Abstract
Information on the sources of sediment is required both for the targeting, development and implementation of best management practices (BMPs) in agricultural regions and for understanding the nature of sediment dynamics within watersheds. Investigations have shown that a number of physical and biogeochemical sediment properties can be used as fingerprints to trace sediment back to its source. Three groups of fingerprints – spectral reflectance (colour), particle morphology (size and shape), and fallout radionuclides (137Cs) – were used to fingerprint the sources of sediment in a predominantly agricultural watershed (14.5 km2) in Atlantic Canada over a 7-year period. Both spectral reflectance and fallout radionuclides were successful in discriminating between sources of sediment identified within the watershed. In contrast, fingerprints based on particle morphology were not able to discriminate between any of the sources of sediment. A composite fingerprint including both spectral reflectance and radionuclide fingerprints was used to estimate the relative contributions from agricultural topsoil (i.e. rill and inter-rill erosion), agricultural subsurface (i.e. streambank and gully erosion) and forested areas. Sediment fingerprinting indicated that topsoil derived from agricultural land was the greatest contributor (77.2%; 95% credible interval (CI95%) [35.5, 92.5]) to suspended sediment collected at the outlet of the watershed. Streambanks and gullies within agricultural areas were also identified as important sources of sediment (17.4%; CI95% [3.1, 46.9]), while forested land was a minor source of sediment in the watershed (3.2%; CI95% [0.2, 18.9]). The fingerprinting results demonstrate that BMPs that target soil erosion in agricultural fields will result in the greatest reduction in the export of sediment from the watershed. This study also established that adjusting the spatial scale at which suspended sediment samples are collected would provide more detailed information on the location of the sources of sediment (i.e. identify sources at the sub-watershed level) and lead to better targeting of BMPs.
Des informations sur les sources des sédiments sont nécessaires pour cibler, le développer et mettre en œuvre de meilleures pratiques de gestion (MPG) dans les régions agricoles et pour comprendre la dynamique des sédiments dans les bassins versants. Des enquêtes ont montré qu'un certain nombre de propriétés physiques et biogéochimiques des sédiments peuvent être utilisées comme empreintes pour retracer la source des sédiments. Trois groupes d’empreintes ont été utilisées, y compris la réflexion spectrale (couleur), la morphologie des particules (taille et forme) et les radionucléides (137Cs) ont été utilisés dans un bassin versant à prédominance agricole (14,5 km2) du Canada atlantique sur une période de sept ans. La réflexion spectrale et les radionucléides ont permis de déterminer les sources de sédiments identifiés dans le bassin hydrographique. En revanche, les empreintes basées sur la morphologie des particules n’ont pas pu discriminer les sources de sédiments. Une empreinte composite incluant à la fois la réflexion spectrale et les empreintes basés sur les radionucléides a été utilisée pour estimer les apports relatifs de la couche superficielle de sol agricole (i.e. érosion "rill" et "interill"), de la couche de sub-surface agricole (i.e. érosion de berge et ravinement) et des zones boisées. Les empreintes des sédiments indiquent que couche arable a été le plus grand contributeur (77,2%) de sédiments en suspension collectés à la sortie du bassin versant. Les berges et les ravins dans les zones agricoles ont également été identifiés comme une source importante de sédiments (17,4%), tandis que les terres boisées ont été une source mineure de sédiments dans le bassin versant (3,2%). Cette étude a également reconnu que l'échelle spatiale à laquelle les échantillons de sédiments en suspension sont collectés doit être intégrée aux données de charge de sédiments pour évaluer adéquatement les MPG.
Introduction
Atlantic Canada has experienced some of the most severe erosion as compared to other agricultural regions in Canada (King et al. Citation2000). In particular, soil erosion and sediment delivery to surface water in the Upper Saint John River Basin of northwestern New Brunswick has been recognized as a major environmental issue (Chow et al. Citation2000). The high soil erosion and sediment delivery in this region is a result of a combination of factors. Firstly, the agricultural land in this region is characterized by rolling to undulating terrain, with long and continuous slopes comprised of easily erodible soils (Su et al. Citation2011). Secondly, the humid cold continental climate results in a hydrological regime that is dominated by snowmelt in the spring and severe rainstorms during the summer and autumn. Finally, potato cultivation (planting, harvesting and tilling) often occurs parallel to the predominant slope direction (Chow et al. Citation1999). The potato production system coupled with the moist climate and highly erodible soil and landscape results in extensive soil erosion in this area.
The Black Brook Watershed (BBW), a small sub-watershed of the Upper Saint John River Basin, has been the focus of several studies investigating the impacts of agricultural production (potato production in particular) on both the rates of soil erosion and in-stream sediment dynamics. Past studies in the BBW have shown significant soil erosion in cultivated fields, and Saini and Grant (Citation1980) and Chow et al. (Citation1990) concluded that the average annual soil losses for continuous potato planting were approximately 1700 Mg km−1 yr−1 and 120 to 2430 Mg km−1 yr−1, respectively. In contrast, the 2003–2007 average suspended sediment yield at the outlet of the watershed was measured at only 180 Mg km−1 yr−1 (Chow et al. Citation2011). Furthermore, Chow et al. (Citation2011) compared suspended sediment loads in the BBW to other nearby watersheds and found that suspended sediment load increased with increasing agricultural intensity. Therefore, it has been assumed that in-stream suspended sediment is originating primarily from cultivated fields. However, discrepancies between field-level erosion estimates (Saini and Grant Citation1980; Chow et al. Citation1990) and in-stream monitoring of sediment yield (Chow et al. Citation2011) often occur (Wolman Citation1977), which highlights that other tools are needed to better examine the linkage between the sources of sediment and the in-stream sediment collected at the outlet of the BBW. Increasingly, sediment fingerprinting is being used to complement both in-field estimates of soil erosion and downstream sediment budgets by providing information on the relative contributions of different sources to in-stream sediment (Walling Citation2005; Krishnappan et al. Citation2009). Therefore, the primary aim of this research was to use the sediment fingerprinting approach within the BBW to directly link potential sources of sediment to the suspended sediment being exported from the watershed.
Sediment fingerprinting
Sediment fingerprinting involves identifying the physical and/or biogeochemical fingerprints that distinctively characterize each source within a watershed (Klages and Hsieh Citation1975; Wall and Wilding Citation1976; Walling et al. Citation1979). By comparing the fingerprint of the suspended sediment to the fingerprint of the sources, the contribution of each potential source can be estimated. Fingerprint properties include: spectral reflectance (i.e. colour; e.g. Grimshaw and Lewin Citation1980; Martínez-Carreras et al. Citation2010), fallout radionuclides (e.g. Peart and Walling Citation1986; Walling and Woodward Citation1992; Olley et al. Citation1993), stable isotopes (e.g. McConnachie and Petticrew Citation2006; Rhoton et al. Citation2008; Reiffarth et al. Citation2016), elemental concentrations (e.g. Dutton et al. Citation2013; Koiter, Lobb et al. Citation2013), mineralogy (e.g. Motha et al. Citation2003) and magnetic properties (e.g. Blake et al. Citation2006). For more detailed reviews of the various sediment properties that can be used as fingerprints see Foster and Lees (Citation2000), Guzman et al. (Citation2013), Owens et al. (Citation2016) and Collins et al. (Citation2017). Ideally a fingerprint property needs to both be measurable (i.e. it exhibits analytical accuracy, precision and resolution) and not change as it is transported through a watershed (i.e. conservative behaviour) so that a direct comparison of fingerprint properties between the source and sediment can be made (Collins and Walling Citation2002; Walling Citation2005; Haddadchi et al. Citation2013). However, the assumption of a direct link between source and sediment has been shown to be tenuous as soil erosion and sediment transport processes are selective in terms of both particle size and organic matter. In addition, environmental conditions (e.g. pH) may differ between the headwaters and the watershed outlet. Both selectivity and changing environmental conditions can distort sediment fingerprints, making it difficult to link sources to sediment in a reliable and robust manner (Motha et al. Citation2002; Koiter, Owens et al. Citation2013; Sherriff et al. Citation2015).
Many fingerprinting studies use particle size, and sometimes organic matter content, correction factors or other methods, to account for differences in these properties between the source and sediment (see Laceby et al. Citation2017 for a review of the different methodologies). However, the use of such correction factors has been the subject of debate as it can introduce additional uncertainty into the source apportionment results (Smith and Blake Citation2014). Another approach is to use multiple groups of fingerprints, thereby providing some independent measures which may ultimately result in a more robust and reliable estimate of the relative contributions from a given source. The inclusion of multiple fingerprints, either within a single fingerprint group or across multiple groups of fingerprints, is part of the composite fingerprint approach which seeks to reduce spurious source–sediment linkages (Collins et al. Citation1997; Collins and Walling Citation2002). The focus of this study is the integration of fallout radionuclides, colour and morphological characteristics (i.e. shape and size) as fingerprints to determine the sources of sediment in the BBW.
Fallout radionuclides (FRNs), including cosmogenic (e.g. 7Be), anthropogenic (e.g. 137Cs) and airborne (e.g. 210Pb) sources, have been used extensively in the study of soil redistribution (e.g. Ritchie and McHenry Citation1990) and as sediment fingerprints (e.g. Walling and Woodward Citation1992). FRNs are deposited in association with precipitation and dry fallout and are quickly and strongly absorbed to soil particles. The distribution of FRNs in undisturbed soil profiles typically exhibits an exponential decrease with soil depth and, therefore, FRNs have the potential to discriminate between surface and subsurface sources (Owens et al. Citation1996). In contrast, in cultivated soils, through the mixing of the soil a uniform distribution through the plough layer is often observed (Ritchie and McHenry Citation1990) and can provide discrimination between surface-derived sources of sediment within non-cultivated and cultivated areas. For example, Wallbrink et al. (Citation1998) used 137Cs and 210Pbunsupported to identify the relative contributions of sediment originating from cultivated land, uncultivated pastoral land and channels/gullies. Furthermore, the physical processes of erosion are one of the main causes of the redistribution of FRNs across the landscape, and this can lead to contrasting activity concentrations between erosional and depositional areas within a localized area. In addition, FRNs are well suited for use in heterogeneous watersheds, since their concentrations are effectively independent of soil type and underlying geology (Walling Citation2005). However, research has demonstrated that there are situations which can limit the utility of FRNs to effectively discriminate between the potential sources of sediment as described above and, therefore, their inclusion within sediment fingerprinting studies needs to be given careful consideration (e.g. Smith and Blake Citation2014). This study will focus on 137Cs which is a radioisotope that is the product of nuclear weapons testing during the 1950s and 1960s (Perkins and Thomas Citation1980).
Colour is an important physical attribute that is used to describe and differentiate soils. Research suggests that colour has the potential to be relatively accurate in characterizing the properties of soil (Torrent and Barrón Citation1993; Islam et al. Citation2003). Variation in soil colour is caused by differences in organic matter, moisture conditions, chemical and biological weathering, and redox reactions (Udelhoven et al. Citation2003). Soil colour is affected by the decomposition of organic matter producing black and brown compounds (Konen et al. Citation2003). Minerals such as iron are known to result in red, brown and yellow hue values, and manganese, sulfur and nitrogen can form black mineral deposits (Viscarra Rossel et al. Citation2009). Soil texture plays a significant role in soil colour due to its influence on organic matter (Konen et al. Citation2003). For example, De Boer (Citation1997) used Munsell soil colour chart values to document the change in the sources of sediment accumulating in a lake following agricultural settlement in the Canadian prairies.
Both particle size and shape are important physical characteristics of soil and sediment, as both influence a particle’s movement in the environment (e.g. Shields Citation1936) and the absorption of nutrients and contaminants (e.g. Horowitz Citation1991). The particle size and shape of a potential source of sediment are the result of the parent material (e.g. depositional process), physical and chemical weathering (e.g. particle breakage), soil formation processes (e.g. translocation of clay) and the erosional history (Schafer Citation2008). Physical signatures such as particle size and shape offer many advantages to fingerprinting studies because these properties are easily measurable and readily identifiable (Krein et al. Citation2003; Davis and Fox Citation2009). For example, De Boer and Crosby (Citation1995) included clay-sized particles as part of their particle morphological assessment and demonstrated that differences in particle morphology could discriminate between agricultural and forest topsoil.
The present study is located in the BBW, a predominately agricultural watershed in northwestern New Brunswick, Canada. The motivation behind this study was to link in-field estimates of soil erosion to downstream estimates of sediment load and to identify the sources of sediment in the watershed. The objectives of this study were to: (1) assess the ability of colour, particle morphology and FRN fingerprints in discriminating between potential sources of sediment; and (2) quantify the relative contributions of sources of suspended sediment using sediment fingerprinting. It was hypothesized that colour, particle morphology and radionuclides would each offer an independent means of discriminating between potential sediment sources, and that rill/inter-rill erosion on agricultural land is the predominant source of sediment at the outlet of the BBW.
Materials and methods
Study area
The Black Brook Watershed is located near the town of Saint-André, New Brunswick (47°05’ to 47°09’ N, 67°43’ to 67°48’ W). The BBW has an area of 1450 ha (14.5 km2) and is characterized by an elongated shape (~7.5 km long and ~3.5 km wide) (DesRoches et al. Citation2014). Black Brook is a tributary of the Little River which discharges into the Saint John River near Grand Falls, New Brunswick. These watersheds are part of the Upper Saint John River Valley Ecoregion in the Atlantic Maritime Ecozone (Marshall et al. Citation1999). The climate is moderately cool boreal with a soil moisture regime ranging from humid to perhumid, with frost periods lasting for approximately 120 days (Mellerowicz et al. Citation1993). The mean annual temperature is 3.2°C (1981–2010, station identifier: 8104928; Environment and Climate Change Canada Citation2017). The region has a mean annual precipitation of 1104.1 mm with monthly averages ranging from a low of 67.5 mm in February to a high of 119.3 mm in July (Environment and Climate Change Canada Citation2017). Approximately one-quarter of the precipitation in this region falls as snow. Although monthly precipitation is relatively uniform from April to September, frequent summer storms tend to relocate large amounts of soil within the watershed. The elevation ranges from 180 to 260 m above sea level (Mellerowicz et al. Citation1993). The lower portion of BBW is characterized by a more prominent rolling topography compared to the central and upper portion, with slopes of 5–16% in the lower portion, 4–9% in the central portion and 1–6% in the upper portion (Mellerowicz et al. Citation1993).
Past glacial activity has characterized the western portion of the region with rill wash composed of mixed sand, stones, reworked till and veins of gravel and silt, and the eastern portion with underlain compact till with hummocks and ridges of stratified gravel, sand and silt (Mellerowicz et al. Citation1993). The underlying geology of the BBW is Ordovician and Silurian calcareous and argillaceous sedimentary rocks from the Matapedia Group (Rappol and Russell Citation1989). Bedrock is weakly deformed, showing evidence of very low-grade metamorphism (Carrol Citation2003). The base formations are dominated by thin-bedded, dark grey calcareous shale, siltstone, and fine-grained sandstone, calciluite, minor to medium bedded calcareous sandstone, and minor amounts of non-calcareous shale and siltstone deposited on a submarine slope environment (St. Peter Citation1978; Stringer and Pickerill Citation1980).
BBW is comprised of six mineral soils (Figure a) – Grand Falls (Orthic Humo-Ferric Podzol), Holmesville (Orthic Humo-Ferric Podzol), Interval (Gleyed Regosols), Muniac (Orthic Humo-Ferric Podzol), Siegas (Gray Luvisol) and Undine (Mini Humo-Ferric Podzol) – and one organic soil association – St. Quentin (Terric Mesisol or Terric Humisol). These soil associations vary substantially from one another with regards to mode of deposition, petrology, depth to compacted layer, texture, colour, drainage and coarse fragments.
Figure 1. Map of the Black Brook Watershed showing the main soil associations (a), land uses (b), and the approximate locations of both the source samples and the suspended sediment.
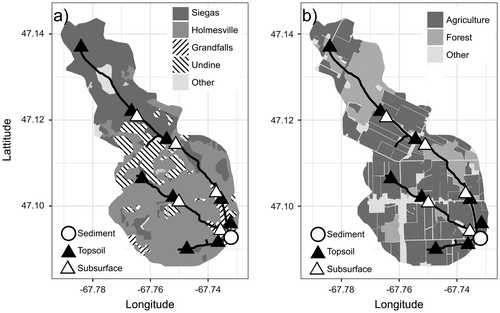
The two primary land uses in the BBW are agriculture and forest (Figure b). Approximately 65% (1050 ha) of the land is used for agriculture, with potato grown in rotation with grains being the dominant crops. A further 21% of the land is forested, with one major forested area at the top of BBW, while the rest is mainly riparian areas along the stream edges. The remaining 14% of the land is comprised of residential areas, wetlands and roads (Chow et al. Citation2011).
Collection of source materials and sediment
Within sediment fingerprinting studies, the potential sources of sediment are often identified based on the interactions among several factors including parent material, soil type, topography, hydrology, climate, weathering processes, amount and type of vegetation and anthropogenic activity. The source sampling design used in BBW was developed to take into account variation in fingerprint properties due to parent material, soil type, land use and erosional processes. Individual source sampling locations were selected based on visible evidence of erosion or soil degradation, distance and connectivity to the stream, access and safety. These source samples were divided between the two major land uses (agriculture and forest), and within each land use the source samples were further subdivided into topsoil (rill/inter-rill erosion) and subsurface (gully and streambank erosion) sources. All source samples were collected in the summer of 2009.
To represent topsoil (rill and inter-rill erosion) in non-cultivated areas, topsoil samples were collected from the soil surface (0–5 cm), while in cultivated areas the depth of sampling extended the entire depth of the plough layer (~0–40 cm) to account for the regular mixing of this layer due to cultivation. Topsoil samples were collected along transects which extended from the stream edge through the riparian zone and into nearby fields. Each transect was sampled parallel to the greatest slope gradient and consisted of 4–8 individual soil sampling locations, depending on the extent and complexity of the contributing area, and at each location six samples were collected and composited into a single sample. A total of nine transects were sampled within the BBW (see Figure for the locations) in order to get good spatial representation of sediment sources throughout the watershed, with a total of 60 topsoil samples (16 forest and 44 agricultural samples). A 3.2-cm (diameter) stainless steel soil-sampling probe was used to collect the majority of soil samples; however, shovels were used in places with many stones.
Subsurface samples (gully and streambank erosion) were collected in the same vicinity as the topsoil sources (Figure ). These samples were collected using a 10 cm × 10 cm box-core sampler along the full depth of the bank profile from the soil surface through to the base, in 10-cm increments. In total, three profiles at five locations were sampled for a total of 93 samples (16 forest and 77 agricultural samples). Forested areas of BBW are situated in the upper portion of the watershed where the streambanks are smaller, resulting in fewer samples. The rationale for using detailed depth profiles, as opposed to single composite samples, was to ensure full representation of each profile as a source of sediment and to aid in the process-based interpretation of fingerprints.
Suspended sediment samples were collected seasonally, excluding winter, from 2008 to 2014 at three locations along a 200-m reach at the outlet of the watershed. The morphology of this reach is characterized by a slope of 1%, width to depth ratio of 11.1 and bankfull depth of 9.4 m, and the channel bed substrate was comprised of 35.7% fine-grained sediment (< 2 mm) (Sutherland et al. Citation2012). In addition, Chow et al. (Citation2011) estimated the 2000–2007 mean annual total discharge to be 0.587 × 106 ± 0.069 × 106 m3 km−2 y−1 (± 1 standard deviation) and found a sediment yield of 181.6 ± 36.26 Mg km−2 y−1. Suspended sediment was collected during spring, summer and autumn periods, occurring from early May to mid-June, from mid-June to mid-September, and from mid-September to early November, respectively. Suspended sediment was collected using time-integrated suspended sediment samplers following the design of Phillips et al. (Citation2000) which have been used in numerous fingerprinting studies (e.g. Gruszowski et al. Citation2003; Gellis et al. Citation2017). The sediment samplers were anchored to the streambed using concrete blocks. Sediment samplers were inspected every 2 weeks for damage and blockages. A total of 64 suspended sediment samples were collected.
Laboratory analysis
Both suspended sediment and source material were analyzed for 137Cs activity, spectral reflectance (colour), and particle size and shape (morphology). Prior to analyses, source samples were air-dried and sieved through a 2-mm sieve to remove coarse fragments. Suspended sediment samples were left to settle for 7 days and air-dried after the clear supernatant was siphoned off. Following the procedure of Barthod et al. (Citation2015), a subsample was crushed and further sieved to < 63 μm to accommodate the spectral-reflectance analysis. The particle size fraction used in fingerprinting studies has the potential to bias the final source apportionment results and, therefore, needs to be given careful consideration (Collins et al. Citation2017; Laceby et al. Citation2017). Both hydraulic sorting (downstream fining) and attrition (particle abrasion and breakage) can make a direct comparison between source material and suspended sediment difficult due to changes in particle size. Sieving and removing coarse-grained particles (e.g. > 63 μm) from analyses is a common approach to make samples more directly comparable. In the BBW the < 2 mm fraction was used because of two main reasons. First, given the small drainage area of the BBW it is expected that the magnitude of hydraulic sorting would be minimal as compared to larger watersheds. Secondly, the soil parent material within the BBW is comprised of friable (i.e. low strength material) shales and as a result significant attrition would be expected and restricting the analyses to fine-grained material would be problematic as the particle size distribution of source material would not remain static. The relationship between fingerprint values and grain size for the potential sources of sediment in the BBW was not examined experimentally and, therefore, differences in grain size between the sources and suspended sediment may result in additional uncertainty in the final apportionment results.
Spectral reflectance
The colour of both source material and sediment samples was measured using spectral readings over a 350–2500-nm wavelength range using a spectroradiometer (ASD FieldSpec Pro, Analytical Spectral Device Inc., Boulder, CO, USA, at the University of Manitoba, Winnipeg, Canada). A 10-cm-diameter transparent plastic support was used to hold the sample that was smoothed with a straight edge in order to reduce shading. The Spectralon standard (white reference) was used to calibrate the spectroradiometer prior to each measurement. The samples were illuminated with a white light source (a 1000-W quartz halogen lamp mounted on a tripod at a distance of 10 cm), and a fibre optic cable was mounted 2 cm away and at an angle of 45° from the sample.
The absolute bidirectional reflectance spectra were obtained using RS3 software (Analytical Spectral Device Inc., Boulder, CO, USA) by multiplying the raw reflectance spectra by the certified reflectivity of the Spectralon standard. Ten absolute reflectance spectra were collected for each sample and averaged. The Commission Internationale de l’Eclairage’s (CIE) method for calculating colour coefficients was used to determine spectral-reflectance measurements over the visible wavelength range (CIE 1931). Using the CIE colour system, differences in colours were represented by x and y, chromaticity coordinates and the brightness (Y). The values of x and y contain saturation and hue information and specify colour variations from blue (B) through green (G) to red (R).
Conversion of the RGB colour values to CIE x, y and Y coefficients followed the procedures and methods used by Martínez-Carreras et al. (Citation2010). The RGB colour coefficients were calculated from the spectral reflectance by averaging reflectance data corresponding to the blue, green and red Landsat bands (Martínez-Carreras et al. Citation2010). The RGB colour values were then transformed to the rest of the colour space models (e.g. CIE XYZ) using methods developed by Viscarra Rossel et al. (Citation2006). The averaged reflectance data were multiplied by 255 to obtain the 8-bit pixel colours. The coefficient z identifies colour differences between samples and is a virtual component of the primary spectra; L represents brightness; and a*, b*, u*, and v* represent the chromaticity coordinates as opponent red–green and blue–yellow scales (Viscarra Rossel et al. Citation2006; Barthod et al. Citation2015). The coefficients c* and h* are transformations of a*and b* into cylindrical coordinates. A description of the 15 colour coefficients is given in Table .
Table 1. Description of spectral-reflectance colour coefficients used as fingerprints.
Particle size and shape
Particle size and shape were measured using image analysis capable of measuring particles ranging from 2 to 600 μm in diameter (Sympatec QICPIC with a LIXELL liquid dispersal unit, Clausthal-Zellerfeld, Germany, at the Fredericton Research and Development Centre, Agriculture and Agri-Food Canada, New Brunswick, Canada). Both source and suspended sediment samples were disaggregated using a solution of sodium hexametaphosphate and shaking (Kroetsch and Cang Citation2007) prior to measurement, and samples were measured in triplicate and averaged. Three measures of particle size were determined: median particle diameter (by volume), specific surface area (assuming a particle density of 2.7 g cm−3) and average Feret length. In addition, three measures of particle shape were determined: sphericity, convexity and aspect ratio.
Radionuclides
The 137Cs activity was measured using gamma spectrometry with high-purity germanium gamma spectrometers (Canberra BE3830, Meriden, CT, USA, at the Fredericton Research and Development Centre, Agriculture and Agri-Food Canada, New Brunswick, Canada). The suspended sediment samples were analyzed on a germanium well detector, while the soil and streambank samples were run on a broad-energy germanium detector. Samples were counted for 24 h, while some low-mass samples were counted for 48 h. Concentrations of 137Cs activity were decay-corrected, with all source and sediment samples corrected to 1 January 2010. Prior to analysis, each detector was calibrated using reference standards obtained from the International Atomic Energy Agency (Vienna, Austria).
Fingerprint selection
For both colour and particle morphology fingerprint groups, a multi-step process was used to select a robust subset of fingerprints that can discriminate between the four potential sources of sediment. Two important assumptions of the source appointment model used include the normality of the fingerprint distribution within each potential source of sediment and the conservative behaviour of the fingerprints during transport though the watershed. Due to the low sample numbers, normality was assessed visually and any fingerprints that showed major deviations from normal were omitted from further analysis. Similar to the commonly used range test (e.g. Collins, Zhang, McChesney et al. Citation2012), the conservative behaviour was assessed by plotting source data for all two-way unique combinations (i.e. bi-plot) of fingerprints to create mixing polygons (i.e. convex hull; see Figure for example bi-plots). In cases where suspended sediment samples fell outside of the mixing polygon (i.e. fingerprint values above or below the source data values), this demonstrated that there was an issue with the behaviour of a fingerprint and it was omitted from further analysis. Past studies have also used additional selection criteria including an assessment of variability and an assessment of paired tracer behaviour within both source and sediment samples (e.g. Pulley et al. Citation2015).
Figure 2. Three example bi-plots demonstrating the colour and radionuclide fingerprint relation for the four potential sediment source groups (error bars represent mean ± 1 standard deviation) and suspended sediment (points). Dashed polygon delineates the mixing polygon (i.e. convex hull) based on non-summarized source fingerprint data. See Table for a description of the spectral-reflectance colour coefficients b*, v*, u*, R, and B).
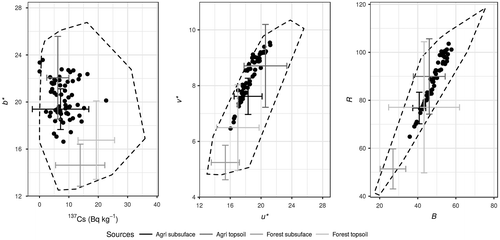
The fingerprints that meet the apportionment model assumptions were then further narrowed down using the two-stage statistical verification procedure outlined by Collins et al. (Citation1997). In the first stage, the Kruskal–Wallis test is used to examine the existence of inter-category contrasts among sources (Collins et al. Citation1997). Any fingerprints that were not able to discriminate between at least two of the sources (P-value > 0.01) were removed. The second stage used a forward stepwise discriminant function analysis based on the minimization of the Wilks’ lambda value (R package klaR v. 0.6-12; Weihs et al. Citation2005). A niveau of 0.01 (threshold level for the approximate F-test decision) was used as the threshold to add fingerprints in a stepwise fashion. This analysis identified the combination of fingerprint properties that results in the minimum number of fingerprints necessary to provide the greatest discrimination between sources (i.e. it removed redundant fingerprints; Collins and Walling Citation2002). Linear discriminant analysis was used to assess the discriminatory power of the fingerprints using a jackknifed (i.e. leave one out) cross-validation procedure (R package MASS v. 7.3-45; Venables and Ripley Citation2002). All statistical analysis was undertaken using R Statistical Software (v. 3.3.2; R Core Team Citation2016) through RStudio Integrated Development Environment (v. 0.97; RStudio Citation2016).
Source apportionment
Both frequentist (e.g. Collins et al. Citation1997) and Bayesian (e.g. Palmer and Douglas Citation2008) mixing models have been used to estimate the relative contributions from a given source to the collected in-stream sediment. Also, research has shown that the apportionment results are sensitive to the mixing model used (e.g. Haddadchi et al. Citation2013). The relative contribution from each potential source of sediment to the suspended sediment collected at the outlet of the BBW was estimated using the MixSIAR model framework (v. 3.1.7; Stock and Semmens Citation2016a). MixSIAR is an ecological mixing model framework designed to investigate food-web and predator–prey relationships (i.e. diet composition) using stable isotopes as fingerprints. However, the model framework can be applied to other environmental mixtures and fingerprints, as long as the assumption of linear mixing is met. MixSIAR uses a Bayesian inference and as a result uses probability density distributions to interpret source contributions (Moore and Semmens Citation2008; Nosrati et al. Citation2014). The statistical sampling method used by MixSIAR to approximate source contributions is Monte Carlo, which is able to propagate uncertainty in model inputs into uncertainties in the model. The model is fit using Markov chain Monte Carlo (MCMC) and the Gibbs Sampler algorithm. The MCMC simulates draws that are slightly dependent and are approximated from a posterior distribution and involve repeatedly guessing values of sediment source apportionment (Parnell et al. Citation2010; Phillips et al. Citation2014). In addition, MixSIAR allows the user to specify the mixture data structure (i.e. categorical covariates), to add enrichment and/or concentration dependence correction factors, to account for sources of fingerprint variability (i.e. covariance structure), and to include prior knowledge of the system through the use of informative priors (Stock and Semmens Citation2016a).
The model options and data structure used to estimate the relative contribution of sources to the downstream suspended sediment are listed below. The year in which the suspended sediment was collected was included as a random factor to account for variation between years. Both residual error (i.e. random and unexplained) and process error (i.e. variation in sediment sources between individual sediment samples) terms were included as a multiplicative error term (Stock and Semmens Citation2016b). Trophic enrichment and concentration dependence correction factors (accounts for the non-conservative behaviour of isotopes/fingerprints) were omitted from the model (i.e. set to 0). The model was run for 300,000 iterations with a burn-in of 200,000 using three chains thinned by 100 iterations. An uninformative prior distribution was specified. Model convergence was assessed using both the Gelman–Rubin (< 1.05) and Geweke (standard z-score; 5% outside ± 1.96) diagnostic tools. All plots were created using the R package ggplot2 (v. 2.2.1; Wickham Citation2009).
Results
Fingerprint selection
Spectral reflectance
A summary of the spectral-reflectance colour coefficients for the potential sources of sediment as well as the suspended sediment can be found in Table . Spectral-reflectance colour coefficients a* and c* (chromatic coordinate opponent red–green scales and CIE hue) did not pass the assumption of normality and were omitted from further analysis. Furthermore, colour coefficients x (chromatic coordinate x), y (chromatic coordinate y) and h* (CIE chroma) were also removed from analysis due to non-conservative behaviour (i.e. sediment fingerprint values fell outside the range of the sources). The results of the Kruskal–Wallis test demonstrate that all 10 of the remaining colour fingerprints showed inter-category contrast (Table ). Using linear discriminant analysis, it was demonstrated that these 10 colour fingerprints provide relatively good discrimination between agricultural topsoil and subsurface sources, but there is substantial overlap between forest topsoil and subsurface sources (Figure ). This suggests that the two forested sources should be collapsed into a single forest land-use source. Following this reclassification of the forest sources, all 10 of the colour fingerprints still demonstrated inter-category contrasts (Table ) and, overall, there is good discrimination among the three sources of sediment (Figure ).
Table 2. Mean values (standard deviation) for sediment fingerprint properties for each potential source of sediment and the suspended sediment.
Table 3. Fingerprint property summary considering four potential sources of sediment, with results of the Kruskal–Wallis test for inter-category contrasts and source sample reclassification rate using individual properties.
Figure 3. Linear discriminant analysis (LDA) score plots of the first two linear discriminant functions (LD 1 and LD 2) for the colour (a) and morphology (b) fingerprints considering four potential sources of sediment. The radionuclide (137Cs) data (c) have been randomly spread across the y-axis (vertically) to improve visualization of the groupings.
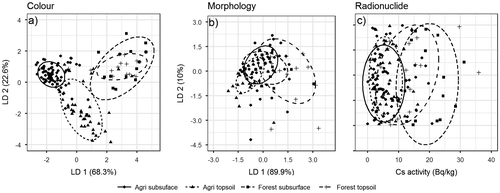
Table 4. Fingerprint property summary considering three potential sources of sediment, with results of the Kruskal–Wallis test for inter-category contrasts and source sample reclassification rate using individual properties.
Figure 4. Linear discriminant analysis (LDA) score plots of the first two linear discriminant functions (LD 1 and LD 2) for the colour (a) and morphology (b) fingerprints individually considering three potential sources of sediment. The radionuclide (137Cs) data (c) have been randomly spread across the y-axis (vertically) to improve visualization of the groupings.
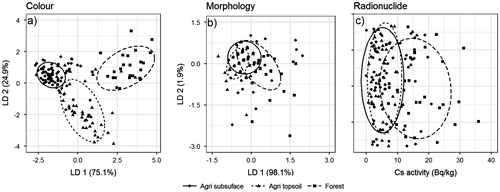
Particle morphology
There was evidence of hydraulic sorting (i.e. downstream fining) within the BBW, as the measures of particle size were consistently smaller in the sediment as compared to the sources (Table ). For example, the mean particle diameter of the collected suspended sediment was 33.1 μm (median diameter: 31.4 μm) whereas the mean diameter of the source material was 54.8 μm (median diameter: 37.0 μm). As a result, all measures of particle size (diameter, specific surface area and Feret length) were considered not to behave in a conservative behaviour and were omitted from all subsequent analyses. The results of the Kruskal–Wallis test demonstrate that all three shape fingerprints showed inter-category contrast (Figure ). These three shape fingerprints were not successful in discriminating among the four sources of sediment. Overall, the cross-validation reclassification was able to correctly identify 46.2% of the source samples to their source group (Table ). The shape fingerprints reclassified most samples, regardless of the actual source grouping, as an agricultural subsurface source. Collapsing the two forest sources into a single forest land-use source did not improve the overall accuracy of the reclassification (overall 46% correct reclassification; Figure ). Collapsing the sources into two groups based on either land use (agriculture and forest) or topsoil/subsurface only marginally improved the overall ability of shape fingerprints to discriminate between potential sources. Grouping samples by land use resulted in only three forest samples (8%) being correctly reclassified. Similarly, by grouping source samples as belonging to either surface or subsurface sources, all topsoil samples but one were misclassified as subsurface.
Radionuclides
The 137Cs fingerprint did demonstrate inter-category contrast (Table ). Similar to the shape fingerprints, the 137Cs fingerprint reclassified most samples as an agricultural subsurface source (Figure and Table ). Combining the forest topsoil and subsurface samples in a single forest land-use source (Table ) resulted in little improvement in the reclassification, with all agriculture topsoil samples being misclassified as agriculture subsurface and only 34% of the forest samples being correctly classified (Figure ). Similar to the shape fingerprints, collapsing the four sediment sources into two groupings based on surface and subsurface sources demonstrated no improvement in the reclassification, as all the samples were reclassified as subsurface sources. In contrast to the shape fingerprints, dividing the samples into forest and agriculture groups did improve the overall discrimination, with 97% and 28% of agricultural and forested samples, respectively, being correctly reclassified. Previous research has demonstrated a strong negative relation between 137Cs activity and particle size, which has implications for the use of this property as a fingerprint (e.g. He and Walling Citation1996). However, the results from this study demonstrated that particle size was a non-conservative fingerprint, whereas 137Cs was a conservative fingerprint (once corrections for radioactive decay were undertaken). This situation identifies that some methods frequently used to identify non-conservative fingerprints may not be adequate and are further complicated by the issue of inconsistent particle size and fingerprint relations (e.g. Russell et al. Citation2001).
Composite fingerprint
Using all the fingerprints that met the mixing model assumptions and demonstrated inter-category contrasts (10 colour, three shape and one FRN fingerprints) showed considerable overlap in the forest topsoil and subsurface sources (Figure ). There was an improvement in the overall discrimination if the two forest sources are combined (Figure ). All fingerprints that showed inter-category contrasts (i.e. Kruskal–Wallis test) among the three source groups identified were entered in the stepwise discriminant function analysis. This analysis resulted in removal of the colour coefficients b* (chromatic coordinate opponent blue–yellow scales) and Y (brightness) and all shape parameters. The remaining eight colour parameters and 137Cs were successfully able to discriminate among the three sources of sediment. Overall, using the nine fingerprints the cross-validation reclassification was able to correctly identify 86% of the source samples to their source group (Table ). There was good discrimination between the forested and agricultural land use but there was some overlap between the samples originating from agricultural land uses as approximately 20% of the agricultural topsoil samples were misclassified as agricultural subsurface.
Figure 5. Linear discriminant analysis (LDA) score plots of the first two linear discriminant functions (LD 1 and LD 2) for the combined fingerprints (colour + morphology + radionuclide) considering four (a) or three (b) potential sources of sediment.
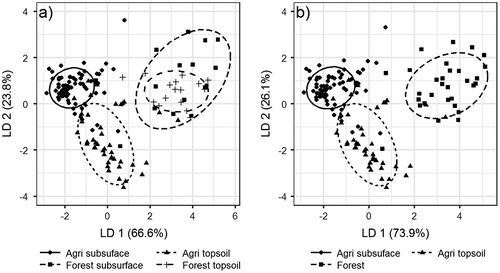
Table 5. Step-wise discriminant function analysis results.
Sediment source apportionment
The composite fingerprint selected by the stepwise discriminant function analysis (v*, R, L, u*, B, G, X, Z and 137Cs) was used to estimate the contributions of the three potential sources of sediment. The source apportionment results from MixSIAR are shown in Figure and results are presented as the median value and the 95% credible interval (CI95%) of the posterior distribution. At the outlet of the BBW agricultural topsoil was found to be the predominant source of sediment, accounting for 77.2% (CI95% [35.5, 92.5]) of the suspended sediment. Agricultural subsurface sources were the second largest contributor to the suspended sediment, accounting for 17.4% (CI95% [3.1, 46.9]). Overall, the contributions from forested areas to the suspended sediment at the BBW outlet were small and only accounted for 3.2% (CI95% [0.2, 18.9]).
Discussion
Source discrimination
The use of multiple groups of properties in a composite fingerprint is common in sediment fingerprinting studies (e.g. Collins and Walling Citation2002). The inclusion of multiple groups of fingerprints often allows for better discrimination between sources, but it also may provide more robust results as both the underlying processes leading to the development of the fingerprint and its behaviour in the environment differ between groups. However, Pulley et al. (Citation2017) demonstrated that both small differences in fingerprint values between sources and high within-source variability can introduce considerable uncertainty in sediment apportionment results. This was the rationale behind reclassifying the forest topsoil and subsurface sources into a single forest source. Despite the reclassification of sources, there was still overlap in the fingerprints and complete discrimination was not achieved. This is not unexpected, and is similar to the results of many other sediment fingerprinting studies (e.g. Walling et al. Citation1999). This may account, in part, for the large confidence intervals surrounding the estimates of source apportionment (Figure ).
The spectral-reflectance group of fingerprints had the best discriminatory power among the three groups of fingerprints used in this study. Organic matter content is likely one of the main contributors to the variation in spectral reflectance fingerprints between forest and agricultural land uses. Organic matter content is generally higher under forest as compared to agriculture as the forest has perennial vegetation and no soil disturbance, which leads to the development of an organic-rich, dark-brown or black surface Ah horizon (Agriculture and Agri-Food Canada Citation1998). In contrast, the regular harvesting of crops and mixing of the topsoil both reduce organic matter input but also increase the rate of decomposition, resulting in a lighter brown Ap horizon. Furthermore, organic matter content decreases with soil depth, often resulting in a change in colour with depth and thus providing discrimination between topsoil and subsurface soils (Agriculture and Agri-Food Canada Citation1998). The lack of discrimination between forest topsoil and subsurface sources may, in part, be due to shallow gullies and streambanks (< 15 cm) as compared to the deeper gullies and streambanks (>30 cm) found in the agricultural areas.
Holmesville (Orthic Humo-Ferric Podzol) is the most prevalent soil association in the BBW (648.7 ha), occupies the majority of the southern portion of the watershed and is primarily associated with agriculture (Figure ). The second most prevalent soil association is Siegas (Gray Luvisol), which is primarily found in the northern portion of BBW (477.3 ha) and contains the majority of the forest (Figure ). Spectral-reflectance fingerprints were able to distinguish between topsoil and subsurface soil as the Holmesville sol association is typically reddish-brown in colour near the surface and both increases in yellow hue and decreases in chroma with depth (Mellerowicz et al. Citation1993). Furthermore, differences in spectral reflectance have also been linked to texture, and the Holmesville soil association has a coarser textured soil (sandy-loam to loam) as compared to the Siegas soil association (clay-loam to heavy loam) (Mellerowicz et al. Citation1993).
Particle morphology fingerprints demonstrated very little discriminatory power between any of the sources regardless of how the source samples were grouped. The two predominant soil types found in BBW have very similar parent material petrology (i.e. particle strength) and mode of deposition (i.e. deposited by glaciers). Both of these have been demonstrated to be important factors influencing particle shape (Santamarina and Cho Citation2004). Because both soil associations were formed on glacial till, this suggests that other soil formation processes were not sufficient to impart a unique particle shape to different soil types or between surface and subsurface soil. Furthermore, the range of the image analysis instrumentation (2–600 μm) used in this study excluded clay-sized particles and may have contributed to the lack of discrimination between sources.
Typically the inclusion of FRNs in sediment fingerprinting studies is to provide discrimination between surface (e.g. topsoil and inter-rill erosion) and subsurface (e.g. streambank and gully erosion) sources, as the activity of FRNs quickly decreases with depth (e.g. Owens et al. Citation1996; Smith and Dragovich Citation2008). However, 137Cs showed the best discrimination between the agricultural and forested samples in this study, and not between surface and subsurface sources. The differentiation between agricultural and forest land use is likely caused by the regular mixing of the soil in the agricultural regions, due to tillage, which homogenizes the surface soil (with its high 137Cs activity) and the soil at depth (which is low in 137Cs activity), which results in an overall lower 137Cs activity. Furthermore, the majority of the forested regions of the BBW are closer to the headwaters and where the streambanks and gullies are much smaller (< 15 cm) as compared to the watershed outlet (> 30 cm). As a consequence the subsurface source samples for the forested regions have an overall higher 137Cs activity than equivalent samples collected from the agricultural areas.
The poor discrimination between surface and subsurface sources using 137Cs in this study highlights the importance of sampling design and protocols on characterizing the 137Cs activity of the sources. If topsoil/surface samples are collected in depositional areas near the stream edge (e.g. riparian zone) and are thought to be representative of material ‘likely to erode’ and contribute to the downstream suspended sediment, this sampling approach would exclude the upslope contributing area as part of the potential source of sediment. In many cases, this area will have a history of net soil loss and, if the upslope area is under an annual cropping rotation, this area may experience homogenization of the upper ~40 cm of soil due to tillage (e.g. Li et al. Citation2016). Both the net soil loss and/or homogenization of the soil will result in a much lower activity of FRNs when included as part of the source as compared to using samples exclusively from depositional and/or undisturbed areas. A similar issue may arise with colour, where the darker, more organic-rich topsoil is homogenized with the lighter subsoil in agricultural fields. Therefore, the overall characterization of FRN activity and other fingerprints in a topsoil/surface source is sensitive to the sampling approach.
A similar issue arises when sampling streambanks and other subsurface sources. The 137Cs profile of streambank samples in this study showed high activity in samples near the surface and low activity at depth. Three typical sampling approaches are used in characterizing subsurface sources of sediment in fingerprinting studies. The first approach is to aggregate samples from the top to the bottom of the profile into a single sample (e.g. Koiter, Lobb et al. Citation2013). In the second approach, surface samples are excluded altogether and material is taken from deeper in the bank profile only (e.g. Rahman and Bakri Citation2010). Finally, samples can be taken in a systematic manner down the entire profile and kept separate (e.g. Barthod et al. Citation2015). The first two approaches, aggregating and excluding surface samples, would generally result in either an overall lower 137Cs activity or lower variability as compared to the third approach (Smith and Dragovich Citation2008). Furthermore, the morphology of the bank (i.e. vertical or sloping banks) can impact the amount of FRNs that are received, and thereby label bank material, and the rate of erosion on sloping bank surfaces (i.e. rate of removal of high-activity bank material) may also be factors in the lack of 137Cs discrimination between surface and subsurface material.
The sampling approach taken in this study to characterize subsurface sources (i.e. the entire bank profile sampled at discrete depth intervals) resulted in surface samples taken in the lower slope and riparian areas to have similar 137Cs activity as compared to sources from the upper portion of the bank. Similarly, samples taken in agricultural fields, from mid to upper slope positions, have comparable 137Cs activity to samples collected lower down the bank profile. As result, the overall ability of 137Cs to discriminate between surface and subsurface sources in this study was poor.
Sediment source apportionment
Agricultural topsoil was determined to be the dominant source (77.2%) of suspended sediment, which is consistent with research that has identified the intensive agricultural activities on soils susceptible to erosion as being the main cause of soil degradation and sediment loading in the region (e.g. Saini and Grant Citation1980; Chow et al. Citation1999, Citation2011). A large amount of human and financial resources have been spent on implementing best management practices (BMPs) in the BBW to reduce soil erosion and sediment delivery. Specifically, flow diversion terraces have been implemented on > 50% of cultivated fields to reduce slope length, limit inter-rill and rill erosion, and promote in-field deposition of eroded material (Yang et al. Citation2009).
It was also determined that streambanks and other subsurface sources in agricultural areas were an important source (17.4%) of suspended sediment. However, the contribution of streambank and gully erosion to suspended sediment has received comparatively little attention in the BBW. Similar to the results found in BBW, many other fingerprinting studies have concluded that streambank sources were an important source of suspended sediment. For example, Koiter, Lobb et al. (Citation2013) found that in an agricultural watershed in south-central Manitoba, Canada, the dominant sources of sediment switched between topsoil in the headwaters and streambanks and shale bedrock sources at the outlet of the watershed. However, as agricultural topsoil contributes more than 3 times the amount of agricultural subsurface and forest sources combined, continued focus on reducing soil erosion and sediment delivery in agricultural fields within the BBW should remain a priority.
In the development, implementation and assessment of BMPs and other management tools, it is important to identify the sources of sediment. However, the relative contributions from potential sources of sediment need to be put into appropriate context by combining them with information on sediment loads. For example, a high relative contribution from a given source may not be problematic if the overall sediment yield is low. Therefore, integrating the results from sediment source fingerprinting with information on the suspended sediment load is an important step in assessing the sediment dynamics in the BBW (Walling et al. Citation2001; Smith et al. Citation2011). Extending the sediment apportionment result for agricultural topsoil (77.2%) to the entire BBW and combining it with information on sediment yield at the outlet (2633.2 Mg y−1; 2003–2007 average from Chow et al. (Citation2011)) and the areal extent of agricultural land use (65%; 9.4 km2), it is estimated that on average 215.7 Mg km−2 y−1 of agricultural topsoil is delivered to the stream network and exported from the BBW. Chow et al. (Citation1999) measured soil loss at two soil-quality monitoring benchmark sites (Agriculture and Agri-Food Canada sites 20-NB and 22-NB) located approximately 10 km southeast of the BBW. Using edge-of-field flumes, the two sites were used to measure the influence of both crop type (grain and potatoes) and land management practices (flow diversion terraces and up-and-down slope cultivation) on soil loss. It was found that under up-and-down slope cultivation the soil loss was on average 2076.0 and 38.7 Mg km−2 y−1 for potato and grain production, respectively. In contrast, the measured soil loss using flow diversion terraces and contour cultivation was on average an order of magnitude lower, at 100.1 and 8.5 Mg km−2 y−1 for potato and grain production, respectively. Therefore, both crop type and land management practice are important factors controlling the magnitude of the sediment being exported from the BBW. Furthermore, annual variations in crop type (i.e. crop rotation) and land management practice are also likely to contribute to the uncertainty in the fingerprinting apportionment results by increasing within-group variation.
Spatial and temporal framework
Fingerprinting studies typically follow the same sampling framework as was implemented in the BBW, where suspended sediments are collected at the main outlet of a watershed and used to draw conclusions for the entire catchment (e.g. Poleto et al. Citation2009; Mukundan et al. Citation2010; Collins, Zhang, Walling et al. Citation2012). Recently, the importance of carefully considering sediment sampling locations within watersheds has been recognized (e.g. Koiter, Lobb et al. Citation2013; Barthod et al. Citation2015). Sediment apportionment results based on suspended sediment collected at the watershed outlet may not reflect sediment dynamics elsewhere in the watershed. This is especially true for watersheds that are characterized by geomorphic disconnectivity, or composed of subwatersheds with different characteristics (e.g. topography, land use). Fingerprinting studies conducted at a range of spatial scales support this hypothesis, as some studies have found that the dominant sources of sediment can change between the headwaters and the outlet (e.g. Koiter, Lobb et al. Citation2013; Barthod et al. Citation2015), while others have found differences in sediment source apportionment along the length of a stream (e.g. Mckinley et al. Citation2013). However, a single sediment sampling location at the outlet of a watershed may be appropriate given the research objective or question. For example, if limiting the amount of sediment entering a reservoir is the research objective, sampling at the outlet of the watershed where the sediment load is the highest may be the most appropriate. This then raises the issue of how far upstream the results of sediment fingerprinting should be extended and how the apportionment results can be used to target management practices. This can be addressed, in part, by using a multi-scale sampling approach or additional lines of evidence (e.g. sediment budgets).
There is also temporal variation in the source apportionment results, as research has demonstrated that the sources of sediment can vary intra-storm, inter-storm and on a seasonal basis (e.g. Cooper et al. Citation2015). Assessing the temporal variability of the sources of sediment can be problematic as linking a particular source to in-stream sediment during a specific event can be difficult due to legacy sediment affects (i.e. remobilization of stored sediment). The current study did not include the snowmelt period (April–May) as access to sampling locations was difficult (i.e. deep snow) and potentially dangerous (i.e. high water levels). There are both geomorphological and hydrological differences between snowmelt and rainfall events. Snowmelt is less erosive as compared to rainfall due to lower kinetic energy and the presence of frozen soil, but the magnitude and the duration of the runoff event is much larger (Tiessen et al. Citation2010). For example, Tiessen et al. (Citation2010) demonstrated that snowmelt accounted for more than 80% of the total runoff and at least 50% of the total suspended sediment load exported from two small field-scale plots in the Canadian Prairies. In the BBW, approximately 40% of the total sediment load occurs during the spring snowmelt (month of April; unpublished data). Therefore, investigating the sedimentological response of snowmelt- versus rainfall-driven erosion and sediment transport events remains an important research objective in the BBW.
Conclusion
Results of the sediment fingerprinting study in the BBW, based on 7 years (2008–2014) of sediment sampling, demonstrated that the dominant source of sediment is from agricultural sources as compared to forest sources. In the BBW, during the snow- and ice-free period, the main source of sediment is agricultural topsoil (77.2%), followed by agricultural streambank and other subsurface sources (17.4%), and forest sources are only minor (3.2%). This research demonstrated that spectral-reflectance colour coefficients, in combination with 137Cs, were able to discriminate between sediment sources in the BBW. In contrast, particle morphology was not able to provide any discrimination between the potential sources of sediment. Sediment fingerprinting provides an effective method to identify sources of sediment; however, the apportionment results from suspended sediment collected at the watershed outlet may not be representative of sediment dynamics occurring elsewhere in the watershed. Furthermore, information on the relative contributions needs to be put into appropriate context by integrating it with data on sediment loads.
Funding
This work was supported by several synergy projects funded by Agriculture and Agri-Food Canada (AAFC), Environment and Climate Change Canada (ECCC) and the Natural Science and Engineering Research Council of Canada (NSERC). Major funding projects include, but are not limited to, AAFC A-base projects #1145: ‘Integrating selected BMPs to maximize environmental and economic benefits at the field and watershed scales for sustainable potato production in New Brunswick’, ECCC National Agri-Environmental Standards Initiative, and NSERC strategic project (STPGP 413426-2011): ‘Development of environmental fingerprinting techniques for sources of sediment and associated phosphorus within agricultural watersheds of Canada’.
Acknowledgements
Assistance with laboratory analyses and fieldwork was provided by Rick Allaby, Fangzhou Zheng, Zisheng Xing, Junyu Qi, Lionel Stevens, Sylvie Lavoie, John Monteith, Meagan Betts, Tegan Smith, Eva Slavicek and Brendon Brooks. Assistance with the translation of the abstract was provide by Mathieu Roy.
References
- Agriculture and Agri-Food Canada. 1998. The Canadian System of Soil Classification. Ottawa, Canada: NRC Research Press.
- Barthod, L., K. Liu, D. A. Lobb, P. N. Owens, N. Martínez-Carreras, A. J. Koiter, E. L. Petticrew, G. K. McCullough, C. Liu, and L. Gaspar. 2015. Selecting color-based tracers and classifying sediment sources in the assessment of sediment dynamics using sediment source fingerprinting. Journal of Environmental Quality 44 (5): 1605–1616.10.2134/jeq2015.01.0043
- Blake, W. H., P. J. Wallbrink, S. H. Doerr, R. A. Shakesby, and G. S. Humphreys. 2006. Magnetic enhancement in wildfire-affected soil and its potential for sediment-source ascription. Earth Surface Processes and Landforms 31 (2): 249–264.10.1002/(ISSN)1096-9837
- de Boer, D. H. 1997. Changing contributions of suspended sediment sources in small basins resulting from European settlement on the Canadian prairies. Earth Surface Processes and Landforms 22: 623–639.10.1002/(ISSN)1096-9837
- de Boer, D. H., and G. Crosby. 1995. Evaluating the potential of SEM/EDS analysis for fingerprinting suspended sediment derived from two contrasting topsoils. Catena 24 (4): 243–258.10.1016/0341-8162(95)00029-4
- Carrol, J. 2003. Geology of the Kedgwick, Gounamitz River, States Brook, and Menneval map areas (NTS 21 O/11, 21 O/12, 21 O/13 and 21 O/14), Restigouche County, New Brunswick. In Current Research 2002. New Brunswick Department of Natural Resources, 23–57.
- Chow, T. L., H. Corimier, J. Daigle, and I. Ghanem. 1990. Effects of potato cropping practices on water runoff and soil erosion. Canadian Journal of Soil Science 70 (2): 137–148.10.4141/cjss90-016
- Chow, T. L., H. W. Rees, and J. L. Daigle. 1999. Effectiveness of terraces/grassed waterway systems for soil and water conservation: A field evaluation. Journal of Soil and Water Conservation 54 (3): 577–583.
- Chow, T. L., H. W. Rees, and J. Monteith. 2000. Seasonal distribution of runoff and soil loss under four tillage treatments in the upper St. John River Valley New Brunswick, Canada. Canadian Journal of Soil Science 80 (4): 649–660.10.4141/S00-006
- Chow, L., Z. Xing, G. Benoy, H. W. Rees, F. Meng, Y. Jiang, and J. L. Daigle. 2011. Hydrology and water quality across gradients of agricultural intensity in the Little River watershed area, New Brunswick, Canada. Journal of Soil and Water Conservation 66 (1): 71–84.10.2489/jswc.66.1.71
- Collins, A. L., and D. E. Walling. 2002. Selecting fingerprint properties for discriminating potential suspended sediment sources in river basins. Journal of Hydrology 261 (1-4): 218–244.10.1016/S0022-1694(02)00011-2
- Collins, A. L., D. E. Walling, and G. J. L. Leeks. 1997. Source type ascription for fluvial suspended sediment based on a quantitative composite fingerprinting technique. Catena 29 (1): 1–27.10.1016/S0341-8162(96)00064-1
- Collins, A. L., Y. Zhang, D. McChesney, D. E. Walling, S. M. Haley, and P. Smith. 2012. Sediment source tracing in a lowland agricultural catchment in southern England using a modified procedure combining statistical analysis and numerical modelling. Science of The Total Environment 414: 301–317.10.1016/j.scitotenv.2011.10.062
- Collins, A. L., Y. Zhang, D. E. Walling, S. E. Grenfell, P. Smith, J. Grischeff, A. Locke, A. Sweetapple, and D. Brogden. 2012. Quantifying fine-grained sediment sources in the River Axe catchment, southwest England: Application of a Monte Carlo numerical modelling framework incorporating local and genetic algorithm optimisation. Hydrological Processes 26 (13): 1962–1983.10.1002/hyp.v26.13
- Collins, A. L., I. D. L. Foster, A. C. Gellis, P. Porto, and A. J. Horowitz. 2017. Sediment source fingerprinting for informing catchment management: Methodological approaches, problems and uncertainty. Journal of Environmental Management 194: 1–3.10.1016/j.jenvman.2017.03.026
- Cooper, R. J., T. Krueger, K. M. Hiscock, and B. G. Rawlins. 2015. High-temporal resolution fluvial sediment source fingerprinting with uncertainty: A Bayesian approach. Earth Surface Processes and Landforms 40 (1): 78–92.10.1002/esp.3621
- Davis, C. M., and J. F. Fox. 2009. Sediment fingerprinting: Review of the method and future improvements for allocating nonpoint source pollution. Journal of Environmental Engineering 135 (7): 490–504.10.1061/(ASCE)0733-9372(2009)135:7(490)
- DesRoches, A., S. Danielescu, and K. Butler. 2014. Structural controls on groundwater flow in a fractured bedrock aquifer underlying an agricultural region of northwestern New Brunswick. Canada. Hydrogeology Journal 22 (5): 1067–1086.10.1007/s10040-014-1134-0
- Dutton, C., S. C. Anisfeld, and H. Ernstberger. 2013. A novel sediment fingerprinting method using filtration: Application to the Mara River, East Africa. Journal of Soils and Sediments 13 (10): 1708–1723.10.1007/s11368-013-0725-z
- Environment and Climate Change Canada. 2017. Weather Office. http://www.weatheroffice.gc.ca (accessed April 4, 2017).
- Foster, I. D. L., and J. A. Lees. 2000. Tracers in geomorphology: Theory and applications in tracing fine particulate sediments. In Tracers in Geomorphology, ed. I.D.L. Foster, 3–20. Chichester, UK: Wiley.
- Gellis, A. C., C. C. Fuller, and P. C. Van Metre. 2017. Sources and ages of fine-grained sediment to streams using fallout radionuclides in the Midwestern United States. Journal of Environmental Management 194 (1): 73–85.10.1016/j.jenvman.2016.06.018
- Grimshaw, D. L., and J. Lewin. 1980. Source identification for suspended sediments. Journal of Hydrology 47 (1-2): 151–162.10.1016/0022-1694(80)90053-0
- Gruszowski, K. E., I. D. L. Foster, J. A. Lees, and S. M. Charlesworth. 2003. Sediment sources and transport pathways in a rural catchment, Herefordshire. UK. Hydrological Processes 17 (13): 2665–2681.10.1002/(ISSN)1099-1085
- Guzman, G., J. N. Quinton, M. A. Nearing, L. Mabit, and J. A. Gomez. 2013. Sediment tracers in water erosion studies: Current approaches and challenges. Journal of Soils and Sediments 13 (4): 816–833.10.1007/s11368-013-0659-5
- Haddadchi, A., D. S. Ryder, O. Evrard, and J. Olley. 2013. Sediment fingerprinting in fluvial systems: Review of tracers, sediment sources and mixing models. International Journal of Sediment Research 28 (4): 560–578.10.1016/S1001-6279(14)60013-5
- He, Q., and D. E. Walling. 1996. Interpreting particle size effects in the adsorption of 137Cs and unsupported 210Pb by mineral soils and sediments. Journal of Environmental Radioactivity 30: 117–137.10.1016/0265-931X(96)89275-7
- Horowitz, A. J. 1991. A primer on sediment-trace element chemistry. Chelsea, Michigan, USA: Lewis.
- Islam, K., B. Singh, and A. McBratney. 2003. Simultaneous estimation of several soil properties by ultra-violet, visible, and near-infrared reflectance spectroscopy. Soil Research 41 (6): 1101–1114.10.1071/SR02137
- King, D. J., J. M. Cossette, R. G. Eilers, B. A. Grant, D. A. Lobb, G. A. Padbury, H. W. Rees, I. J. Shelton, J. Tajek, G. J. Wall, and L. J. P. van Vliet. 2000. Risk of tillage erosion. In Environmental Health of Canadian Agroecosystems, eds. T. McRae, S. Smith, and L. J. Gregorich, 77–83. Ottawa, Canada: Agriculture and Agri-Food Canada.
- Klages, M. G., and Y. P. Hsieh. 1975. Suspended solids carried by the Galatin River of Southwestern Montana. II. Using mineralogy for inferring sources. Journal of Environmental Quality 4: 68–73.10.2134/jeq1975.00472425000400010016x
- Koiter, A. J., P. N. Owens, E. L. Petticrew, and D. A. Lobb. 2013. The behavioural characteristics of sediment properties and their implications for sediment fingerprinting as an approach for identifying sediment sources in river basins. Earth-Science Reviews 125: 24–42.10.1016/j.earscirev.2013.05.009
- Koiter, A. J., D. A. Lobb, P. N. Owens, E. L. Petticrew, K. H. Tiessen, and S. Li. 2013. Investigating the role of connectivity and scale in assessing the sources of sediment in an agricultural watershed in the Canadian prairies using sediment source fingerprinting. Journal of Soils and Sediments 13 (10): 1676–1691.10.1007/s11368-013-0762-7
- Konen, M. E., C. L. Burras, and J. A. Sandor. 2003. Organic carbon, texture, and quantitative color measurement relationships for cultivated soils in north central Iowa. Soil Science Society of America Journal 67 (6): 1823.10.2136/sssaj2003.1823
- Krein, A., E. L. Petticrew, and T. Udelhoven. 2003. The use of fine sediment fractal dimensions and colour to determine sediment sources in a small watershed. Catena 53 (2): 165–179.10.1016/S0341-8162(03)00021-3
- Krishnappan, B. G., P. A. Chambers, G. Benoy, and J. Culp. 2009. Sediment source identification: A review and a case study in some Canadian streams. Canadian Journal of Civil Engineering 36: 1622–1633.10.1139/L09-110
- Kroetsch, D., and C. Cang. 2007. Particle size distribution. In Soil Sampling and Methods of Analysis, eds. M.R. Carter and E.G. Gregorich, 713–727. Boca Raton, FL, USA: CRC Press.
- Laceby, J. P., O. Evrard, H. G. Smith, W. H. Blake, J. M. Olley, J. P. G. Minella, and P. N. Owens. 2017. The challenges and opportunities of addressing particle size effects in sediment source fingerprinting: A review. Earth-Science Reviews 169: 85–103.10.1016/j.earscirev.2017.04.009
- Li, M., W. Yao, J. Yang, Z. Shen, and E. Yang. 2016. Distribution characteristics of 137Cs in soil profiles under different land uses and its implication. Journal of Radioanalytical and Nuclear Chemistry 308 (1): 173–178.10.1007/s10967-015-4319-7
- Marshall, I. B., Schut, P. H. and Ballard, M. 1999. A national ecological framework for Canada: Attribute data. Ottawa, Canada: Agriculture and Agri-Food Canada, Research Branch, Centre for Land and Biological Resources Research, and Environment Canada, State of the Environment Directorate, Ecozone Analysis Branch.
- Martínez-Carreras, N., A. Krein, F. Gallart, J. F. Iffly, L. Pfister, L. Hoffmann, and P. N. Owens. 2010. Assessment of different colour parameters for discriminating potential suspended sediment sources and provenance: A multi-scale study in Luxembourg. Geomorphology 118 (1-2): 118–129.10.1016/j.geomorph.2009.12.013
- McConnachie, J. L., and E. L. Petticrew. 2006. Tracing organic matter sources in riverine suspended sediment: Implications for fine sediment transfers. Geomorphology 79 (1-2): 13–26.10.1016/j.geomorph.2005.09.011
- Mckinley, R., D. Radcliffe, and R. Mukundan. 2013. A streamlined approach for sediment source fingerprinting in a Southern Piedmont watershed, USA. Journal of Soils and Sediments 13 (10): 1754–1769.10.1007/s11368-013-0723-1
- Mellerowicz, K., H. W. Rees, T. L. Chow, and I. Ghanem. 1993. Soils of the Black Brook Watershed St. Andre Parish Madawaska County New Brunswick. New Brunswick: Department of Agriculture and Agriculture Canada.
- Moore, J. W., and B. X. Semmens. 2008. Incorporating uncertainty and prior information into stable isotope mixing models. Ecology Letters 11 (5): 470–480.10.1111/j.1461-0248.2008.01163.x
- Motha, J. A., P. J. Wallbrink, P. B. Hairsine, and R. B. Grayson. 2002. Tracer properties of eroded sediment and source material. Hydrological Processes 16: 1983–2000.10.1002/(ISSN)1099-1085
- Motha, J. A., P. J. Wallbrink, P. B. Hairsine, and R. B. Grayson. 2003. Determining the sources of suspended sediment in a forested catchment in southeastern Australia. Water Resources Research 39 (3): 1056.
- Mukundan, R., D. E. Radcliffe, J. C. Ritchie, L. M. Risse, and R. A. McKinley. 2010. Sediment fingerprinting to determine the source of suspended sediment in a southern piedmont stream. Journal of Environment Quality 39 (4): 1328.10.2134/jeq2009.0405
- Nosrati, K., G. Govers, B. X. Semmens, and E. J. Ward. 2014. A mixing model to incorporate uncertainty in sediment fingerprinting. Geoderma 217-218: 173–180.10.1016/j.geoderma.2013.12.002
- Olley, J. M., A. S. Murray, D. H. Mackenzie, and K. Edwards. 1993. Identifying sediment sources in a gullied catchment using natural and anthropogenic radioactivity. Water Resources Research 29 (4): 1037–1043.10.1029/92WR02710
- Owens, P. N., D. E. Walling, and Q. He. 1996. The behaviour of bomb-derived caesium-137 fallout in catchment soils. Journal of Environmental Radioactivity 32: 169–191.10.1016/0265-931X(96)84941-1
- Owens, P. N., W. H. Blake, L. Gaspar, D. Gateuille, A. J. Koiter, D. A. Lobb, E. L. Petticrew, D. Raiffarth, H. G. Smith, and J. C. Woodward. 2016. Fingerprinting and tracing the sources of soils and sediments: Earth and ocean science, geoarchaeological, forensic, and human health applications. Earth-Science Reviews 162: 1–23.10.1016/j.earscirev.2016.08.012
- Palmer, M. J., and G. B. Douglas. 2008. A Bayesian statistical model for end member analysis of sediment geochemistry, incorporating spatial dependences. Journal of the Royal Statistical Society: Series C (Applied Statistics) 57: 313–327.10.1111/j.1467-9876.2007.00615.x
- Parnell, A. C., R. Inger, S. Bearhop, and A. L. Jackson. 2010. Source partitioning using stable isotopes: Coping with too much variation. PLoS ONE 5 (3): e9672.10.1371/journal.pone.0009672
- Peart, M. R., and D. E. Walling. 1986. Fingerprinting sediment source: The example of a drainage basin in Devon, UK. In Drainage Basin sediment delivery, Vol. 159, 41–55. Wallingford, UK: IAHS Publication.
- Perkins, R. W., and C. W. Thomas. 1980. Worldwide fallout. In Transuranic Elements in the Environment, ed. W. C. Hanson, 53–82. Washington, DC, USA: US Department of the Environment.
- Phillips, J. M., M. A. Russell, and D. E. Walling. 2000. Time-integrated sampling of fluvial suspended sediment: A simple methodology for small catchments. Hydrological Processes 14 (14): 2589–2602.10.1002/(ISSN)1099-1085
- Phillips, D. L., R. Inger, S. Bearhop, A. L. Jackson, J. W. Moore, A. C. Parnell, B. X. Semmens, and E. J. Ward. 2014. Best practices for use of stable isotope mixing models in food-web studies. Canadian Journal of Zoology 92 (10): 823–835.10.1139/cjz-2014-0127
- Poleto, C., G. H. Merten, and J. P. Minella. 2009. The identification of sediment sources in a small urban watershed in southern Brazil: An application of sediment fingerprinting. Environmental Technology 30 (11): 1145–1153.10.1080/09593330903112154
- Pulley, S., I. D. L. Foster, and P. Antunes. 2015. The uncertainties associated with sediment fingerprinting suspended and recently deposited fluvial sediment in the Nene river basin. Geomorphology 228: 303–319.10.1016/j.geomorph.2014.09.016
- Pulley, S., I. Foster, and A. L. Collins. 2017. The impact of catchment source group classification on the accuracy of sediment fingerprinting outputs. Journal of Environmental Management 194: 16–26.10.1016/j.jenvman.2016.04.048
- R Core Team. 2016. R: A language and environment for statistical computing. R Foundation for Statistical Computing. http://www.R-project.org.
- Rahman, A. K. M., and D. A. Bakri. 2010. Contribution of diffuse sources to the sediment and phosphorus budgets in Ben Chifley Catchment, Australia. Environmental Earth Sciences 60 (3): 463–472.10.1007/s12665-009-0187-1
- Rappol, M., and Russell, H. 1989. Glacial dispersal of Precambrian Shield and local Appalachian rocks in the lower St. Lawrence region in western Gaspésie, Quebec, and in adjacent New Brunswick. In Current Research, Part B, Geological Survey of Canada, Paper 89-1B: 127–136.
- Reiffarth, D. G., E. L. Petticrew, P. N. Owens, and D. A. Lobb. 2016. Sources of variability in fatty acid (FA) biomarkers in the application of compound-specific stable isotopes (CSSIs) to soil and sediment fingerprinting and tracing: A review. Science of The Total Environment 565: 8–27.10.1016/j.scitotenv.2016.04.137
- Rhoton, F. E., W. E. Emmerich, D. A. DiCarlo, D. S. McChesney, M. A. Nearing, and J. C. Ritchie. 2008. Identification of suspended sediment sources using soil characteristics in a semiarid watershed. Soil Science Society of America Journal 72 (4): 1102.10.2136/sssaj2007.0066
- Ritchie, J. C., and J. R. McHenry. 1990. Application of radioactive fallout cesium-137 for measuring soil erosion and sediment accumulation rates and patterns: A review. Journal of Environmental Quality 19: 215–233.10.2134/jeq1990.00472425001900020006x
- RStudio. 2016. RStudio: Integrated development environment for R. http://www.rstudio.org/
- Russell, M. A., D. E. Walling, and R. A. Hodgkinson. 2001. Suspended sediment sources in two small lowland agricultural catchments in the UK. Journal of Hydrology 252: 1–24.10.1016/S0022-1694(01)00388-2
- Saini, G. R., and W. J. Grant. 1980. Long-term effects of intensive cultivation on soil quality in the potato-growing areas of New Brunswick (Canada) and Maine (USA). Canadian Journal of Soil Science 60 (3): 421–428.10.4141/cjss80-047
- Santamarina, J. C., and G. C. Cho. 2004. Soil behaviour: The role of particle shape. Advances in Geotechnical Engineering 1: 604–617.
- Schafer, B. M. 2008. Particle Shape. In Encyclopedia of Soil Science, ed. W. Chesworth, 1249–1250. Dordrecht, Netherlands: Springer.
- Sherriff, S. C., S. W. Franks, J. S. Rowan, O. Fenton, and Ó’hUllancháin, D. 2015. Uncertainty-based assessment of tracer selection, tracer non-conservativeness and multiple solutions in sediment fingerprinting using synthetic and field data. Journal of Soils and Sediments 15 (10): 2101–2116.10.1007/s11368-015-1123-5
- Shields, A. 1936. Anwendung der Aehnlichkeitsmechanik und der urbulenzforschung auf die eschiebebewegung. Berlin, Germany: Preussischen Versuchsanstalt für Wasserbau.
- Smith, H. G., and W. H. Blake. 2014. Sediment fingerprinting in agricultural catchments: A critical re-examination of source discrimination and data corrections. Geomorphology 204: 177–191.10.1016/j.geomorph.2013.08.003
- Smith, H. G., and D. Dragovich. 2008. Improving precision in sediment source and erosion process distinction in an upland catchment, south-eastern Australia. Catena 72 (1): 191–203.10.1016/j.catena.2007.05.013
- Smith, H. G., G. J. Sheridan, P. N. J. Lane, P. Noske, and H. Heijnis. 2011. Changes to sediment sources following wildfire in a forested upland catchment, southeastern Australia. Hydrological Processes 25: 2878–2889.10.1002/hyp.v25.18
- St. Peter, C.. 1978. Geology of parts of Restigouche, Victoria and Madawaska counties, northwestern New Brunswick. Mineral Resources Branch, New Brunswick Department of Natural Resources.
- Stock, B. C., and Semmens, B. X. 2016a. MixSIAR GUI user manual.
- Stock, B. C., and B. X. Semmens. 2016b. Unifying error structures in commonly used biotracer mixing models. Ecology 97 (10): 2562–2569.10.1002/ecy.1517
- Stringer, P., and Pickerill, R. K. 1980. Structure and sedimentology of the Siluro-Devonian between Edmundston and Grand Falls, New Brunswick. In The Geology of northeastern Maine and neighboring New Brunswick, eds. D. C. Roy and N. S. Naylor, 262–277. New England Intercollegiate Geological Conference.
- Su, J. J., E. van Bochove, G. Thériault, B. Novotna, J. Khaldoune, J. T. Denault, J. Zhou, et al. 2011. Effects of snowmelt on phosphorus and sediment losses from agricultural watersheds in Eastern Canada. Agricultural Water Management 98 (5): 867–876.10.1016/j.agwat.2010.12.013
- Sutherland, A. B., J. M. Culp, and G. A. Benoy. 2012. Evaluation of deposited sediment and macroinvertebrate metrics used to quantify biological response to excessive sedimentation in agricultural streams. Environmental Management 50 (1): 50–63.10.1007/s00267-012-9854-1
- Tiessen, K. H. D., J. A. Elliott, J. Yarotski, D. A. Lobb, D. N. Flaten, and N. E. Glozier. 2010. Conventional and conservation tillage: Influence on seasonal runoff, sediment, and nutrient losses in the Canadian prairies. Journal of Environmental Quality 39 (3): 964–980.10.2134/jeq2009.0219
- Torrent, J., and V. Barrón. 1993. Laboratory measurement of soil color: Theory and practice. In Soil Color, eds. J.M. Bigham and E.I. Ciolkosz, 21–33. MadisonWI.
- Udelhoven, T., C. Emmerling, and T. Jarmer. 2003. Quantitative analysis of soil chemical properties with diffuse reflectance spectrometry and partial least-square regression: A feasibility study. Plant and Soil 251 (2): 319–329.10.1023/A:1023008322682
- Venables, S., and B. D. Ripley. 2002. Modern Applied Statistics with S. New York: Springer.10.1007/978-0-387-21706-2
- Viscarra Rossel, R. A., D. J. J. Walvoort, A. B. McBratney, L. J. Janik, and J. O. Skjemstad. 2006. Visible, near infrared, mid infrared or combined diffuse reflectance spectroscopy for simultaneous assessment of various soil properties. Geoderma 131 (1-2): 59–75.10.1016/j.geoderma.2005.03.007
- Viscarra Rossel, R. A., S. R. Cattle, A. Ortega, and Y. Fouad. 2009. In situ measurements of soil colour, mineral composition and clay content by vis–NIR spectroscopy. Geoderma 150 (3-4): 253–266.10.1016/j.geoderma.2009.01.025
- Wall, G. J., and L. P. Wilding. 1976. Mineralogy and related parameters of fluvial suspended sediments in northwestern Ohio. Journal of Environmental Quality 5: 168–173.10.2134/jeq1976.00472425000500020012x
- Wallbrink, P. J., A. S. Murray, J. M. Olley, and L. J. Olive. 1998. Determining sources and transit times of suspended sediment in the Murrumbidgee River, New South Wales, Australia, using fallout 137Cs and 210Pb. Water Resources Research 34 (4): 879–887.10.1029/97WR03471
- Walling, D. E. 2005. Tracing suspended sediment sources in catchments and river systems. Science of the Total Environment 344 (1-3): 159–184.10.1016/j.scitotenv.2005.02.011
- Walling, D. E., and Woodward, J. C. 1992. Use of radiometric fingerprints to derive information on suspended sediment sources. In Erosion and sediment transport monitoring programmes in river basins, 153–164. Wallingford, UK: IAHS Publication 210, IAHS Press.
- Walling, D. E., M. R. Peart, F. Oldfield, and R. Thompson. 1979. Suspended sediment sources identified by magnetic measurements. Nature 281: 110–113.10.1038/281110a0
- Walling, D. E., P. N. Owens, and G. J. L. Leeks. 1999. Fingerprinting suspended sediment sources in the catchment of the River Ouse, Yorkshire, UK. Hydrological Processes 13: 955–975.10.1002/(ISSN)1099-1085
- Walling, D. E., A. L. Collins, H. M. Sichingabula, and G. J. L. Leeks. 2001. Integrated assessment of catchment suspended sediment budgets: A Zambian example. Land Degradation and Development 12: 387–415.10.1002/(ISSN)1099-145X
- Weihs, C., U. Ligges, K. Luebke, and N. Raabe. 2005. klaR Analyzing German Business Cycles. In Data Analysis and Decision Support, eds. D. Baier, R. Decker, and L. Schmidt-Thieme, 335–343. Berlin, Germany: Springer.10.1007/3-540-28397-8
- Wickham, H. 2009. Ggplot2: Elegant Graphics for Data Analysis. New York: Springer.10.1007/978-0-387-98141-3
- Wolman, M.G.. 1977. Changing needs and opportunities in the sediment field. Water Resources Research 13 (1): 50–54.
- Yang, Q., F.-R. Meng, Z. Zhao, T. L. Chow, G. Benoy, H. W. Rees, and C. P.-A. Bourque. 2009. Assessing the impacts of flow diversion terraces on stream water and sediment yields at a watershed level using SWAT model. Agriculture, Ecosystems & Environment 132 (1–2): 23–31.