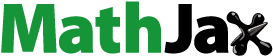
ABSTRACT
Global cloud resolving models (GCRMs) are a new type of general circulation model that explicitly calculates the growth of cloud systems with fine spatial resolutions and more than 10 GCRMs have been developed at present. This work reviews cloud microphysics schemes used in GCRMs with introductions to the recent progress and researches with GCRMs. Especially, research progress using a pioneer of GCRMs, Nonhydrostatic ICosahedral Atmospheric Model (NICAM), is focused. Since GCRMs deal with climatology and meteorology, it is a challenging issue to establish cloud microphysics schemes for GCRMs. A brief history of the development of cloud microphysics schemes and cloud-radiation coupling in NICAM is described. In addition, current progress in analytical techniques using satellite simulators is described. The combined use of multi-optical sensors enables us to constrain uncertain processes in cloud microphysics without artificial tuning. As a result, cloud microphysics schemes used in the NICAM naturally represent cloud systems, and hence, the radiative budget is well balanced with little optimization. Finally, a new satellite and a ground validation campaign are introduced for future work.
RÉSUMÉ
[Traduit par la rédaction] Les modèles globaux de résolution des nuages (Global cloud resolving models ou GCRM) sont un nouveau type de modèle de circulation générale qui calcule explicitement la croissance des systèmes nuageux avec de fines résolutions spatiales. Plus de dix GCRM ont été créés à ce jour. Le présent travail passe en revue les schémas de microphysique des nuages utilisés dans les GCRM avec des introductions aux progrès récents et aux recherches sur les GCRM. Les progrès de la recherche utilisant un pionnier des GCRM, le modèle atmosphérique non hydrostatique ICosahedral (NICAM), sont particulièrement ciblés. Puisque les GCRM traitent de la climatologie et de la météorologie, l’établissement de schémas de microphysique des nuages pour les GCRM est un défi. Un bref historique de l’élaboration des schémas de microphysique des nuages et du couplage nuage-radiation dans NICAM est décrit. En outre, les progrès actuels des techniques analytiques utilisant des simulateurs de satellites sont décrits. L’emploi combiné de capteurs multi-optiques nous permet de contraindre les processus incertains de la microphysique des nuages sans réglage artificiel. Par conséquent, les schémas de microphysique des nuages utilisés dans le NICAM représentent naturellement les systèmes nuageux, et donc, le bilan radiatif est bien équilibré avec peu d’optimisation. Enfin, un nouveau satellite et une campagne de validation au sol sont présentés pour les travaux futurs.
1 Introduction
This work reviews cloud microphysics schemes used in global cloud resolving models (GCRMs) with introductions to the recent progress and researches with GCRMs. Section 1 briefly introduces the background and design of GCRMs. Cloud microphysics schemes in GCRMs are reviewed in Section 2 and model evaluation using optical sensors are reviewed in Section 3. Especially, research progress using a pioneer of GCRMs, Nonhydrostatic ICosahedral Atmospheric Model (NICAM), is focused. Finally, Section 4 summarizes this review. Acronyms of numerical models, satellites, and optical instruments are described in .
Table 1. List of acronyms of models, satellites, and instruments.
a Global cloud resolving models
Climatology and meteorology have separated spatiotemporal scales and hence have been individually investigated in separate research communities (see ). However, ongoing climate change increases social risks such as record breaking intense precipitation, intense tropical cyclones, and extensive flood damage. Recent extreme events have been intensively analysed around the world and have been edited as a special issue for “Explaining Extreme Events from a Climate Perspective” for the Bulletin of the American Meteorological Society every year starting in 2011 (e.g. Herring et al., Citation2019). To meet social demands, the World Climate Research Programme (WCRP) has promoted research on “weather and climate extremes” as one of the current grand challenges (https://www.wcrp-climate.org/grand-challenges/grand-challenges-overview). The two research communities have started to work together to tackle this issue across spatiotemporal scales.
Fig. 1 Typical spatiotemporal scales of some examples of weather and climate phenomena. The numerical weather prediction model (NWP), general circulation model (GCM), and global cloud resolving model (GCRM) cover different but partially overlapping scales.
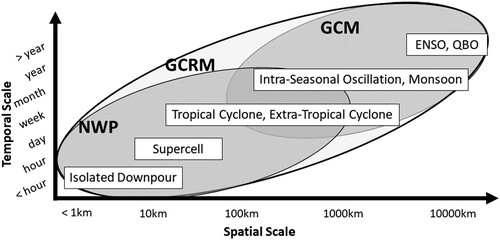
For the next decade, the WCRP has newly proposed lighthouse activities (https://www.wcrp-climate.org/wcrp-ip-la), in which a “digital twin of Earth” is to be utilized for modeling earth systems more realistically (e.g. Bauer et al., Citation2021). Recent advances in parallel computing have enabled us to achieve global atmospheric simulations with finer horizontal resolution (e.g. Satoh et al., Citation2017; Schär et al., Citation2020; Wedi, Citation2014). Thus, motivation and research infrastructure are now prepared for global cloud resolving simulations that fill the scale gap between climate research and weather forecasting.
In the UK, for example, the UPSCALE (UK on PRACE: weather-resolving Simulations of Climate for globAL Environmental risk) project was organized for global weather prediction using a general circulation model (GCM) with a horizontal resolution of up to 25 km (Mizielinski et al., Citation2014). In Europe, multi-high-resolution GCMs have been used for predicting regional climate as the PRocess-based climate sIMulation: AdVances in high-resolution modelling and European climate Risk Assessment (PRIMAVERA) project (https://www.primavera-h2020.eu/). In the Pan-Pacific region, the International laboratory for High-resolution Earth System Prediction (iHESP) project has started to examine intense cyclones for decadal prediction by using a 25-km atmosphere and 10-km ocean-coupled GCM (Zhang et al., Citation2020a; Chang et al., Citation2020). Finally, the High Resolution Model Intercomparison Project (HighResMIP) was coordinated as a part of the Coupled Model Intercomparison Project Phase 6 (CMIP6) for examining the capability of capturing mesoscale phenomena using GCMs only by increasing the horizontal resolution up to 25 km (Haarsma et al., Citation2016; Roberts et al., Citation2018). However, in HighResMIP, most participants are conventional GCMs, which do not predict precipitating hydrometeors (e.g. rain, snow, graupel, and hail) [cloud microphysics in CMIP6 models are described in the ES-DOC Explorer (https://explore.es-doc.org/)] and rely on parameterizations for representing convective clouds, although some GCMs have recently incorporated explicit calculations for rain and snow [ARPEGE-Climat (Roehrig et al., Citation2020), CAM (Gettelman & Morrison, Citation2015; Morrison & Gettelman, Citation2008; Song et al., Citation2012), ECHAM/ICON-A (Posselt & Lohmann, Citation2008; Sant et al., Citation2015), IFS (Forbes et al., Citation2011), MIROC (Michibata et al., Citation2019), and UM-Global Atmosphere (Boutle et al., Citation2014; Walters et al., Citation2019)]. Explicit (grid-scale) representations of convective clouds are necessary for seamlessly simulating the interaction between a mesoscale convective system and its environmental state at finer resolutions (e.g. Miyakawa et al., Citation2012; Takasuka et al., Citation2015). Climate models without convective parameterizations can successfully better represent some aspects of climate states than those with convective parameterizations by increasing horizontal resolution even if the climate models cannot fully resolve convective clouds (e.g. Senf et al., Citation2020; Stevens et al., Citation2020; Vergara-Temprado et al., Citation2020; Wedi et al., Citation2020).
In the era of global simulations with horizontal resolutions of a few kilometers, nonhydrostatic dynamical cores (e.g. Saito et al., Citation2007) are required for resolving convection in an environmental state (e.g. Kato & Saito, Citation1995; Weisman et al., Citation1997; Yang et al., Citation2017). In addition, cloud microphysics for precipitating hydrometeors should be explicitly calculated as is done in mesoscale models. Global atmospheric models that explicitly calculate the growth of cloud systems are a new type of GCM and are now called global cloud resolving models (Satoh et al., Citation2019). In particular, explicit representation of smaller clouds is a great advantage of GCRMs compared to conventional GCMs even if the cloud microphysics in GCMs become sophisticated (details are described in Section 3.2). Global cloud resolving models are now practically available for various research fields in many countries thanks to prevailing massive parallel computers and advanced network environments. For example, Nakano et al. (Citation2017) demonstrated that forecasts of tropical cyclones generally improve by using three GCRMs with the horizontal resolution of less than 10 km. In contrast to operational global numerical weather prediction models (e.g. Kalnay et al., Citation1990; Zhang et al., Citation2019a), GCRMs are not restricted by operating time and hence can be used for challenging issues in terms of spatiotemporal resolution and complexity of physics. Currently, the first international intercomparison project for GCRMs, the initiative DYnamics of the Atmospheric general circulation Modeled On Non-hydrostatic Domains (DYAMOND), has been organized (Stevens et al., Citation2019) and GCRMs are becoming established (See ). summarizes the GCRMs that have been developed at present. Note that multimodel framework (MMF) is another approach to modeling convective cloud systems in GCMs (e.g. Tao et al., Citation2009).
Fig. 2 Cloud images calculated by the GCRMs in the DYAMOND project and from the geostationary satellite Himawari-8 (After in Stevens et al., Citation2019). From left to right: IFS with a horizontal resolution of 4, 9 km, and NICAM (top row); ARPEGE-NH, Himawari-8, and ICON (second row); FV3, GEOS5, and UM (third row); and SAM and MPAS (bottom row).
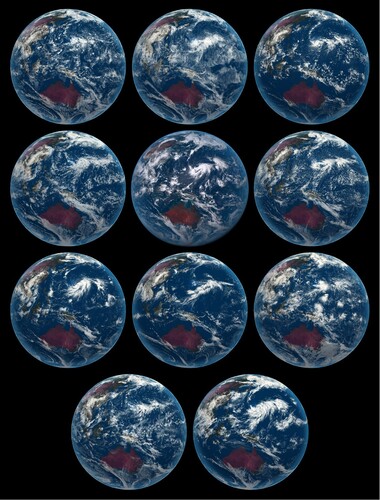
Table 2. List of GCRMs. Short names and references to the model-frameworks and recent settings are summarized. Cloud microphysics schemes in the DYAMOND GCRMs refer to the schemes used for the DYAMOND project. The subscripts v, c, r, i, s, and g indicate vapor, cloud water, rain, cloud ice, snow, and graupel categories, respectively.
b A baseline for spatial resolution
Cloud microphysics schemes for GCRMs are designed to work with horizontal resolutions from 1 to 15 km based on 15 years of experience with global high-resolution simulations using the NICAM. Similarly, a common cloud microphysics scheme is used in a unified system for weather-to-seasonal prediction developed in the United States of America that uses a horizontal resolution of 13 km for medium-range weather prediction and a horizontal resolution of 3 km for global cloud resolving simulations [The geophysical fluid dynamics laboratory (GFDL) System for High-resolution prediction on Earth-to-Local Domains (SHiELD), Harris et al., Citation2020]. In addition, IFS has been well evaluated with a horizontal resolution of 9 km, and its results are comparable to IFS with a horizontal resolution of 1.4 km in many aspects (Wedi et al., Citation2020). Thus, IFS with a horizontal resolution of 9 km works for research purpose in terms of practical use, although Wedi et al. (Citation2020) emphasized the advantages of using a 1 km resolution for resolving deep convection by IFS.
As a pioneer of GCRMs, the NICAM was developed in the early 2000s (Satoh, Citation2002; Satoh, Citation2003; Tomita et al., Citation2001; Tomita & Satoh, Citation2004) and has been improved over the past twenty years (Kodama et al., Citation2021; Satoh et al., Citation2008a; Satoh et al., Citation2014). NICAM developers first conducted cloud resolving simulations with horizontal resolutions of up to 3.5 km on an Aqua-Planet (Tomita et al., Citation2005) and then achieved cloud resolving simulations with realistic land-ocean distributions (Miura et al., Citation2007a; Citation2007b). Miyamoto et al. (Citation2013) finally attained the world’s first global simulations with a subkilometer horizontal resolution () on the K computer with 20,480 nodes (163,840 cores).
Fig. 3 Cloud images calculated by the NICAM with horizontal resolutions of 14, 3.5 and 0.87 km (after Kajikawa et al., Citation2016). Zoom images of a tropical cyclone are shown in the right column.
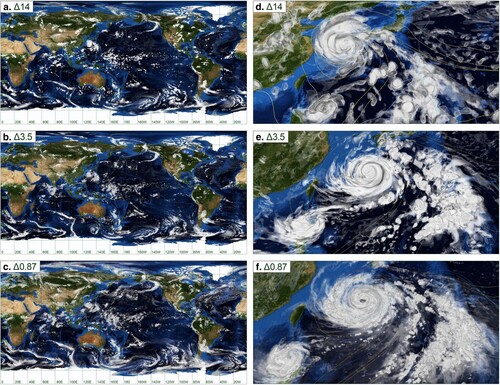
Global statistics of convective cores from subkilometer simulations have shown that the number of convective cores does not converge, even at a 870 m resolution (Miyamoto et al., 2013; Kajikawa et al., Citation2016). Sueki et al. (Citation2019) further investigated the resolved size of convective cores over the tropics and found similar results at a horizontal resolution of 50 m. In general, convective cores were found to become resolved at a horizontal resolution of 1/6 of their sizes (Kajikawa et al., Citation2016; Miyamoto et al., 2013; Miyamoto et al., Citation2015) and to become fully resolved at horizontal resolutions of 1/40–1/20 of their sizes (Sueki et al., Citation2019).
On the other hand, it has been found that individual convective cores are not necessarily resolved for capturing large-scale convective systems that last longer than the daily scale (cf. ) or some aspects of climate states as mentioned above. For example, in practice, a NICAM with a horizontal resolution of 14 km is widely used for Madden-Julian oscillation (MJO), tropical cyclones, and extratropical cyclones. A NICAM with a horizontal resolution of 14 km successfully shows the top-performing skill score of the MJO prediction (Miyakawa et al., Citation2014). Similarly, a NICAM with a horizontal resolution of 14 km successfully reproduces tropical cyclones (e.g. Nakano et al., Citation2015; Oouchi et al., Citation2009; Taniguchi et al., Citation2010; Yanase et al., Citation2010), as was done using a NICAM with finer horizontal resolutions (e.g. Fudeyasu et al., Citation2008; Nakano et al., Citation2017; Yamada et al., Citation2016). In particular, the eyewall structure has been clearly captured for intense tropical cyclones (Yamada et al., Citation2010; Yamada et al., Citation2017; Yamada & Satoh, Citation2013), although the detailed wind structure of tropical cyclones was not sufficiently resolved even at a horizontal resolution of 7 km (Miyamoto et al., Citation2014 and see also ).
We conclude that a horizontal resolution of 14 km, which roughly captures meso-beta-scale (20–200 km) cloud systems according to Sueki et al. (Citation2019), is a baseline for global cloud (system) resolving simulations without cumulus parameterizations provided by the NICAM. In terms of the response of clouds to global warming, the response of clouds smaller than 40–100 km is found to be very different from the response of larger clouds (Noda et al., Citation2014, Citation2016). In terms of the radiative budget, the global mean values almost converged at a horizontal resolution of 3.5 km and did not significantly differ at horizontal resolutions equal to or finer than 14 km (Kajikawa et al., Citation2016), whereas global simulations with horizontal resolutions of 28 km or 56 km suffer from nonnegligible radiation biases (Kodama et al., Citation2021; Seiki et al., Citation2015b). The radiative budget (cloud amount) is found to be affected by the numerical settings of physical processes rather than horizontal resolution (Kodama et al., Citation2021; Roh et al., Citation2017; Seiki et al., Citation2014; Citation2015a), as was shown in other GCMs (e.g. Meehl et al., Citation2019). Therefore, we efficiently optimize a cloud microphysics scheme at a horizontal resolution of 14 km in a strategic way and then fine-tune cloud microphysics at finer objective horizontal resolutions because of their high computational cost.
c How to evaluate cloud microphysics
Explicit coupling between radiation and cloud microphysics enables us to evaluate cloud microphysics through cloud radiative forcing (e.g. Hashino et al., Citation2016). Moreover, the use of a consistent assumption between the radiative transfer model and cloud microphysics represents cloud radiative forcing depending on the particle shape and growth stage of cloud systems (Matsui et al., Citation2020; Seiki et al., Citation2014; Citation2015a; Thompson et al., Citation2016). In addition, the coupling reduces the freedom of tunable model parameters. This substantially reduces efforts for model optimization and hence provides model developers an insight into the improvements in model performance (e.g. Kodama et al., Citation2021).
Satellite simulators push forward the idea of cloud-radiation coupling for the purpose of model evaluation, data assimilation, and observing system simulation experiments (e.g. Masunaga et al., Citation2010). Satellite simulators compile forward radiative transfer models that use model results as an atmospheric environment to match remote sensing (). Thanks to satellite simulators, various types of cloud systems and their dominant cloud microphysics can be evaluated from various aspects by using multisensor analyses. Model evaluations using satellite simulators are reviewed in Section 3 in greater detail.
Fig. 4 A schematic image of the comparison between models and satellites using satellite simulators. From Masunaga et al. (Citation2010), © American Meteorological Society. Used with permission.
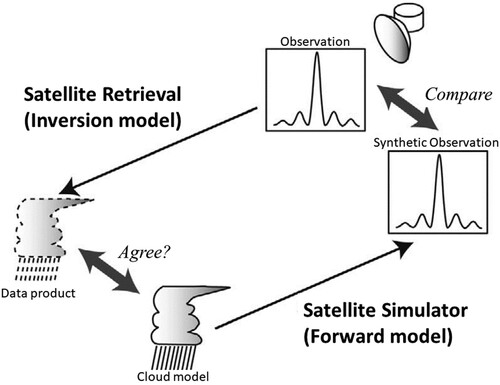
d Benefits to optimizing cloud radiative forcing
Cloud radiative forcing (CRF) is considered the most important parameter to optimize in the performance of cloud microphysics in GCRMs, whereas initialization and reproducibility of specific events are important for mesoscale models. Climate states are realized in the semiequilibrium condition of the energy balance; it is difficult to understand the causal relationship in the balanced state (e.g. Morrison et al., Citation2012; Stevens & Feingold, Citation2009). Therefore, one may design a cloud microphysics scheme that represents correct physical mechanisms with few tuning parameters and then optimize the CRF. Uncertainties in CRF significantly affect climate projection through various pathways of cloud feedback (e.g. Meehl et al., Citation2020; Sherwood et al., Citation2020). In terms of practical use for long-term simulations, CRF should be optimized because climate drift is inevitable because of energy imbalance (e.g. Stockdale, Citation1997).
One can strategically evaluate cloud microphysics schemes by using in situ observations or remote sensing whose objective is a specific type of cloud (details are described in Section 2). Cloud radiative forcing differs by cloud type (e.g. Hartmann et al., Citation1992): distributions of cloud types are climatologically determined by region and season (Rossow & Schiffer, Citation1999). In general, longwave CRF is dominated by high clouds; shortwave CRF is dominated by high thick clouds and low clouds; both longwave and shortwave CRF are effective over the intertropical convergence zone. In particular, cirrus generally has a strong long CRF even with a small optical thickness (Ackerman et al., Citation1988; Fu & Liou, Citation1993; Jensen et al., Citation1994; Liou, Citation1986) and extensively covers the upper troposphere (e.g. Hagihara et al., Citation2010; Haladay & Stephens, Citation2009; Liou, Citation1986; Rossow & Schiffer, Citation1999; Sassen et al., Citation2008) and hence has a strong influence on a broad range of atmospheric layers. In addition, low-level clouds are commonly biased as “too few and too bright” among GCMs (Nam et al., Citation2012) and have been a major source of uncertainties in climate sensitivity (e.g. Andrews et al., Citation2015; Bony & Dufresne, Citation2005; Zhang et al., Citation2013). In particular, a negative bias in low-level clouds strongly increases the downward shortwave radiative flux to the sea surface and consequently affects atmospheric circulation through ocean feedback (e.g. Hyder et al., Citation2018; Kang et al., Citation2009; Kay et al., Citation2016; Trenberth & Fasullo, Citation2010). On the other hand, in the Arctic region, supercooled liquid water in low-level clouds strongly contributes to the longwave CRF to enhance sea ice (land surface ice) melting (e.g. Bennartz et al., Citation2013; Curry et al., Citation1993; Francis et al., Citation2005; Kapsch et al., Citation2013; Citation2016; Shupe & Intrieri, Citation2004). Recently, these strong impacts of CRF from various cloud types on climate states have been used for making a scale to measure the reliability of climate projections as “emergent constraints on future climate change” (e.g. Hall et al., Citation2019). Current progress in understanding climate sensitivity and cloud feedback is comprehensively reviewed in Sherwood et al. (Citation2020).
Improvements in cloud radiative forcing evidently have positive feedback to improvements in model performances through cloud radiation interactions (e.g. Tao et al., Citation1996). For instance, satellite observations indicate that cirrus clouds generally support invigoration of convective clouds over the tropics through longwave CRF (Masunaga & Bony, Citation2018; Masunaga & Mapes, Citation2020). More specifically, the longwave component of the CRF has strong impacts on the structure and track of tropical cyclones (Bu et al., Citation2014; Citation2017; Fovell et al., Citation2010) and the onset of MJO (Takasuka et al., Citation2018). Thus, a cloud microphysics scheme, which is evaluated in terms of CRF, is expected to also work for case studies, regional weather prediction, and regional climate research.
e Global and regional simulations
The hybrid use of a GCRM for global and regional simulations accelerates the evaluation of cloud microphysics using in situ measurements (see and details are described in Section 2). The use of in situ measurements [e.g. the Atmospheric Radiation Measurement (ARM)] will greatly contribute to improvements in GCRMs, as was done for GCMs (e.g. Randall et al., Citation2016). However, it is not efficient to perform global high-resolution simulations for comparison to in situ observations. Therefore, the NICAM employs two types of regional settings that are easily switched with a namelist in the running configuration. One is a stretched grid system on a global domain [so-called stretched NICAM (Shibuya et al., Citation2016; Tomita, Citation2008a; Uchida et al., Citation2016), see (b)]. The other picks up one diamond panel of the icosahedron and uses the panel as the regional domain [so-called diamond NICAM (Uchida et al., Citation2017), see (c)]. Both grid systems share icosahedral grid data, numerical operators, and physical packages with the original global settings. In addition, the single-column model has also been prepared (Seiki & Roh, Citation2020). This kind of regional setting is also used in other GCRMs [ICON (Stevens et al., Citation2020; Zängl et al., Citation2015), FV3 (Harris et al., Citation2019; Citation2020); MPAS (Skamarock, et al., 2012; Citation2018); and UM (Davies et al., Citation2005)].
Fig. 5 Sample images of (a) the default NICAM with the global quasi-uniform icosahedral grid system, (b) stretched NICAM, and (c) diamond NICAM (after Uchida et al., Citation2017, © American Meteorological Society. Used with permission.).
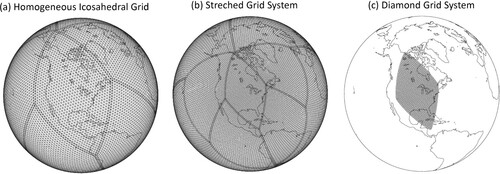
In addition, portable usage of cloud microphysics among GCRMs, mesoscale models, and LES models will complement the evaluation of cloud microphysics and will develop the application of cloud microphysics. Cloud microphysics schemes in the NICAM are shared with SCALE (Nishizawa et al., Citation2015; Sato et al., Citation2015), which works as a mesoscale model and a large eddy simulation model. SCALE is highly optimized for massive parallel supercomputers with codesign by researchers in computational science and computer science (https://scale.riken.jp/) and hence can be used for ultrafine atmospheric simulations with a grid resolution of finer than 10 m (Sato et al., Citation2018) and for experimental applications such as prediction of the electric field by solving the Poisson equation (Sato et al., Citation2019). In addition, one can examine the performance of the cloud microphysics schemes in the NICAM in comparison to more complex schemes such as a spectral bin cloud microphysics scheme or a Lagrangian particle model by using SCALE (e.g. Sato et al., Citation2015; Citation2018). This common usage of cloud microphysics is also found between ICON and COSMO and between MPAS and WRF. In addition, one can use common packages for cloud modeling to obtain widely used cloud microphysics schemes [e.g. Kinematic Driver (Shipway & Hill, Citation2012); and libcloudph++ (Arabas et al., Citation2015; Jaruga & Pawlowska, Citation2018)].
2 Cloud microphysics in the NICAM
A brief history of the development of cloud microphysics schemes and related processes is described in this section. The NICAM has various options for bulk cloud microphysics schemes that assume particle size distribution (PSD) as specific analytic functions. Single-moment bulk cloud microphysics schemes (SMBs) predict the total mass concentration, and double-moment bulk cloud microphysics schemes (DMBs) predict the total number concentration in addition to the total mass concentration to represent the time evolution of PSDs of hydrometeors. Spectral bin cloud microphysics schemes have not yet worked for global simulations with the NICAM because of the difficulty of tuning with their expensive computational cost. Single-moment bulk cloud microphysics schemes are widely used for GCRMs because of their simplicity and cheaper computational cost. summarizes the cloud microphysics schemes used in the DYAMOND GCRMs. Most cloud microphysics in the DYAMOND GCRMs have not yet been comprehensively evaluated for global simulations. Some GCRMs developers have individually started to analyse elemental variables such as surface precipitation (e.g. Arnold et al., Citation2020; Dueben et al., Citation2020; Hohenegger et al., Citation2020) and only a few researches examined regional cloud systems using the DYAMOND dataset (Roh et al., Citation2021; Turbeville et al., Citation2022). Therefore, performances of cloud microphysics in GCRMs other than NICAM have not yet been sufficiently documented. Hereafter we focus on cloud microphysics in NICAM.
Thermodynamics in cloud microphysics schemes are modified to match the NICAM. In particular, the total air density and the moist internal energy are exactly conserved in common procedures such as diagnosis of the saturated vapor pressure (see Satoh, Citation2003 and Satoh et al., Citation2008a, for details). Gravitational sedimentation is commonly solved using a semi-Lagrangian scheme, which is conservative and positive definite (Xiao et al., Citation2003).
a Single-moment bulk cloud microphysics scheme with five water categories
The NICAM team began realistic global cloud resolving simulations with a SMB with five water categories proposed by Grabowski (Citation1998) (hereafter G98). This scheme predicts the specific water content of vapor, cloud water and rain (qv, qc, and qr, respectively) and diagnoses the specific water content of cloud ice and snow (qi and qs, respectively) by using temperature. The concept of G98 is to represent different latent heat between the ice phase and liquid phase (e.g. latent heat of vaporization Lv = 2.50e6 J kg−1 and latent heat of sublimation Ls = 2.83e6 J kg−1 at atmospheric temperature Ta = 273 K) and different terminal velocities between rain and snow (typically 3–7 m s−1 for rain and at most 1 m s−1 for snow in G98), although G98 cannot deal with melting and freezing due to the diagnosis. The diagnosis of ice categories is also used in other SMBs for weather prediction (e.g. Khairoutdinov & Randall, Citation2003; Lopez, Citation2002). As G98 is simple, there are only four tunable processes: auto-conversion and accretion of cloud water, auto-conversion and accretion of cloud ice, terminal velocity of rain, and terminal velocity of snow. Therefore, radiative fluxes of G98 were optimized by modifying the terminal velocity of snow (Iga et al., Citation2007) with the assumed effective radii of cloud ice and snow as 40 μm.
These five category types of cloud microphysics do not separate dense ice (graupel and hail) and light ice (snow) and hence do not capture differences in dominant growth processes between convective precipitation systems (riming and accretion) and stratiform precipitation systems (aggregation). In addition, the diagnosis of cloud ice and snow results in the absence of the melting layer (freezing layer). These characteristics are apparently observed in the vertical structure of radar echo by reference to satellite observations (Masunaga et al., Citation2008; Satoh et al., Citation2008b). Nevertheless, G98 sufficiently worked to capture deep convective systems over the tropics, such as tropical cyclones (e.g. Fudeyasu et al., Citation2008; Oouchi et al., Citation2009; Taniguchi et al., Citation2010; Yanase et al., Citation2010) and MJO (e.g. Miura et al., Citation2007a; Miyakawa et al., Citation2012; Nasuno et al., Citation2009; Taniguchi et al., Citation2010).
b Single-moment bulk cloud microphysics scheme with six water categories
The NICAM contains a SMB with six water categories referred to as NSW6 (NICAM single-moment bulk cloud microphysics with six water categories). This scheme predicts the specific water content of vapor, cloud water, rain, cloud ice, snow, and graupel (qv, qc, qr, qi, qs, and qg, respectively). Tomita (Citation2008b) simplified a six-category type based on Lin et al. (Citation1983) and Rutledge and Hobbs (Citation1984) by omitting hail production and replacing the interaction between cloud water and cloud ice with a saturation adjustment to reduce the computational cost. These simplifications allow NSW6 to be similar to G98 except for microphysical processes involving graupel. Kodama et al. (Citation2012) optimized the longwave CRF by modifying the timescale of the auto-conversion of cloud ice to snow. The 2012 version of the NICAM (NICAM.12), which was generally used for published works from 2012 to 2019 [e.g. an atmospheric model intercomparison project (AMIP) type experiment (Kodama et al., Citation2015)], was established based on this version of NSW6. The reproducibility and forecasting skills for tropical cyclones, MJO, and boreal-summer intra-seasonal oscillation improved by using NSW6 (e.g. Kikuchi et al., Citation2017a; Miyakawa et al., Citation2014; Miyakawa & Kikuchi, Citation2018; Nakano et al., Citation2015; Citation2017; Nakano & Kikuchi, Citation2019; Shibuya et al., Citation2021).
Major revision of microphysical processes involving rain, snow, and graupel in NSW6 was accomplished by Roh and Satoh (Citation2014) using the stretched NICAM by reference to TRMM satellite observations. The objectives of the revisions were to capture the vertical structure of convective systems over the tropics and to separately capture different types of cloud systems. Specifically, a rescaled bimodal shape of snow particle size distribution and variable bulk snow density were assumed following Field et al. (Citation2005) and Thompson et al. (Citation2008); excessive graupel was suppressed by switching off accretion of snow and cloud ice by graupel following Lang et al. (Citation2007) and by changing the intercept parameter of the graupel particle size distribution following Gilmore et al. (Citation2004); and a variable formulation of the intercept parameter of the rain particle size distribution was incorporated to represent stratiform rain systems following Zhang et al. (Citation2008). In addition, the saturation adjustment for cloud ice was replaced with vapor deposition and sublimation to solve well-known issues (e.g. Gettelman et al., Citation2010). These modifications significantly increased cloud ice and snow globally, and decreased graupel in the mid-latitudes. As a result, vertical profiles of ice water content from this version of NSW6 more closely approximated the satellite observations compared with those from NSW6 in NICAM.12 ((a–c)).
Fig. 6 Comparison of the vertical profiles of annual mean ice water content (IWC) [mg m-3] from (a) CloudSat satellite observations [2B-CWC-RO product (Austin et al., Citation2009; Austin & Stephens, Citation2001)], (b) NSW6 in NICAM.12, (c) NSW6 in NICAM.16, and NDW6 in NICAM.16. The solid lines represent 273-K isotherms. The global simulation data with horizontal resolution of 14 km and 38 vertical layers were provided by courtesy of C. Kodama and A. T. Noda.
![Fig. 6 Comparison of the vertical profiles of annual mean ice water content (IWC) [mg m-3] from (a) CloudSat satellite observations [2B-CWC-RO product (Austin et al., Citation2009; Austin & Stephens, Citation2001)], (b) NSW6 in NICAM.12, (c) NSW6 in NICAM.16, and NDW6 in NICAM.16. The solid lines represent 273-K isotherms. The global simulation data with horizontal resolution of 14 km and 38 vertical layers were provided by courtesy of C. Kodama and A. T. Noda.](/cms/asset/df848e83-151e-4ada-8c2a-4b743157dd7a/tato_a_2075310_f0006_ob.jpg)
Finally, the cloud radiative forcing was successfully improved by explicitly coupling cloud microphysics and radiative transfer using the coupling procedure provided by Seiki et al. (Citation2014) [Kodama et al. (Citation2021); details are described in Section 2.4]. The 2016 version of the NICAM (NICAM.16), which has been used for published works after 2020 [e.g. production for HighResMIP (Kodama et al., Citation2021)], was established based on this version of NSW6.
The next version of NSW6 is now under development. Global simulations with NSW6 were found to suffer from the underestimation bias in low-level mixed-phase clouds in the midlatitudes to polar regions (Hashino et al., Citation2016; Kodama et al., Citation2015; Roh et al., Citation2020). Recently, Seiki and Roh (Citation2020) improved the bias by replacing rain-production terms with the auto-conversion and accretion parameterizations by Khairoutdinov and Kogan (Citation2000), an ice nucleation term with heterogeneous ice nucleation by Phillips et al. (Citation2007), and suppressing vapor deposition and riming with reasonable thresholds. These modifications successfully prolonged the lifetime of mixed-phase low-level clouds by sustaining supercooled liquid water. A similar approach was used to improve low-level mixed-phase clouds by Furtado and Field (Citation2017) with UM and Engdahl et al. (Citation2020) with AROME. The improvements were confirmed in long-term global simulations (Noda et al., Citation2021).
c Double-moment bulk cloud microphysics scheme with six water categories
The NICAM contains a DMB with six water categories referred to as NDW6 (NICAM double-moment bulk cloud microphysics with six water categories). This scheme predicts the specific water content of vapor, cloud water, rain, cloud ice, snow, and graupel (qv, qc, qr, qi, qs, and qg, respectively) and the number concentration of each hydrometeor category (nc, nr, ni, ns, and ng, respectively). Seiki and Nakajima (Citation2014) developed NDW6 based on Seifert and Beheng (Citation2001; Citation2006) and Seifert (Citation2008) (hereafter SB) with minor modifications (e.g. saturation adjustment of cloud water is replaced with condensation/evaporation). The SB scheme was evaluated in terms of surface precipitation but not for CRF (e.g. Seifert et al., Citation2006). As a result, large biases in outgoing longwave radiation at the top of the atmosphere (OLR) were found in global simulations with NDW6 ((a)). This version of NDW6 is referred to as NDW6-SN14.
Fig. 7 Comparison of outgoing longwave radiation at the top of the atmosphere (OLR) from CERES satellite observations (solid lines with points) and NICAM simulations with various versions of NDW6 (solid lines) (after Satoh et al., Citation2018).
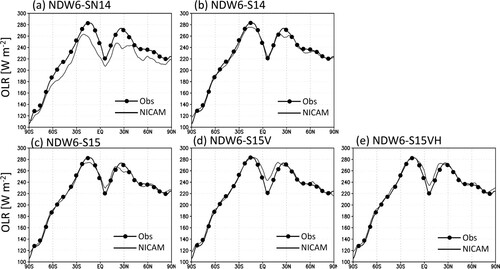
Seiki et al. (Citation2014) elaborated ice modelling of NDW6 to improve the OLR biases originating from cirrus clouds: the heterogeneous ice nucleation scheme was replaced with the scheme based on Phillips et al. (Citation2007), who referred to experimental data from field campaign observations by DeMott et al. (Citation2003); nonsphericity was incorporated into ice cloud microphysics assuming power-law relationships between the projected area and the particle mass and between the maximum dimension and the particle mass based on Mitchell (Citation1996); and nonsphericity was incorporated into CRF based on Fu (Citation1996) and Fu et al. (Citation1998) (details are described in Section 2.4). These improvements were examined using a stretched NICAM by reference to in situ sonde observations. These modifications significantly improved the OLR biases in global simulations ((b)). This version of NDW6 is referred to as NDW6-S14.
Seiki et al. (Citation2015b) incorporated homogeneous ice nucleation into NDW6 based on Ren and Mackenzie (Citation2005) and Kärcher et al. (Citation2006) to alleviate the underestimation bias in ni found by Seiki et al. (Citation2014). In addition, the lower limit of the sticking efficiency was incorporated into ice aggregation because of a lack of experimental evidence at atmospheric temperatures colder than 253 K (Pruppacher and Klett, Citation2010). Uncertainties in the sticking efficiency were also discussed in Li et al. (Citation2010). The impacts of the former change on OLR, which caused a significant decrease in OLR, were cancelled by the latter change, and thus, OLR did not significantly change in this latest version of NDW6 ((c)). This version of NDW6 is referred to as NDW6-S15 and is implemented in NICAM.16. Ice water content from NDW6-S15 (NDW6 in NICAM.16) is a little bit underestimated, especially just above the freezing level ((a and d)). Ice cloud microphysics in winter snowfall systems, in which interaction between cloud ice and snow dominated, are well represented by NDW6-S15 (Kondo et al., Citation2021), and hence, hydrometeor interactions between rain and graupel might be problematic. The bias in IWC and higher OLR over the tropics indicate that NDW6-S15 underestimate high-density ice (graupel) originated by convection.
The remaining biases in OLR were found to originate from the spatial resolution of the NICAM (Seiki et al., Citation2015b). Specifically, negative biases in OLR over the subtropical regions originated from insufficient vertical resolution for capturing thin cirrus layers, and positive biases in OLR over the tropics originated from insufficient horizontal resolution for capturing convective organization. Both biases clearly diminish as vertical layers increase (NDW6-S15 V, (d)) and horizontal resolution increases (NDW6-S15VH, (e)). Therefore, NDW6 does not need extensive tuning in terms of CRF. In the current status of the NICAM (e.g. Stevens et al., Citation2019), 78 vertical layers with a layer thickness of approximately 400 m in the free troposphere are used as a standard setting based on Seiki et al. (Citation2015b).
d Coupling procedure between cloud microphysics and radiative transfer
Cloud radiative forcing significantly differs based on the assumption of single-scattering properties (SSPs). For example, the nonsphericity of ice particles had an impact on outgoing solar radiation at the top of the atmosphere (OSR) by more than 100 W m−2 in a cirrus case [Seiki et al. (Citation2014) and see ]. In global simulations, an invalid assumption of effective radii as a globally fixed value, which was used in NICAM.12, caused nonnegligible biases in both OLR and OSR [10–20 W m−2 from the tropics to mid-latitudes (Kodama et al., Citation2021; Seiki et al., Citation2015a), and see ]. In addition, the nonsphericity of ice particles also has systematically nonnegligible biases in OSR ((b)). These biases in CRF are comparable to or greater than the biases originating from uncertainties in cloud microphysics. Thus, it is better to optimize cloud microphysics after explicitly coupling cloud microphysics and radiative transfer. Importance of cloud-radiation coupling for numerical weather prediction was discussed also using other models (e.g. Thompson et al., Citation2016; Matsui et al., Citation2020).
Fig. 8 The vertical profiles of (a) downward shortwave radiation and (b) upward shortwave radiation (after Seiki et al., Citation2014). Thick lines indicate observations by radiometer sonde, thin solid lines indicate NICAM simulations using NDW6 with spherical SSPs, and thin dashed lines indicate NICAM simulations using NDW6 with nonspherical SSPs. The dashed rectangles indicate the location of a cirrus layer.
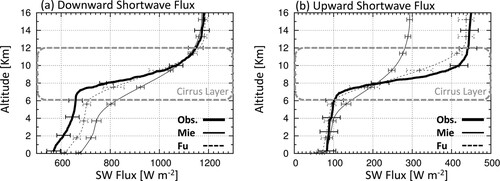
Fig. 9 Comparison of (a) OLR and (b) outgoing shortwave radiation at the top of the atmosphere (OSR) from CERES satellite observations (solid lines) and NICAM.16 simulations with various settings of cloud and radiation coupling. NSW6 uses full coupling between cloud and radiation (dashed lines), NSW6-Mie assumes variable effective radii but spherical SSPs (long dashed short dashed lines), and NSW6-Mie-ReFIX assumes constant effective radii and spherical SSPs (dotted lines). The global simulation data were provided by courtesy of C. Kodama.
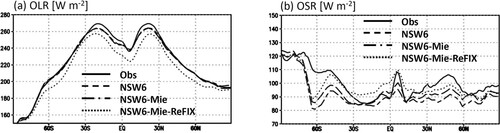
Cloud microphysics is coupled with radiative transfer through SSPs of hydrometeors. The single-scattering properties strongly depend on the ratio of particle size to wavelength (e.g. Hansen & Travis, Citation1974); hence, SSPs are generally prepared as a look-up table of effective radii at each wavelength band in radiative transfer models. In the NICAM, radiative transfer is calculated with a broadband model mstrnX (Sekiguchi & Nakajima, Citation2008), which requires the extinction coefficient per unit volume of hydrometeor particles, scattering coefficients per unit volume of the hydrometeor particles, and moments of the phase function (asymmetry factor and truncation factor) used for the delta two-stream approximation (Nakajima et al., Citation2000). Thus, input data to the coupling procedure are the volume of the hydrometeors and effective radii derived from cloud microphysics.
In the latest version of the NICAM [NICAM.16 (Kodama et al., Citation2021)], nonsphericity is newly assumed for the SSP of ice hydrometeors because nonsphericity has been found to have a strong impact on both shortwave radiation (e.g. Fu, Citation2007; McFarquhar et al., Citation1999; Macke, Citation1993; Pollack & Cuzzi, Citation1980; Stephens et al., Citation1990; Takano & Liou, Citation1989, Citation1995; Yang & Liou, Citation1995) and longwave radiation (e.g. Ackerman & Stephens, Citation1987; Mitchell & Arnott, Citation1994; Mitchell et al., Citation1996). A nonspherical database provided by Fu (Citation1996) and Fu et al. (Citation1998) was compiled for the wavelength bands used in mstrnX, and then the SSP look-up table was prepared in the range of effective radii from 1 μm to 1 mm (Seiki et al., Citation2014). One may choose another nonspherical SSP database that has options to choose specific shapes, such as Yang et al. (Citation2013) [e.g. NU-WRF (Matsui et al., 2018); MIROC (Tatebe et al., Citation2019)]. Seiki et al. (Citation2014) found that the dependence of the asymmetry factor on the effective radii, which vary by particle shape, is key to determining the cloud albedo of cirrus, as shown in .
The definition of the effective radii of nonspherical ice particles is another issue for nonspherical modelling because the “effective” size is not obvious for irregular shapes in contrast to a sphere. Therefore, the concept of “effective distance”, which is defined by the ratio of the volume to the projected area, was proposed by Mitchell and Arnott (Citation1994). The concept was confirmed by Fu (1998) by reference to light scattering calculated using the finite difference time domain method. Following equations (3.11) in Fu (1996) or (2.5) in Fu et al. (Citation1998), the effective radii of ice hydrometeors re are defined as follows:
(1)
(1) where ρa is the air density, ρice = 916.7 kg m−3 is the ice density, A is the projected area, x is the particle mass, and f is the particle mass distribution.
EquationEquation (1)(1)
(1) is analytically integrated with the power-law relationship between A and x for each hydrometeor category (A = γxσ). In NDW6, particle models compiled by Mitchell (Citation1996) are used: hexagonal columns for cloud ice, assemblages of planar polycrystals in cirrus clouds for snow, and lump graupel for graupel (Seiki et al., Citation2014). In NSW6, sponge-like spherical ice is assumed for cloud ice and graupel, and two-dimensional fractal shapes (e.g. A ∝ D2 and x ∝ D2) are assumed for snow (Kodama et al., Citation2021; Roh & Satoh, Citation2014). Thus, the effective radii of snow are assumed to be constant (res = 125 μm). The cloud radiative forcing of NSW6 significantly decreases with this assumption for the effective radii (see NSW6, NSW6-Mie, and NSW6-Mie-ReFIX in ).
e Impact of precipitating hydrometeors on the radiation budget
The impacts of precipitating hydrometeors on CRF can be examined by means of the new look-up table. CMIP3 and CMIP5 GCMs commonly have large biases in CRF over the ITCZ region (Li et al., Citation2013). Past studies suggested that these biases originated from the lack of CRF by precipitating hydrometeors in conventional GCMs (e.g. Li et al., Citation2014; Citation2016; Waliser et al., Citation2011). Chen et al. (Citation2018) analysed the role of precipitating hydrometeors by using an off-line radiation model with NICAM results and found that the layering structure of snow underlying cloud ice had impacts on OLR over the tropics and storm-track regions up to 2 W m−2. Similarly, snow has strong impacts on OSR up to 3 W m−2 in the summer hemisphere.
The degree of the possible biases related to precipitating hydrometeors could differ by model (Matsui et al., 2018; Michibata et al., Citation2019), but it is evident that the systematic effect of precipitating hydrometeors on radiation is nonnegligible in terms of climate projection. Recently, precipitating hydrometeors have been found to have important indirect effects on aerosol-cloud interactions (so-called buffering effects) with MIROC (Michibata et al., Citation2020; Michibata & Suzuki, Citation2020).
f Differences between SMBs and DMBs
Representation of changing number concentrations is essential for representing realistic cloud growth. shows the typical cloud microphysical processes used in SMBs and DMBs. It is clearly seen that most processes change the number concentrations in addition to the mass concentration. SMBs generally deal with this issue by assuming PSD functions (qj and nj relationship) based on observations. Thus, the performance of SMBs relies on the diagnosis technique of PSDs.
Fig. 10 Cloud microphysical processes and cloud interaction among water classes (after Satoh et al., Citation2018). Solid lines indicate the processes that change the number and mass concentration of hydrometeors, while dashed lines indicate the processes that change only the mass concentration of hydrometeors. Hom/het indicates homogeneous/heterogeneous, respectively.
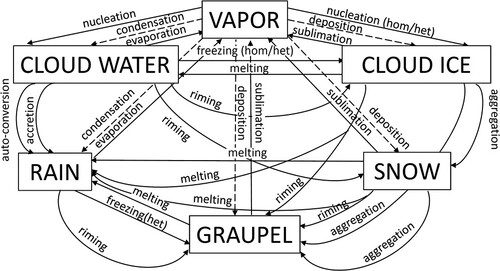
The complexity and dependence of cloud microphysical processes inevitably differ between DMBs and SMBs regardless of the diagnosis technique of PSDs. For example, condensation increases only qr, but evaporation decreases both qr and nr (e.g. Morrison & Grabowski, Citation2008). In addition, larger liquid droplets are more likely to freeze [heterogeneous and homogeneous freezing of supercooled liquid water (e.g. Bigg, Citation1953; Fukuta & Schaller, Citation1982; Khvorostyanov & Curry, Citation2009)], but smaller ice crystals more easily melt away. This kind of asymmetry between a pair of growth and decay processes (see the pairs of arrows in ) induces hysteresis, and hence, cannot be represented by SMBs. As a result, SMBs necessarily simplify these processes and modify the balance among the hydrometeor interactions. The ratio of the tendency between the mass concentration and the number concentration [(∂qj/∂t)/(∂nj/∂t)] differs by process, and hence, these inconsistencies in the tendency result in bias in the mean particle mass or mean particle diameter
when using SMBs.
Biases in the mean particle mass (diameter) feedback to the performance of cloud microphysics schemes through the dependence of particle-growth equations (e.g. Igel et al., Citation2015; Seiki et al., Citation2015a; Seiki & Roh, Citation2020). The dependences of cloud-related processes on bulk cloud microphysical parameters are summarized in . For example, in collisional processes, the collisional cross section is proportional to the square of the particle diameter. In addition, in evaporation (sublimation) and condensation (deposition), vapor flux onto the particles is proportional to their surface area (square of the particle diameter). Moreover, terminal velocity, which is contained in many microphysical processes, is mostly determined by the particle diameter (Böhm, Citation1989; Citation1992; Mitchell, Citation1996). In terms of CRF, the extinction efficiency is represented as a function of the size parameter, and the extinction cross-section is proportional to the square of the particle radii as mentioned in Section 2.4. Thus, strong feedbacks of xj (or Dj) to cloud microphysics and CRF imply that cloud microphysics can potentially be evaluated by monitoring xj (or Dj) from observations. Specifically, optical sensors, which are sensitive to various moments of particle radii, are powerful tools to define the growth mode of clouds. Evaluation of cloud microphysics in the NICAM using satellite observations is described in Section 3.
Table 3. Dependences of cloud-related processes on bulk cloud microphysical parameters.
3 Model evaluation using optical sensors
a Sensitivity of optical sensors
There are two kinds of optical sensors to observe clouds and precipitating hydrometeors. Passive sensors can observe the radiance from clouds and precipitation and have more spatial coverage than active sensors. Active sensors have a transmitter and a receiver and can detect the vertical profiles of clouds and precipitation, but they have a smaller swath than passive sensors. Thus, the combined use of the two types of optical sensors can complementarily reveal the nature of cloud systems in detail. In addition, satellite sensors have different characteristics depending on the wavelength of the sensor. The major satellites used for model evaluation are summarized in .
Table 4. Optical sensors and corresponding sensitive physical parameters.
Visible (VIS) channels can observe optically thick clouds, and the observations are limited during daytime. Visible channels have been widely used for estimating warm-cloud optical properties [e.g. optical thickness and effective radius (Nakajima & King, Citation1990)]. The infrared (IR) channels, especially from 10 to 13 μm wavelength, are sensitive to cloud-top temperature and can detect even very thin cirrus clouds. Infrared channels have also been utilized for detecting cloud optical properties (e.g. Iwabuchi et al., Citation2016; Citation2018). The combined use of VIS and IR channels has been used for categorizing cloud systems using the cloud optical thickness and cloud-top temperature [e.g. the International Satellite Cloud Climatology Project cloud category proposed by Rossow and Schiffer (Citation1999)].
Passive microwaves are sensitive to precipitating hydrometeors. Low frequency microwaves (<20 GHz) are used to capture the emissions from the path-integrated water content of rain over the ocean. On the other hand, high-frequency microwaves (>80 GHz) are used for capturing scattering signals of large ice particles such as snow and graupel over land and ocean. These various frequencies of microwave observations enable us to obtain integrated column information about precipitating hydrometeors, particularly in deep convective systems.
Active microwave observations referred to as radar are now available at 94 GHz/3.2 mm (so-called cloud radar) and at 13.6 GHz/2.2 cm and 35.5 GHz/8.5 mm (so-called precipitation radar). In addition, lidar with wavelengths of 532 and 1064 nm is available. In general, the backscattering coefficient rapidly decreases at size parameters (2πr/λ) smaller than 1 according to Mie theory, and hence, the detectable particle size differs by the frequency/wavelength. In addition, too strong backscattering results in strong attenuation of return signals. The Cloud Profilin Radar (CPR) on CloudSat can clearly detect vertical profiles of nonprecipitating and light-precipitating clouds and slightly detect intense precipitating clouds due to attenuation (Stephens et al., Citation2008). CALIOP on CALIPSO can detect optical thin cirrus and the thermodynamic phase of clouds using the depolarization ratio (Winker et al., Citation2009). The precipitation radar on TRMM and GPM core satellites can detect the vertical profiles of precipitating clouds but cannot detect nonprecipitating clouds (Hou et al., Citation2014). Even when using GPM satellite observations, it is difficult to accurately capture extreme precipitation systems such as hail storms due to strong attenuation, multiple scattering, and spatial inhomogeneity (e.g. Mroz et al., Citation2018). Thus, the combined use of lidar, cloud radar, and precipitation radar enables us to integrate understandings of cloud organization from shallow clouds to deep convective clouds.
Consistency in the assumption of cloud microphysics between a model and a satellite retrieval algorithm is an important issue. For example, so-called Z-R and k-Z relationships, which implicitly assume a PSD, are used in the retrieval of precipitation flux using PR (Iguchi et al., Citation2009). However, the assumptions are generally not used in cloud microphysics schemes. In contrast, some cloud microphysics schemes (e.g. Milbrandt & Yau, Citation2005; Seifert, Citation2008) have more degrees of freedom to represent PSDs than these retrieval algorithms. In addition, satellite products inherently suffer from contamination: a product consists of a major portion of an objective physical parameter and a minor portion of other physical parameters. For example, in the past, it was difficult to separate cloud water paths from liquid water paths in the retrieval of microwaves (Elsaesser et al., Citation2017). It is still difficult to retrieve snowfall over sea ice or land ice using microwaves. Thus, we need to understand the characteristics of optical sensors in comparing model results to satellite observations.
Satellite simulators solve the issue by directly comparing emulated satellite signals from model results to level 1 satellite data (see ). This comparison method enables us to share microphysical assumptions between a model and satellite simulators. On the other hand, it is difficult to interpret radiance-based comparisons to find biases in cloud microphysics schemes. Current progress in analytical techniques using satellite simulators is described in the following sections. Note that the accuracy of emulated satellite signals depends on the forward radiative transfer calculation method used in a satellite simulator and hence it needs to be examined, especially in data assimilation (e.g. Okamoto, Citation2017; Okamoto et al., Citation2019). At present, various satellite simulators (optical sensor simulators) have been developed [e.g. COSP (Bodas-Salcedo et al., Citation2011; Swales et al., Citation2018), CRTM (Chen et al., Citation2008; Ding et al., Citation2011), ECSIM (Reverdy et al., Citation2015; Voors et al., Citation2017), Joint-Simulator (Hashino et al., Citation2013; Satoh et al., Citation2016), POLARRIS (Matsui et al., Citation2019), RTTOV (Saunders et al., Citation2018), SDSU (Masunaga et al., Citation2010), and Goddard-SDSU (Matsui et al., Citation2013; Citation2014)].
b Evaluation of the size distribution of cloud systems
The horizontal resolution of GCRMs that is comparable to satellite observations is a milestone for the evaluation of cloud representation. For example, infrared channels on a geostationary satellite MTSAT, which were frequently used for capturing cirrus clouds (e.g. Inoue, Citation1987; Mapes & Houze, Citation1993), had a footprint size of approximately 4 km. Thus, cloud size can be newly evaluated by the advent of GCRMs, whereas cloud fraction is derived from macrophysics in conventional GCMs (e.g. Park et al., Citation2014; Watanabe et al., Citation2009). Horizontal inhomogeneity in cloud distribution develops as the cloud regime changes (Kawai & Teixeira, Citation2010; Citation2012), and hence, explicit representation of cloud systems is the unique approach of GCRMs. One can use GCRMs’ cloud distribution to evaluate sub-grid inhomogeneity assumed in conventional GCMs (e.g. Hotta et al., Citation2020; Watanabe et al., Citation2009).
The split-window technique, which uses brightness temperature of 11 and 12 μm, is generally applied to classify high clouds (e.g. Inoue, Citation1987; Liou, Citation2002). In addition, OLR or IWP can also be applicable to classifying relatively thick high clouds over the subtropics to tropics (e.g. Inoue et al., Citation2006; Citation2008; Citation2010; Inoue & Ackerman, Citation2002). The size distribution of high clouds derived from MTSAT was well reproduced by the NICAM with a horizontal resolution of 3.5 km (Inoue et al., Citation2008), and smaller clouds are likely to be underestimated as the horizontal resolution decreases to 7 km or 14 km (Inoue et al., Citation2008; Noda et al., Citation2014). Recently, CloudSat and CALPSO satellite observations have also been available for estimating cloud sizes with the categorization of cloud types (e.g. Seiki et al., Citation2019)
A new issue arises from the NICAM simulations: formation processes of smaller high clouds, which are not well represented in GCRMs or conventional GCMs. Even small clouds make a large contribution to the total CRF over the tropics due to their large population. This is true for the response of high clouds to global warming (Noda et al., Citation2014; Citation2016). Simulated results from the NICAM indicated that the response of high clouds with cloud radii smaller than 50 km to global warming significantly differs from the response with cloud radii larger than 100 km (Noda et al., Citation2014; Citation2016). In particular, the robustness of the fixed anvil temperature (FAT) hypothesis (Hartmann & Larson, Citation2002; Zelinka & Hartmann, Citation2010) is found to depend on cloud sizes (Noda et al., Citation2016). shows an example of the different responses of high clouds to global warming by cloud size. The OLR change under global warming attributes to a change in emissivity, a change in cloud top temperature, and a change in clear sky radiation. A reduction in the emissivity is found to be dominant in the response of smaller thin cirrus whereas an increase in the cloud top temperature is dominant in the response of larger clouds as was established by conventional GCMs with the FAT hypothesis. Moreover, Noda et al. (Citation2019) argued that convective self-aggregation (e.g. Wing et al., Citation2017) does not sufficiently work to reduce CRF over the tropics from cloud-size analysis using global high-resolution simulations. Note that cirrus clouds contain very small-scale structures, such as uncinus and streak structures (e.g. Heymsfield, Citation1975), which can be captured by using large eddy simulation models (e.g. Sölch & Kärcher, Citation2011). Thus, the response of smaller cirrus formations to climate change is an underdeveloped issue.
Fig. 11 Breakdown of OLR change under global warming simulated by the NICAM (after Noda et al., Citation2016). An OLR change ΔF is attributed to a change in emissivity Δε, a change in cloud-top temperature ΔTCT, and a change in clear sky radiation ΔFCLR. The breakdown was calculated by cloud size. Sum shows the summation of the contributions and the difference between ΔF and Sum indicates the error of the analysis.
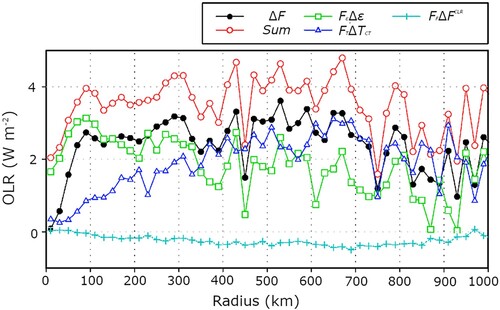
c Evaluation of vertical structure of cloud systems
The vertical structures of precipitating cloud systems from NICAM simulations have been evaluated using active sensors: TRMM-PR, CloudSat-CPR, and CALIPSO-CALIOP. In particular, TRMM-PR has a footprint of 5 km, and hence, rain microphysics is directly evaluated without assuming subgrid variability. TRMM-PR enables us to evaluate the difference in the vertical structure of precipitation flux between convective cloud systems and stratiform cloud systems (Satoh et al., Citation2008b; Takayabu, Citation2002). Here, the physical parameter “precipitation flux” has inconsistency in the terminal velocities of precipitating hydrometeors between the NICAM and TRMM algorithms. Therefore, the evaluation of models using retrieved products is not an apple-to-apple comparison. After Satoh et al. (Citation2008b), we generally used satellite simulators to evaluate the vertical structures of cloud systems.
The contoured frequency by altitude diagram (CFAD), which illustrates the probability density function of the radar reflectivity at each vertical level, is frequently used for evaluating the vertical structure of cloud systems (e.g. Marchand et al., Citation2009; Matsui et al., Citation2016). Atmospheric temperature is also used as the vertical axis of the CFAD to capture differences in the freezing level and the tropopause level by latitude. The shape of the CFAD is sensitive to cloud microphysics or assumptions of particle shapes (e.g. Hashino et al., Citation2013; Masunaga et al., Citation2008; Satoh et al., Citation2010). In addition to the CFAD, a cloud-top beta-temperature radar-conditioned diagram was also proposed by Hashino et al. (Citation2013) to analyse the relationship between ice water content and ice effective radii. The sensitivity of cloud microphysics to the CFAD was clearly observed within only 7 days from NICAM simulations (e.g. Roh et al., Citation2017; Roh & Satoh, Citation2014; Satoh et al., Citation2010). Therefore, we did not require longer simulations (e.g. seasonal to annual simulations) to evaluate cloud microphysics schemes using satellite observations.
Nakajima et al. (Citation2010) proposed a contoured frequency by using the optical depth diagram (CFODD), which uses the cloud optical depth from the cloud top (COD) as the vertical axis, by using Aqua and CloudSat satellite observations. This alternative diagram successfully captures the transition of the droplet growth mode from condensational growth to collisional growth by means of the characteristics that the COD is directly related to the liquid water path and effective radius (Suzuki et al., Citation2010; Citation2011). Auto-conversion and accretion in warm clouds have been globally evaluated by using the CFODD (Suzuki et al., Citation2013; Citation2015). Kuba et al. (Citation2015) analysed the detectable cloud microphysics in the CFODD using a spectral bin cloud microphysics scheme.
In terms of the evaluation of cloud microphysics schemes in the NICAM, Kuba et al. (Citation2020) found that the CFODD is sensitive to the shape parameters assumed in the PSD function from the comparison between NDW6 and a spectral bin cloud microphysics scheme. The contoured frequency by optical depth diagram can also be utilized for the evaluation of vertical structures of cirrus clouds by combining CloudSat and CALIPSO satellite observations (Seiki et al., Citation2015b). One can easily analyze the CFODD for cirrus clouds by using the EarthCARE Research Product Monitor (https://www.eorc.jaxa.jp/EARTHCARE/research_product/ecare_monitor.html) provided by the Japan Aerospace Exploration Agency.
d Evaluation of cloud organization
Masunaga et al. (Citation2005) proposed an analytical method to categorize cloud systems using cloud-top temperature and radar echo top height from TRMM satellite observations (see (a)). The categorized cloud systems were found to be closely related to large scale circulation (Masunaga & Kummerow, Citation2006). Thus, the cloud-top and rain-top diagrams can be used for the evaluation of cloud organization in GCRMs (e.g. Masunaga et al., Citation2008). Recently, Roh and Satoh (Citation2018) proposed a method to extend the observable coverage by using the polarization-corrected brightness temperature at 89 GHz (PCT89) instead of radar echo to detect the rain-top height.
Fig. 12 The cloud-top and rain-top diagram using Ku-band radar echo and infrared brightness temperature from (a) satellite observations, (c) NSW6 in NICAM.12, and (e) NSW6 in NICAM.16. CFAD using Ku-band radar echo from (b) satellite observations, (d) NSW6 in NICAM.12, and (f) NSW6 in NICAM.16. Here, simulated results were processed by a Joint-Simulator. From Roh et al. (Citation2017), © American Meteorological Society. Used with permission.
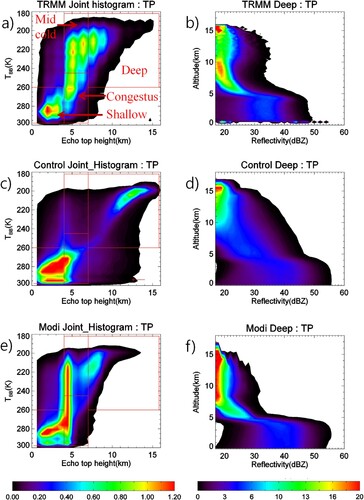
Matsui et al. (Citation2009) further established a method to systematically evaluate the cloud microphysics scheme [Triple-Sensor Three-Step Evaluation Framework (T3EF)]: comparisons of (1) the cloud-top and rain-top diagram from IR radiance and radar echo, (2) CFAD from radar echo, and (3) cumulative probability distribution of PCT85 from a high-frequency microwave imager. T3EF clearly revealed problematic processes in a cloud microphysics scheme (Li et al., Citation2010). NSW6 was successfully revised by using T3EF (Roh & Satoh, Citation2014; Roh et al., Citation2017, and see ).
e Evaluation of hydrometeor classes
Global observations of the depolarization ratio from CALIOP enable us to discriminate the thermodynamic phase of hydrometeors and the shape of ice particles (Hu et al., Citation2009; Citation2010; Saito et al., Citation2017; Yoshida et al., Citation2010). Recently, the GCM-Oriented CALIPSO Cloud Product (CALIPSO-GOCCP) was released for easy comparison between models and CALIPSO satellite observations (Chepfer et al., Citation2010). One can evaluate the frequency of occurrence of liquid and ice clouds to evaluate the freezing process in cloud microphysics schemes using GOCCP (Cesana & Chepfer, Citation2012). The GCM results were more consistently evaluated by satellite observations with the aid of a satellite simulator (Cesana et al., Citation2015; Cesana & Chepfer, Citation2013). Note that hydrometeor classification using CALIOP is limited to nonprecipitating particles near the cloud top due to the limitation of attenuation (e.g. Hagihara et al., Citation2014).
Roh et al. (Citation2020) developed a single parameterization to diagnose the depolarization ratio from the backscattering coefficient and then evaluated NSW6 and NDW6 using the hydrometeor discrimination method proposed by Yoshida et al. (Citation2010) (see ). NSW6 underestimated mixed-phase low-level clouds over the Southern Ocean due to poor representation of supercooled liquid water, whereas NDW6 reproduced mixed-phase low-level clouds well. The bias in NSW6 was successfully alleviated by Seiki and Roh (Citation2020), as described in Section 2.2.
Fig. 13 Joint probability density function of the depolarization ratio δ and the ratio of attenuated backscattering coefficients for successive layers x from (a) satellite observations, (b) NSW6 in NICAM.16, and (c) NDW6. Low-level clouds behind a frontal cloud system were sampled over the Southern Ocean. Signals of supercooled liquid water are highlighted by circles. From Roh et al. (Citation2020), © American Meteorological Society. Used with permission.
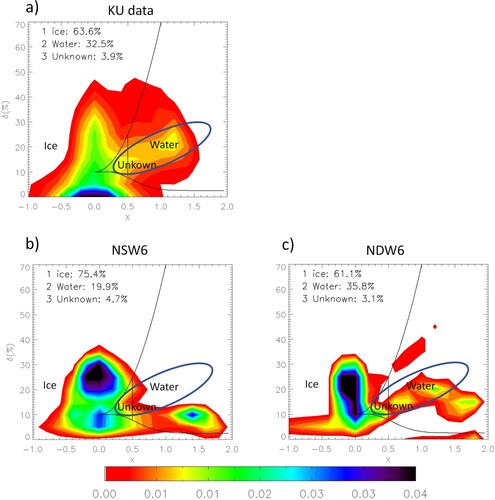
Large particles can be evaluated using cloud radar and precipitation radar. Kikuchi et al. (Citation2017b) proposed a hydrometeor classification method using only CPR radar echo and atmospheric temperature based on the CloudsSat-CALIPSO combined dataset. Recently, hydrometeor classification using GPM-DPR satellite observations has been developed. Snow, rain, and mixed-phase clouds are categorized by using Ku-band radar echo and the dual-frequency ratio (Liao et al., Citation2020; Liao & Meneghini, Citation2011; Citation2016). In addition, the hail detection method was newly proposed by Seiki (Citation2021). Future work should evaluate the microphysics of precipitating hydrometeors using CPR and DPR.
f Challenging issues in satellite simulators
A challenging new satellite project is now underway: The Earth Clouds, Aerosol and Radiation Explorer (EarthCARE, Illingworth et al., Citation2015) satellite, which is a joint mission by the European Space and Japanese Aerospace Exploration Agencies. The EarthCARE satellite has multiple passive and active sensors (): Cloud Profiling Radar (CPR), ATmospheric LIDar (ATLID), Multi Spectral Imager (MSI), and Broad Band Radiometer (BBR). The CPR on EarthCARE has a Doppler capability to provide information on the terminal velocity of rain and ice and convective motions. Multiple sensors have synergy to understand the interaction between clouds and aerosols and to provide new insights into cloud microphysics related to vertical motion for evaluating GCRMs. It is confirmed that the EarthCARE satellite will be launched in 2023.
Fig. 14 Observation geometry of the EarthCARE satellite (from https://www.eorc.jaxa.jp/EARTHCARE/museum/poster_gallary.html, ©JAXA).
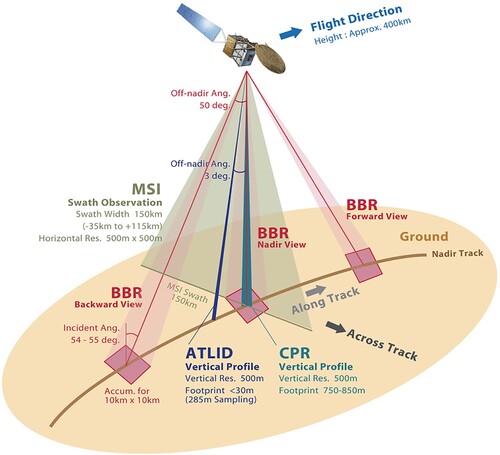
Global cloud resolving models can be used for the observing system simulation experiments (OSSE) to evaluate retrieval algorithms for optical sensors at the planning stage of new satellite projects. A NICAM with a Joint-Simulator was used to create the EarthCARE-like radiances to estimate the accuracy of the retrieved Doppler velocity. In particular, OSSE using the NICAM were helpful to investigate the noise of Doppler velocity of CPR depending on the instrument setting (pulse repetition frequency) before the launch (Hagihara et al., Citation2021).
There are ongoing challenging issues in the development of satellite simulators. First, satellite simulators will be utilized to estimate a range of uncertainties from the observations, as was done by Hagihara et al. (Citation2021). Second, the sensitivity of the nonspherical assumption to cloud representation is also a challenging issue. Several satellite simulators consider the nonspherical shapes of ice from a database using the discrete dipole approximation (DDA) and T-matrix (e.g. Hashino et al., Citation2013; Matsui et al., Citation2019). In addition, the application of depolarization provides insight into nonspherical modelling (Matsui et al., Citation2019). Finally, the 3D radiation of clouds is a long-standing issue (e.g. Benner & Evans, Citation2001; Okata et al., Citation2017), whereas radiative transfer is generally calculated in the vertical dimension. Three-dimensional scattering from cloud sides will be nonnegligible when the horizontal resolution of GCRMs increases to finer than 1 km (global LES). Monte Carlo simulations or other 3D techniques will be introduced into satellite simulators.
g Ground validation for satellites and models
The ULTra-sIte for Measuring Atmosphere of Tokyo metropolitan Environment (ULTIMATE) project has started to validate satellite observations and cloud microphysics schemes in numerical models by using intensive ground observation data in Japan (Satoh et al., Citation2022). The ULTIMATE project obtained several active sensors to detect the vertical distributions of clouds and precipitation. For example, polarimetric Doppler radars were set to derive information about the size distributions, hydrometeor types, and terminal velocity of hydrometeors. In addition, several weather radars (c-band polarimetric radars, x-band phased array polarimetric radar, and w-band cloud radar) work in cooperation around the Tokyo metropolitan area (). Wind profiler network and data acquisition system (WINDAS, Ishihara et al., Citation2006) data are also available to observe the vertical profiles of wind. To utilize these ground radar networks, the POLArimetric Radar Retrieval and Instrument Simulator (POLARRIS, Matsui et al., Citation2019) is newly introduced into the Joint-Simulator. The synergy of intensive ground, remote-sensing observations and satellite data will contribute to the understanding of microphysical processes and improvements of microphysics in GCRMs.
4 Summary
This work reviewed the cloud microphysics in GCRMs and the way to improve cloud microphysics. From the twenty-year history of NICAM development, we recognized that the combined use of multi-optical sensors enables us to constrain uncertain processes in cloud microphysics without artificial tuning. As a result, cloud microphysics schemes used in the NICAM naturally represent cloud systems, and hence, the radiative budget is well balanced with little optimization. On the other hand, some cloud systems have not yet been evaluated because of the diversity of cloud systems in Earth and the limited sensitivity of spaceborne optical sensors. The evaluation method using satellite simulators is underdeveloped, and new satellites will be continuously planned and launched. In addition, intensive ground observation systems and satellite observations are complementary to each other. Thus, a new frontier for cloud microphysics development is still spreading toward the future.
Acknowledgments
The CloudSat and CERES satellite products were obtained from the NASA Langley Research Center Atmospheric Science Data Center. Authors were supported by the Integrated Research Program for Advancing Climate Models (TOUGOU) Grant (JPMXD0717935457) and “Program for Promoting Researches on the Supercomputer Fugaku” (Large Ensemble Atmospheric and Environmental Prediction for Disaster Prevention and Mitigation) (JPMXP1020351142, JPMXP1020200305), which are promoted by the Ministry of Education, Culture, Sports, Science and Technology (MEXT), Japan. Authors were supported by the EarthCARE Program, Earth Observation Research Center of JAXA, and Program for Promoting Technological Development of Transportation (Ministry of Land, Infrastructure, Transport and Tourism of Japan), and Japan Society for the Promotion of Science, Grant-in-Aid for Scientific Research B (JP20H01967) for Ultra Site for Measuring Atmosphere of Tokyo Metropolitan Environment and Collaboration Studies with High-Resolution Atmospheric Models (ULTIMATE).
Disclosure statement
No potential conflict of interest was reported by the author(s).
Additional information
Funding
References
- Ackerman, S. A., & Stephens, G. L. (1987). The absorption of solar radiation by cloud droplets: An application of anomalous diffraction theory. Journal of the Atmospheric Sciences, 44(12), 1574–1588. https://doi.org/10.1175/1520-0469(1987)044<1574:TAOSRB>2.0.CO;2
- Ackerman, T. P., Liou, K.-N., Valero, F. P. J., & Pfister, L. (1988). Heating rates in tropical anvils. Journal of the Atmospheric Sciences, 45(10), 1606–1623. https://doi.org/10.1175/1520-0469(1988)045<1606:HRITA>2.0.CO;2
- Andrews, T., Gregory, J. M., & Webb, M. J. (2015). The dependence of radiative forcing and feedback on evolving patterns of surface temperature change in climate models. Journal of Climate, 28(4), 1630–1648. https://doi.org/10.1175/JCLI-D-14-00545.1
- Arabas, S., Jaruga, A., Pawlowska, H., & Grabowski, W. W. (2015). Libcloudph++ 1.0: A single-moment bulk, double-moment bulk, and particle-based warm-rain microphysics library in C++. Geoscientific Model Development, 8(6), 1677–1707. https://doi.org/10.5194/gmd-8-1677-2015
- Arnold, N. P., Putman, W. M., & Freitas, S. R. (2020). Impact of resolution and parameterized convection on the diurnal cycle of precipitation in a global nonhydrostatic model. Journal of the Meteorological Society of Japan. Ser. II, 98(6), 1279–1304. https://doi.org/10.2151/jmsj.2020-066
- Austin, R. T., Heymsfield, A. J., & Stephens, G. L. (2009). Retrieval of ice cloud microphysical parameters using the CloudSat millimeter-wave radar and temperature. Journal of Geophysical Research: Atmospheres, 114(D8), 1–19. https://doi.org/10.1029/2008JD010049
- Austin, R. T., & Stephens, G. L. (2001). Retrieval of stratus cloud microphysical parameters using millimeter-wave radar and visible optical depth in preparation for CloudSat: 1. Algorithm formulation. Journal of Geophysical Research: Atmospheres, 106(D22), 28233–28242. https://doi.org/10.1029/2000JD000293
- Baldauf, M., Seifert, A., Förstner, J., Majewski, D., Raschendorfer, M., & Reinhardt, T. (2011). Operational convective-scale numerical weather prediction with the COSMO model: description and sensitivities. Monthly Weather Review, 139(12), 3887–3905. https://doi.org/10.1175/MWR-D-10-05013.1
- Bauer, P., Stevens, B., & Hazeleger, W. (2021). A digital twin of earth for the green transition. Nature Climate Change, 11(2), 80–83. https://doi.org/10.1038/s41558-021-00986-y
- Bennartz, R., Shupe, M. D., Turner, D. D., Walden, V. P., Steffen, K., Cox, C. J., Kulie, M. S., Miller, N. B., & Pettersen, C. (2013). July 2012 Greenland melt extent enhanced by low-level liquid clouds. Nature, 496(7443), 83–86. https://doi.org/10.1038/nature12002
- Benner, T. C., & Evans, K. F. (2001). Three-dimensional solar radiative transfer in small tropical cumulus fields derived from high-resolution imagery. Journal of Geophysical Research: Atmospheres, 106(D14), 14975–14984. https://doi.org/10.1029/2001JD900158
- Bigg, E. K. (1953). The formation of atmospheric ice crystals by the freezing of droplets. Quarterly Journal of the Royal Meteorological Society, 79(342), 510–519. https://doi.org/10.1002/qj.49707934207
- Bodas-Salcedo, A., Webb, M. J., Bony, S., Chepfer, H., Dufresne, J.-L., Klein, S. A., Zhang, Y., Marchand, R., Haynes, J. M., Pincus, R., & John, V. O. (2011). COSP: Satellite simulation software for model assessment. Bulletin of the American Meteorological Society, 92(8), 1023–1043. https://doi.org/10.1175/2011BAMS2856.1
- Bony, S., & Dufresne, J.-L. (2005). Marine boundary layer clouds at the heart of tropical cloud feedback uncertainties in climate models. Geophysical Research Letters, 32(20), 1–4. https://doi.org/10.1029/2005GL023851
- Boutle, I. A., Eyre, J. E. J., & Lock, A. P. (2014). Seamless stratocumulus simulation across the turbulent gray zone. Monthly Weather Review, 142(4), 1655–1668. https://doi.org/10.1175/MWR-D-13-00229.1
- Böhm, H. P. (1989). A general equation for the terminal fall speed of solid hydrometeors. Journal of the Atmospheric Sciences, 46(15), 2419–2427. https://doi.org/10.1175/1520-0469(1989)046<2419:AGEFTT>2.0.CO;2
- Böhm, J. P. (1992). A general hydrodynamic theory for mixed-phase microphysics. Part I: Drag and fall speed of hydrometeors. Atmospheric Research, 27(4), 253–274. https://doi.org/10.1016/0169-8095(92)90035-9
- Bu, Y. P., Fovell, R. G., & Corbosiero, K. L. (2014). Influence of cloud–radiative forcing on tropical cyclone structure. Journal of the Atmospheric Sciences, 71(5), 1644–1662. https://doi.org/10.1175/JAS-D-13-0265.1
- Bu, Y. P., Fovell, R. G., & Corbosiero, K. L. (2017). The influences of boundary layer mixing and cloud-radiative forcing on tropical cyclone size. Journal of the Atmospheric Sciences, 74(4), 1273–1292. https://doi.org/10.1175/JAS-D-16-0231.1
- Bubnová, R., Hello, G., Bénard, P., & Geleyn, J.-F. (1995). Integration of the fully elastic equations cast in the hydrostatic pressure terrain-following coordinate in the framework of the ARPEGE/aladin NWP system. Monthly Weather Review, 123(2), 515–535. https://doi.org/10.1175/1520-0493(1995)123<0515:IOTFEE>2.0.CO;2
- Cesana, G., & Chepfer, H. (2012). How well do climate models simulate cloud vertical structure? A comparison between CALIPSO-GOCCP satellite observations and CMIP5 models. Geophysical Research Letters, 39(20), 1–6. https://doi.org/10.1029/2012GL053153
- Cesana, G., & Chepfer, H. (2013). Evaluation of the cloud thermodynamic phase in a climate model using CALIPSO-GOCCP. Journal of Geophysical Research: Atmospheres, 118(14), 7922–7937. https://doi.org/10.1002/jgrd.50376
- Cesana, G., Waliser, D. E., Jiang, X., & Li, J.-L. F. (2015). Multimodel evaluation of cloud phase transition using satellite and reanalysis data. Journal of Geophysical Research: Atmospheres, 120(15), 7871–7892. https://doi.org/10.1002/2014JD022932
- Chang, P., Zhang, S., Danabasoglu, G., Yeager, S. G., Fu, H., Wang, H., Castruccio, F. S., Chen, Y., Edwards, J., Fu, D., Jia, Y., Laurindo, L. C., Liu, X., Rosenbloom, N., Small, R. J., Xu, G., Zeng, Y., Zhang, Q., Bacmeister, J., … Wu, L. (2020). An unprecedented Set of high-resolution earth system simulations for understanding multiscale interactions in climate variability and change. Journal of Advances in Modeling Earth Systems, 12(12), 1–52. https://doi.org/10.1029/2020MS002298
- Chen, Y.-W., Seiki, T., Kodama, C., Satoh, M., & Noda, A. T. (2018). Impact of precipitating Ice hydrometeors on longwave radiative effect estimated by a global cloud-system resolving model. Journal of Advances in Modeling Earth Systems, 10(2), 284–296. https://doi.org/10.1002/2017MS001180
- Chen, Y., Weng, F., Han, Y., & Liu, Q. (2008). Validation of the community radiative transfer model by using CloudSat data. Journal of Geophysical Research: Atmospheres, 113(D8), 1–15. https://doi.org/10.1029/2007JD009561
- Chepfer, H., Bony, S., Winker, D., Cesana, G., Dufresne, J. L., Minnis, P., Stubenrauch, C. J., & Zeng, S. (2010). The GCM-Oriented CALIPSO Cloud Product (CALIPSO-GOCCP). Journal of Geophysical Research: Atmospheres, 115(D4), 1–13. https://doi.org/10.1029/2009JD012251
- Curry, J. A., Schramm, J. L., & Ebert, E. E. (1993). Impact of clouds on the surface radiation balance of the Arctic ocean. Meteorology and Atmospheric Physics, 51(3), 197–217. https://doi.org/10.1007/BF01030494
- Davies, T., Cullen, M. J. P., Malcolm, A. J., Mawson, M. H., Staniforth, A., White, A. A., & Wood, N. (2005). A new dynamical core for the Met office’s global and regional modelling of the atmosphere. Quarterly Journal of the Royal Meteorological Society, 131(608), 1759–1782. https://doi.org/10.1256/qj.04.101
- DeMott, P. J., Cziczo, D. J., Prenni, A. J., Murphy, D. M., Kreidenweis, S. M., Thomson, D. S., Borys, R., & Rogers, D. C. (2003). Measurements of the concentration and composition of nuclei for cirrus formation. Proceedings of the National Academy of Sciences, 100(25), 14655–14660. https://doi.org/10.1073/pnas.2532677100
- Ding, S., Yang, P., Weng, F., Liu, Q., Han, Y., van Delst, P., Li, J., & Baum, B. (2011). Validation of the community radiative transfer model. Journal of Quantitative Spectroscopy and Radiative Transfer, 112(6), 1050–1064. https://doi.org/10.1016/j.jqsrt.2010.11.009
- Dueben, P. D., Wedi, N., Saarinen, S., & Zeman, C. (2020). Global simulations of the Atmosphere at 1.45 km grid-spacing with the integrated forecasting system. Journal of the Meteorological Society of Japan. Ser. II, 98(3), 551–572. https://doi.org/10.2151/jmsj.2020-016
- Elsaesser, G. S., O’Dell, C. W., Lebsock, M. D., Bennartz, R., Greenwald, T. J., & Wentz, F. J. (2017). The multisensor advanced climatology of liquid water path (MAC-LWP). Journal of Climate, 30(24), 10193–10210. https://doi.org/10.1175/JCLI-D-16-0902.1
- Engdahl, B. J. K., Thompson, G., & Bengtsson, L. (2020). Improving the representation of supercooled liquid water in the HARMONIE-AROME weather forecast model. Tellus A: Dynamic Meteorology and Oceanography, 72(1), 1–18. https://doi.org/10.1080/16000870.2019.1697603
- Field, P. R., Hogan, R. J., Brown, P. R. A., Illingworth, A. J., Choularton, T. W., & Cotton, R. J. (2005). Parametrization of ice-particle size distributions for mid-latitude stratiform cloud. Quarterly Journal of the Royal Meteorological Society, 131(609), 1997–2017. https://doi.org/10.1256/qj.04.134
- Forbes, R., Tompkins, A. M., & Untch, A. (2011). A new prognostic bulk microphysics scheme for the IFS. ECMWF. https://doi.org/10.21957/bf6vjvxk
- Fovell, R. G., Corbosiero, K. L., Seifert, A., & Liou, K.-N. (2010). Impact of cloud-radiative processes on hurricane track. Geophysical Research Letters, 37(7), 1–6. https://doi.org/10.1029/2010GL042691
- Francis, J. A., Hunter, E., Key, J. R., & Wang, X. (2005). Clues to variability in Arctic minimum sea ice extent. Geophysical Research Letters, 32(21), 1–4. https://doi.org/10.1029/2005GL024376
- Fu, Q. (1996). An accurate parameterization of the solar radiative properties of cirrus clouds for climate models. Journal of Climate, 9(9), 2058–2082. https://doi.org/10.1175/1520-0442(1996)009<2058:AAPOTS>2.0.CO;2
- Fu, Q. (2007). A New Parameterization of an asymmetry factor of cirrus clouds for climate models. Journal of the Atmospheric Sciences, 64(11), 4140–4150. https://doi.org/10.1175/2007JAS2289.1
- Fu, Q., & Liou, K. N. (1993). Parameterization of the Radiative Properties of Cirrus clouds. Journal of the Atmospheric Sciences, 50(13), 2008–2025. https://doi.org/10.1175/1520-0469(1993)050<2008:POTRPO>2.0.CO;2
- Fu, Q., Yang, P., & Sun, W. B. (1998). An Accurate Parameterization of the infrared radiative properties of cirrus clouds for climate models. Journal of Climate, 11(9), 2223–2237. https://doi.org/10.1175/1520-0442(1998)011<2223:AAPOTI>2.0.CO;2
- Fudeyasu, H., Wang, Y., Satoh, M., Nasuno, T., Miura, H., & Yanase, W. (2008). Global cloud-system-resolving model NICAM successfully simulated the lifecycles of two real tropical cyclones. Geophysical Research Letters, 35(22), 1–6. https://doi.org/10.1029/2008GL036003
- Fukuta, N., & Schaller, R. C. (1982). Ice nucleation by Aerosol particles. Theory of condensation-freezing nucleation. Journal of the Atmospheric Sciences, 39(3), 648–655. https://doi.org/10.1175/1520-0469(1982)039<0648:INBAPT>2.0.CO;2
- Furtado, K., & Field, P. (2017). The role of Ice microphysics parametrizations in Determining the prevalence of supercooled liquid water in high-resolution simulations of a Southern Ocean Midlatitude cyclone. Journal of the Atmospheric Sciences, 74(6), 2001–2021. https://doi.org/10.1175/JAS-D-16-0165.1
- Gettelman, A., Liu, X., Ghan, S. J., Morrison, H., Park, S., Conley, A. J., Klein, S. A., Boyle, J., Mitchell, D. L., & Li, J.-L. F. (2010). Global simulations of ice nucleation and ice supersaturation with an improved cloud scheme in the Community Atmosphere model. Journal of Geophysical Research: Atmospheres, 115(D18), 1–19. https://doi.org/10.1029/2009JD013797
- Gettelman, A., & Morrison, H. (2015). Advanced Two-Moment Bulk Microphysics for Global Models. Part I: Off-line Tests and comparison with other schemes. Journal of Climate, 28(3), 1268–1287. https://doi.org/10.1175/JCLI-D-14-00102.1
- Gilmore, M. S., Straka, J. M., & Rasmussen, E. N. (2004). Precipitation uncertainty Due to Variations in Precipitation Particle parameters within a Simple microphysics scheme. Monthly Weather Review, 132(11), 2610–2627. https://doi.org/10.1175/MWR2810.1
- Grabowski, W. W. (1998). Toward cloud Resolving Modeling of large-scale tropical circulations: A Simple cloud microphysics parameterization. Journal of the Atmospheric Sciences, 55(21), 3283–3298. https://doi.org/10.1175/1520-0469(1998)055<3283:TCRMOL>2.0.CO;2
- Haarsma, R. J., Roberts, M. J., Vidale, P. L., Senior, C. A., Bellucci, A., Bao, Q., Chang, P., Corti, S., Fučkar, N. S., Guemas, V., von Hardenberg, J., Hazeleger, W., Kodama, C., Koenigk, T., Leung, L. R., Lu, J., Luo, J.-J., Mao, J., Mizielinski, M. S., … von Storch, J.-S. (2016). High resolution model intercomparison project (HighResMIP v1.0) for CMIP6. Geoscientific Model Development, 9(11), 4185–4208. https://doi.org/10.5194/gmd-9-4185-2016
- Hagihara, Y., Ohno, Y., Horie, H., Roh, W., Satoh, M., Kubota, T., & Oki, R. (2021). Assessments of Doppler velocity errors of EarthCARE cloud profiling radar using global cloud system resolving simulations: Effects of Doppler broadening and folding. IEEE Transactions on Geoscience and Remote Sensing, 60, 1–9. https://doi.org/10.1109/TGRS.2021.3060828
- Hagihara, Y., Okamoto, H., & Luo, Z. J. (2014). Joint analysis of cloud top heights from CloudSat and CALIPSO: New insights into cloud top microphysics. Journal of Geophysical Research: Atmospheres, 119(7), 4087–4106. https://doi.org/10.1002/2013JD020919
- Hagihara, Y., Okamoto, H., & Yoshida, R. (2010). Development of a combined CloudSat-CALIPSO cloud mask to show global cloud distribution. Journal of Geophysical Research: Atmospheres, 115(D4), 1–17. https://doi.org/10.1029/2009JD012344
- Haladay, T., & Stephens, G. (2009). Characteristics of tropical thin cirrus clouds deduced from joint CloudSat and CALIPSO observations. Journal of Geophysical Research: Atmospheres, 114(D8), 1–13. https://doi.org/10.1029/2008JD010675
- Hall, A., Cox, P., Huntingford, C., & Klein, S. (2019). Progressing emergent constraints on future climate change. Nature Climate Change, 9(4), 269–278. https://doi.org/10.1038/s41558-019-0436-6
- Hansen, J. E., & Travis, L. D. (1974). Light scattering in planetary atmospheres. Space Science Reviews, 16(4), 527–610. https://doi.org/10.1007/BF00168069
- Harris, L. M., Rees, S. L., Morin, M., Zhou, L., & Stern, W. F. (2019). Explicit prediction of continental Convection in a skillful variable-resolution global model. Journal of Advances in Modeling Earth Systems, 11(6), 1847–1869. https://doi.org/10.1029/2018MS001542
- Harris, L., Zhou, L., Lin, S.-J., Chen, J.-H., Chen, X., Gao, K., Morin, M., Rees, S., Sun, Y., Tong, M., Xiang, B., Bender, M., Benson, R., Cheng, K.-Y., Clark, S., Elbert, O. D., Hazelton, A., Huff, J. J., Kaltenbaugh, A., … Stern, W. (2020). GFDL SHiELD: A Unified system for weather-to-seasonal prediction. Journal of Advances in Modeling Earth Systems, 12(10), 1–25. https://doi.org/10.1029/2020MS002223
- Hartmann, D. L., & Larson, K. (2002). An important constraint on tropical cloud—climate feedback. Geophysical Research Letters, 29(20), 12-1–12-14. https://doi.org/10.1029/2002GL015835
- Hartmann, D. L., Ockert-Bell, M. E., & Michelsen, M. L. (1992). The effect of cloud type on Earth’s energy balance: Global analysis. Journal of Climate, 5(11), 1281–1304. https://doi.org/10.1175/1520-0442(1992)005<1281:TEOCTO>2.0.CO;2
- Hashino, T., Satoh, M., Hagihara, Y., Kato, S., Kubota, T., Matsui, T., Nasuno, T., Okamoto, H., & Sekiguchi, M. (2016). Evaluating Arctic cloud radiative effects simulated by NICAM with A-train. Journal of Geophysical Research: Atmospheres, 121(12), 7041–7063. https://doi.org/10.1002/2016JD024775
- Hashino, T., Satoh, M., Hagihara, Y., Kubota, T., Matsui, T., Nasuno, T., & Okamoto, H. (2013). Evaluating cloud microphysics from NICAM against CloudSat and CALIPSO. Journal of Geophysical Research: Atmospheres, 118(13), 7273–7292. https://doi.org/10.1002/jgrd.50564
- Herring, S. C., Christidis, N., Hoell, A., Hoerling, M. P., & Stott, P. A. (2019). Explaining Extreme Events of 2017 from a Climate perspective. Bulletin of the American Meteorological Society, 100(1), S1–S117. https://doi.org/10.1175/BAMS-ExplainingExtremeEvents2017.1
- Heymsfield, A. (1975). Cirrus Uncinus Generating cells and the Evolution of cirriform clouds. Part II: The structure and Circulations of the Cirrus Uncinus Generating head. Journal of the Atmospheric Sciences, 32(4), 809–819. https://doi.org/10.1175/1520-0469(1975)032<0809:CUGCAT>2.0.CO;2
- Hohenegger, C., Kornblueh, L., Klocke, D., Becker, T., Cioni, G., Engels, J. F., Schulzweida, U., & Stevens, B. (2020). Climate Statistics in Global simulations of the atmosphere, from 80 to 2.5 km grid spacing. Journal of the Meteorological Society of Japan. Ser. II, 98(1), 73–91. https://doi.org/10.2151/jmsj.2020-005
- Hotta, H., Suzuki, K., Goto, D., & Lebsock, M. (2020). Climate Impact of cloud water inhomogeneity through microphysical processes in a Global Climate model. Journal of Climate, 33(12), 5195–5212. https://doi.org/10.1175/JCLI-D-19-0772.1
- Hou, A. Y., Kakar, R. K., Neeck, S., Azarbarzin, A. A., Kummerow, C. D., Kojima, M., Oki, R., Nakamura, K., & Iguchi, T. (2014). The Global Precipitation Measurement mission. Bulletin of the American Meteorological Society, 95(5), 701–722. https://doi.org/10.1175/BAMS-D-13-00164.1
- Hu, Y., Rodier, S., Xu, K., Sun, W., Huang, J., Lin, B., Zhai, P., & Josset, D. (2010). Occurrence, liquid water content, and fraction of supercooled water clouds from combined CALIOP/IIR/MODIS measurements. Journal of Geophysical Research: Atmospheres, 115(D4), 1–13. https://doi.org/10.1029/2009JD012384
- Hu, Y., Winker, D., Vaughan, M., Lin, B., Omar, A., Trepte, C., Flittner, D., Yang, P., Nasiri, S. L., Baum, B., Holz, R., Sun, W., Liu, Z., Wang, Z., Young, S., Stamnes, K., Huang, J., & Kuehn, R. (2009). CALIPSO/CALIOP cloud phase discrimination algorithm. Journal of Atmospheric and Oceanic Technology, 26(11), 2293–2309. https://doi.org/10.1175/2009JTECHA1280.1
- Hyder, P., Edwards, J. M., Allan, R. P., Hewitt, H. T., Bracegirdle, T. J., Gregory, J. M., Wood, R. A., Meijers, A. J. S., Mulcahy, J., Field, P., Furtado, K., Bodas-Salcedo, A., Williams, K. D., Copsey, D., Josey, S. A., Liu, C., Roberts, C. D., Sanchez, C., Ridley, J., … Belcher, S. E. (2018). Critical Southern Ocean climate model biases traced to atmospheric model cloud errors. Nature Communications, 9(1), 1–17. https://doi.org/10.1038/s41467-018-05634-2
- Iga, S., Tomita, H., Tsushima, Y., & Satoh, M. (2007). Climatology of a nonhydrostatic global model with explicit cloud processes. Geophysical Research Letters, 34(22), 1–6. https://doi.org/10.1029/2007GL031048
- Igel, A. L., Igel, M. R., & Heever, S. C. v. d. (2015). Make It a double? Sobering results from simulations using single-moment microphysics schemes. Journal of the Atmospheric Sciences, 72(2), 910–925. https://doi.org/10.1175/JAS-D-14-0107.1
- Iguchi, T., Kozu, T., Kwiatkowski, J., Meneghini, R., Awaka, J., & Okamoto, K. (2009). Uncertainties in the rain Profiling Algorithm for the TRMM Precipitation radar. Journal of the Meteorological Society of Japan. Ser. II, 87A, 1–30. https://doi.org/10.2151/jmsj.87A.1
- Illingworth, A. J., Barker, H. W., Beljaars, A., Ceccaldi, M., Chepfer, H., Clerbaux, N., Cole, J., Delanoë, J., Domenech, C., Donovan, D. P., Fukuda, S., Hirakata, M., Hogan, R. J., Huenerbein, A., Kollias, P., Kubota, T., Nakajima, T., Nakajima, T. Y., Nishizawa, T., … Zadelhoff, G.-J. v. (2015). The EarthCARE satellite: The Next Step forward in Global measurements of clouds, aerosols, precipitation, and radiation. Bulletin of the American Meteorological Society, 96(8), 1311–1332. https://doi.org/10.1175/BAMS-D-12-00227.1
- Inoue, T. (1987). A cloud type classification with NOAA 7 split-window measurements. Journal of Geophysical Research: Atmospheres, 92(D4), 3991–4000. https://doi.org/10.1029/JD092iD04p03991
- Inoue, T., & Ackerman, S. A. (2002). Radiative effects of various cloud types as classified by the Split Window technique over the eastern Sub-tropical Pacific derived from collocated ERBE and AVHRR data. Journal of the Meteorological Society of Japan. Ser. II, 80(6), 1383–1394. https://doi.org/10.2151/jmsj.80.1383
- Inoue, T., Satoh, M., Hagihara, Y., Miura, H., & Schmetz, J. (2010). Comparison of high-level clouds represented in a global cloud system–resolving model with CALIPSO/CloudSat and geostationary satellite observations. Journal of Geophysical Research: Atmospheres, 115(D4), 1–15. https://doi.org/10.1029/2009JD012371
- Inoue, T., Satoh, M., Miura, H., & Mapes, B. (2008). Characteristics of cloud size of Deep Convection Simulated by a Global cloud Resolving Model over the western tropical pacific. Journal of the Meteorological Society of Japan. Ser. II, 86A, 1–15. https://doi.org/10.2151/jmsj.86A.1
- Inoue, T., Ueda, H., & Inoue, T. (2006). Cloud Properties over the Bay of Bengal derived from NOAA-9 Split Window data and the TRMM PR product. Sola, 2, 41–44. https://doi.org/10.2151/sola.2006-011
- Ishihara, M., Kato, Y., Abo, T., Kobayashi, K., & Izumikawa, Y. (2006). Characteristics and performance of the Operational wind profiler network of the Japan Meteorological agency. Journal of the Meteorological Society of Japan. Ser. II, 84(6), 1085–1096. https://doi.org/10.2151/jmsj.84.1085
- Iwabuchi, H., Putri, N. S., Saito, M., Tokoro, Y., Sekiguchi, M., Yang, P., & Baum, B. A. (2018). Cloud property Retrieval from multiband Infrared measurements by himawari-8. Journal of the Meteorological Society of Japan. Ser. II, 96B(0), 27–42. https://doi.org/10.2151/jmsj.2018-001
- Iwabuchi, H., Saito, M., Tokoro, Y., Putri, N. S., & Sekiguchi, M. (2016). Retrieval of radiative and microphysical properties of clouds from multispectral infrared measurements. Progress in Earth and Planetary Science, 3(1), 1–18. https://doi.org/10.1186/s40645-016-0108-3
- Jaruga, A., & Pawlowska, H. (2018). Libcloudph++ 2.0: Aqueous-phase chemistry extension of the particle-based cloud microphysics scheme. Geoscientific Model Development, 11(9), 3623–3645. https://doi.org/10.5194/gmd-11-3623-2018
- Jensen, E. J., Kinne, S., & Toon, O. B. (1994). Tropical cirrus cloud radiative forcing: Sensitivity studies. Geophysical Research Letters, 21(18), 2023–2026. https://doi.org/10.1029/94GL01358
- Kajikawa, Y., Miyamoto, Y., Yoshida, R., Yamaura, T., Yashiro, H., & Tomita, H. (2016). Resolution dependence of deep convections in a global simulation from over 10-kilometer to sub-kilometer grid spacing. Progress in Earth and Planetary Science, 3(1), 1–14. https://doi.org/10.1186/s40645-016-0094-5
- Kalnay, E., Kanamitsu, M., & Baker, W. E. (1990). Global Numerical Weather prediction at the national Meteorological center. Bulletin of the American Meteorological Society, 71(10), 1410–1428. https://doi.org/10.1175/1520-0477(1990)071<1410:GNWPAT>2.0.CO;2
- Kang, S. M., Frierson, D. M. W., & Held, I. M. (2009). The tropical response to extratropical thermal forcing in an idealized GCM: The Importance of radiative feedbacks and convective parameterization. Journal of the Atmospheric Sciences, 66(9), 2812–2827. https://doi.org/10.1175/2009JAS2924.1
- Kapsch, M.-L., Graversen, R. G., & Tjernström, M. (2013). Springtime atmospheric energy transport and the control of Arctic summer sea-ice extent. Nature Climate Change, 3(8), 744–748. https://doi.org/10.1038/nclimate1884
- Kapsch, M.-L., Graversen, R. G., Tjernström, M., & Bintanja, R. (2016). The effect of downwelling longwave and shortwave radiation on Arctic Summer Sea Ice. Journal of Climate, 29(3), 1143–1159. https://doi.org/10.1175/JCLI-D-15-0238.1
- Kato, T., & Saito, K. (1995). Hydrostatic and Non-hydrostatic simulations of Moist convection. Journal of the Meteorological Society of Japan. Ser. II, 73(1), 59–77. https://doi.org/10.2151/jmsj1965.73.1_59
- Kawai, H., & Teixeira, J. (2010). Probability Density Functions of Liquid Water Path and cloud amount of Marine Boundary Layer Clouds: Geographical and seasonal Variations and controlling Meteorological factors. Journal of Climate, 23(8), 2079–2092. https://doi.org/10.1175/2009JCLI3070.1
- Kawai, H., & Teixeira, J. (2012). Probability Density Functions of Liquid Water Path and total water content of Marine Boundary Layer clouds: Implications for cloud parameterization. Journal of Climate, 25(6), 2162–2177. https://doi.org/10.1175/JCLI-D-11-00117.1
- Kay, J. E., Wall, C., Yettella, V., Medeiros, B., Hannay, C., Caldwell, P., & Bitz, C. (2016). Global Climate impacts of fixing the Southern Ocean shortwave radiation bias in the Community Earth System Model (CESM). Journal of Climate, 29(12), 4617–4636. https://doi.org/10.1175/JCLI-D-15-0358.1
- Kärcher, B., Hendricks, J., & Lohmann, U. (2006). Physically based parameterization of cirrus cloud formation for use in global atmospheric models. Journal of Geophysical Research: Atmospheres, 111(D1), 1–11. https://doi.org/10.1029/2005JD006219
- Khairoutdinov, M. F., & Randall, D. A. (2003). Cloud Resolving Modeling of the ARM Summer 1997 IOP: Model formulation, results, uncertainties, and sensitivities. Journal of the Atmospheric Sciences, 60(4), 607–625. https://doi.org/10.1175/1520-0469(2003)060<0607:CRMOTA>2.0.CO;2
- Khairoutdinov, M., & Kogan, Y. (2000). A New cloud physics Parameterization in a large-eddy simulation Model of Marine stratocumulus. Monthly Weather Review, 128(1), 229–243. https://doi.org/10.1175/1520-0493(2000)128<0229:ANCPPI>2.0.CO;2
- Khvorostyanov, V. I., & Curry, J. A. (2009). Critical humidities of homogeneous and heterogeneous ice nucleation: Inferences from extended classical nucleation theory. Journal of Geophysical Research: Atmospheres, 114(D4), 1–16. https://doi.org/10.1029/2008JD011197
- Kikuchi, K., Kodama, C., Nasuno, T., Nakano, M., Miura, H., Satoh, M., Noda, A. T., & Yamada, Y. (2017a). Tropical intraseasonal oscillation simulated in an AMIP-type experiment by NICAM. Climate Dynamics, 48(7), 2507–2528. https://doi.org/10.1007/s00382-016-3219-z
- Kikuchi, M., Okamoto, H., Sato, K., Suzuki, K., Cesana, G., Hagihara, Y., Takahashi, N., Hayasaka, T., & Oki, R. (2017b). Development of Algorithm for discriminating hydrometeor particle types With a synergistic Use of CloudSat and CALIPSO. Journal of Geophysical Research: Atmospheres, 122(20), 11,022–11,044. https://doi.org/10.1002/2017JD027113
- Kodama, C., Noda, A. T., & Satoh, M. (2012). An assessment of the cloud signals simulated by NICAM using ISCCP, CALIPSO, and CloudSat satellite simulators. Journal of Geophysical Research: Atmospheres, 117(D12), 1–17. https://doi.org/10.1029/2011JD017317
- Kodama, C., Ohno, T., Seiki, T., Yashiro, H., Noda, A. T., Nakano, M., Yamada, Y., Roh, W., Satoh, M., Nitta, T., Goto, D., Miura, H., Nasuno, T., Miyakawa, T., Chen, Y.-W., & Sugi, M. (2021). The Nonhydrostatic ICosahedral Atmospheric Model for CMIP6 HighResMIP simulations (NICAM16-S): experimental design, model description, and impacts of model updates. Geoscientific Model Development, 14(2), 795–820. https://doi.org/10.5194/gmd-14-795-2021
- Kodama, C., Yamada, Y., Noda, A. T., Kikuchi, K., Kajikawa, Y., Nasuno, T., Tomita, T., Yamaura, T., Takahashi, H. G., Hara, M., Kawatani, Y., Satoh, M., & Sugi, M. (2015). A 20-year Climatology of a NICAM AMIP-type simulation. Journal of the Meteorological Society of Japan. Ser. II, 93(4), 393–424. https://doi.org/10.2151/jmsj.2015-024
- Kondo, M., Sato, Y., Inatsu, M., & Katsuyama, Y. (2021). Evaluation of cloud Microphysical Schemes for Winter snowfall Events in hokkaido: A case Study of snowfall by Winter monsoon. Sola, 17(0), 74–80. https://doi.org/10.2151/sola.2021-012
- Kuba, N., Seiki, T., Suzuki, K., Roh, W., & Satoh, M. (2020). Evaluation of rain microphysics using a radar simulator and Numerical models: Comparison of Two-Moment bulk and spectral Bin cloud microphysics schemes. Journal of Advances in Modeling Earth Systems, 12(3), 1–18. https://doi.org/10.1029/2019MS001891
- Kuba, N., Suzuki, K., Hashino, T., Seiki, T., & Satoh, M. (2015). Numerical experiments to analyze cloud microphysical processes depicted in vertical profiles of radar reflectivity of Warm clouds. Journal of the Atmospheric Sciences, 72(12), 4509–4528. https://doi.org/10.1175/JAS-D-15-0053.1
- Lang, S., Tao, W.-K., Simpson, J., Cifelli, R., Rutledge, S., Olson, W., & Halverson, J. (2007). Improving simulations of convective systems from TRMM LBA: Easterly and westerly regimes. Journal of the Atmospheric Sciences, 64(4), 1141–1164. https://doi.org/10.1175/JAS3879.1
- Li, J.-L. F., Forbes, R. M., Waliser, D. E., Stephens, G., & Lee, S. (2014). Characterizing the radiative impacts of precipitating snow in the ECMWF Integrated forecast system global model. Journal of Geophysical Research: Atmospheres, 119(16), 9626–9637. https://doi.org/10.1002/2014JD021450
- Li, J.-L. F., Lee, W.-L., Waliser, D., Wang, Y.-H., Yu, J.-Y., Jiang, X., L’Ecuyer, T., Chen, Y.-C., Kubar, T., Fetzer, E., & Mahakur, M. (2016). Considering the radiative effects of snow on tropical Pacific Ocean radiative heating profiles in contemporary GCMs using A-train observations. Journal of Geophysical Research: Atmospheres, 121(4), 1621–1636. https://doi.org/10.1002/2015JD023587
- Li, J.-L. F., Waliser, D. E., Stephens, G., Lee, S., L’Ecuyer, T., Kato, S., Loeb, N., & Ma, H.-Y. (2013). Characterizing and understanding radiation budget biases in CMIP3/CMIP5 GCMs, contemporary GCM, and reanalysis. Journal of Geophysical Research: Atmospheres, 118(15), 8166–8184. https://doi.org/10.1002/jgrd.50378
- Li, X., Tao, W.-K., Matsui, T., Liu, C., & Masunaga, H. (2010). Improving a spectral bin microphysical scheme using TRMM satellite observations. Quarterly Journal of the Royal Meteorological Society, 136(647), 382–399. https://doi.org/10.1002/qj.569
- Liao, L., & Meneghini, R. (2011). A Study on the feasibility of Dual-Wavelength radar for identification of hydrometeor phases. Journal of Applied Meteorology and Climatology, 50(2), 449–456. https://doi.org/10.1175/2010JAMC2499.1
- Liao, L., & Meneghini, R. (2016). A Dual-Wavelength Radar technique to detect hydrometeor phases. IEEE Transactions on Geoscience and Remote Sensing, 54(12), 7292–7298. https://doi.org/10.1109/TGRS.2016.2599022
- Liao, L., Meneghini, R., Tokay, A., & Kim, H. (2020). Assessment of Ku- and Ka-band dual-frequency radar for snow retrieval. Journal of the Meteorological Society of Japan. Ser. II, 98(6), 1129–1146. https://doi.org/10.2151/jmsj.2020-057
- Lin, S.-J. (2004). A “Vertically lagrangian” Finite-Volume dynamical core for Global models. Monthly Weather Review, 132(10), 2293–2307. https://doi.org/10.1175/1520-0493(2004)132<2293:AVLFDC>2.0.CO;2
- Lin, S.-J., & Rood, R. B. (1997). An explicit flux-form semi-lagrangian shallow-water model on the sphere. Quarterly Journal of the Royal Meteorological Society, 123(544), 2477–2498. https://doi.org/10.1002/qj.49712354416
- Lin, Y.-L., Farley, R. D., & Orville, H. D. (1983). Bulk Parameterization of the snow field in a cloud model. Journal of Applied Meteorology and Climatology, 22(6), 1065–1092. https://doi.org/10.1175/1520-0450(1983)022<1065:BPOTSF>2.0.CO;2
- Liou, K.-N. (1986). Influence of cirrus clouds on Weather and Climate processes: A Global perspective. Monthly Weather Review, 114(6), 1167–1199. https://doi.org/10.1175/1520-0493(1986)114<1167:IOCCOW>2.0.CO;2
- Liou, K. N. (2002). An introduction to Atmospheric radiation. Elsevier.
- Locatelli, J. D., & Hobbs, P. V. (1974). Fall speeds and masses of solid precipitation particles. Journal of Geophysical Research (1896-1977), 79(15), 2185–2197. https://doi.org/10.1029/JC079i015p02185
- Long, A. B. (1974). Solutions to the droplet collection equation for polynomial kernels. Journal of the Atmospheric Sciences, 31(4), 1040–1052. https://doi.org/10.1175/1520-0469(1974)031<1040:STTDCE>2.0.CO;2
- Lopez, P. (2002). Implementation and validation of a new prognostic large-scale cloud and precipitation scheme for climate and data-assimilation purposes. Quarterly Journal of the Royal Meteorological Society, 128(579), 229–257. https://doi.org/10.1256/00359000260498879
- Macke, A. (1993). Scattering of light by polyhedral ice crystals. Applied Optics, 32(15), 2780–2788. https://doi.org/10.1364/AO.32.002780
- Malardel, S., Wedi, N., Deconinck, W., Diamantakis, M., Kuehnlein, C., Mozdzynski, G., Hamrud, M., & Smolarkiewicz, P. (2016). A new grid for the IFS. ECMWF. https://doi.org/10.21957/zwdu9u5i
- Mapes, B. E., & Houze, R. A. (1993). Cloud clusters and superclusters over the Oceanic Warm pool. Monthly Weather Review, 121(5), 1398–1416. https://doi.org/10.1175/1520-0493(1993)121<1398:CCASOT>2.0.CO;2
- Marchand, R., Haynes, J., Mace, G. G., Ackerman, T., & Stephens, G. (2009). A comparison of simulated cloud radar output from the multiscale modeling framework global climate model with CloudSat cloud radar observations. Journal of Geophysical Research: Atmospheres, 114(D8), 1–18. https://doi.org/10.1029/2008JD009790
- Masunaga, H., & Bony, S. (2018). Radiative invigoration of Tropical Convection by preceding cirrus clouds. Journal of the Atmospheric Sciences, 75(4), 1327–1342. https://doi.org/10.1175/JAS-D-17-0355.1
- Masunaga, H., & Kummerow, C. D. (2006). Observations of tropical precipitating clouds ranging from shallow to deep convective systems. Geophysical Research Letters, 33(16), 1–5. https://doi.org/10.1029/2006GL026547
- Masunaga, H., L’Ecuyer, T. S., & Kummerow, C. D. (2005). Variability in the Characteristics of Precipitation systems in the tropical pacific. Part I: Spatial structure. Journal of Climate, 18(6), 823–840. https://doi.org/10.1175/JCLI-3304.1
- Masunaga, H., & Mapes, B. E. (2020). A Mechanism for the maintenance of sharp tropical margins. Journal of the Atmospheric Sciences, 77(4), 1181–1197. https://doi.org/10.1175/JAS-D-19-0154.1
- Masunaga, H., Matsui, T., Tao, W., Hou, A. Y., Kummerow, C. D., Nakajima, T., Bauer, P., Olson, W. S., Sekiguchi, M., & Nakajima, T. Y. (2010). Satellite Data Simulator Unit: a multisensor, multispectral satellite simulator package. Bulletin of the American Meteorological Society, 91(12), 1625–1632. https://doi.org/10.1175/2010BAMS2809.1
- Masunaga, H., Satoh, M., & Miura, H. (2008). A joint satellite and global cloud-resolving model analysis of a Madden-Julian Oscillation event: Model diagnosis. Journal of Geophysical Research: Atmospheres, 113(D17), 1–11. https://doi.org/10.1029/2008JD009986
- Matsui, T., Chern, J.-D., Tao, W.-K., Lang, S., Satoh, M., Hashino, T., & Kubota, T. (2016). On the land–Ocean contrast of Tropical Convection and microphysics Statistics derived from TRMM satellite signals and Global Storm-Resolving models. Journal of Hydrometeorology, 17(5), 1425–1445. https://doi.org/10.1175/JHM-D-15-0111.1
- Matsui, T., Dolan, B., Rutledge, S. A., Tao, W.-K., Iguchi, T., Barnum, J., & Lang, S. E. (2019). POLARRIS: A POLArimetric Radar Retrieval and Instrument simulator. Journal of Geophysical Research: Atmospheres, 124(8), 4634–4657. https://doi.org/10.1029/2018JD028317
- Matsui, T., Iguchi, T., Li, X., Han, M., Tao, W.-K., Petersen, W., L’Ecuyer, T., Meneghini, R., Olson, W., Kummerow, C. D., Hou, A. Y., Schwaller, M. R., Stocker, E. F., & Kwiatkowski, J. (2013). GPM satellite simulator over ground validation sites. Bulletin of the American Meteorological Society, 94(11), 1653–1660. https://doi.org/10.1175/BAMS-D-12-00160.1
- Matsui, T., Santanello, J., Shi, J. J., Tao, W.-K., Wu, D., Peters-Lidard, C., Kemp, E., Chin, M., Starr, D., Sekiguchi, M., & Aires, F. (2014). Introducing multisensor satellite radiance-based evaluation for regional Earth System modeling. Journal of Geophysical Research: Atmospheres, 119(13), 8450–8475. https://doi.org/10.1002/2013JD021424
- Matsui, T., Zeng, X., Tao, W.-K., Masunaga, H., Olson, W. S., & Lang, S. (2009). Evaluation of long-term Cloud-Resolving Model Simulations using satellite radiance observations and multifrequency satellite simulators. Journal of Atmospheric and Oceanic Technology, 26(7), 1261–1274. https://doi.org/10.1175/2008JTECHA1168.1
- Matsui, T., Zhang, S. Q., Lang, S. E., Tao, W.-K., Ichoku, C., & Peters-Lidard, C. D. (2020). Impact of radiation frequency, precipitation radiative forcing, and radiation column aggregation on convection-permitting West african monsoon simulations. Climate Dynamics, 55(1), 193–213. https://doi.org/10.1007/s00382-018-4187-2
- McFarquhar, G. M., Heymsfield, A. J., Macke, A., Iaquinta, J., & Aulenbach, S. M. (1999). Use of observed ice crystal sizes and shapes to calculate mean-scattering properties and multispectral radiances: CEPEX April 4, 1993, case study. Journal of Geophysical Research: Atmospheres, 104(D24), 31763–31779. https://doi.org/10.1029/1999JD900802
- Meehl, G. A., Senior, C. A., Eyring, V., Flato, G., Lamarque, J.-F., Stouffer, R. J., Taylor, K. E., & Schlund, M. (2020). Context for interpreting equilibrium climate sensitivity and transient climate response from the CMIP6 Earth system models. Science Advances, 6(26), 1–10. https://doi.org/10.1126/sciadv.aba1981
- Meehl, G. A., Yang, D., Arblaster, J. M., Bates, S. C., Rosenbloom, N., Neale, R., Bacmeister, J., Lauritzen, P. H., Bryan, F., Small, J., Truesdale, J., Hannay, C., Shields, C., Strand, W. G., Dennis, J., & Danabasoglu, G. (2019). Effects of Model resolution, physics, and coupling on Southern hemisphere Storm tracks in CESM1.3. Geophysical Research Letters, 46(21), 12408–12416. https://doi.org/10.1029/2019GL084057
- Michibata, T., & Suzuki, K. (2020). Reconciling compensating Errors between Precipitation constraints and the Energy Budget in a Climate model. Geophysical Research Letters, 47(12), 1–10. https://doi.org/10.1029/2020GL088340
- Michibata, T., Suzuki, K., Sekiguchi, M., & Takemura, T. (2019). Prognostic Precipitation in the MIROC6-SPRINTARS GCM: Description and Evaluation against satellite observations. Journal of Advances in Modeling Earth Systems, 11(3), 839–860. https://doi.org/10.1029/2018MS001596
- Michibata, T., Suzuki, K., & Takemura, T. (2020). Snow-induced buffering in aerosol–cloud interactions. Atmospheric Chemistry and Physics, 20(22), 13771–13780. https://doi.org/10.5194/acp-20-13771-2020
- Milbrandt, J. A., & Yau, M. K. (2005). A multimoment bulk Microphysics Parameterization. Part II: A proposed three-moment closure and scheme description. Journal of the Atmospheric Sciences, 62(9), 3065–3081. https://doi.org/10.1175/JAS3535.1
- Mitchell, D. L. (1996). Use of mass- and area-dimensional power laws for Determining Precipitation particle terminal velocities. Journal of the Atmospheric Sciences, 53(12), 1710–1723. https://doi.org/10.1175/1520-0469(1996)053<1710:UOMAAD>2.0.CO;2
- Mitchell, D. L., & Arnott, W. P. (1994). A Model predicting the Evolution of Ice particle size spectra and radiative Properties of Cirrus clouds. Part II: Dependence of Absorption and extinction on Ice crystal morphology. Journal of the Atmospheric Sciences, 51(6), 817–832. https://doi.org/10.1175/1520-0469(1994)051<0817:AMPTEO>2.0.CO;2
- Mitchell, D. L., Liu, Y., & Macke, A. (1996). Modeling cirrus clouds. Part II: treatment of radiative properties. Journal of the Atmospheric Sciences, 53(20), 2967–2988. https://doi.org/10.1175/1520-0469(1996)053<2967:MCCPIT>2.0.CO;2
- Miura, H., Satoh, M., Nasuno, T., Noda, A. T., & Oouchi, K. (2007a). A Madden-Julian Oscillation Event realistically simulated by a Global Cloud-Resolving model. Science, 318(5857), 1763–1765. https://doi.org/10.1126/science.1148443
- Miura, H., Satoh, M., Tomita, H., Noda, A. T., Nasuno, T., & Iga, S. (2007b). A short-duration global cloud-resolving simulation with a realistic land and sea distribution. Geophysical Research Letters, 34(2), 1–5. https://doi.org/10.1029/2006GL027448
- Miyakawa, T., & Kikuchi, K. (2018). CINDY2011/DYNAMO Madden-Julian oscillation successfully reproduced in global cloud/cloud-system resolving simulations despite weak tropical wavelet power. Scientific Reports, 8(1), 1–9. https://doi.org/10.1038/s41598-018-29931-4
- Miyakawa, T., Satoh, M., Miura, H., Tomita, H., Yashiro, H., Noda, A. T., Yamada, Y., Kodama, C., Kimoto, M., & Yoneyama, K. (2014). Madden–Julian Oscillation prediction skill of a new-generation global model demonstrated using a supercomputer. Nature Communications, 5(1), 1–6. https://doi.org/10.1038/ncomms4769
- Miyakawa, T., Takayabu, Y. N., Nasuno, T., Miura, H., Satoh, M., & Moncrieff, M. W. (2012). Convective momentum Transport by Rainbands within a madden–Julian Oscillation in a Global Nonhydrostatic Model with explicit deep convective processes. Part I: Methodology and general results. Journal of the Atmospheric Sciences, 69(4), 1317–1338. https://doi.org/10.1175/JAS-D-11-024.1
- Miyamoto, Y., Kajikawa, Y., Yoshida, R., Yamaura, T., Yashiro, H., & Tomita, H. (2013). Deep moist atmospheric convection in a subkilometer global simulation. Geophysical Research Letters, 40(18), 4922–4926. https://doi.org/10.1002/grl.50944.
- Miyamoto, Y., Satoh, M., Tomita, H., Oouchi, K., Yamada, Y., Kodama, C., & Kinter, J. (2014). Gradient wind balance in tropical cyclones in High-Resolution Global experiments. Monthly Weather Review, 142(5), 1908–1926. https://doi.org/10.1175/MWR-D-13-00115.1
- Miyamoto, Y., Yoshida, R., Yamaura, T., Yashiro, H., Tomita, H., & Kajikawa, Y. (2015). Does convection vary in different cloud disturbances? Atmospheric Science Letters, 16(3), 305–309. https://doi.org/10.1002/asl2.558
- Mizielinski, M. S., Roberts, M. J., Vidale, P. L., Schiemann, R., Demory, M.-E., Strachan, J., Edwards, T., Stephens, A., Lawrence, B. N., Pritchard, M., Chiu, P., Iwi, A., Churchill, J., del Cano Novales, C., Kettleborough, J., Roseblade, W., Selwood, P., Foster, M., Glover, M., & Malcolm, A. (2014). High-resolution global climate modelling: The UPSCALE project, a large-simulation campaign. Geoscientific Model Development, 7(4), 1629–1640. https://doi.org/10.5194/gmd-7-1629-2014
- Morrison, H., de Boer, G., Feingold, G., Harrington, J., Shupe, M. D., & Sulia, K. (2012). Resilience of persistent Arctic mixed-phase clouds. Nature Geoscience, 5(1), 11–17. https://doi.org/10.1038/ngeo1332
- Morrison, H., & Gettelman, A. (2008). A New Two-Moment bulk stratiform cloud microphysics scheme in the Community Atmosphere Model, version 3 (CAM3). part I: Description and Numerical tests. Journal of Climate, 21(15), 3642–3659. https://doi.org/10.1175/2008JCLI2105.1
- Morrison, H., & Grabowski, W. W. (2008). Modeling supersaturation and subgrid-scale Mixing with Two-Moment Bulk Warm microphysics. Journal of the Atmospheric Sciences, 65(3), 792–812. https://doi.org/10.1175/2007JAS2374.1
- Mroz, K., Battaglia, A., Lang, T. J., Tanelli, S., & Sacco, G. F. (2018). Global Precipitation Measuring dual-frequency Precipitation radar observations of hailstorm vertical structure: Current capabilities and drawbacks. Journal of Applied Meteorology and Climatology, 57(9), 2161–2178. https://doi.org/10.1175/JAMC-D-18-0020.1
- Nakajima, T., & King, M. D. (1990). Determination of the optical thickness and effective particle radius of clouds from reflected Solar radiation measurements. Part I: Theory. Journal of the Atmospheric Sciences, 47(15), 1878–1893. https://doi.org/10.1175/1520-0469(1990)047<1878:DOTOTA>2.0.CO;2
- Nakajima, T., Tsukamoto, M., Tsushima, Y., Numaguti, A., & Kimura, T. (2000). Modeling of the radiative process in an atmospheric general circulation model. Applied Optics, 39(27), 4869–4878. https://doi.org/10.1364/AO.39.004869
- Nakajima, T. Y., Suzuki, K., & Stephens, G. L. (2010). Droplet growth in Warm water clouds observed by the A-train. Part II: A Multisensor view. Journal of the Atmospheric Sciences, 67(6), 1897–1907. https://doi.org/10.1175/2010JAS3276.1
- Nakano, M., & Kikuchi, K. (2019). Seasonality of Intraseasonal variability in Global Climate models. Geophysical Research Letters, 46(8), 4441–4449. https://doi.org/10.1029/2019GL082443
- Nakano, M., Sawada, M., Nasuno, T., & Satoh, M. (2015). Intraseasonal variability and tropical cyclogenesis in the western North Pacific simulated by a global nonhydrostatic atmospheric model. Geophysical Research Letters, 42(2), 565–571. https://doi.org/10.1002/2014GL062479
- Nakano, M., Wada, A., Sawada, M., Yoshimura, H., Onishi, R., Kawahara, S., Sasaki, W., Nasuno, T., Yamaguchi, M., Iriguchi, T., Sugi, M., & Takeuchi, Y. (2017). Global 7 km mesh nonhydrostatic Model Intercomparison Project for improving TYphoon forecast (TYMIP-G7): experimental design and preliminary results. Geoscientific Model Development, 10(3), 1363–1381. https://doi.org/10.5194/gmd-10-1363-2017
- Nam, C., Bony, S., Dufresne, J.-L., & Chepfer, H. (2012). The ‘too few, too bright’ tropical low-cloud problem in CMIP5 models. Geophysical Research Letters, 39(21), 1–7. https://doi.org/10.1029/2012GL053421
- Nasuno, T., Miura, H., Satoh, M., Noda, A. T., & Oouchi, K. (2009). Multi-scale organization of Convection in a Global Numerical simulation of the December 2006 MJO Event using explicit Moist processes. Journal of the Meteorological Society of Japan. Ser. II, 87(2), 335–345. https://doi.org/10.2151/jmsj.87.335
- Nishizawa, S., Yashiro, H., Sato, Y., Miyamoto, Y., & Tomita, H. (2015). Influence of grid aspect ratio on planetary boundary layer turbulence in large-eddy simulations. Geoscientific Model Development, 8(10), 3393–3419. https://doi.org/10.5194/gmd-8-3393-2015
- Noda, A. T., Kodama, C., Yamada, Y., Satoh, M., Ogura, T., & Ohno, T. (2019). Responses of clouds and large-scale circulation to Global warming evaluated from multidecadal simulations using a Global Nonhydrostatic model. Journal of Advances in Modeling Earth Systems, 11(9), 2980–2995. https://doi.org/10.1029/2019MS001658
- Noda, A. T., Satoh, M., Yamada, Y., Kodama, C., & Seiki, T. (2014). Responses of tropical and subtropical high-cloud Statistics to Global warming. Journal of Climate, 27(20), 7753–7768. https://doi.org/10.1175/JCLI-D-14-00179.1
- Noda, A. T., Seiki, T., Roh, W., Satoh, M., & Ohno, T. (2021). Improved representation of low-level mixed-phase clouds in a global cloud-system-resolving simulation. Journal of Geophysical Research: Atmospheres, 126(17), e2021JD035223, 1-15. https://doi.org/10.1029/2021JD035223.
- Noda, A. T., Seiki, T., Satoh, M., & Yamada, Y. (2016). High cloud size dependency in the applicability of the fixed anvil temperature hypothesis using global nonhydrostatic simulations. Geophysical Research Letters, 43(5), 2307–2314. https://doi.org/10.1002/2016GL067742
- Okamoto, K. (2017). Evaluation of IR radiance simulation for all-sky assimilation of himawari-8/AHI in a mesoscale NWP system. Quarterly Journal of the Royal Meteorological Society, 143(704), 1517–1527. https://doi.org/10.1002/qj.3022
- Okamoto, K., Sawada, Y., & Kunii, M. (2019). Comparison of assimilating all-sky and clear-sky infrared radiances from himawari-8 in a mesoscale system. Quarterly Journal of the Royal Meteorological Society, 145(719), 745–766. https://doi.org/10.1002/qj.3463
- Okata, M., Nakajima, T., Suzuki, K., Inoue, T., Nakajima, T. Y., & Okamoto, H. (2017). A study on radiative transfer effects in 3-D cloudy atmosphere using satellite data. Journal of Geophysical Research: Atmospheres, 122(1), 443–468. https://doi.org/10.1002/2016JD025441
- Onishi, R., & Takahashi, K. (2012). A warm-Bin–cold-bulk hybrid cloud microphysical model. Journal of the Atmospheric Sciences, 69(5), 1474–1497. https://doi.org/10.1175/JAS-D-11-0166.1
- Onishi, R., Sugiyama, D., & Matsuda, K. (2019). Super-Resolution simulation for real-time prediction of urban micrometeorology. Sola, 15(0), 178–182. https://doi.org/10.2151/sola.2019-032
- Oouchi, K., Noda, A. T., Satoh, M., Miura, H., Tomita, H., Nasuno, T., & Iga, S. (2009). A simulated preconditioning of Typhoon genesis controlled by a Boreal Summer Madden-Julian Oscillation Event in a Global cloud-system-resolving model. Sola, 5, 65–68. https://doi.org/10.2151/sola.2009-017
- Park, S., Bretherton, C. S., & Rasch, P. J. (2014). Integrating cloud processes in the Community Atmosphere Model, version 5. Journal of Climate, 27(18), 6821–6856. https://doi.org/10.1175/JCLI-D-14-00087.1
- Phillips, V. T. J., Donner, L. J., & Garner, S. T. (2007). Nucleation processes in Deep Convection Simulated by a Cloud-System-Resolving Model with double-moment bulk microphysics. Journal of the Atmospheric Sciences, 64(3), 738–761. https://doi.org/10.1175/JAS3869.1
- Pollack, J. B., & Cuzzi, J. N. (1980). Scattering by nonspherical particles of size comparable to a wavelength: A New semi-empirical Theory and Its Application to tropospheric aerosols. Journal of the Atmospheric Sciences, 37(4), 868–881. https://doi.org/10.1175/1520-0469(1980)037<0868:SBNPOS>2.0.CO;2
- Posselt, R., & Lohmann, U. (2008). Introduction of prognostic rain in ECHAM5: Design and single column model simulations. Atmospheric Chemistry and Physics, 8(11), 2949–2963. https://doi.org/10.5194/acp-8-2949-2008
- Pruppacher, H. R., & Klett, J. D. (2010). Microphysics of clouds and precipitation (2nd ed.). Springer Netherlands. https://doi.org/10.1007/978-0-306-48100-0.
- Putman, W. M., & Lin, S.-J. (2007). Finite-volume transport on various cubed-sphere grids. Journal of Computational Physics, 227(1), 55–78. https://doi.org/10.1016/j.jcp.2007.07.022
- Putman, W. M., & Suarez, M. (2011). Cloud-system resolving simulations with the NASA Goddard Earth Observing system global atmospheric model (GEOS-5). Geophysical Research Letters, 38(16), 1–5. https://doi.org/10.1029/2011GL048438
- Randall, D. A., Genio, A. D. D., Donner, L. J., Collins, W. D., & Klein, S. A. (2016). The Impact of ARM on Climate modeling. Meteorological Monographs, 57(1), 26.1–26.16. https://doi.org/10.1175/AMSMONOGRAPHS-D-15-0050.1
- Ren, C., & Mackenzie, A. R. (2005). Cirrus parametrization and the role of ice nuclei. Quarterly Journal of the Royal Meteorological Society, 131(608), 1585–1605. https://doi.org/10.1256/qj.04.126
- Reverdy, M., Chepfer, H., Donovan, D., Noel, V., Cesana, G., Hoareau, C., Chiriaco, M., & Bastin, S. (2015). An EarthCARE/ATLID simulator to evaluate cloud description in climate models. Journal of Geophysical Research: Atmospheres, 120(21), 11,090–11,113. https://doi.org/10.1002/2015JD023919
- Roberts, M. J., Vidale, P. L., Senior, C., Hewitt, H. T., Bates, C., Berthou, S., Chang, P., Christensen, H. M., Danilov, S., Demory, M.-E., Griffies, S. M., Haarsma, R., Jung, T., Martin, G., Minobe, S., Ringler, T., Satoh, M., Schiemann, R., Scoccimarro, E., … Wehner, M. F. (2018). The Benefits of Global high resolution for Climate simulation: Process understanding and the enabling of stakeholder decisions at the regional scale. Bulletin of the American Meteorological Society, 99(11), 2341–2359. https://doi.org/10.1175/BAMS-D-15-00320.1
- Roehrig, R., Beau, I., Saint-Martin, D., Alias, A., Decharme, B., Guérémy, J.-F., Voldoire, A., Abdel-Lathif, A. Y., Bazile, E., Belamari, S., Blein, S., Bouniol, D., Bouteloup, Y., Cattiaux, J., Chauvin, F., Chevallier, M., Colin, J., Douville, H., Marquet, P., … Sénési, S. (2020). The CNRM Global Atmosphere Model ARPEGE-Climat 6.3: Description and evaluation. Journal of Advances in Modeling Earth Systems, 12(7), 1–53. https://doi.org/10.1029/2020MS002075
- Roh, W., & Satoh, M. (2014). Evaluation of precipitating hydrometeor parameterizations in a single-moment Bulk Microphysics scheme for deep convective systems over the tropical central pacific. Journal of the Atmospheric Sciences, 71(7), 2654–2673. https://doi.org/10.1175/JAS-D-13-0252.1
- Roh, W., & Satoh, M. (2018). Extension of a Multisensor satellite radiance-based Evaluation for cloud system resolving models. Journal of the Meteorological Society of Japan. Ser. II, 96(1), 55–63. https://doi.org/10.2151/jmsj.2018-002
- Roh, W., Satoh, M., Hashino, T., Okamoto, H., & Seiki, T. (2020). Evaluations of the thermodynamic Phases of clouds in a Cloud-System-Resolving Model Using CALIPSO and a satellite simulator over the Southern Ocean. Journal of the Atmospheric Sciences, 77(11), 3781–3801. https://doi.org/10.1175/JAS-D-19-0273.1
- Roh, W., Satoh, M., & Hohenegger, C. (2021). Intercomparison of cloud properties in DYAMOND simulations over the Atlantic Ocean. Journal of the Meteorological Society of Japan. Ser. II, 99(6), 1439–1451. https://doi.org/10.2151/jmsj.2021-070
- Roh, W., Satoh, M., & Nasuno, T. (2017). Improvement of a cloud microphysics scheme for a Global Nonhydrostatic Model Using TRMM and a satellite simulator. Journal of the Atmospheric Sciences, 74(1), 167–184. https://doi.org/10.1175/JAS-D-16-0027.1
- Rossow, W. B., & Schiffer, R. A. (1999). Advances in understanding clouds from ISCCP. Bulletin of the American Meteorological Society, 80(11), 2261–2288. https://doi.org/10.1175/1520-0477(1999)080<2261:AIUCFI>2.0.CO;2
- Rutledge, S. A., & Hobbs, P. V. (1984). The mesoscale and microscale structure and organization of clouds and Precipitation in Midlatitude cyclones. XII: A Diagnostic Modeling Study of Precipitation Development in narrow cold-frontal rainbands. Journal of the Atmospheric Sciences, 41(20), 2949–2972. https://doi.org/10.1175/1520-0469(1984)041<2949:TMAMSA>2.0.CO;2
- Saito, K., Ishida, J., Aranami, K., Hara, T., Segawa, T., Narita, M., & Honda, Y. (2007). Nonhydrostatic Atmospheric models and Operational Development at JMA. Journal of the Meteorological Society of Japan. Ser. II, 85B, 271–304. https://doi.org/10.2151/jmsj.85B.271
- Saito, M., Iwabuchi, H., Yang, P., Tang, G., King, M. D., & Sekiguchi, M. (2017). Ice particle morphology and microphysical properties of cirrus clouds inferred from combined CALIOP-IIR measurements. Journal of Geophysical Research: Atmospheres, 122(8), 4440–4462. https://doi.org/10.1002/2016JD026080
- Sant, V., Posselt, R., & Lohmann, U. (2015). Prognostic precipitation with three liquid water classes in the ECHAM5–HAM GCM. Atmospheric Chemistry and Physics, 15(15), 8717–8738. https://doi.org/10.5194/acp-15-8717-2015
- Sasaki, W., Onishi, R., Fuchigami, H., Goto, K., Nishikawa, S., Ishikawa, Y., & Takahashi, K. (2016). MJO simulation in a cloud-system-resolving global ocean-atmosphere coupled model. Geophysical Research Letters, 43(17), 9352–9360. https://doi.org/10.1002/2016GL070550
- Sassen, K., Wang, Z., & Liu, D. (2008). Global distribution of cirrus clouds from CloudSat/Cloud-Aerosol Lidar and Infrared Pathfinder Satellite observations (CALIPSO) measurements. Journal of Geophysical Research: Atmospheres, 113(D8), 1–12. https://doi.org/10.1029/2008JD009972
- Sato, Y., Miyamoto, Y., & Tomita, H. (2019). Large dependency of charge distribution in a tropical cyclone inner core upon aerosol number concentration. Progress in Earth and Planetary Science, 6(1), 1–13. https://doi.org/10.1186/s40645-019-0309-7
- Sato, Y., Nishizawa, S., Yashiro, H., Miyamoto, Y., Kajikawa, Y., & Tomita, H. (2015). Impacts of cloud microphysics on trade wind cumulus: Which cloud microphysics processes contribute to the diversity in a large eddy simulation? Progress in Earth and Planetary Science, 2(1), 1–16. https://doi.org/10.1186/s40645-015-0053-6
- Sato, Y., Shima, S., & Tomita, H. (2018). Numerical Convergence of shallow Convection cloud field simulations: Comparison between double-moment eulerian and particle-based Lagrangian microphysics coupled to the same dynamical core. Journal of Advances in Modeling Earth Systems, 10(7), 1495–1512. https://doi.org/10.1029/2018MS001285
- Satoh, M. (2002). Conservative Scheme for the Compressible Nonhydrostatic models with the horizontally explicit and Vertically implicit time Integration scheme. Monthly Weather Review, 130(5), 1227–1245. https://doi.org/10.1175/1520-0493(2002)130<1227:CSFTCN>2.0.CO;2
- Satoh, M. (2003). Conservative Scheme for a Compressible Nonhydrostatic Model with Moist processes. Monthly Weather Review, 131(6), 1033–1050. https://doi.org/10.1175/1520-0493(2003)131<1033:CSFACN>2.0.CO;2
- Satoh, M., Inoue, T., & Miura, H. (2010). Evaluations of cloud properties of global and local cloud system resolving models using CALIPSO and CloudSat simulators. Journal of Geophysical Research: Atmospheres, 115(D4), 1–18. https://doi.org/10.1029/2009JD012247
- Satoh, M., Matsugishi, S., Roh, W., Ikuta, Y., Kuba, N., Seiki, T., Hashino, T., & Okamoto, H. (2022). Evaluation of cloud and precipitation processes in regional and global models with ULTIMATE (ULTra-sIte for Measuring Atmosphere of Tokyo metropolitan environment): A case study using the dual-polarization Doppler weather radars. [Manuscript submitted for publication]. Research Square. https://doi.org/10.21203/rs.3.rs-1484431/v1
- Satoh, M., Matsuno, T., Tomita, H., Miura, H., Nasuno, T., & Iga, S. (2008a). Nonhydrostatic icosahedral atmospheric model (NICAM) for global cloud resolving simulations. Journal of Computational Physics, 227(7), 3486–3514. https://doi.org/10.1016/j.jcp.2007.02.006
- Satoh, M., Nasuno, T., Miura, H., Tomita, H., Iga, S., & Takayabu, Y. (2008b). Precipitation Statistics comparison between Global cloud resolving simulation with NICAM and TRMM PR data. In K. Hamilton, & W. Ohfuchi (Eds.), High Resolution Numerical Modelling of the Atmosphere and ocean (pp. 99–112). Springer. https://doi.org/10.1007/978-0-387-49791-4_6
- Satoh, M., Noda, A. T., Seiki, T., Chen, Y.-W., Kodama, C., Yamada, Y., Kuba, N., & Sato, Y. (2018). Toward reduction of the uncertainties in climate sensitivity due to cloud processes using a global non-hydrostatic atmospheric model. Progress in Earth and Planetary Science, 5(1), 1–29. https://doi.org/10.1186/s40645-018-0226-1
- Satoh, M., Roh, W., & Hashino, T. (2016). Evaluations of clouds and precipitations in NICAM using the joint simulator for satellite sensors. CGER’S SUPERCOMPUTER MONOGRAPH REPORT, 22. Center for Global Environmental Research. http://www.cger.nies.go.jp/publications/report/i127/i127.pdf.
- Satoh, M., Stevens, B., Judt, F., Khairoutdinov, M., Lin, S.-J., Putman, W. M., & Düben, P. (2019). Global Cloud-Resolving models. Current Climate Change Reports, 5(3), 172–184. https://doi.org/10.1007/s40641-019-00131-0
- Satoh, M., Tomita, H., Yashiro, H., Kajikawa, Y., Miyamoto, Y., Yamaura, T., Miyakawa, T., Nakano, M., Kodama, C., Noda, A. T., Nasuno, T., Yamada, Y., & Fukutomi, Y. (2017). Outcomes and challenges of global high-resolution non-hydrostatic atmospheric simulations using the K computer. Progress in Earth and Planetary Science, 4(1), 1–24. https://doi.org/10.1186/s40645-017-0127-8
- Satoh, M., Tomita, H., Yashiro, H., Miura, H., Kodama, C., Seiki, T., Noda, A. T., Yamada, Y., Goto, D., Sawada, M., Miyoshi, T., Niwa, Y., Hara, M., Ohno, T., Iga, S., Arakawa, T., Inoue, T., & Kubokawa, H. (2014). The Non-hydrostatic icosahedral Atmospheric model: Description and development. Progress in Earth and Planetary Science, 1(1), 1–32. https://doi.org/10.1186/s40645-014-0018-1
- Saunders, R., Hocking, J., Turner, E., Rayer, P., Rundle, D., Brunel, P., Vidot, J., Roquet, P., Matricardi, M., Geer, A., Bormann, N., & Lupu, C. (2018). An update on the RTTOV fast radiative transfer model (currently at version 12). Geoscientific Model Development, 11(7), 2717–2737. https://doi.org/10.5194/gmd-11-2717-2018
- Schär, C., Fuhrer, O., Arteaga, A., Ban, N., Charpilloz, C., Girolamo, S. D., Hentgen, L., Hoefler, T., Lapillonne, X., Leutwyler, D., Osterried, K., Panosetti, D., Rüdisühli, S., Schlemmer, L., Schulthess, T. C., Sprenger, M., Ubbiali, S., & Wernli, H. (2020). Kilometer-Scale Climate Models: Prospects and challenges. Bulletin of the American Meteorological Society, 101(5), E567–E587. https://doi.org/10.1175/BAMS-D-18-0167.1
- Seifert, A. (2008). On the Parameterization of evaporation of raindrops as simulated by a One-dimensional rainshaft model. Journal of the Atmospheric Sciences, 65(11), 3608–3619. https://doi.org/10.1175/2008JAS2586.1
- Seifert, A., & Beheng, K. D. (2001). A double-moment parameterization for simulating autoconversion, accretion and selfcollection. Atmospheric Research, 59–60, 265–281. https://doi.org/10.1016/S0169-8095(01)00126-0
- Seifert, A., & Beheng, K. D. (2006). A two-moment cloud microphysics parameterization for mixed-phase clouds. Part 1: Model description. Meteorology and Atmospheric Physics, 92(1), 45–66. https://doi.org/10.1007/s00703-005-0112-4
- Seifert, A., Khain, A., Pokrovsky, A., & Beheng, K. D. (2006). A comparison of spectral bin and two-moment bulk mixed-phase cloud microphysics. Atmospheric Research, 80(1), 46–66. https://doi.org/10.1016/j.atmosres.2005.06.009
- Seiki, T. (2021). Near-global three-dimensional hail signals detected by using GPM-DPR observations. Journal of the Meteorological Society of Japan. Ser. II, 99(2), 379–402. https://doi.org/10.2151/jmsj.2021-018
- Seiki, T., Kodama, C., Noda, A. T., & Satoh, M. (2015a). Improvement in Global cloud-system-resolving simulations by using a double-moment bulk cloud microphysics scheme. Journal of Climate, 28(6), 2405–2419. https://doi.org/10.1175/JCLI-D-14-00241.1
- Seiki, T., Kodama, C., Satoh, M., Hagihara, Y., & Okamoto, H. (2019). Characteristics of Ice clouds over mountain regions Detected by CALIPSO and CloudSat satellite observations. Journal of Geophysical Research: Atmospheres, 124(20), 10858–10877. https://doi.org/10.1029/2019JD030519
- Seiki, T., Kodama, C., Satoh, M., Hashino, T., Hagihara, Y., & Okamoto, H. (2015b). Vertical grid spacing necessary for simulating tropical cirrus clouds with a high-resolution atmospheric general circulation model. Geophysical Research Letters, 42(10), 4150–4157. https://doi.org/10.1002/2015GL064282
- Seiki, T., & Nakajima, T. (2014). Aerosol effects of the condensation process on a convective cloud simulation. Journal of the Atmospheric Sciences, 71(2), 833–853. https://doi.org/10.1175/JAS-D-12-0195.1
- Seiki, T., & Roh, W. (2020). Improvements in supercooled liquid water simulations of Low-level mixed-phase clouds over the Southern Ocean using a single-column model. Journal of the Atmospheric Sciences, 77(11), 3803–3819. https://doi.org/10.1175/JAS-D-19-0266.1
- Seiki, T., Satoh, M., Tomita, H., & Nakajima, T. (2014). Simultaneous evaluation of ice cloud microphysics and nonsphericity of the cloud optical properties using hydrometeor video sonde and radiometer sonde in situ observations. Journal of Geophysical Research: Atmospheres, 119(11), 6681–6701. https://doi.org/10.1002/2013JD021086
- Sekiguchi, M., & Nakajima, T. (2008). A k-distribution-based radiation code and its computational optimization for an atmospheric general circulation model. Journal of Quantitative Spectroscopy and Radiative Transfer, 109(17), 2779–2793. https://doi.org/10.1016/j.jqsrt.2008.07.013
- Senf, F., Voigt, A., Clerbaux, N., Hünerbein, A., & Deneke, H. (2020). Increasing resolution and resolving Convection improve the simulation of Cloud-Radiative effects over the North atlantic. Journal of Geophysical Research: Atmospheres, 125(19), 1–26. https://doi.org/10.1029/2020JD032667
- Sherwood, S. C., Webb, M. J., Annan, J. D., Armour, K. C., Forster, P. M., Hargreaves, J. C., Hegerl, G., Klein, S. A., Marvel, K. D., Rohling, E. J., Watanabe, M., Andrews, T., Braconnot, P., Bretherton, C. S., Foster, G. L., Hausfather, Z., Heydt, A. S. v. d., Knutti, R., Mauritsen, T., … Zelinka, M. D. (2020). An Assessment of Earth’s Climate sensitivity using multiple lines of evidence. Reviews of Geophysics, 58(4), 1–92. https://doi.org/10.1029/2019RG000678
- Shibuya, R., Miura, H., & Sato, K. (2016). A Grid Transformation method for a quasi-uniform, circular fine region using the Spring dynamics. Journal of the Meteorological Society of Japan. Ser. II, 94(5), 443–452. https://doi.org/10.2151/jmsj.2016-022
- Shibuya, R., Nakano, M., Kodama, C., Nasuno, T., Kikuchi, K., Satoh, M., Miura, H., & Miyakawa, T. (2021). Prediction skill of the Boreal Summer intra-seasonal Oscillation in Global Non-hydrostatic Atmospheric Model simulations with Explicit Cloud microphysics. Journal of the Meteorological Society of Japan. Ser. II, 99(4), 973–992. https://doi.org/10.2151/jmsj.2021-046
- Shipway, B. J., & Hill, A. A. (2012). Diagnosis of systematic differences between multiple parametrizations of warm rain microphysics using a kinematic framework. Quarterly Journal of the Royal Meteorological Society, 138(669), 2196–2211. https://doi.org/10.1002/qj.1913
- Shupe, M. D., & Intrieri, J. M. (2004). Cloud radiative forcing of the Arctic surface: The Influence of Cloud Properties, surface albedo, and Solar zenith angle. Journal of Climate, 17(3), 616–628. https://doi.org/10.1175/1520-0442(2004)017<0616:CRFOTA>2.0.CO;2
- Skamarock, W. C., Duda, M. G., Ha, S., & Park, S.-H. (2018). Limited-Area Atmospheric Modeling using an Unstructured mesh. Monthly Weather Review, 146(10), 3445–3460. https://doi.org/10.1175/MWR-D-18-0155.1
- Skamarock, W. C., Klemp, J. B., Duda, M. G., Fowler, L. D., Park, S.-H., & Ringler, T. D. (2012). A Multiscale Nonhydrostatic Atmospheric Model using centroidal voronoi tesselations and C-grid staggering. Monthly Weather Review, 140(9), 3090–3105. https://doi.org/10.1175/MWR-D-11-00215.1
- Sölch, I., & Kärcher, B. (2011). Process-oriented large-eddy simulations of a midlatitude cirrus cloud system based on observations. Quarterly Journal of the Royal Meteorological Society, 137(655), 374–393. https://doi.org/10.1002/qj.764
- Song, X., Zhang, G. J., & Li, J.-L. F. (2012). Evaluation of microphysics Parameterization for convective clouds in the NCAR Community Atmosphere Model CAM5. Journal of Climate, 25(24), 8568–8590. https://doi.org/10.1175/JCLI-D-11-00563.1
- Stephens, G. L., Tsay, S.-C., Stackhouse, P. W., & Flatau, P. J. (1990). The relevance of the microphysical and radiative Properties of Cirrus clouds to Climate and climatic feedback. Journal of the Atmospheric Sciences, 47(14), 1742–1754. https://doi.org/10.1175/1520-0469(1990)047<1742:TROTMA>2.0.CO;2
- Stephens, G. L., Vane, D. G., Tanelli, S., Im, E., Durden, S., Rokey, M., Reinke, D., Partain, P., Mace, G. G., Austin, R., L’Ecuyer, T., Haynes, J., Lebsock, M., Suzuki, K., Waliser, D., Wu, D., Kay, J., Gettelman, A., Wang, Z., & Marchand, R. (2008). CloudSat mission: Performance and early science after the first year of operation. Journal of Geophysical Research: Atmospheres, 113(D8), 1–18. https://doi.org/10.1029/2008JD009982
- Stevens, B., Acquistapace, C., Hansen, A., Heinze, R., Klinger, C., Klocke, D., Rybka, H., Schubotz, W., Windmiller, J., Adamidis, P., Arka, I., Barlakas, V., Biercamp, J., Brueck, M., Brune, S., Buehler, S. A., Burkhardt, U., Cioni, G., Costa-Surós, M., … Zängl, G. (2020). The added value of large-eddy and storm-resolving models for simulating clouds and precipitation. Journal of the Meteorological Society of Japan. Ser. II, 98(2), 395–435. https://doi.org/10.2151/jmsj.2020-021
- Stevens, B., & Feingold, G. (2009). Untangling aerosol effects on clouds and precipitation in a buffered system. Nature, 461(7264), 607–613. https://doi.org/10.1038/nature08281
- Stevens, B., Satoh, M., Auger, L., Biercamp, J., Bretherton, C. S., Chen, X., Düben, P., Judt, F., Khairoutdinov, M., Klocke, D., Kodama, C., Kornblueh, L., Lin, S.-J., Neumann, P., Putman, W. M., Röber, N., Shibuya, R., Vanniere, B., Vidale, P. L., … Zhou, L. (2019). DYAMOND: The DYnamics of the Atmospheric general circulation Modeled On Non-hydrostatic domains. Progress in Earth and Planetary Science, 6(1), 1–17. https://doi.org/10.1186/s40645-019-0304-z
- Stockdale, T. N. (1997). Coupled ocean–Atmosphere forecasts in the presence of Climate drift. Monthly Weather Review, 125(5), 809–818. https://doi.org/10.1175/1520-0493(1997)125<0809:COAFIT>2.0.CO;2
- Sueki, K., Yamaura, T., Yashiro, H., Nishizawa, S., Yoshida, R., Kajikawa, Y., & Tomita, H. (2019). Convergence of convective updraft ensembles With respect to the grid Spacing of Atmospheric models. Geophysical Research Letters, 46(24), 14817–14825. https://doi.org/10.1029/2019GL084491
- Suzuki, K., Golaz, J.-C., & Stephens, G. L. (2013). Evaluating cloud tuning in a climate model with satellite observations. Geophysical Research Letters, 40(16), 4464–4468. https://doi.org/10.1002/grl.50874
- Suzuki, K., Nakajima, T. Y., & Stephens, G. L. (2010). Particle growth and drop collection efficiency of Warm clouds as inferred from Joint CloudSat and MODIS observations. Journal of the Atmospheric Sciences, 67(9), 3019–3032. https://doi.org/10.1175/2010JAS3463.1
- Suzuki, K., Stephens, G., Bodas-Salcedo, A., Wang, M., Golaz, J.-C., Yokohata, T., & Koshiro, T. (2015). Evaluation of the Warm Rain formation process in Global Models with satellite observations. Journal of the Atmospheric Sciences, 72(10), 3996–4014. https://doi.org/10.1175/JAS-D-14-0265.1
- Suzuki, K., Stephens, G. L., Heever, S. C. v. d., & Nakajima, T. Y. (2011). Diagnosis of the Warm Rain process in Cloud-Resolving Models using Joint CloudSat and MODIS observations. Journal of the Atmospheric Sciences, 68(11), 2655–2670. https://doi.org/10.1175/JAS-D-10-05026.1
- Swales, D. J., Pincus, R., & Bodas-Salcedo, A. (2018). The cloud feedback Model Intercomparison Project observational simulator package: Version 2. Geoscientific Model Development, 11(1), 77–81. https://doi.org/10.5194/gmd-11-77-2018
- Takahashi, K., Peng, X., Onishi, R., Ohdaira, M., Goto, K., Fuchigami, H., & Sugimura, T. (2008). Impact of coupled Nonhydrostatic atmosphere-ocean-land model with high resolution. In K. Hamilton, & W. Ohfuchi (Eds.), High Resolution Numerical Modelling of the Atmosphere and ocean (pp. 261–273). Springer. https://doi.org/10.1007/978-0-387-49791-4_15
- Takano, Y., & Liou, K. N. (1995). Radiative transfer in cirrus clouds. Part III: Light scattering by irregular ice crystals. Journal of the Atmospheric Sciences, 52(7), 818–837. https://doi.org/10.1175/1520-0469(1995)052<0818:RTICCP>2.0.CO;2
- Takano, Y., & Liou, K.-N. (1989). Solar radiative transfer in cirrus clouds. Part I: Single-scattering and optical properties of hexagonal Ice crystals. Journal of the Atmospheric Sciences, 46(1), 3–19. https://doi.org/10.1175/1520-0469(1989)046<0003:SRTICC>2.0.CO;2
- Takasuka, D., Miyakawa, T., Satoh, M., & Miura, H. (2015). Topographical effects on internally produced MJO-like disturbances in an Aqua-Planet version of NICAM. Sola, 11(0), 170–176. https://doi.org/10.2151/sola.2015-038
- Takasuka, D., Satoh, M., Miyakawa, T., & Miura, H. (2018). Initiation processes of the tropical Intraseasonal variability simulated in an Aqua-Planet experiment: What is the intrinsic Mechanism for MJO onset? Journal of Advances in Modeling Earth Systems, 10(4), 1047–1073. https://doi.org/10.1002/2017MS001243
- Takayabu, Y. N. (2002). Spectral representation of rain profiles and diurnal variations observed with TRMM PR over the equatorial area. Geophysical Research Letters, 29(12), 25-1–25-4. https://doi.org/10.1029/2001GL014113
- Taniguchi, H., Yanase, W., & Satoh, M. (2010). Ensemble simulation of Cyclone Nargis by a Global cloud-system-resolving model—Modulation of cyclogenesis by the Madden-Julian oscillation. Journal of the Meteorological Society of Japan. Ser. II, 88(3), 571–591. https://doi.org/10.2151/jmsj.2010-317
- Tao, W.-K., Chern, J.-D., Atlas, R., Randall, D., Khairoutdinov, M., Li, J.-L., Waliser, D. E., Hou, A., Lin, X., Peters-Lidard, C., Lau, W., Jiang, J., & Simpson, J. (2009). A Multiscale Modeling system: Developments, applications, and Critical issues. Bulletin of the American Meteorological Society, 90(4), 515–534. https://doi.org/10.1175/2008BAMS2542.1
- Tao, W.-K., Lang, S., Simpson, J., Sui, C.-H., Ferrier, B., & Chou, M.-D. (1996). Mechanisms of cloud-radiation interaction in the tropics and midlatitudes. Journal of the Atmospheric Sciences, 53(18), 2624–2651. https://doi.org/10.1175/1520-0469(1996)053<2624:MOCRII>2.0.CO;2
- Tatebe, H., Ogura, T., Nitta, T., Komuro, Y., Ogochi, K., Takemura, T., Sudo, K., Sekiguchi, M., Abe, M., Saito, F., Chikira, M., Watanabe, S., Mori, M., Hirota, N., Kawatani, Y., Mochizuki, T., Yoshimura, K., Takata, K., O’ishi, R., … Kimoto, M. (2019). Description and basic evaluation of simulated mean state, internal variability, and climate sensitivity in MIROC6. Geoscientific Model Development, 12(7), 2727–2765. https://doi.org/10.5194/gmd-12-2727-2019
- Thompson, G., Field, P. R., Rasmussen, R. M., & Hall, W. D. (2008). Explicit forecasts of Winter Precipitation using an improved bulk microphysics scheme. Part II: Implementation of a New snow parameterization. Monthly Weather Review, 136(12), 5095–5115. https://doi.org/10.1175/2008MWR2387.1
- Thompson, G., Tewari, M., Ikeda, K., Tessendorf, S., Weeks, C., Otkin, J., & Kong, F. (2016). Explicitly-coupled cloud physics and radiation parameterizations and subsequent evaluation in WRF high-resolution convective forecasts. Atmospheric Research, 168, 92–104. https://doi.org/10.1016/j.atmosres.2015.09.005
- Tomita, H. (2008a). A stretched icosahedral grid by a New grid transformation. Journal of the Meteorological Society of Japan. Ser. II, 86A(0), 107–119. https://doi.org/10.2151/jmsj.86A.107
- Tomita, H. (2008b). New microphysical schemes with five and Six categories by Diagnostic Generation of cloud Ice. Journal of the Meteorological Society of Japan. Ser. II, 86A, 121–142. https://doi.org/10.2151/jmsj.86A.121
- Tomita, H., Miura, H., Iga, S., Nasuno, T., & Satoh, M. (2005). A global cloud-resolving simulation: Preliminary results from an aqua planet experiment. Geophysical Research Letters, 32(8), 1–4. https://doi.org/10.1029/2005GL022459
- Tomita, H., & Satoh, M. (2004). A new dynamical framework of nonhydrostatic global model using the icosahedral grid. Fluid Dynamics Research, 34(6), 357–400. https://doi.org/10.1016/j.fluiddyn.2004.03.003
- Tomita, H., Tsugawa, M., Satoh, M., & Goto, K. (2001). Shallow water model on a modified icosahedral geodesic grid by using spring dynamics. Journal of Computational Physics, 174(2), 579–613. https://doi.org/10.1006/jcph.2001.6897
- Trenberth, K. E., & Fasullo, J. T. (2010). Simulation of present-Day and twenty-first-century energy budgets of the Southern Oceans. Journal of Climate, 23(2), 440–454. https://doi.org/10.1175/2009JCLI3152.1
- Turbeville, S. M., Nugent, J. M., Ackerman, T. P., Bretherton, C. S., & Blossey, P. N. (2022). Tropical cirrus in global storm-resolving models: 2. Cirrus life cycle and top-of-Atmosphere radiative fluxes. Earth and Space Science, 9(2), 1–19. https://doi.org/10.1029/2021EA001978
- Uchida, J., Mori, M., Hara, M., Satoh, M., Goto, D., Kataoka, T., Suzuki, K., & Nakajima, T. (2017). Impact of lateral Boundary Errors on the simulation of clouds with a Nonhydrostatic regional Climate model. Monthly Weather Review, 145(12), 5059–5082. https://doi.org/10.1175/MWR-D-17-0158.1
- Uchida, J., Mori, M., Nakamura, H., Satoh, M., Suzuki, K., & Nakajima, T. (2016). Error and energy budget analysis of a Nonhydrostatic stretched-grid Global Atmospheric model. Monthly Weather Review, 144(4), 1423–1447. https://doi.org/10.1175/MWR-D-15-0271.1
- Vergara-Temprado, J., Ban, N., Panosetti, D., Schlemmer, L., & Schär, C. (2020). Climate Models permit Convection at much coarser resolutions than previously considered. Journal of Climate, 33(5), 1915–1933. https://doi.org/10.1175/JCLI-D-19-0286.1
- Voldoire, A., Decharme, B., Pianezze, J., Lebeaupin Brossier, C., Sevault, F., Seyfried, L., Garnier, V., Bielli, S., Valcke, S., Alias, A., Accensi, M., Ardhuin, F., Bouin, M.-N., Ducrocq, V., Faroux, S., Giordani, H., Léger, F., Marsaleix, P., Rainaud, R., … Riette, S. (2017). SURFEX v8.0 interface with OASIS3-MCT to couple atmosphere with hydrology, ocean, waves and sea-ice models, from coastal to global scales. Geoscientific Model Development, 10(11), 4207–4227. https://doi.org/10.5194/gmd-10-4207-2017
- Voors, R., Donovan, D., Acarreta, J., Eisinger, M., Franco, R., Lajas, D., Moyano, R., Pirondini, F., Ramos, J., & Wehr, T. (2007). ECSIM: The simulator framework for EarthCARE. Sensors, Systems, and Next-Generation Satellites XI, 6744, 1–11. https://doi.org/10.1117/12.737738
- Waliser, D. E., Li, J.-L. F., L’Ecuyer, T. S., & Chen, W.-T. (2011). The impact of precipitating ice and snow on the radiation balance in global climate models. Geophysical Research Letters, 38(6), 1–6. https://doi.org/10.1029/2010GL046478
- Walters, D., Baran, A. J., Boutle, I., Brooks, M., Earnshaw, P., Edwards, J., Furtado, K., Hill, P., Lock, A., Manners, J., Morcrette, C., Mulcahy, J., Sanchez, C., Smith, C., Stratton, R., Tennant, W., Tomassini, L., Van Weverberg, K., Vosper, S., … Zerroukat, M. (2019). The Met Office Unified Model Global Atmosphere 7.0/7.1 and JULES Global land 7.0 configurations. Geoscientific Model Development, 12(5), 1909–1963. https://doi.org/10.5194/gmd-12-1909-2019
- Watanabe, M., Emori, S., Satoh, M., & Miura, H. (2009). A PDF-based hybrid prognostic cloud scheme for general circulation models. Climate Dynamics, 33(6), 795–816. https://doi.org/10.1007/s00382-008-0489-0
- Wedi, N. P. (2014). Increasing horizontal resolution in numerical weather prediction and climate simulations: Illusion or panacea? Philosophical Transactions of the Royal Society A: Mathematical, Physical and Engineering Sciences, 372(2018), 1–12. https://doi.org/10.1098/rsta.2013.0289
- Wedi, N. P., Polichtchouk, I., Dueben, P., Anantharaj, V. G., Bauer, P., Boussetta, S., Browne, P., Deconinck, W., Gaudin, W., Hadade, I., Hatfield, S., Iffrig, O., Lopez, P., Maciel, P., Mueller, A., Saarinen, S., Sandu, I., Quintino, T., & Vitart, F. (2020). A baseline for Global Weather and Climate simulations at 1 km resolution. Journal of Advances in Modeling Earth Systems, 12(11), 1–17. https://doi.org/10.1029/2020MS002192
- Weisman, M. L., Skamarock, W. C., & Klemp, J. B. (1997). The resolution dependence of explicitly Modeled convective systems. Monthly Weather Review, 125(4), 527–548. https://doi.org/10.1175/1520-0493(1997)125<0527:TRDOEM>2.0.CO;2
- Wilson, D. R., & Ballard, S. P. (1999). A microphysically based precipitation scheme for the UK meteorological office unified model. Quarterly Journal of the Royal Meteorological Society, 125(557), 1607–1636. https://doi.org/10.1002/qj.49712555707
- Wing, A. A., Emanuel, K., Holloway, C. E., & Muller, C. (2017). Convective self-aggregation in Numerical simulations: A review. Surveys in Geophysics, 38(6), 1173–1197. https://doi.org/10.1007/s10712-017-9408-4
- Winker, D. M., Vaughan, M. A., Omar, A., Hu, Y., Powell, K. A., Liu, Z., Hunt, W. H., & Young, S. A. (2009). Overview of the CALIPSO mission and CALIOP data processing algorithms. Journal of Atmospheric and Oceanic Technology, 26(11), 2310–2323. https://doi.org/10.1175/2009JTECHA1281.1
- Wood, N., Staniforth, A., White, A., Allen, T., Diamantakis, M., Gross, M., Melvin, T., Smith, C., Vosper, S., Zerroukat, M., & Thuburn, J. (2014). An inherently mass-conserving semi-implicit semi-Lagrangian discretization of the deep-atmosphere global non-hydrostatic equations. Quarterly Journal of the Royal Meteorological Society, 140(682), 1505–1520. https://doi.org/10.1002/qj.2235
- WRF Users’ Guide. (n.d.). Retrieved May 19, 2021, from https://www2.mmm.ucar.edu/wrf/users/docs/user_guide_V3/user_guide_V3.8/contents.html
- Xiao, F., Okazaki, T., & Satoh, M. (2003). An Accurate Semi-Lagrangian scheme for Raindrop sedimentation. Monthly Weather Review, 131(5), 974–983. https://doi.org/10.1175/1520-0493(2003)131<0974:AASSFR>2.0.CO;2
- Yamada, H., Nasuno, T., Yanase, W., & Satoh, M. (2016). Role of the vertical structure of a simulated tropical cyclone in Its motion: A case Study of Typhoon fengshen (2008). Sola, 12(0), 203–208. https://doi.org/10.2151/sola.2016-041
- Yamada, Y., Oouchi, K., Satoh, M., Tomita, H., & Yanase, W. (2010). Projection of changes in tropical cyclone activity and cloud height due to greenhouse warming: Global cloud-system-resolving approach. Geophysical Research Letters, 37(7), 1–10. https://doi.org/10.1029/2010GL042518
- Yamada, Y., & Satoh, M. (2013). Response of Ice and liquid water paths of tropical cyclones to Global warming simulated by a Global Nonhydrostatic Model with Explicit Cloud microphysics. Journal of Climate, 26(24), 9931–9945. https://doi.org/10.1175/JCLI-D-13-00182.1
- Yamada, Y., Satoh, M., Sugi, M., Kodama, C., Noda, A. T., Nakano, M., & Nasuno, T. (2017). Response of tropical cyclone activity and structure to Global warming in a High-Resolution Global Nonhydrostatic model. Journal of Climate, 30(23), 9703–9724. https://doi.org/10.1175/JCLI-D-17-0068.1
- Yanase, W., Taniguchi, H., & Satoh, M. (2010). The genesis of tropical cyclone Nargis (2008): Environmental Modulation and Numerical predictability. 気象集誌. 第2輯, 88(3), 497–519. https://doi.org/10.2151/jmsj.2010-314
- Yang, P., Bi, L., Baum, B. A., Liou, K.-N., Kattawar, G. W., Mishchenko, M. I., & Cole, B. (2013). Spectrally consistent scattering, absorption, and polarization properties of Atmospheric Ice crystals at wavelengths from 0.2 to 100 μm. Journal of the Atmospheric Sciences, 70(1), 330–347. https://doi.org/10.1175/JAS-D-12-039.1
- Yang, P., & Liou, K. N. (1995). Light scattering by hexagonal ice crystals: Comparison of finite-difference time domain and geometric optics models. JOSA A, 12(1), 162–176. https://doi.org/10.1364/JOSAA.12.000162
- Yang, Q., Leung, L. R., Lu, J., Lin, Y.-L., Hagos, S., Sakaguchi, K., & Gao, Y. (2017). Exploring the effects of a nonhydrostatic dynamical core in high-resolution aquaplanet simulations. Journal of Geophysical Research: Atmospheres, 122(6), 3245–3265. https://doi.org/10.1002/2016JD025287
- Yoshida, R., Okamoto, H., Hagihara, Y., & Ishimoto, H. (2010). Global analysis of cloud phase and ice crystal orientation from Cloud-Aerosol Lidar and Infrared Pathfinder Satellite observation (CALIPSO) data using attenuated backscattering and depolarization ratio. Journal of Geophysical Research: Atmospheres, 115(D4), 1–12. https://doi.org/10.1029/2009JD012334
- Zängl, G., Reinert, D., Rípodas, P., & Baldauf, M. (2015). The ICON (ICOsahedral Non-hydrostatic) modelling framework of DWD and MPI-M: Description of the non-hydrostatic dynamical core. Quarterly Journal of the Royal Meteorological Society, 141(687), 563–579. https://doi.org/10.1002/qj.2378
- Zelinka, M. D., & Hartmann, D. L. (2010). Why is longwave cloud feedback positive? Journal of Geophysical Research: Atmospheres, 115(D16), 1–16. https://doi.org/10.1029/2010JD013817
- Zhang, F., Sun, Y. Q., Magnusson, L., Buizza, R., Lin, S.-J., Chen, J.-H., & Emanuel, K. (2019a). What is the Predictability limit of Midlatitude weather? Journal of the Atmospheric Sciences, 76(4), 1077–1091. https://doi.org/10.1175/JAS-D-18-0269.1
- Zhang, G., Xue, M., Cao, Q., & Dawson, D. (2008). Diagnosing the intercept parameter for exponential Raindrop size distribution based on video disdrometer observations: Model development. Journal of Applied Meteorology and Climatology, 47(11), 2983–2992. https://doi.org/10.1175/2008JAMC1876.1
- Zhang, M., Bretherton, C. S., Blossey, P. N., Austin, P. H., Bacmeister, J. T., Bony, S., Brient, F., Cheedela, S. K., Cheng, A., Genio, A. D. D., Roode, S. R. D., Endo, S., Franklin, C. N., Golaz, J.-C., Hannay, C., Heus, T., Isotta, F. A., Dufresne, J.-L., Kang, I.-S., … Zhao, M. (2013). CGILS: Results from the first phase of an international project to understand the physical mechanisms of low cloud feedbacks in single column models. Journal of Advances in Modeling Earth Systems, 5(4), 826–842. https://doi.org/10.1002/2013MS000246
- Zhang, S., Fu, H., Wu, L., Li, Y., Wang, H., Zeng, Y., Duan, X., Wan, W., Wang, L., Zhuang, Y., Meng, H., Xu, K., Xu, P., Gan, L., Liu, Z., Wu, S., Chen, Y., Yu, H., Shi, S., … Guo, Y. (2020a). Optimizing high-resolution Community Earth System Model on a heterogeneous many-core supercomputing platform. Geoscientific Model Development, 13(10), 4809–4829. https://doi.org/10.5194/gmd-13-4809-2020
- Zhang, Y., Li, J., Yu, R., Liu, Z., Zhou, Y., Li, X., & Huang, X. (2020b). A Multiscale dynamical Model in a Dry-mass Coordinate for Weather and Climate Modeling: Moist Dynamics and Its coupling to physics. Monthly Weather Review, 148(7), 2671–2699. https://doi.org/10.1175/MWR-D-19-0305.1
- Zhang, Y., Li, J., Yu, R., Zhang, S., Liu, Z., Huang, J., & Zhou, Y. (2019b). A layer-averaged Nonhydrostatic dynamical framework on an Unstructured Mesh for Global and regional Atmospheric Modeling: Model description, baseline evaluation, and sensitivity exploration. Journal of Advances in Modeling Earth Systems, 11(6), 1685–1714. https://doi.org/10.1029/2018MS001539
- Zhou, L., Lin, S.-J., Chen, J.-H., Harris, L. M., Chen, X., & Rees, S. L. (2019). Toward Convective-Scale prediction within the Next Generation Global prediction system. Bulletin of the American Meteorological Society, 100(7), 1225–1243. https://doi.org/10.1175/BAMS-D-17-0246.1
- Zhou, Y., Zhang, Y., Li, J., Yu, R., & Liu, Z. (2020). Configuration and evaluation of a global unstructured mesh atmospheric model (GRIST-A20.9) based on the variable-resolution approach. Geoscientific Model Development, 13(12), 6325–6348. https://doi.org/10.5194/gmd-13-6325-2020