of Great Lakes Research 38(Suppl. 3): 6–13.
- Schwarz, G. E., A. B. Hoos, R. B. Alexander, and R. A. Smith. 2006. The SPARROW surface water-quality model: Theory, application and user documentation. US Geological Survey Techniques and Methods Report, Book 6, Chapter B3. Reston, VA: USGS.
- Schwede, D. B., R. L. Dennis, and M. A. Bitz. 2009. The Watershed Deposition Tool: a tool for incorporating atmospheric deposition in water-quality analyses. Journal of the American Water Resources Association 45(4): 973–985.
- Sekely, A. C., D. J. Mulla, and D. W. Bauer. 2002. Streambank slumping and its contribution to the phosphorus and suspended sediment loads of the blue earth river. Minnesota Journal of Soil and Water Conservation 57 (5): 243–250.
- Shrestha, R. R., Y. B. Dibike, and T. D. Prowse. 2012. Modeling climate change impacts on hydrology and nutrient loading in the Upper Assiniboine catchment. Journal of the American Water Resources Association 48(1): 74–89.
- Smith, R. A., G. E. Schwarz, and R. B. Alexander. 1997. Regional interpretation of water-quality monitoring data. Water Resources Research 33(12): 2781–2798.
- United States Environmental Protection Agency (USEPA). 2012. Atmospheric modeling and analysis research: Watershed deposition modeling tool. http://www.epa.gov/air-research/watershed-deposition-tool-air-quality-impacts (accessed February, 2016).
- United States Geological Survey (USGS). 2016. National Water Information System. http://waterdata.usgs.gov/nwis/rt (accessed February, 2016).
- University of Wisconsin-Madison, College of Agricultural and Life Sciences (UW-CALS). 2005. The Wisconsin Buffer Initiative: A Report to the Natural Resources Board of the Wisconsin Department of Natural Resources. http://www.faculty.nelson.wisc.edu/people/nowak/reports/nrbFinalReport.pdf (accessed September, 2013).
- Walker Jr., W. W. 1981. Empirical methods for predicting eutrophication in impoundments. Report 1. Phase I. Data base development. US Army Corps of Engineers. Concord, MA.
- Yang, Q., L. F. Leon, W. G. Booty, I. W. Wong, C. McCrimmon, P. Fong, P. Michiels, J. Vanrobaeys, and G. A. Benoy. 2014. Land use change impacts on water quality in three Lake Winnipeg watersheds. Journal of Environmental Quality 43 (5): 1690–1701.