Abstract
In recent years, the use of synthetic aperture radar (SAR) for iceberg detection has been increasing thanks to the greater availability and coverage of SAR data, particularly polarimetric data. Greater amounts of polarimetric information can increase detection performance, preventing false alarms and missed detections. However, quad-polarization (quad-pol) SAR systems have increased data rate and power usage requirements, causing quad-pol modes to have generally half the swath width of dual-polarization (dual-pol) modes. Compact polarimetry is a compromise that allows the approximation of quad-pol information (referred to as “pseudo quad-pol”) using a dual-pol SAR. In this paper, using compact polarimetric data simulated from RADARSAT-2 quad-pol imagery, we show how pseudo-quad-pol data can be used for iceberg detection using the likelihood ratio test method. We show that use of the pseudo-quad-pol HV intensity can augment the detection performance of a compact polarimetric SAR system. We also compare the performance of the various compact polarimetric configurations to linearly polarized dual-pol and quad-pol data.
[Traduit par la rédaction] Ces dernières années, le radar à synthèse d'ouverture (RSO) a été de plus en plus utilisé pour la détection des icebergs grâce à une meilleure disponibilité et une meilleure couverture des données RSO, en particulier des données polarimétriques. De plus grandes quantités d'informations polarimétiques peuvent améliorer la performance de détection, ce qui évite les fausses alarmes et les détections manquées. Cependant, les systèmes RSO à quadruple polarisation (quad-pol) exigent des débits binaires et une consommation d’énergie plus élevés, de telle sorte que les modes quad-pol utilisent généralement une largeur de couloir la moitié moindre que celle des modes à double polarisation (dual-pol). La polarimétrie compacte est un compromis qui permet d'approximer l'information quad-pol (alors appelée « pseudo-quad-pol ») au moyen d'un RSO dual-pol. Dans cet article, en utilisant des données de polarimétrie compacte simulées à partir de l'imagerie RADARSAT-2 quad-pol, nous montrons comment les données pseudo-quad-pol peuvent servir à la détection des icebergs au moyen de la méthode du test du rapport des vraisemblances. Nous montrons que l'utilisation de l'intensité HV pseudo-quad-pol peut augmenter la performance de détection d'un système RSO polarimétrique compact. Nous comparons aussi la performance des diverses configurations polarimétriques compactes aux données dual-pol et quad-pol linéairement polarisées.
1 Introduction
Icebergs are masses of freshwater ice that have broken off the edges of glaciers or ice shelves or have been created by larger icebergs breaking up in the ocean.Footnote Although there is a great deal of variety in iceberg shapes and sizes, all icebergs can present potential hazards to shipping and other maritime operations and are, therefore, worth monitoring. The monitoring of icebergs can also be of scientific interest, such as for monitoring the effects of climate change.
Traditionally, icebergs were monitored using field observations from ships or aircraft, but the use of remote sensing for this purpose has become more common as the number of earth-observing satellites has increased. Satellite-borne synthetic aperture radar (SAR), with its wide area coverage and all-weather day and night operation, is a natural choice for iceberg detection.
The first SAR systems only utilized a single polarization of radiation, that is, the transmitted and received polarizations were the same (often this was the horizontal or vertical polarization). In recent years, the development of polarimetric SAR systems has provided a new dimension of information, allowing targets to be characterized based on their polarimetric scattering behaviour rather than their single-channel reflectivity. This additional information has also increased the potential accuracy with which icebergs can be detected and classified. Accuracy is important, because false detections can result in ships being unnecessarily rerouted, causing delays and financial loss, while missed detections can be catastrophic.
Microwave attenuation depths in freshwater ice generally range from 108–510 cm at X-band to 360–1801 cm at S-band (Lewis et al., Citation1987), with scattering caused by both the iceberg's surface as well as by air bubbles and other impurities within the ice. As a result of the relatively extensive microwave attenuation depth, most icebergs display significant volume scattering, at least in the fall and winter months. In summer, when there is likely to be wet snow or liquid water on the top of the iceberg, volume scattering is reduced, while surface scattering from the top of the iceberg is increased (Willis et al., Citation1996). Imaging geometry also plays an important role in the types of microwave scattering observed, for example, tabular icebergs, whose sides are oriented perpendicularly to the sea, have strong double-bounce scattering where radiation is reflected from the iceberg wall and then from the sea (or vice versa) (Willis et al., Citation1996). Because of the wide variety of scattering mechanisms presented by iceberg targets, and the fact that these mechanisms are dependent on the size, dielectric properties, and imaging geometry of the iceberg, no generic model for iceberg backscattering has yet been developed.
Despite this lack of a polarimetric scattering model, polarimetric SAR has shown much promise in the detection of icebergs, with dual-polarization (dual-pol) systems being superior to single-polarization (single-pol) systems (Howell et al., Citation2004, Citation2006, Citation2008). Although fully polarimetric SAR data have been available for several years, to our knowledge there have been no published studies on its use for iceberg detection in the open literature.
a Fully Polarimetric SAR Data
Recall that the polarization of an electromagnetic wave is simply a measurement of the orientation of the oscillation in a wave's electric field and how this orientation changes over time. Fully polarimetric SAR provides the greatest amount of polarization information possible by alternately transmitting two orthogonal polarizations (usually the horizontal and vertical linear polarizations) and receiving two orthogonal polarizations for each transmission, yielding four channels in total (quad-pol). The scattering vector of a traditional quad-pol SAR system can be written as:
The first letter in each subscript represents the transmitted polarization, and the second letter represents the received polarization (e.g., “H” for horizontal and “V” for vertical). The scattering coefficient for each channel, S, is a complex number containing both amplitude and phase information and describes how the incident wave is reflected by the scatterers in a particular resolution cell in a SAR image. With these four channels, we can completely characterize the polarimetric scattering behaviour of a target.
When working with quad-pol data, we often assume reflection symmetry (i.e., S HV = S VH), yielding the three element quad-pol scattering vector:
Unfortunately, for a given swath width, a quad-pol SAR system requires twice the pulse repetition frequency, data rate and power usage of a dual-pol SAR system. This results from the quad-pol system's need to alternate the transmission of two orthogonal polarizations, whereas a dual-pol SAR system transmits only one polarization. This is a particularly important consideration for spaceborne platforms, where the available power and data rate are extremely limited. To keep power usage constant, most spaceborne platforms use quad-pol modes with half the swath width of their dual-pol modes, limiting the available coverage of quad-pol data.
It is extremely important to image as large an area as possible when mapping the locations of icebergs. Because quad-pol modes have half the swath width of dual-pol modes, the Canadian Ice Service does not use quad-pol SAR in their operations.
b Compact Polarity SAR Data
Because dual-pol SAR transmits only one polarization (e.g., either horizontal or vertical) and receives two orthogonal polarizations (e.g., both horizontal and vertical), this results in the following most common scattering vectors:
Envisat also has an “alternating polarization” mode, where the two channels can be HH/HV, VV/VH or HH/VV. However, when operating in the HH/VV mode, the relative HH–VV phase coherence is not preserved (Inglada et al., Citation2004).
While most dual-pol SAR systems used for earth observation transmit a linear polarization, this is not a requirement. A dual-pol SAR system can be built to transmit any polarization, such as the superposition of horizontal and vertical polarizations (i.e., the π/4 mode, named for the angle of its transmitted polarization, as proposed by Souyris et al. (Citation2005), or a circularly polarized wave. For example, Stacy and Preiss (Citation2006) proposed an architecture where circularly polarized waves were transmitted, and left and right circular polarization waves were received. The words “left” and “right” denote the direction of rotation of the polarization vector, with left and right circular polarizations being orthogonal to each other. Raney (Citation2007) proposed the circular transmit, linear receive (CL-pol or CTLR) mode, where a circularly polarized wave is transmitted, and horizontal and vertical polarizations are received. Despite their differences, these three architectures are ways of implementing “compact polarimetry” (CP)—a method of obtaining as much polarimetric scattering information about a target or surface as possible from what is still a dual-pol SAR system (with all the benefits this entails in terms of pulse repetition frequency, data rate and power usage). CP SAR systems have been used in planetary imaging for some time. Most recently, the miniSAR on-board Chandrayaan-1 (Raney et al., Citation2010) collected CP SAR images of our moon (Carter et al., Citation2011).
For the CTLR mode, transmitting right circular polarization, the scattering vector is as follows:
As usual, the “R” denotes that the transmitted polarization is right circular, while “H” and “V” stand for the horizontal and vertical polarizations, respectively. This vector can also be rewritten entirely in the linear (H,V) basis as:
We see that the scattering vector for a CTLR system (or, indeed, any compact polarimetric system) essentially consists of the superposition of co-polarized (HH, VV) and cross-polarized (HV, VH) backscatter.
This is an important result because, by making a number of assumptions, the co-polarized and cross-polarized information can be separated, allowing us to approximate quad-pol information. This process, known as pseudo-quad-pol (or pseudo-quad or PQ) reconstruction, was originally developed by Souyris et al. (Citation2005) and expanded upon by Nord et al. (Citation2009). Nord et al. (Citation2009) showed that, for an airborne L-Band E-SAR scene in the Oberpfaffenhofen area, although certain compact polarimetric modes were superior at reconstructing certain polarimetric parameters, none of the three modes was clearly the best overall in terms of reconstruction accuracy. Our own tests, using fine-quad RADARSAT-2 sample data of the Oberpfaffenhofen area, agreed with these findings.
Therefore, from a reconstruction performance perspective, the three compact polarimetric modes described above are reasonably similar. Nevertheless, the CTLR mode has several advantages over the other two as elucidated by Raney (Citation2007). For example, the Stokes parameters can be easily calculated from CTLR data without the need for any assumptions (Raney, Citation2007), which is not the case for the π/4 and dual-circular modes.
For these reasons, we have chosen to focus on the CTLR mode in this paper. Although the reconstruction process can be applied to the other modes using slightly different equations (Nord et al., Citation2009), the CTLR mode has greater operational potential and has already been shown to be effective at planetary imaging.
Although there are currently no spaceborne CP SAR systems available for earth observation, three are on the horizon: Risat-1 is scheduled for launch in the spring of 2012; the Radarsat Constellation Mission (RCM), a fleet of three SAR systems, is scheduled for launch between 2014 and 2015; and DESDynI (Raney, Citation2009) is currently scheduled for launch sometime in 2016. Even in the absence of CP data, several earth observation studies have been published over the last six years using CP data that have been simulated from quad-pol data (Souyris et al., Citation2005; Raney, Citation2006). The majority of applications of CP SAR data have been terrestrial including crop classification (Souyris et al., Citation2005; Ainsworth et al., Citation2009), soil moisture estimation (Truong-Loi et al., Citation2009), vegetation characterization (Angelliaume et al., Citation2007; Lardeux et al., Citation2011) and land cover mapping (Ainsworth et al., Citation2009; Nord et al., Citation2009).
Recently Yin et al. (Citation2011) and Shirvany et al. (Citation2012) reported the first applications of CP to ship detection. Yin et al. refined the Souyris reconstruction algorithm for complex targets (ships) by adding a helix scattering component to the covariance matrix model and changing the volume scattering component. They tested this reconstruction algorithm by comparing the ship detection performance of the original quad-pol data, PQ data using both the original Souyris algorithm and their own algorithm, and the raw CTLR dual-pol data. Shirvany et al. used the degree of polarization, a parameter derived from the Stokes vector, to detect ships. Their results, although encouraging, were purely qualitative. In addition to these two studies, Liu et al. (Citation2010), in an internal technical report, reported ship detection performance results comparing quad-pol, |HH–HV|, H–H and CTLR for ten ships in a single RADARSAT-2 fine-quad (FQ4) scene.
As part of an ongoing effort to explore the use of CP data in maritime surveillance, in this study we report on the application of CP data to iceberg detection. We have previously reported the application of CP data to ship detection (Atteia and Collins, unpublished manuscript). Some adjustments to the reconstruction process were required to produce useful results for ocean imagery. We use RADARSAT-2 fine-quad scenes to simulate compact polarimetric data in the CTLR mode. We then perform pseudo-quad reconstruction on this simulated data. We use this pseudo-quad-pol information with the likelihood ratio test detection method (Liu and Meek, Citation2005; Liu et al., Citation2010) to calculate a decision variable with which iceberg targets in a scene can be detected. Using a number of validated iceberg locations from in-field observations, we finally compare the detection performance using pseudo-quad and CTLR data against traditional dual-pol and quad-pol configurations for 25 icebergs spread across 12 RADARSAT-2 scenes.
2 Methods
a Data
A summary of the RADARSAT-2 scenes used in this study is shown in , sorted by fine-quad beam position. The scenes are located around 70°–71°N, 58°–60°W. Scenes will be referred to in this paper based on the Scene ID. The “Beam” column in the table lists the fine-quad beam position, with lower positions having lower incidence angles, and vice versa. The “W.S. (m s−1)” column lists the mean wind speed of each scene calculated using the mean value of the HV σ
0, according to the equation developed by Vachon and Wolfe (Citation2011). A value of <3 in the table denotes that the wind speed was too low to estimate accurately, and we can only state that it is less than 3 m s−1. “
” is the mean value of the constant of proportionality in each scene and is described in the following section detailing the pseudo-quad reconstruction process. While not all of the scenes contained validated icebergs, all were nevertheless used in order to better model the behaviour of N at certain incidence angles.
Table 1. An overview of the RADARSAT-2 fine-quad scenes used in our study. Beam refers to the fine quad beam position, W.S. stands for estimated wind speed for the scene and
N
is an estimated reconstruction parameter (see Section 2b).
All scenes have a range sample spacing of 4.73 m, with azimuth sample spacing varying from 4.8–5.0 m according to beam position. Nominal RADARSAT-2 fine quad-pol resolution is approximately 5.2 m in range by 7.6 m in azimuth.
b Pseudo-Quad-Pol Reconstruction
The reconstruction of the quad-pol covariance matrix from simulated CTLR data is a two step process: (i) first simulate the CTLR from linearly polarized quad-pol data and (ii) then reconstruct elements of the quad-pol covariance matrix. The algorithms we used are described in detail in a companion paper (Collins et al., Citation2012). Here we briefly summarize the process.
From the CTLR scattering vector in Eq. (6), we can write the CTLR covariance matrix as a sum of three components,
This assumption simplifies the PQ covariance matrix, by removing the third component, reducing the number of unknowns to five: |S
HH
|
2, |S
HV
|
2, |S
VV
|
2, and the real and imaginary parts of .
To further constrain the solution, Souyris developed a general relation between the linear HH–VV coherence and the cross-polarization ratio (Souyris et al., Citation2005),
The angle brackets represent spatial averaging and ρ is the HH–VV coherence, defined as
In this paper, we use a 5 × 5 pixel window for the necessary spatial averaging. Larger averaging windows will generally reduce the effects of reflection asymmetry and, therefore, improve the accuracy of the reconstruction, up to a certain point. However, larger windows will also reduce the resolution of the imagery. Because our application is iceberg detection, we have chosen a relatively small averaging window in order to preserve the resolution of the imagery.
Equations (9) and (10) are iteratively solved to estimate |S
HV
|
2 and the linear coherence (ρ) (see Collins et al., (Citation2012) for details). The intensities of the other pseudo-co-pol components (S
HH, S
VV) and their relative phase (
) are estimated directly from the covariance matrix elements of the CP mode and the estimated |S
HV
|
2 value as follows,
Thus, the covariance matrix of the pseudo-quad-pol data derived from the CTLR CP mode can be written as,
The variable N in Eq. (9) is a crucial parameter in reconstructing the quad-pol covariance matrix. It depends mainly on the dominant scattering mechanism of the area under study. Souyris et al. (Citation2005) used a constant value of 4 for N based on the assumption that volume scattering was the dominant scattering mechanism. Nord et al. (Citation2009) defined a model for N as the ratio of the double-bounce backscatter to the cross-pol backscatter,
In their algorithm, N is estimated with a sliding window that compensates for the varying strength of double-bounce backscatter to accommodate scattering from urban areas. In our study the objective is to detect icebergs in a background of ocean “clutter,” with little or no land. It is well known that backscatter from the ocean is well modelled by single bounce scattering, so neither the original reconstruction algorithm proposed by Souyris et al. (Citation2005), which assumes volume scattering, nor the modified algorithm proposed by Nord et al. (Citation2009), which compensates for the double bounce scattering, are consistent with C-band microwave backscattering from the ocean. We have developed an empirical model for N that retains the basic logic behind the original Souyris algorithm, but estimates N directly from the data (Collins et al., Citation2012). We found that there is a strong and robust relationship between the mean N (
) for ocean RADARSAT-2 FQ scenes and the incidence angle of the scene. We also found a weak dependence on wind speed, but it is not possible to estimate wind speed independently at the spatial scale of the SAR data, so we have not pursued it.
Using all of our RADARSAT-2 scenes, we calculated the regression N = 300900 θ −2.936, shown in , where θ is the mean incidence angle of the scene (in degrees). This regression model had an r 2 of 0.935. To avoid bias as a result of using the same data for both modelling and testing, we also calculated a regression using only half our scenes, yielding the equation N = 226700 θ −2.864, with an r 2 of 0.907. This slightly weaker regression was used for the results in this paper. The effect of N on target detection performance will be discussed in the results section.
Fig. 1 Mean N value versus mean incidence angle for each scene. The red line shows the regression y = 300900x −2.936 , with r2 of 0.935.
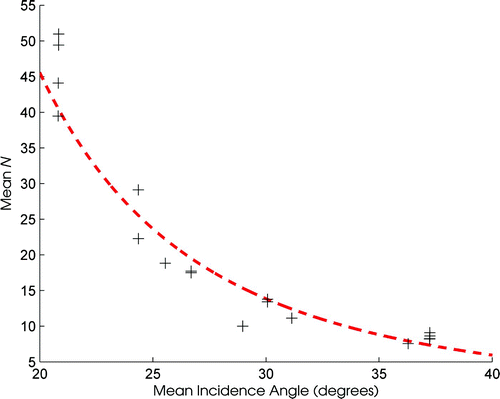
Thus, in our work we estimate N from our model and follow the reconstruction algorithm outlined above.
We should note that for some pixels, generally those with N values significantly greater than the value we had selected, the magnitude of our ρ estimate would converge to a value greater than one. Since such a value is impossible, it represents a failure of the reconstruction algorithm to model the behaviour of the data. For these pixels, we set |S HV | 2 to zero, stop iterating, then calculate the pseudo-quad covariance matrix as normal (i.e., |S HH | 2 = C 11, and so on). These pixels with abnormally high N values were mostly ocean pixels rather than iceberg pixels, so this actually worked to our advantage, because it allowed us to set some of the pseudo-HV ocean clutter to zero. This should enhance the detection performance.
c Likelihood Ratio Test Detection
To perform the target detection, we used the likelihood ratio test method as described by Liu et al. (Citation2010) and Liu and Meek (Citation2005) to combine the different polarimetric channels into a single decision variable using the Neyman-Pearson criteria. We can quantify the detection performance at a particular threshold (i.e., pixels with a decision variable above the threshold are considered targets) by calculating the probability of missed detection and the probability of false alarm for that decision variable value. Once the polarimetric data have been combined into a single decision variable in this fashion, they can be used as input to a constant false alarm rate (CFAR) target detector, or other target detection algorithm.
In general, the decision variable for a multiple channel, coherent system can be calculated as in Liu et al. (Citation2010) and Liu and Meek (Citation2005) using the equation,
For a non-coherent system (i.e., amplitude only, without meaningful phase information), the decision variable is calculated by dividing the intensity of each channel by the mean intensity of the ocean pixels for that channel. For example, for a system with non-coherent HH and HV channels, we can calculate the decision variable using the equation:
SAR systems are generally either coherent or non-coherent in their entirety (i.e., if one channel has phase information, so do the others). This is not the case with our pseudo-quad-pol data. We have the HH–VV phase, but no HV phase information. We thus decided to calculate the decision variable for the pseudo-quad-pol data as follows:
Note that k in Eq. 18 does not necessarily need to contain the pseudo-HH–VV information. We also tested the detection performance using a scattering vector of the RH and RV channels (the channels directly measured by a CTLR SAR) alongside the pseudo-HV.
Since the pseudo-quad-pol reconstruction process uses a covariance matrix as input (which has been spatially averaged) rather than the original quad-pol scattering vector, the pseudo-quad-pol data are also spatially averaged by extension. When we failed to account for this during the detection process, the pseudo-quad-pol data outperformed all other modes. Spatial averaging of a dataset's Hermitian products (such as when we average the CTLR covariance matrix at the beginning of the reconstruction process) improves detection performance, with the obvious tradeoff being that the resolution of the imagery is degraded.
In order to spatially average our non-pseudo-data in a similar fashion to the pseudo-quad-pol data, see that Eq. (16) can be written as follows (for an n channel system):
By writing the equation in this form, it becomes clear that we can spatially average the Hermitian products of the data during the decision variable calculation as follows (where angle brackets denote spatial averaging, for an n channel system):
For example, for a two channel system:
We cannot just spatially average the scattering vector k, because that is a fundamentally different operation than averaging the Hermitian products. Performing the necessary averaging for non-coherent data is easy by comparison—just spatially average the intensity.
In this paper, the detection results for the non-pseudo-data use a spatially averaged decision variable as in Eq. (20). We found that this gives the fairest comparison between the pseudo-quad-pol data and the other modes. While the results in this paper were obtained using a 5 × 5 window, we also tested window sizes of 3 × 3 and 5 × 7 (azimuth × range, to produce roughly square pixels). This certainly had an effect on the missed detection probability in an absolute sense (generally, more averaging resulted in lower missed detection probabilities on the receiving operating characteristic (ROC) curves) but had little effect on the relative performance of the different polarimetric modes with respect to each other.
d LRT Detection Performance
The detection performance of a likelihood ratio test (LRT) detector may be characterized with a plot of the ROC (Scharf, Citation1990; Liu and Meek, Citation2005; Liu et al., Citation2005). The ROC curve is generated by plotting the probability of missed detection (PMD) versus the probability of false alarm (PFA) at various threshold values (Scharf, Citation1990). PMD and PFA are calculated as follows,
To compute the PFA for each system, a 1000 × 1000 pixel region of the ocean with no targets is selected. The decision variables for each pixel in the selected ocean-only region are calculated using ocean statistics. These decision variables are compared with a set of thresholds. For each threshold, if the decision variable is larger than the threshold, the pixel is considered a false alarm. To form the PFA at each threshold, the number of false alarms is divided by the total number of selected ocean pixels.
For the same set of thresholds, the probability of detection (PD) values are computed as follows: first, we manually detected icebergs using the FQ HV channel and manually created a mask for that iceberg. Then the decision variable for each iceberg pixel within the mask is recalculated using the ocean statistics. When the decision variable is higher than the threshold, the pixel is considered a detected pixel. The PD is computed by dividing the detected pixels by the total number of iceberg pixels and the PMD is calculated using Eq. (22). The ROC curve is plotted for each system using the (PFA, PMD) pairs.
Because the iceberg masks were created manually, they are ultimately subjective. Also, because the size of the mask determines the probability of missed detection in an absolute sense, these probabilities are ultimately subjective. However, we experimented with different mask shapes and sizes, and although the mask changed the absolute probabilities, it had little effect on the ranking of the probabilities of the various polarimetric modes (i.e., regardless of how the mask was drawn, certain polarimetric modes consistently detected more pixels of each mask, and the trends of the ROC plots were largely unchanged).
Because the masks were created from the original RADARSAT-2 fine-quad imagery, we had to take into account the fact that the data had been spatially averaged. To do this, we ran a window over the iceberg masks that was the same size as the spatial averaging window. Wherever the centre pixel of the window was part of the iceberg mask, the mask was expanded to all pixels inside the window.
e Experimental Methods
After simulating CTLR data from the observed FQ data, we reconstructed the quad-pol data as described in Section 2b using the appropriate value of N from the N versus incidence angle model.
We obtained 25 validated iceberg locations based on field observations collected from a ship. The validation data contained the iceberg's number, location (in latitude and longitude), time sighted, type (blocky, dome, tabular, etc.), and size (small, medium and large), with which we were able to identify each iceberg in the corresponding fine-quad RADARSAT-2 scene. Unfortunately, we are not able to share iceberg details in this paper because of copyright issues.
These icebergs are used to study the LRT detection performance of several quad-pol, dual-pol and single-pol SAR systems as a function of incidence angle. We compare the performance of observed quad-pol, dual-pol and single-pol systems to the simulated CTLR system and to several reconstructed quad-pol, dual-pol and single channel systems. The following eight systems are compared,
Observed FQ: | = |
quad-pol; coherent HH/HV, coherent VV/VH, and amplitude-only HH/VV |
Simulated CTLR: | = |
coherent CTLR |
Reconstructed PQ: | = |
full-PQ, PQ HV, and a combination of coherent CTLR and PQ HV |
Our research on ship detection with PQ data showed that incidence angle had a strong effect on detection performance (Atteia and Collins, unpublished manuscript). To explore the effect of incidence angle in the context of iceberg detection, we subdivided the RADARSAT-2 scenes into four categories:
1. | FQ2: 19.9°–21.8° (1.9°), | ||||
2. | FQ5/6/7: 23.4°–27.6° (4.2), | ||||
3. | FQ9/10/11: 28.0°–32.0° (4.0°), and | ||||
4. | FQ16: 36.5°–38.0° (1.5°). |
The angles in parentheses are the incidence angle ranges of the category. Because there is insufficient space to report and discuss 25 ROC curves, we summarize the performance results by reporting the PMD for a fixed PFA of 10−6 for each iceberg. To explore the effect of incidence angle, we also report the median PMD for each incidence angle category. We also provide median ROC curves for each incidence angle category to illustrate performance as a function of both PMD and PFA. We use the median because the sample sizes are small, and the median is less affected by outliers.
3 Results
The summarized detection performance results are presented in . The detectors (columns) have been organized into three sets of columns corresponding to FQ quad-pol, FQ dual-pol and CP systems. The table is also organized into four sections according to incidence angle, from the steepest incidence angle category (FQ2: 19.9°–21.8°) at the top to the shallowest category (FQ16: 36.5°–38.0°) at the bottom. Each beam section contains icebergs identified in the scenes in that category. Within each category, the rows refer to icebergs denoted by a letter. For icebergs that have been sighted in more than one scene, we have included a number to indicate the sighting. The bottom row of each incidence angle category is the median detector result over all icebergs in that category and the bottommost row in the table is the median detector performance over all 25 icebergs in our study. The grey shaded entries are discussed below.
Table 2. Probabilities of missed detection for icebergs at a fixed probability of false alarm equal (PFA) of 10−6. Iceberg IDs are letters. When an iceberg has been observed in more than one scene, it also has a number to differentiate the sightings. The lowest PMD is shaded a dark grey. In cases where the lowest PMD was achieved with the quad-pol data, the next lowest non-quad-pol detector is also shaded with dark grey. Detectors whose PMD is within 0.05 of the lowest PMD are shaded with lighter grey.
The first observation is that all CP detectors perform very poorly at the steepest incidence angle. Many of the results are 1.0, meaning that the detector failed to detect a single pixel in the iceberg mask. We found that the accuracy of the reconstruction was strongly dependent on incidence angle and that the uncertainties in the reconstructed HV values were very high (Collins et al., Citation2012), thus the poor performance at this steep angle is not entirely surprising.
For the three other incidence angle groups the performance of the CP detectors improve significantly. For the icebergs in FQ5/6/7, the PQ HV detector, full-PQ detector and the CLTR-PQHV detector outperform the linear dual-pol detectors, although for many icebergs, the performance of the linear dual-pol detectors is comparable (i.e., within 0.05). The CTLR-PQHV detector has the highest median performance for this range of incidence angles with the PQHV single-channel detectors and the two linear dual-pol detectors having comparable performance.
For the icebergs at incidence angles greater than 28°, the PQ HV detector outperforms all other detectors with two exceptions. For iceberg J, the CTLR and FQ HH/VV have the highest performance and for iceberg F, the CTLR/PQHV has a slightly higher performance. However, the median performance of the PQHV single channel detector for the two shallow incidence angles is comparable to the FQ quad-pol detector. The PMD of the PQHV detector is nearly half that of the FQ dual-pol detectors.
Finally, the PQHV detector has the strongest overall performance with the CTLR/PQHV close behind. Both these detectors have an overall performance that is comparable to the FQ quad-pol detector and have PMD values nearly half that of the FQ linear quad-pol detector. This result is consistent with our ship detection research which also showed the PQHV detector to be a stronger performer than any linear dual-pol detector and comparable to quad-pol at incidence angles greater than 28°.
The median ROC curves for each incidence angle category are shown in . These confirm the poor performance of the CP detectors at the steep FQ2 angle and performance of the PQHV and CTLR/PQHV at 23.4°–27.6° that is comparable to HH/HV and VV/HV. At the two shallower angles, these two CP detectors are quite strong across all PFA values.
4 Conclusions
Our study provides the first analysis of iceberg detection performance with compact polarimetric SAR. We have provided a comparison of iceberg detection performance between quad-pol data, several linear dual-pol combinations that have been reported in the literature, and CTLR compact polarimetry data––both the raw coherent dual-pol CTLR data, reconstructed PQ data as well as a combination of CTLR and PQHV.
Our results show that the performance of the compact polarimetry detection is a strong function of incidence angle. At the steepest FQ2 angle, the performance is quite weak. Between 23° and 28° the CTLR/PQHV detector had the strongest performance although it was comparable to PQHV and two linear dual-pol detectors. However, at angles greater than 28°, the median performance of the PQHV and CTLR/PQHV was greater than any dual-pol detectors and comparable to quad-pol.
The overall performance of PQHV data and the combination of CTLR and PQHV was greater than all linear dual-pol modes and comparable to quad-pol data. Improvements to the reconstruction process, as well as to our model of N, are likely to improve the detection results further.
Thus, our results suggest that compact polarity data offer great potential for iceberg detection applications. The results reported here are limited to the relatively fine spatial resolution (5 m range by 8 m azimuth) of the RADARSAT-2 fine-quad data. We are currently exploring maritime surveillance with CTLR data simulated using lower resolution beam modes from the upcoming RCM mission and with Stokes vector parameters derived from these data.
Acknowledgements
Paris Vachon of Defence Research and Development Canada (DRDC) provided funding and helped make the data accessible. Desmond Power of C-CORE provided the field observations of icebergs. Chen Liu, also of DRDC, helped us implement the LRT. We also acknowledge François Charbonneau, of the Canada Centre for Remote Sensing, for leading the RCM compact polarimetry project sponsored by the Canadian Space Agency. All RADARSAT-2 data were provided through this project. We further acknowledge funding support from the Natural Sciences and Engineering Research Council (NSERC) of Canada. All RADARSAT-2 data copyright 2009 MacDonald Dettwiler and Associates. This work was completed while MJC was on sabbatical at the Jet Propulsion Lab in Pasadena CA. We acknowledge Ben Holt of JPL for facilitating this.
Notes
This work was completed while MJC was a visiting research engineer at the Jet Propulsion Laboratory in Pasadena CA USA.
References
- Ainsworth , T. , Kelly , J. and Lee , J.-S. 2009 . Classification comparisons between dual-pol, compact polarimetric and quad-pol sar imagery . ISPRS J. Photogramm. Remote Sens. , 64 : 464 – 471 . (doi:10.1016/j.isprsjprs.2008.12.008)
- Angelliaume, S.; P. Dubois-Fernandez and J.-C. Souyris. 2007. Compact polinSAR for vegetation characterisation. In Proc. IEEE International Geoscience and Remote Sensing Symposium (IGARSS) (pp. 1136–1138). IEEE.
- Carter , L. , Campbell , D. and Campbell , B. 2011 . Geologic studies of planetary surfaces using radar polarimetric imaging . Proc. IEEE , 99 ( 5 ) : 770 – 782 . (doi:10.1109/JPROC.2010.2099090)
- Collins , M. , Denbina , M. and Atteia , G. 2012 . On the reconstruction of quad-pol SAR data from compact polarimetry data for ocean target detection . IEEE Trans. Geosci. Remote Sens , 99 doi:10.1109/TGRS.2012.2199760
- Howell, C.; J. Youden, K. Lane, D. Power, C. Randell and D. Flett. 2004. Iceberg and ship discrimination with ENVISAT multi-polarization ASAR. In Proc. IEEE International Geoscience and Remote Sensing Symposium (IGARSS) (Vol. 1, pp. 113–116). IEEE.
- Howell, C.; J. Mills, D. Power, J. Youden, K. Dodge, C. Randell and D. Flett. 2006. A multivariate approach to iceberg and ship classification in HH/HV ASAR data. In Proc. IEEE International Geoscience and Remote Sensing Symposium (IGARSS) (pp. 3583–3586). IEEE.
- Howell, C.; D. Power, M. Lynch, K. Dodge, P. Bobby, C. Randell, P. Vachon and G. Staples. 2008. Dual polarization detection of ships and icebergs – recent results with ENVISAT ASAR and data stimulations of RADARSAT-2. In Proc. IEEE International Geoscience and Remote Sensing Symposium (IGARSS) (pp. 206–209). IEEE.
- Inglada, J.; C. Henry and J.-C. Souyris. 2004. ASAR multi-polarization images phase difference: Assessment in the framework of persistent scatterers interferometry. In Proceedings of the Envisat & ERS symposium.
- Lardeux , C. , Frison , P.-L. , Tison , C. , Souyris , J.-C. , Stoll , B. , Fruneau , B. and Rudant , J.-P. 2011 . Classification of tropical vegetation using multifrequency partial SAR polarimetry . IEEE Geosci. Remote Sens. Lett. , 8 ( 1 ) : 133 – 137 . (doi:10.1109/LGRS.2010.2053836)
- Lewis , E. , Currie , B. and Haykin , S. 1987 . Detection and classification of ice , Letchworth , , UK : Research Studies Press Ltd .
- Liu, C. and A. Meek. 2005. Likelihood ratio test polarimetric SAR ship detection application. (Defence Research and Development Canada, Technical Memorandum, TM 2005-243), Ottawa, ON.
- Liu , C. , Vachon , P. W. and Geling , G. W. 2005 . Improved ship detection with airborne polarimetric SAR data . Can. J. Remote Sens , 31 ( 1 ) : 122 – 131 . (doi:10.5589/m04-056)
- Liu, C.; P.W. Vachon, R.A. English and N. Sandirasegaram. 2010. Ship detection using RADARSAT-2 fine quad mode and simulated compact polarimetry data. (Defence Research and Development Canada, Technical Memorandum, TM 2009-285), Ottawa, ON.
- Nord , M. , Ainsworth , T. , Lee , J.-S. and Stacy , N. 2009 . Comparison of compact polarimetric synthetic aperture radar modes . IEEE Trans. Geosci. Remote Sens. , 47 ( 1 ) : 174 – 188 . (doi:10.1109/TGRS.2008.2000925)
- Raney , R. 2006 . Dual-polarized SAR and Stokes parameters . IEEE Geosci. Remote Sens. Lett. , 3 ( 3 ) : 317 – 319 . (doi:10.1109/LGRS.2006.871746)
- Raney , R. 2007 . Hybrid-polarity SAR architecture . IEEE Trans. Geosci. Remote Sens. , 45 ( 11 ) : 3397 – 3404 . (doi:10.1109/TGRS.2007.895883)
- Raney, R. 2009. Desdyni adopts hybrid polarity sar architecture. In IEEE radar conference – radar09 (pp. 1–4).
- Raney , R. , Spudis , P. , Bussey , B. , Crusan , J. , Jensen , J. , Marinelli , W. , McKerracher , P. , Neish , C. , Palsetia , M. , Schulze , R. , Sequeira , H. and Winters , H. 2010 . The lunar mini-rf radars: Hybrid polarimetric architecture and initial results . Proc. IEEE , 99 ( 5 ) : 808 – 823 . (doi:10.1109/JPROC.2010.2084970)
- Scharf , L. 1990 . Statistical signal processing: Detection, estimation, and time series analysis , Reading , MA : Prentice Hall .
- Shirvany , R. , Chabert , M. and Tourneret , J.-Y. 2012 . Ship and oil-spill detection using the degree of polarization in linear and hybrid/compact dual-pol sar . IEEE J. Sel. Top. Appl. Earth Obs. Remote Sens. , 5 ( 3 ) : 885 – 892 . (doi:10.1109/JSTARS.2012.2182760)
- Souyris , J.-C. , Imbo , P. , Fjørtoft , R. F. , Mingot , S. and Lee , J.-S. 2005 . Compact polarimetry based on symmetry properties of geophysical media: The π/4 mode . IEEE Trans. Geosci. Remote Sens. , 43 ( 3 ) : 634 – 646 . (doi:10.1109/TGRS.2004.842486)
- Stacy, N. and M. Preiss. 2006. Compact polarimetric analysis of X-band SAR data. In Proc. European conference on synthetic aperture radar (EUSAR).
- Truong-Loi , M.-L. , Freeman , A. , Dubois-Fernandez , P. and Pottier , E. 2009 . Estimation of soil moisture and Faraday rotation from bare surfaces using compact polarimetry . IEEE Trans. Geosci. Remote Sens. , 47 ( 11 ) : 3608 – 3615 . (doi:10.1109/TGRS.2009.2031428)
- Vachon , P. and Wolfe , J. 2011 . C-band cross-polarization wind speed retrieval . IEEE Geosci. Remote Sens. Lett. , 8 ( 3 ) : 456 – 459 . (doi:10.1109/LGRS.2010.2085417)
- Willis , C. , Macklin , J. , Partington , K. , Teleki , K. , Rees , W. and Williams , R. 1996 . Iceberg detection using ERS-1 synthetic aperture radar . Int. J. Remote Sens. , 17 ( 9 ) : 1777 – 1795 . (doi:10.1080/01431169608948739)
- Yin, J.; J. Yang and X. Zhang. 2011. On the ship detection performance with compact polarimetry. In Proceedings of the IEEE radar conference (radar) (pp. 675–680).