Abstract
The estimation of extreme precipitation events is a topic of growing interest and concern, particularly in highly urbanized areas. The Fifth Assessment Report by the Intergovernmental Panel on Climate Change (IPCC) reported that land-surface air temperatures have increased and that more extreme precipitation events are expected in a warmer climate. Hydrometeorological data often display nonstationary characteristics in a changing climate, prompting numerous studies worldwide. This research proposes a peaks-over-threshold (POT) approach using both stationary and covariate-dependent nonstationary thresholds (bivariate and multivariate models). The generalized Pareto (GP) distribution is fit to threshold exceedance data, which accounts for potential nonstationarity in the variability of the extreme events. The analysis herein focuses on coastal British Columbia (BC), which includes the Greater Vancouver Regional District (Metro Vancouver), due to the potential impacts of climate change in the region. Results from quantile estimates and an uncertainty analysis indicate that in the winter and summer months, there is stronger evidence of stationarity in 50-year quantiles. A trend analysis is also performed on the magnitude and frequency of POT events for winter and summer for two time periods (1976–2014 and 1986–2014). Results of this analysis indicate that globally significant trends are found in the threshold exceedance and frequency of peak events data.
L’estimation des événements de précipitations extrêmes est un sujet d'intérêt et de préoccupation croissant, en particulier dans les zones fortement urbanisées. Le cinquième rapport d’évaluation du Groupe d’experts intergouvernemental sur l’évolution du climat (GIEC) a signalé que les températures de l’air à la surface du sol ont augmenté et que des précipitations plus extrêmes sont attendues dans un climat plus chaud. Les données hydrométéorologiques présentent souvent des caractéristiques non stationnaires dans un climat changeant, ce qui a donné lieu à de nombreuses études dans le monde entier. Cette recherche propose une approche de point plus de seuil en utilisant à la fois des seuils non stationnaires et dépendants des covariables (modèles bivariés et multivariés). La distribution de Pareto généralisée est adaptée aux données de dépassement de seuil, ce qui explique la non-stationnarité potentielle de la variabilité des événements extrêmes. L’analyse présentée ici porte sur la côte de la Colombie-Britannique, qui comprend le district régional du métro Vancouver, en raison des impacts potentiels du changement climatique dans la région. Les résultats des estimations quantiles et une analyse de l’incertitude indiquent que pendant les mois d’hiver et d’été, il y a des preuves plus fortes de la stationnarité dans les quantiles de 50 ans. Une analyse des tendances est également effectuée sur l’ampleur et la fréquence des événements POT pour l’hiver et l’été pour deux périodes (1976-2014 et 1986-2014). Les résultats de cette analyse indiquent que des tendances globalement significatives sont trouvées dans le dépassement du seuil et la fréquence des données sur les événements de pointe.
Introduction
Intensifications of the magnitude and/or frequency of severe precipitation events could result in more extensive and frequent flooding and can have far-reaching socioeconomic consequences in highly populated or urbanized areas. For example, the widespread 2013 Alberta, Canada, floods affected approximately 100,000 residents, resulted in four deaths, and caused US$5.7 million in damages (EM-DAT Citation2016). The Fifth Assessment Report by the Intergovernmental Panel on Climate Change (IPCC) states that global land-surface air temperatures have increased and that increases in extreme precipitation are consistent with a warmer climate (Hartmann et al. Citation2013). Hartmann et al. (Citation2013) also note that there is convincing evidence of increases in the frequency and intensity of heavy precipitation in North America. Therefore, the potential for nonstationarity in the magnitude and frequency of extreme precipitation events is of paramount concern. Additionally, with the assertion by Milly et al. (Citation2008) that ‘stationarity is dead’, the time-independence of the statistics of historical rainfall records is brought into question.
Hydrometeorological quantile estimates for low-frequency, high-magnitude events are essential for civil infrastructure design. For instance, several Canadian provinces have imposed guidelines requiring that stormwater infrastructure adequately contain/convey runoff from a 100-year rainfall event (Stephens et al. Citation2002; Ontario Ministry of the Environment [OME] Citation2008). Standard statistical techniques assume data to be independently and identically distributed (IID), meaning the data arise from the same statistical probability distribution (Katz et al. Citation2002). This assumption is not valid in the presence of nonstationarity, thus establishing a need for covariate-dependent approaches in a changing climate. Estimating hydrometeorological extremes in a changing climate has henceforth become a topic which has gained a great deal of interest (Kharin and Zwiers Citation2005; Nadarajah Citation2005; El Adlouni et al. Citation2007; Hundecha et al. Citation2008; Sugahara et al. Citation2009; Rajagopalan Citation2010; Villarini et al. Citation2010; Beguería et al. Citation2011; Burn et al. Citation2011; Ouarda and El-Adlouni Citation2011; Villarini et al. Citation2011; Gilroy and McCuen Citation2012; Roth et al. Citation2012; Tramblay et al. Citation2013; Li and Tan Citation2015).
The extremes within hydrological data can be characterized in a variety of manners. Two commonly used techniques include the block maxima series and the partial duration series, or peaks over threshold (POT). The block maxima series is comprised of the largest peak value of a data set in a predetermined block length, typically selected to be 1 year and resulting in the annual maximum series (Katz et al. Citation2002). This research adopts the POT technique, in which all values that fall above a suitably high threshold are retained for analysis (Coles Citation2001). In addition to the peak annual data, this method facilitates the inclusion of supplementary extreme events in wet years. The timing of exceedances of a sufficiently high threshold are frequently modelled using a homogeneous Poisson process, while excesses over the threshold are commonly modelled using the generalized Pareto (GP) distribution, typically referred to as the Poisson–GP model (Khaliq et al. Citation2006). The GP distribution parameters may, however, be characterized by temporal covariates (Renard et al. Citation2006; Sugahara et al. Citation2009; Kyselý et al. Citation2010; Beguería et al. Citation2011; Roth et al. Citation2012; Tramblay et al. Citation2013; Sungwook et al. Citation2016) including the use of a nonhomogeneous Poisson process with the inclusion of a covariate-dependent intensity parameter (Katz Citation2010; Beguería et al. Citation2011). A number of studies have focused on modelling nonstationarity in the selected threshold (Kyselý et al. Citation2010; Northrop and Jonathan Citation2011; Roth et al. Citation2012; Jonathan, Ewans, et al. Citation2013; Jonathan, Randell, et al. Citation2013, Citation2014; Roth et al. Citation2014), but to the authors’ knowledge, none has developed a goodness-of-fit technique for selecting the most appropriate stationary or nonstationary threshold/GP model combination. Furthermore, this study addresses the confounding issue of time dependence and large-scale ocean–atmosphere phenomena in nonstationary threshold selection.
The purpose of this study is to assess nonstationarity in both threshold models and GP distribution parameters to determine those models that provide superior goodness of fit and less uncertainty for the purpose of quantile estimation. Through this POT analysis a combination of bivariate and multivariate (linear and quadratic) covariate-dependent threshold models are evaluated. This research focuses on coastal British Columbia (BC), Canada. Extreme precipitation is associated with numerous hazards in the area, including flooding and landslides (Walker et al. Citation2008). With approximately 75% of BC’s population living in coastal BC, primarily in Metro Vancouver (Walker et al. Citation2008), research of this nature is required to determine the magnitude and potential changes in extreme precipitation events that may be expected in one of Canada’s most populated regions.
The Rocky Mountains act as a barrier to arctic air masses, giving rise to mild seasonal temperatures in coastal BC (Hare and Thomas Citation1979). Although the area is one of the highest seismic activity zones in Canada (Chang et al. Citation2012), extreme weather events are the foremost hazard, affecting British Columbians more than any other climate hazard (Walker et al. Citation2008; Moore et al. Citation2010). The climate in southern BC is influenced by the El Niño Southern Oscillation (ENSO) and the Pacific Decadal Oscillation (PDO), both of which are large-scale ocean–atmosphere phenomena (Walker et al. Citation2008). Several indices are used to describe ENSO; the Niño 3.4 index is used herein. The Niño 3.4 index is a measure of the area average sea surface temperature (SST) from 5°S–5°N and 170°E–120°W (Rayner et al. Citation2003). The PDO Index is the leading principal component of the North Pacific monthly sea surface variability (poleward of 20°N; Mantua et al. Citation1997).
Methodology
A POT approach is developed for the analysis of hydrometeorological data using stationary and nonstationary thresholds in which the resulting threshold exceedance data are modelled using the GP distribution. Trend detection is carried out on stationary threshold exceedance data using the Mann–Kendall nonparametric trend test (Mann Citation1945; Kendall Citation1975) on total daily precipitation and rainfall frequency.
Climate oscillation selection
Due to the potential influence of both ENSO and PDO on coastal BC’s climate, the correlation between both of these climate indices and the precipitation data is assessed (Gershunov and Barnett Citation1998; Walker et al. Citation2008). The Niño 3.4 Index is obtained from the National Oceanic & Atmospheric Administration – Earth System Research Library (NOAA-ESRL) Physical Sciences Division (https://www.esrl.noaa.gov/psd/gcos_wgsp/Timeseries/Data/nino34.long.anom.data), while the PDO Index is retrieved from NOAA National Climate Data Center (http://www.ncdc.noaa.gov/teleconnections/pdo/). An analysis of the correlation between total monthly precipitation at each observation station and the Niño 3.4 and PDO indices is carried out through a cross-correlation analysis.
Generalized Pareto distribution model
The most prominent benefit of the POT approach over the block maxima approach is that more data are retained for analysis, as opposed to using only one peak value in a given block length. The Balkema–de Haan–Pickands theorem states that for a series of IID data, excesses over a sufficiently large threshold can be approximated using the GP distribution (Balkema and de Hann Citation1974; Pickands Citation1975). Also, in the POT framework it can be demonstrated that the occurrence of exceedances over a high threshold (u) follows a Poisson distribution with rate parameter .
For a sequence of IID random variables , where xi > u, the cumulative distribution function,
, of xi - u for a suitably large
is given by (Coles Citation2001):
(1)
where σ and κ are the scale and shape parameters, respectively. In the event that κ = 0, the distribution reduces to the exponential distribution, which is not employed in this analysis. The quantile function, Qp of the GP distribution is required to calculate the T-year event, which is an event exceeded, on average, once every T = 1/(1 − p), where 0 < p < 1. The quantile function is commonly expressed in terms of the scale and shape parameters, rate parameter and T-year event (Coles Citation2001):(2)
In the event that data are nonstationary and therefore not IID, the GP distribution can be expressed in terms of covariates. If distributional parameters are, for example, a function of time, the cumulative distribution function of the GP distribution may be expressed as (Coles Citation2001):(3)
where are the time-dependent data;
and
are the time-varying scale and shape parameters, respectively, and are expressed most generally by (O’Brien and Burn Citation2014):
(4)
(5)
when m = 1, Equation (4) reduces to a linear model, whereas if m = 2, Equation (4) assumes a quadratic form. Evidence of a constant shape parameter has been found in various hydrometeorological studies and this assumption is used hereafter (Vinnikov and Robock Citation2002; Kharin and Zwiers Citation2005; Kyselý et al. Citation2010; Kay and Jones Citation2012).
If nonstationarity is present in the mean of the original data, the use of a stationary threshold will result in a nonhomogeneous Poisson process for the excesses. This inhomogeneity can be modelled using a nonstationary rate parameter (Katz Citation2010; Beguería et al. Citation2011); however, the use of a nonstationary threshold will result in a homogeneous Poisson process. The latter approach is used herein to model potential changes in the central tendency of the data.
Threshold selection
The distribution of exceedances over a threshold asymptotically approaches the GP distribution, given that a suitably high threshold is chosen. Therefore, threshold selection must be done with care to avoid undue bias or, in the case of too high a threshold, high variance (Coles Citation2001). To ascertain whether change-points exist in the data, Pettitt’s test (Pettitt Citation1979) is applied prior to both stationary and nonstationary threshold selection. The following sections discuss the stationary and nonstationary threshold selection criteria that are applied for this research.
Stationary threshold
Stationary thresholds are selected through the use of several exploratory plots (Lang et al. Citation1999; Mailhot et al. Citation2013; Osman et al. Citation2013; Roth et al. Citation2016; Sungwook et al. Citation2016). Assessing the validity of the homogeneous Poisson process assumption is carried out through the dispersion index plot (Cunnane Citation1979), where an optimally selected threshold has a dispersion index equal to 1 (Cunnane Citation1979; Lang et al. Citation1999). The mean residual life plot depicts the mean excess above a given threshold versus a range of threshold values. The GP distribution provides a suitable fit to excesses over the selected threshold when linearity is apparent in the mean residual life plot. Complimentary threshold choice (TC) plots involve fitting the GP distribution to a range of threshold exceedances and assessing the stability of the resulting distributional parameters. These include the scale and shape parameters for the GP distribution (Coles Citation2001). An overview of the threshold selection process is provided in Figure for the Mission West Abbey summer season data. Figure (a) demonstrates that an appropriate threshold range falls somewhere between 14 and 17 mm. A final threshold of 17 mm was selected as it provides slightly more linearity in Figure (b), which is the mean residual life plot. The modified scale and shape parameters are also suitably stable after this point, and are depicted in Figure (c) and (d), respectively.
Nonstationary threshold
Quantile regression is used to determine nonstationary thresholds, which relies on the fact that sample quantiles can be determined by the solution of a linear optimization problem. Given a set of observations (xi), the τth regression quantile is the solution of
(6)
where:
(7)
(Koenker and Basset Citation1978; Koenker Citation2005). In the event that the covariate in question varies linearly with time (t), for example, the τth conditional quantile function becomes:
(8)
which can be estimated by:(9)
where xt is a set of time-dependent observations, and β0 and β1 are the intercept and the slope components of the regression quantiles, respectively. For a more detailed explanation of quantile regression, please refer to Koenker (Citation2005).
This research follows the methodology of Roth et al. (Citation2014), which provides a basis for time-dependent threshold selection. That is, for a selected time-dependent threshold, the TC plot of the scale parameter for the selected threshold (τ0) should be approximately constant and the mean residual life plot should be approximately linear for all . In addition to the mean residual life plot and TC plots, dispersion index plots were examined for all
to ensure a homogeneous Poisson process for threshold exceedances.
Four bivariate quantile regression models are used for nonstationary threshold modelling along with two multivariate models, all of which incorporate a climate oscillation index and/or time as covariates.
Trend detection
There exist comparable trend detection tests in terms of their power of trend detection (Yue et al. Citation2002) yet the Mann–Kendall test is widely applied and is therefore used for this research (Mann Citation1945; Kendall Citation1975). The presence of positive/negative serial correlation in hydrometric data can increase/decrease the likelihood of finding a significant trend (von Storch Citation1995). Although the data used for this analysis were declustered to ensure independence of events, any potential residual serial correlation was accounted for using the nonparametric block bootstrap (BBS) technique (Kundzewicz and Robson Citation2000). This approach is carried out by resampling data in blocks, the length of which is selected based on the number of contiguous lags of significant autocorrelation. After resampling is carried out a large number of times (2000 is implemented for this research), the empirical distribution of the results can be used to assess the significance of trends in the data set. The benefit in using the BBS technique is that the autocorrelation structure of the data is preserved, whereas most techniques require the adoption of a lag-one autoregressive model [AR(1)]. For more information regarding the BBS technique, please refer to Khaliq et al. (Citation2009) or Önöz and Bayazit (Citation2012).
To determine whether significant (local) trends found using the Mann–Kendall test have occurred by chance, the field (global) significance is determined. Field significance is determined using a group block bootstrapping approach (GBBS) which assesses the significance of increasing and decreasing trends separately (Yue et al. Citation2003; Khaliq et al. Citation2009). The goal of this technique is to determine the number of significant trends that occur by chance for a selected number of stations while maintaining the serial structure of the original data. Vector resampling of years is carried out in blocks that preserve the serial structure of the original data. Each station having data for the block bootstrapped year vector is added into a resampled data set, and this continues until the desired number of station years are met (i.e. the resampled data set contains the same number of station-years of data as the original data set). This process is repeated a large number of times (1000 herein), where trend detection is carried out for each resampled data set. The empirical distribution of trends and subsequent p-value are then determined, which are then employed to determine field significance.
Parameter estimation
The method of maximum likelihood (ML) is used for parameter estimation due to the ease of incorporation of covariates into distributional parameters. In the case of a stationary model, the log-likelihood function, , is expressed as (Coles Citation2001):
(10)
where θ is a vector of parameters, is the probability density function, and xi is the time-independent series of data. In the event that parameter estimation is to be carried out in a nonstationary (time-dependent) context, the log-likelihood function is denoted by (O’Brien and Burn Citation2014):
(11)
where θt is a vector of nonstationary parameters, is the density function, xt are the time-dependent at-site data; and
represents the nonstationary distribution parameters. Due to the complex nature of the log-likelihood function, parameter estimates are commonly determined numerically through the use of non-linear optimization techniques; the Nelder–Mead simplex algorithm is used for this analysis (Nelder and Mead Citation1965).
Model selection
Figure outlines the two-stage approach that is applied in choosing the most suitable model for each data set. The first stage involves calculating Akaike information criterion (AIC) weights that are used as goodness-of-fit criteria for the (non)stationary GP model selection. After the most suitable GP model is selected for each threshold, the best fitting threshold models are selected by means of the root mean square error (RMSE).
Akaike weights are initially employed as a goodness-of-fit measure to facilitate the selection of the stationary or nonstationary scale parameter models for each threshold. The AIC is given by (Akaike Citation1974):
(12)
where K is the number of parameters. Using raw AIC values, the difference between the minimum AIC value and those of the other models is determined (Burnham and Anderson Citation2002):
(13)
where is the difference in AIC with respect to the minimum AIC; AICj is the raw AIC for each model; and minAIC is the minimum AIC value. Akaike weights are then calculated by (Burnham and Anderson Citation2002):
(14)
where is the AIC weight for model j and
The GP distribution model with the largest AIC weight is selected as the most suitable. If any AIC weight falls within 10% of the maximum, the more parsimonious model is selected. Estimates of the GP distribution model parameters are determined using the ‘ismev’ package in R (Heffernan and Stephenson Citation2009; R Core Team Citation2016). The ‘gp.fit’ function in this package allows for the estimation of stationary and nonstationary GP distribution parameters through ML estimation, with the option of adding a trend component into selected parameters.
Having selected the best-fitting GP model for each threshold (stationary or nonstationary), the goodness of fit of the threshold models is ascertained through a quantile plot (Q–Q) comparison. In the event of a nonstationary scale parameter and/or nonstationary threshold, a residual quantile plot is produced by transforming data to a standard exponential distribution (Coles Citation2001), where (for example) time-dependent parameters are included:
(15)
where is the transformed exponential time-dependent data, and ut is the nonstationary threshold at time t. The selection of the best-fitting threshold was determined using the RMSE, through which the agreement between the empirical and modelled quantiles is assessed. The threshold model having the lowest RMSE is selected, although if any threshold model falls within 10% difference of the lowest RMSE, the model with the smaller number of parameters is selected to preserve model parsimony.
Uncertainty
A balanced resampling approach is implemented for the calculation of quantile confidence intervals (CI). This technique involves first creating a large number of copies (999 is used herein) of the original sample data and concatenating the copies. This concatenated vector of data is then randomly permutated and broken back into the original number of copies. The resulting 999 bootstrapped resamples can then be used to estimate the uncertainty present in the quantile estimates (Burn Citation2003).
In the event that nonstationarity is present in the scale parameter of the original data set of interest, data are transformed to remove the covariate-dependence before resampling. Given that the GP distribution is applied for this research and using time as an example, nonstationary data were transformed to IID data using the following (Coles Citation2001):
where is the transformed residual series at time t, xt is the original data series at time t, and ut is the time-dependent threshold. Data are transformed before bootstrapping and final samples are calculated using the inverse of Equation (14).
Application
Case study area
Total daily precipitation data, retrieved from the National Climate Data Archive of Environment and Climate Change Canada, are used for this research. Data from 30 gauges throughout coastal BC are used for the implementation of a POT analysis. Before threshold selection is carried out, data are separated into winter season events, spanning from mid-October to mid-April, and summer season events, encompassing the remainder of the year. Winter storms in BC are dominated by midlatitude cyclonic activity, resulting in substantial precipitation amounts. The summer climate is characterized by a subtropical high-pressure system that results in less frequent and intense storms (Walker et al. Citation2008). Therefore, seasonal partitioning of the data is necessary to reflect the different storm-generating mechanisms.
Figure shows the locations of 30 observation stations used in the analysis, six of which are located in Metro Vancouver. Table provides a spatial summary of the station characteristics in three geographical areas, which include the North Coast, the South Coast and Vancouver Island. The elevations and locations of the meteorological stations have a discernible impact on the mean annual precipitation recorded at these locations. Substantial variability in mean annual precipitation is evident throughout coastal BC. Windward areas and those at higher elevations receive greater annual precipitation amounts. Also, the rain shadow of Vancouver Island produces drier conditions in the south coast interior (Moore et al. Citation2010).
Table 1. Summary of stations used for analysis.
The precipitation data used for this analysis were initially screened for obvious transcription errors and uncharacteristically low or high values. Observation stations for this analysis were retained for analysis if they have no more than four consecutive years of missing observations. All stations with daily data west of the mainland Coast Mountains meeting these criteria were included in the analysis. Table provides a summary of the percentage of missing data at each of the 30 retained meteorological stations. All the stations, aside from North Pender Island, have less than 20% missing observations from the period of record; 27 of the stations have less than 10% missing data. It is acknowledged that bias may be present in the precipitation measurements due to variations in wind speed and differences in instrumentation. Furthermore, the current analysis employs a 2-day separation time between maxima as a means of retaining peak precipitation from individual events (Coles Citation1993; Caires et al. Citation2006; Kyselý and Beranová Citation2009; Roth et al. Citation2012). Also, all data were found to be free of step-changes prior to parameter estimation.
Results
Threshold selection and models
Correlations between climate indices and monthly precipitation amounts were assessed for positive and negative lags of cross-correlation function (CCF) plots at the 5% significance level for the Niño 3.4 and PDO indices. It was determined that the PDO index is more strongly correlated with the monthly precipitation amounts in both the summer (73% of cases for PDO/27% for Niño 3.4) and winter seasons (76% of cases for PDO/24% for Niño 3.4). For this reason, the PDO index and/or time are used as covariates for the univariate and bivariate thresholds and GP models used for this analysis. Table outlines the nonstationary threshold models employed.
Table 2. Quantile regression threshold models.
GP distribution – model selection
The GP distribution is fit to threshold exceedance data established through the use of stationary and nonstationary thresholds; the shape parameter was kept constant in all instances. The stationary and nonstationary GP distribution models used for this analysis are outlined in Table . Using the log-likelihood estimates provided by the ‘gp.fit’ function, AIC weights are calculated for the stationary and nonstationary threshold exceedance data. Results from the Mission West Abbey observation station are provided in Table for the summer season data as an example of the model selection process, and are typical of all results. The GP scale parameter model with the highest weight is selected as the most appropriate model for all thresholds except for ucov2 and ucov6, where ucov2 is the linear, time-dependent threshold and ucov6 is the quadratic time- and PDO-dependent threshold. In these two instances, there was a tie for the highest weight; therefore, the more parsimonious model was selected for both threshold models.
Table 3. Peaks over threshold data for the generalized Pareto distribution quantile functions.
Table 4. Akaike information criterion weights for the Mission West Abbey observation station – summer season.
The best fitting threshold model was then determined using the models that provided the highest AIC weights. This is carried out through the comparison of quantile or residual quantile plots (for nonstationary models), where the agreement between the model and empirical quantiles is measured using the RMSE. An example is provided in Figure , showing the results for the summer season quantile plots at the Mission West Abbey observation station. Figure (c) was chosen as the most suitable model as it is the most parsimonious within 10% difference of the minimum RMSE of all the threshold models. Tables and outline the final selection of models, the associated parameter estimates of the GP distribution models, and the applicable stationary or nonstationary threshold parameters.
Figure 4. Comparison of residual quantile plots of above threshold data from the summer season at observation station Mission West Abbey: (a) stationary threshold and quadratic PDO-dependent scale parameter; (b) linear time-dependent threshold and linear PDO-dependent scale parameter; (c) linear PDO-dependent threshold and linear PDO-dependent scale parameter; (d) linear time- and PDO-dependent threshold and linear PDO-dependent scale parameter; (e) quadratic time-dependent threshold and linear PDO-dependent scale parameter; (f) quadratic PDO-dependent threshold and linear PDO-dependent scale parameter; (g) quadratic time- and PDO-dependent threshold and linear PDO-dependent scale parameter.
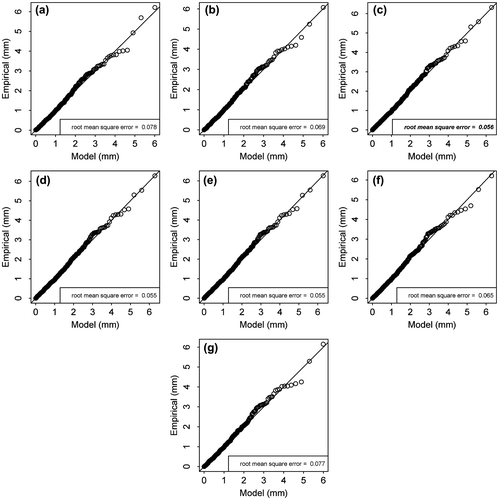
Table 5. Final threshold selection and model parameters for the winter season data.
Table 6. Final threshold selection and model parameters for the summer season data.
Trend detection summary
A summary of the trend analysis of the magnitude and frequency of the POT data is presented in Figures through . Two analysis periods are examined (39 and 29 years) for all 30 stations and for the winter and summer seasons at 5% and 10% significance levels.
Figure 5. Winter season stationary threshold exceedance trend locations. Bold and italicized legend fonts indicate trends that are globally significant.
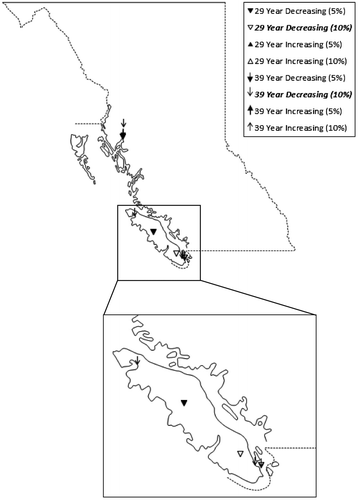
Figure indicates that the winter season threshold exceedance data are dominated by decreasing trends. Trends are observed at 5% and 10% significance levels for threshold exceedances for both analysis periods at several sites in the North Coast and Vancouver Island regions. Although a number of statistically significant decreasing trends were identified, only those for the 1976–2014 (39-year) period at the 10% significance level were determined to be field significant. A globally significant increasing trend is also identified in Figure for the 1986–2014 (29-year) analysis period at 5% and 10% significance levels at the Metchosin (V8) station only.
Figure 6. Winter season frequency of stationary threshold exceedance trend locations. Bold and italicized legend fonts indicate trends that are globally significant.
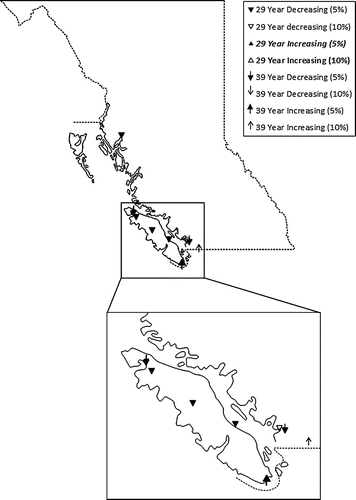
Results of the Mann–Kendall trend test for the summer season above threshold and frequency data (Figures and , respectively) indicate that there are a number of both increasing and decreasing trends, which are located primarily in the South Coast and Vancouver Island region. It should be noted, however, that globally significant trends were only found in the peak data (Figure ), which include 39-year increasing trends at the 10% significance level and 29-year decreasing trends at the 10% significance level. These results highlight the importance of trend identification in different analysis periods.
Figure 7. Summer season stationary threshold exceedance trend locations. Bold and italicized legend fonts indicate trends that are globally significant.
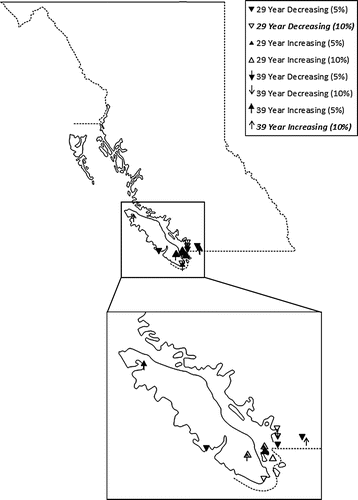
Quantile estimation summary
Quantile estimates are calculated for the 50-year events for each of the winter and summer season data using Equation (2), and are illustrated in Figures 9 and 10, respectively. If a stationary model is determined to be the best fit, a comparison with nonstationary quantiles is not warranted and is therefore not presented. For all the remaining data sets, a comparison between the best-fitting stationary and nonstationary model quantiles is provided. Due to the inclusion of PDO as a covariate, the quantile estimates are not consistently smooth, particularly in the case of a PDO-dependent GP scale parameter. Trends identified through the Mann–Kendall trend test are included in the top right corner of Figures 9 and 10 as a means of comparison.
For the winter season data, 12 of the 30 sites are identified as stationary through the model selection technique. All of the sites in the North Coast region are identified as nonstationary (decreasing), whereas 56% (5 of 9) and 39% (7 of 18) of sites in the South Coast and Vancouver Island regions are, respectively. Of the remaining four stations on the South Coast, two (S1 and S7) display very mild nonstationarity, while the remaining two (S8 and S9) reveal increasing 50-year quantiles. Within the Vancouver Island region, seven of the stations have stationary quantiles (V1, V3, V11, V15, V16, V17 and V18), while seven of the remaining stations show nominal changes in quantiles with respect to time (V2, V4, V5, V8, V9, V12 and V13). The four remaining sites exhibit discernable nonstationarity, three of which are increasing (V7, V10 and V14) and one of which is decreasing (V6). A decreasing trend was also identified at station V6 at the 5% significance level for the 29-year period (1986–2014). An inconsistent decreasing trend is identified at station V14, but this could be due to the marked difference in the period of record of the station data and the 29- or 39-year time frames. The magnitude of uncertainty in the quantile estimates is similar for both stationary and nonstationary models, aside from site V10 which has smaller stationary CIs. Given that the stationary threshold and GP model provides substantially less uncertainty for this particular site, confidence in these nonstationary estimates is low.
The summer season data show slightly less stationarity, with 10 of the 30 sites having time-independent quantiles. In the North Coast region, two of the three sites are stationary, with the remaining nonstationary site (N2) showing little discernable change over the period of record. Three of the nine sites in the South Coast region are stationary (S3, S7 and S9) and three have minimal nonstationarity or fluctuate around a constant mean value (S2, S4 and S5). Of the remaining three stations, two show decreasing and one increasing 50-year quantiles, although nonstationary estimates for station S6 have slightly more uncertainty than their stationary counterparts. Finally, Vancouver Island contains five stationary sites, but of those remaining, only two (V16 – decreasing and V18 – increasing) show apparent changes in 50-year peak return period precipitation estimates. In agreement with the winter season findings, the uncertainty levels in the stationary and nonstationary 50-year quantile estimates are relatively comparable, aside from those of site V18. There is noticeably more uncertainty in the nonstationary quantiles for this station; therefore, there is minimal confidence in these estimates.
Regional pattern overview
Figure demonstrates that the North Coast, winter-season 50-year quantile estimates are generally decreasing, although one site (N2) shows very little change over the period of record. Furthermore, the trend analysis identified a decreasing trend at N1 for the 1986–2014 period at the 5% significant level, and a decreasing trend for the 1976–2014 period was detected at site N3 at the 10% significance level. One trend was also identified in the North Coast region frequency data in the winter season, a variable for which the trend results are field significant (Figure ). Although the detected trends in the magnitude of the POT data were not determined to be field significant, these findings suggest overall decreasing peak precipitation in the winter months. An analysis of Figure reveals that there is minimal indication of nonstationarity in the summer peak quantile estimates, as well as no trends being identified at those sites. The GP and threshold model parameters in the winter season for the North Coast displayed both PDO- and time-dependence, although the models selected for the summer months are dominated by stationarity (Tables and ). Furthermore, divergent nonstationary behaviour is observed in the winter quantile estimates at stations N1 and N2, although these two stations are very closely located. Differences in the nonstationary quantile estimates at these two stations may be due to other factors such as changes in instrumentation and location over time.
Figure 9a. (N1–S9). 50-year nonstationary and/or stationary quantiles and their associated 95% confidence intervals – winter season.
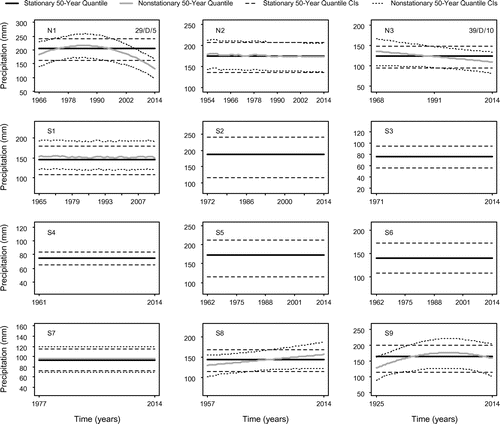
Figure 9b. (V1–V12). 50-year nonstationary and/or stationary quantiles and their associated 95% confidence intervals – winter season.
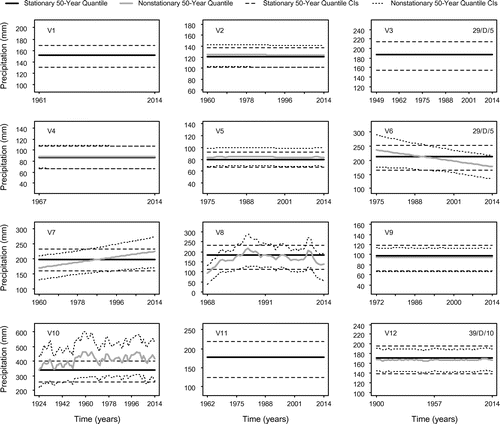
Figure 9c. (V13–V18). 50-year nonstationary and/or stationary quantiles and their associated 95% confidence intervals – winter season.
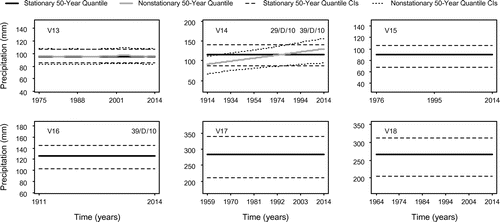
Figure 10a. (N1–S9). 50-year nonstationary and/or stationary quantiles and their associated 95% confidence intervals – summer season.
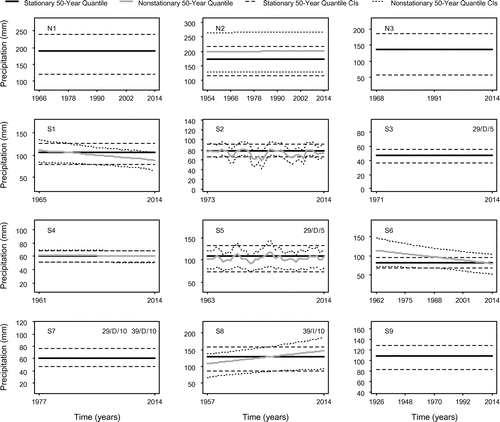
Figure 10b. (V1–V12). 50-year nonstationary and/or stationary quantiles and their associated 95% confidence intervals – summer season.
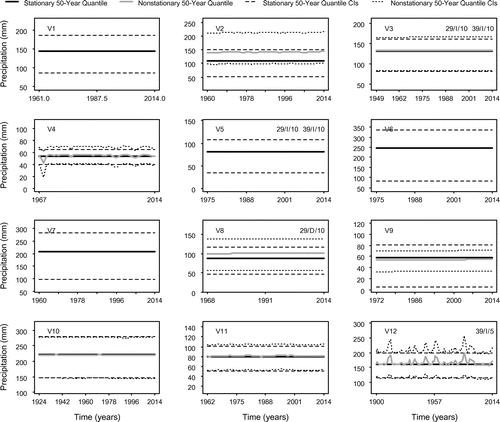
Figure 10c. (V13–V18). 50-year nonstationary and/or stationary quantiles and their associated 95% confidence intervals – summer season.
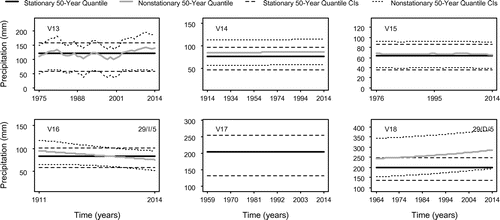
Figures and illustrate that Vancouver Island is dominated by decreasing trends in above-threshold occurrences and the frequency of those occurrences in the winter season. Globally significant decreasing trends were identified for the above-threshold occurrences for the 39-year period decreasing trends at the 10% significance level. Additionally, one field-significant increasing trend was identified (Figure ) for the winter 1986–2014 period at 5% and 10% significance levels. A combination of increasing and decreasing trends were found in the summer months in the magnitude and frequency of POT events; however, only increasing above-threshold trends for the 39-year period (10% significance level) and decreasing trends for the 29-year period (10% significance level) were found to be field significant. The winter quantile estimates have somewhat contradictory results, given that a number of sites are discernably increasing. Also, the summer 50-year quantile estimates were predominantly stationary, yet a number of significant trends were identified through the trend analysis. Finally, an analysis of the threshold models from Tables and selected for Vancouver Island suggests that the central tendency of the winter and summer season data is influenced by both time and PDO. An analysis of the GP scale parameter estimates reveals that the winter variability is more likely stationary.
The South Coast region is characterized by relatively stationary quantiles for both seasons, although, several fluctuate about a constant mean. However, two sites have noticeably increasing 50-year quantile estimates in the winter, and summer quantiles indicate that several sites have discernably increasing and decreasing quantiles as well. Two significant decreasing trends were identified in the winter season, while summer season trends for both the frequency and magnitude of POT events were generally decreasing. Additionally, the South Coast region shows a mixture of time- and PDO-dependence in the summer in all model parameters, while the winter season appears to be rather stationary.
Discussion
This section includes a comparison of this analysis with other similar studies. Such comparison is a challenging task due to differences in methodologies, but is nonetheless a valuable undertaking.
In an analysis of daily precipitation data throughout Canada, Stone et al. (Citation2000) found decreasing trends in heavy precipitation frequency in winter months in coastal BC, which is consistent with the findings of this work. Increases in the frequency of peak summer events were also identified by Stone et al. (Citation2000); however, their research did not find globally significant trends in summer frequency data. Zhang et al. (Citation2000) found statistically significant increases in gridded daily precipitation in summer months in the southwestern portion of BC for the 1900–1998 and 1950–1998 periods. The findings of the quantile analysis for the South Coast region herein are in agreement with these findings. Zhang et al. (Citation2000) also identified significant increasing trends in an area similar to that of the North Coast region for the 1900–1998 period, which is generally consistent with the quantile analysis of this area. In a Canada-wide analysis, Vincent and Mekis (Citation2006) examined numerous precipitation and temperature indices from 1950 to 2003. They found an increase in the number of days with snow and rain in coastal BC, although these finding were not statistically significant. However, significant increasing trends in very wet days (95th percentile) were primarily identified on Vancouver Island and in the North Coast region, which is inconsistent with the results of this research, given that the detected trends in frequency of over-threshold events were generally decreasing (significant or otherwise). Burn et al. (Citation2011) used hourly precipitation to carry out a POT analysis of events of varying duration in BC. The results of this analysis for the summer season found statistically and globally significant trends in the frequency of above-threshold events for 24-h-duration storms (40-year period). However, no globally significant trends were found in the summer frequency data herein. Also, the winter season frequency data of Burn et al. (Citation2011) are dominated by increasing trends, although none was field significant. These trend results are somewhat consistent with those of the winter trend analysis carried out herein, whereby a globally significant increasing trend was found for the winter frequency data. The winter peak magnitude data herein are dominated by decreasing trends. However, some evidence of increasing winter quantiles was found in the Vancouver Island and South Coast regions.
Mote (Citation2003) found predominantly increasing trends in daily precipitation for the winter season from 1950 to 2000 in southern coastal BC, which is consistent with the quantile estimates from the South Coast region. Mote (Citation2003) also found a mixture of increasing and decreasing trends on Vancouver Island, which is also consistent with the results herein. In an analysis of Canadian daily total rainfall, Vincent and Mekis (Citation2009) found increasing trends in summer and decreases in winter precipitation in southwestern BC. These results are somewhat similar to those found for the North Coast region in the winter and the South Coast region in the summer. Although the work of Mote (Citation2003) and Vincent and Mekis (Citation2009) used daily precipitation indices, they did not assess peak precipitation trends; therefore, a direct comparison between these two studies is not possible.
General circulation model (GCM) projections for coastal BC indicate the potential for wetter winter seasons and drier conditions in the summer months (British Columbia Ministry of Environment [BCME] Citation2007; Walker et al. Citation2008). The results established through the trend analysis are somewhat consistent with the above-noted projections for the winter season given that globally and statistically significant increasing trends in frequency were found for the 29-year analysis period at both significance levels. Also in agreement, a number of trends in the summer season were found to be decreasing. It should also be noted that the results of the quantile analysis provided better agreement with likely climate change projections.
Whitfield et al. (Citation2010) describe the PDO as a powerful post hoc explanatory concept, limiting the forecasting potential with this index. Therefore, care should be taken in extrapolating the quantile estimates past the period of record, particularly in instances with strong quadratic behaviour. One of the anonymous reviewers suggested that concave quadratic behaviour may possibly be due to a period of predominant La Niña between approximately 1990 and 2012, which led to a long period of cold PDO SSTs.
Conclusions
This paper incorporates existing nonstationary extreme-value approaches to develop a methodology for the selection of stationary/nonstationary thresholds. Nonstationary threshold selection is carried out through quantile regression, which allows for the inclusion of bivariate and multivariate models. A two-stage selection process is proposed, in which AIC weights are initially employed to determine the best-fitting (non)stationary GP distribution model for each threshold. Threshold/GP distribution models are then compared through the use of Q–Q plots. This methodology is developed as a complementary approach to trend detection which allows the user to visually identify potential trends in peak quantile estimates.
There is more evidence of stationarity in the North Coast winter and summer over-threshold data, which is confirmed by the quantiles and trend analyses. Similar results are revealed for the South Coast region, although there is some evidence of increasing quantiles in the winter. Vancouver Island shows the most potential for nonstationary increasing peak 50-year quantiles in both seasons, although there is some evidence of decreases in the winter. Through the trend analysis, it was revealed that a number of significant trends were found in the magnitude and frequency of POT data for both seasons.
In general, the results from the quantile and trend analyses are in agreement with previously published work. Furthermore, dissimilarities in the trend and quantiles analyses reinforce the usefulness of investigating trends through various means and highlight the importance of comparisons within various periods of record. Based on this historical data trend analysis, there is less risk of serious precipitation-related winter events; however, the frequency of these events is increasing in recent years. Furthermore, the quantile analysis suggests that there is moderate risk of increases/decreases in winter or summer hydrometeorological hazards in coastal BC.
Acknowledgements
The authors greatly appreciate the useful comments by the Associate Editor and two anonymous reviewers. The authors gratefully acknowledge the support of the Natural Sciences and Engineering Research Council of Canada (NSERC). Data used for this research were obtained from Environment and Climate Change Canada’s Historical Climate Database.
References
- Akaike, H. 1974. New look at statistical-model identification. IEEE Transaction on Automatic Control, AC 19 (6): 716–723.10.1109/TAC.1974.1100705
- Balkema, A. A., and L. de Hann. 1974. Residual life time at great age. Annals of Probability 2 (5): 792–804.10.1214/aop/1176996548
- Beguería, S., M. Angula-Martínez, S. Vincente-Serrano, J. I. López-Moreno, and A. El-Kenawy. 2011. Assessing trends in extreme precipitation events intensity and magnitude using non- stationary peaks-over-threshold analysis: A case study in northeast Spain from 1930 to 2006. International Journal of Climatology 31: 2102–2114.10.1002/joc.v31.14
- British Columbia Ministry of Environment (BCME). 2007. Environmental trends in British Columbia: 2007, 352 pp.
- Burn, D. H. 2003. The use of resampling for estimating confidence intervals for single site and pooled frequency analysis. Hydrological Sciences Journal 48 (1): 25–38.10.1623/hysj.48.1.25.43485
- Burn, D. H., R. Mansour, K. Zhang, and P. H. Whitfield. 2011. Trends in variability in extreme rainfall event in British Columbia. Canadian Water Resources Journal 36 (1): 67–82.10.4296/cwrj3601067
- Burnham, K. P., and D. R. Anderson. 2002. Model selection and multimodel inference. New York, NY: Springer, 488 pp.
- Caires, S., V. R. Swail, and X. L. Wang. 2006. Projection and analysis of extreme wave climate. Journal of Climate 19: 5581–5605.10.1175/JCLI3918.1
- Chang, S. E., M. Gregorian, K. Pathman, L. Yumagulova, and W. Tse. 2012. Urban growth and long-term changes in natural hazard risk. Environment and Planning A 44: 989–1008.10.1068/a43614
- Coles, S. 1993. Regional modelling of extreme storms via max-stable processes. Journal of the Royal Statistical Society: Series B 55 (4): 797–816.
- Coles, S. 2001. An introduction to statistical modeling of extreme values. London, United Kingdom: Springer, 208 pp.10.1007/978-1-4471-3675-0
- Cunnane, C. 1979. A note on the Poisson assumption on partial duration series models. Water Resources Research 15 (2): 489–494.10.1029/WR015i002p00489
- El Adlouni, S., T. B. M. J. Ouarda, X. Zhang, R. Roy, and B. Bobée. 2007. Generalized maximum likelihood estimators for the nonstationary generalized extreme value model. Water Resources Research 43: W03410. doi:10.1029/2005WR004545.
- Gershunov, A., and T. P. Barnett. 1998. ENSO influence on intraseasonal extreme rainfall and temperature frequencies in the contiguous United States: Observations and model results. Journal of Climate 11: 1575–1586.10.1175/1520-0442(1998)011<1575:EIOIER>2.0.CO;2
- Gilroy, K. L., and R. H. McCuen. 2012. A nonstationary flood frequency analysis method to adjust for future climate change and urbanization. Journal of Hydrology 414-415: 40–48.10.1016/j.jhydrol.2011.10.009
- Hare, F. K., and M. K. Thomas. 1979. Climate Canada. 2nd ed. New York, NY: Wiley, 230 pp.
- Hartmann, D. L., A. M. G. Klein Tank, M. Rusticucci, L. V. Alexander, S. Brönnimann, Y. Charabi, F. J. Dentener, et al. 2013. Observations: Atmosphere and surface. In Climate change 2013: The physical science basis. Contribution of Working Group I to the Fifth Assessment Report of the Intergovernmental Panel on Climate Change, eds. T. F. Stocker, D. Qin, G.–K. Platter, M. Tignor, S. K. Allen, J. Boschung, A. Nauels, et al. Cambridge, United Kingdom and New York, NY, USA: Cambridge University Press, 30 pp.
- Heffernan, J. E., and A. G. Stephenson. 2009. An introduction to statistical modeling of extreme values. R package ‘ismev’. http://cran.r-project.org/web/packages/ismev/ismev.pdf.
- Hundecha, Y., A. St-Hilaire, T. B. M. J. Ouarda, and S. El Adlouni. 2008. A nonstationary extreme value analysis for the assessment of changes in extreme annual wind speed over the Gulf of St. Lawrence, Canada. Journal of Applied Meteorology and Climatology 47: 2745–2759. doi:10.1175/2008JAMC1665.1.
- Jonathan, P., K. Ewans, and D. Randell. 2013. Joint modelling of extreme ocean environments incorporating covariate effects. Coastal Engineering 79: 22–31.10.1016/j.coastaleng.2013.04.005
- Jonathan, P., D. Randell, Y. Wu, and K. Ewans. 2013. Nonstationary conditional extremes of northern North Sea storm characteristics. Environmentrics 25: 172–188. doi:10.1002/env.2262.
- Jonathan, P., D. Randell, Y. Wu, and K. Ewans. 2014. Return level estimation from non- stationary spatial data exhibiting multidimensional covariate effects. Ocean Engineering 88: 520–532.10.1016/j.oceaneng.2014.07.007
- Katz, R. W. 2010. Statistics of extremes in climate change. Climatic Change 100: 71–76.10.1007/s10584-010-9834-5
- Katz, R. W., M. B. Parlange, and P. Naveau. 2002. Statistics of extremes in hydrology. Advances in Water Resources 25: 1287–1304.10.1016/S0309-1708(02)00056-8
- Kay, A. L., and D. A. Jones. 2012. Transient changes in flood frequency and timing in Britain under potential projections of climate change. International Journal of Climatology 32: 498–502.
- Kendall, M. G. 1975. Rank correlation methods. London: Griffin, 202 pp.
- Khaliq, M. N., T. B. M. J. Ouarda, P. Gachon, L. Sushama, and A. St-Hilaire. 2009. Identification of hydrological trends in the presence of serial and cross correlations: A review of selected methods and their application to annual flow regimes of Canadian rivers. Journal of Hydrology 368: 117–130.10.1016/j.jhydrol.2009.01.035
- Khaliq, M. N., T. B. M. J. Ouarda, J.-C. Ondo, P. Gachon, and B. Bobée. 2006. Frequency analysis of a sequence of dependent and/or non-sationary hydro-meteorological observations: A review. Journal of Hydrology 329: 534–552.10.1016/j.jhydrol.2006.03.004
- Kharin, V. V., and F. W. Zwiers. 2005. Estimating extremes in transient climate change simulations. Journal of Climate 18: 1156–1173.10.1175/JCLI3320.1
- Koenker, R. 2005. Quantile regression. New York, NY: Cambridge University Press, 352 pp.10.1017/CBO9780511754098
- Koenker, R., and B. G. Basset. 1978. Regression quantiles. Econometrica 46 (1): 33–50.10.2307/1913643
- Kundzewicz, Z. W. and A. J. Robson. 2000. Detecting trend and other changes in hydrological data. World Climate Program-Data and Monitoring. World Meteorological Organization, Geneva (WMO/TD-No. 1013).
- Kyselý, J., and R. Beranová. 2009. Climate-change effects on extreme precipitation in central Europe: Uncertainties of scenarios based on regional climate models. Theoretical and Applied Climatology 95: 361–374.10.1007/s00704-008-0014-8
- Kyselý, J., J. Picek, and R. Beronová. 2010. Estimating extremes in climate change simulations using the peaks-over-threshold method with a non-stationary threshold. Global and Planetary Change 72: 55–68.10.1016/j.gloplacha.2010.03.006
- Lang, M., T. B. M. J. Ouarda, and B. Bobée. 1999. Towards operational guideline for over- threshold modeling. Journal of Hydrology 225: 103–117.10.1016/S0022-1694(99)00167-5
- Li, J., and S. Tan. 2015. Nonstationary flood frequency analysis for annual flood peak series, adopting climate indices and check dam index as covariates. Water Resources Management 29: 5533–5550.10.1007/s11269-015-1133-5
- Mailhot, A., S. Lachance-Cloutier, G. Talbot, and A.-C. Favre. 2013. Regional estimates of intense rainfall based on peaks-over-threshold (POT) approach. Journal of Hydrology 476: 188–199.10.1016/j.jhydrol.2012.10.036
- Mann, H. B. 1945. Nonparametric tests against trend. Econometrica 13: 245–259.
- Mantua, N. J., S. R. Hare, Y. Zhang, J. M. Wallace, and R. C. Francis. 1997. A Pacific interdecadal climate Oscillation with impact on salmon production. Bulletin of the American Meteorological Society 78: 1069–1079.10.1175/1520-0477(1997)078<1069:APICOW>2.0.CO;2
- Milly, P. D. D., J. Betancourt, M. Falkenmark, R. Hirsch, W. Zbigniew, D. Kundzewicz, D. P. Lettenmaier, and R. J. Stouffer. 2008. Stationarity is dead: Whither water management? Science 319 (5863): 573–574.10.1126/science.1151915
- Moore, R.D., D.L. Spittlehouse, P.H. Whitfield, and K. Stahl. 2010. Chapter 3: Weather and climate. In Compendium of forest hydrology and geomorphology in British Columbia, eds, R.G. Pike, T.E. Redding, R.D. Moore, R.D. Winkler, and K.D. Bladon, 47–84. BC Ministry of Forests and Range, Research Branch, Victoria, BC and FORREX Forest Research Extension Partnership, Kamloops, BC.
- Mote, P. W. 2003. Twentieth-century fluctuations and trends in temperature, precipitation, and mountain snowpack in the Georgia Basin – Puget Sound region. Canadian Water Resources Journal 28 (4): 567–585.10.4296/cwrj2804567
- Nadarajah, S. 2005. Extremes of daily rainfall in west central Florida. Climate Change 63: 325–342.10.1007/s10584-005-1812-y
- Nelder, J. A., and R. Mead. 1965. A simplex method for function minimization. Computer Journal 7: 308–313.10.1093/comjnl/7.4.308
- Northrop, P. J., and P. Jonathan. 2011. Threshold modelling of spatially dependent non-stationary extremes with application to hurricane-induced wave heights. Environmetrics 22(7): 799–809. doi:10.1002/env.1106.
- O’Brien, N. L., and D. H. Burn. 2014. A nonstationary index-flood technique for estimating extreme quantiles for annual maximum streamflow. Journal of Hydrology 519: 2040–2048.10.1016/j.jhydrol.2014.09.041
- Önöz, B., and M.Bayazit. 2012. Block bootstrap for Mann-Kendall trend test of serially dependent data. Hydrological Processes 26: 3552–3560.
- Ontario Ministry of the Environment (OME). 2008. Design guidelines for sewage works. ISBN 978-1-4249-8438-1. https://www.ontario.ca/document/design-guidelines-sewage-works.
- Osman, Y. Z., R. Fealy, and J. C. Sweeney. 2013. Downscaling extreme precipitation in Ireland using combined peaks-over-threshold generalized Pareto distribution model of varying parameters. Journal of Water and Climate Change 4 (4): 21–47. doi:10.2166/wcc.2013.071.
- Ouarda, T. B. M. J., and S. El-Adlouni. 2011. Bayesian nonstationary frequency analysis of hydrological variables. Journal of the American Water Resources Association 47 (3): 496–505.10.1111/j.1752-1688.2011.00544.x
- Pettitt, A. N. 1979. A non-parametric approach to the change-point problem. Journal of Applied Statistics 28: 126–135.10.2307/2346729
- Pickands, J. 1975. Statistical inference using extreme order statistics. Annals of Statistics 3 (1): 119–131.
- R Core Team. 2016. R: A Language and Environment for Statistical Computing. Vienna, Austria: R Foundation for Statistical Computing. http://www.R-project.org/. ISBN 3-900051-07-0.
- Rajagopalan, B. 2010. Hydrologic frequency analysis in a changing climate. Workshop on Nonstationarity, Hydrologic Frequency Analysis, and Water Management, Jan 13-15, 107-114. Boulder, Colorado, U.S.A: Millennium Harvest House.
- Rayner, N. A., D. E. Parker, E. B. Horton, C. K. Folland, L. V. Alexander, D. P. Rowell, E. C. Kent, A. Kaplan. 2003. Global analyses of sea surface temperature, sea ice, and night marine air temperature since the late nineteenth century. Journal of Geophysical Research 108: 4407. doi. 10.1029/2002JD002670.
- Renard, B., M. Lang, and P. Bois. 2006. Statistical analysis of extreme events in a non- stationary context via a Bayesian framework: Case study with peak-over-threshold data. Stochastic Environmental Research and Risk 21 (2): 97–112.10.1007/s00477-006-0047-4
- Roth, M., T. A. Buishand, G. Jongbloed, A. M. G. Klein Tank, and J. H. van Zanten. 2012. A regional peaks-over-threshold model in a nonstationary climate. Water Resources Research 48: W11533. doi:10.1029/2012WR012214.
- Roth, M., T. A. Buishand, G. Jongbloed, A. M. G. Klein Tank, and J. H. van Zanten. 2014. Projections of precipitation extremes based on a regional non-stationary peaks-over-threshold approach: A case study for the Netherlands and north-western Germany. Weather and Climate Extremes 4: 1–10.10.1016/j.wace.2014.01.001
- Roth, M., G. Jongbloed, and T. A. Buishand. 2016. Threshold selection for regional peaks- over-threshold data. Journal of Applied Statistics 43 (7): 1291–1309.10.1080/02664763.2015.1100589
- Stephens, K. A., and P. Graham, D. Reid 2002. Stormwater planning: A guidebook for British Columbia. Province of British Columbia. http://www.env.gov.bc.ca/epd/epdpa/mpp/stormwater/guidebook/pdfs/stormwater.pdf.
- Stone, D. A., A. J. Weaver, and F. W. Zwiers. 2000. Trends in Canadian precipitation intensity. Atmosphere-Ocean 38 (2): 321–347.
- Sugahara, S., R. Porfírio da Rocha, and R. Silveira. 2009. Non-stationary frequency analysis of extreme daily rainfall in Sao Paulo, Brazil. International Journal of Climatology 29: 1339–1349.10.1002/(ISSN)1097-0088
- Sungwook, W., J. B. Valdés, S. Steinschneider, and T.-W. Kim. 2016. Non-stationary frequency analysis of extreme precipitation is South Korea using peaks-over-threshold and annual maxima. Stochastic Environmental Research and Risk Assessment 30: 583–606.
- The International Disaster Database – Center for Research on the Epidemiology of Disasters – CRED (EM-DAT). 2016. http://www.emdat.be/.
- Tramblay, Y., L. Neppel, J. Carreau, and K. Najiib. 2013. Non-stationary frequency analysis of heavy rainfall events in southern France. Hydrological Sciences Journal 58 (2): 280–294.10.1080/02626667.2012.754988
- Villarini, G., J. A. Smith, and F. Napolitano. 2010. Nonstationary modeling of a long record of rainfall an temperature over Rome. Advances in Water Resources 33: 1256–1267.10.1016/j.advwatres.2010.03.013
- Villarini, G., J. A. Smith, A. A. Ntelekos, and U. Schwarz. 2011. Annual maximum and peaks- over-threshold analysis of daily rainfall accumulations for Austria. Journal of Geophysical Research 116: D05103. doi:10.1029/2010JD015038.
- Vincent, L. A., and É. Mekis. 2006. Changes in daily and extreme temperature and precipitation indices for Canada over the twentieth century. Atmosphere-Ocean 44 (2): 177–193.10.3137/ao.440205
- Vincent, L. A., and É. Mekis. 2009. Discontinuities in due to joining precipitation station observations in Canada. Journal of Applied Meteorology and Climatology 48: 156–166.10.1175/2008JAMC2031.1
- Vinnikov, K. Y., and A. Robock. 2002. Trends in moments of climate indices. Geophysical Research Letters 29 (2): 743. doi:10.1029/2001GL014025.
- von Storch, V. H. 1995. Misuses of statistical analysis in climate research. In Anlysis of climate variability: Applications of statistical techniques, ed. H. V. von Storch and A. Navarra, 11-26. Berlin: Springer-Verlag.10.1007/978-3-662-03167-4
- Walker, I., R. Sydneysmith, D. Allen, K. Bodtker, D. Bonin, B. Bonsal, S. Cohen, et al. 2008. Chapter 8: British Columbia. In From impacts to adaptation: Canada in a changing climate 2007, eds. D. S. Lemmen, F. J. Warren, J. Lacroix, and E. Bush, 329-386. Ottawa: Government of Canada.
- Whitfield, P. H., R. D. Moore, S. W. Flemming, and A. Zawadzki. 2010. Pacific decadal ocillation and the hydroclimatology of western Canada – review and prospects. Canadian Water Resources Journal. 35 (1): 1–28.10.4296/cwrj3501001
- Yue, S., P. Pilon, and G. Cavadias. 2002. Power of the Mann-Kendall and Spearman’s rho tests for Detecting monotonic trends in hydrological series. Journal of Hydrology 259: 254–271.10.1016/S0022-1694(01)00594-7
- Yue, S., P. Pilon, and B. Phinney. 2003. Canadian streamflow trend detection: Impacts of serial and cross-correlation. Hydrological Sciences Journal 48 (1): 51–63.10.1623/hysj.48.1.51.43478
- Zhang, X., L. A. Vincent, W. D. Hogg, and A. Niitsoo. 2000. Temperature and precipitation trends in Canada during the 20th century. Atmosphere-Ocean 38 (3): 395–429.10.1080/07055900.2000.9649654