Abstract
Selected crop, microclimate and pathogen variables were monitored in carrot crops for four years to identify important variables associated with the development of Sclerotinia sclerotiorum and the start of epidemics of Sclerotinia rot of carrot. Soil moisture, and occasionally soil temperature, were the variables most closely associated with the development of apothecia and ascospores. Initial development of apothecia and ascospores occurred after one week of mean soil matric potential of −20 kPa or higher and maximum soil temperature up to 24 °C. At matric potentials of −30 to −40 kPa, development of apothecia and ascospores occurred in up to two weeks, and the occurrence of apothecia and ascospores was sporadic below −40 kPa. Preliminary risk algorithms were proposed to predict the occurrence of apothecia and ascospores, the start of epidemics, and the need for initial application of fungicides. Architectural and phenological stages of carrot development were used as primary risk factors incorporated into two predictive models. Ninety-five per cent closure of the carrot canopy was selected as a critical crop threshold to activate inoculum predictors. The critical crop thresholds to activate the disease forecasting system were 100% closure of the canopy plus 70 to 80% of plants with one to two collapsed senescing leaves and one to three healthy leaves lodged on the soil. The efficacy and accuracy of the model were tested over a two-year period. Applying the fungicide boscalid according to the forecast model resulted in equivalent disease control to using calendar-based sprays and decreased the number of fungicide applications in both years by up to 80%. Predicted inoculum values from the model were correlated with observed inoculum values at commercial field sites.
Résumé
Chez la carotte, les variables relatives à la culture, au microclimat et aux agents pathogènes ont été suivies de près durant quatre années afin de déterminer celles qui étaient associées au développement de Sclerotinia sclerotiorum et à l'apparition d’épidémies de pourriture sclérotique. L'humidité du sol et parfois sa température étaient les variables le plus souvent associées au développement des apothécies et des ascospores. Le développement initial des apothécies et des ascospores s'est manifesté au bout d'une semaine au cours de laquelle le potentiel hydrique matériel du sol s’était maintenu à −20 kPa ou plus et la température du sol avait atteint un maximum de 24 °C. À un potentiel hydrique matériel de −30 kPa à −40 kPa, le développement des apothécies et des ascospores s'est produit pendant jusqu’à deux semaines, et, à moins de −40 kPa, leur occurrence était sporadique. Des algorithmes de risque préliminaires ont été proposés afin de prédire l'occurrence des apothécies et des ascospores, le début des épidémies et la nécessité de procéder à une première application de fongicides. Les stades architectural et phénologique du développement de la carotte ont été utilisés à titre de facteurs primaires de risque et intégrés à deux modèles de prévision. Une fermeture de 95% du couvert végétal des carottes a été utilisée comme seuil critique de la culture pour activer les prédicteurs de l'inoculum. Les seuils critiques de la culture qui activaient la prédiction de la maladie étaient la fermeture complète (100%) du couvert végétal ainsi qu'une proportion de 70 à 80% de plants comprenant une ou deux feuilles sénescentes flétries et une à trois feuilles saines couchées sur le sol. L'efficacité et la précision du modèle ont été testées pendant deux ans. L'application de boscalide en fonction des prévisions du modèle a contribué à prévenir la maladie tout autant que les pulvérisations prévues au calendrier et à diminuer jusqu’à 80% le nombre d'applications de fongicide chaque année. Les valeurs prévues de l'inoculum par le modèle ont été corrélées avec celles qui ont été observées dans les cultures commerciales.
Introduction
Sclerotinia sclerotiorum (Lib. de Bary) can cause significant damage to a number of crops, including carrot (Boland & Hall, Citation1994; Kora et al., Citation2005 a). Sclerotinia rot of carrot (SRC) affects both foliage and roots and has a bicyclic pattern of development (Kora et al., Citation2003). Pre-harvest, the disease is mainly observed on senescing and lodged leaves within the canopy, but root disease primarily develops in post-harvest storage (Geary, Citation1978; Kora et al., 2003, 2005a). The primary inoculum for SRC is airborne ascospores produced from apothecia that typically develop under a closed plant canopy. Ascospores require an external nutrient source to infect healthy tissue. In carrots, this nutrient source comes from senescing foliage (Geary, Citation1978; Kora et al., Citation2005 a). Fungicides can be used to effectively control disease but routine applications of fungicides are considered cost-effective only in high value crops, such as vegetables, and sometimes in heavily infested field crops (Steadman, Citation1979).
Similarly to other crops, development of S. sclerotiorum and epidemics of SRC occur sporadically in time and space (Kora et al., 2005a). Forecasting models have, therefore, been developed for a number of diseases caused by S. sclerotiorum to determine the need for or timing of fungicide application (Hunter et al., Citation1984; Turkington & Morrall, Citation1993; Twengstrom et al., 1998; Bom & Boland, Citation2000; McDonald & Boland, Citation2004). Models for forecasting stem rot in rapeseed utilize a combination of variables such as rainfall, soil moisture, canopy density, level of infestation of petals by S. sclerotiorum, crop history and patterns of blooming, to predict inoculum presence, disease risk or allow for better timing of fungicidal application (Turkington & Morrall, Citation1993; Twengstrom et al., 1998; Bom & Boland, Citation2000). Forecast models for rapeseed utilizing factors of petal infestation are limited due to a time delay as petal testing requires three to five days to determine if inoculum is present (McLaren et al., Citation2004). More recently, air temperature, relative humidity, rainfall and sunshine duration were used to calculate an index of infection hours, and this variable was incorporated into a two-tiered forecasting model for winter oilseed rape (Koch et al., Citation2007). In bean crops, forecasting systems have utilized measurements of soil matric potential, canopy density and flowering (Hunter et al., 1984; McDonald & Boland, Citation2004).
Forecast models that do not include variables related to the pathogen often assume the continuous presence of inoculum based on the field history (Hunter, Citation1981). However, measurement of ascosporic inoculum provided more consistent results and improved the efficacy of predictive models, particularly when external inoculum was important for disease development (Gugel & Morrall, Citation1986; Turkington & Morrall, Citation1991, 1993; Bom & Boland, Citation2000). A predictive model for carpogenic germination of sclerotia of S. sclerotiorum has also been successfully developed as part of a disease forecasting system for Sclerotinia disease in lettuce (Clarkson et al., Citation2004, Citation2007). Typically, quantification of ascospores or apothecia within crops may qualify as a measure of available inoculum when a direct relationship is observed between inoculum and disease incidence (Gugel & Morrall, Citation1986; Boland & Hall, Citation1988 a, Citation1988 b). In carrots, ascospores, rather than apothecia, were more consistently present in fields where SRC developed and may be a better predictor for timing fungicide applications (Kora et al., 2005a).
Most disease prediction models proposed for Sclerotinia diseases have been based on qualitative measures. Disease risk algorithms have been widely used to develop forecast models with satisfactory applications in the field (Hunter, Citation1981; Thomas, Citation1984; Yuen et al., Citation1996; Steadman & O'Keefe, Citation1998; Twengstrom et al., Citation1998; Langston et al., Citation2002). The term ‘algorithm’ refers to any computation that uses observations of one or more components of the disease triangle: the host crop, pathogen and environment, to make an assessment of the need for crop protection measures (Hughes et al., Citation1999). Risk algorithms consist of scales of risk points assigned to risk factors that are significant for the epidemiology of disease. The risk points are either summed (additive models) or multiplied (multiplicative models) and the outcome score is compared to a predetermined action threshold that indicates the development of a certain disease level in the field. Spray applications are deemed necessary if the scores exceed the action threshold. Risk assessment can be a one-time action for decisions whether to apply a fungicide (Twengstrom et al., Citation1998), or a multiple-time action for decisions on the timing of the first and subsequent fungicide applications (Hughes et al., Citation1999).
The objectives of this study were to: (i) examine quantitative relationships among selected variables of environment, host and pathogen and examine their relationship to the development of SRC; (ii) use the variables that possess epidemiological significance to develop preliminary risk algorithms for forecasting the occurrence of inoculum of S. sclerotiorum, and for timing fungicide application; (iii) examine the efficacy of fungicide sprays based on the forecast models compared with calendar-based sprays, and (iv) determine the effectiveness of the forecast models to predict the occurrence of S. sclerotiorum inoculum.
Materials and methods
Epidemiology and validation plots
Carrot plots for studying the epidemiology of SRC were located at the Muck Crops Research Station (MCRS) and in commercial fields in the Holland/Bradford Marsh, Ontario (44° 15′ N latitude, 79° 35′′ W longitude). All plots were on organic (muck) soil (60% organic matter, pH 5.5–6.5) that was naturally infested with S. sclerotiorum. Epidemiology plots at the MCRS (13.7 × 36 m) (Plots 1, 3) and commercial fields (25 × 40 m) (Plots 2, 4) were established in 1999 and 2000 to develop the forecast model (). To validate the efficacy of fungicide spray regimes based on the forecast model, experimental validation plots (13.7 × 60 m) were established at the MCRS in 2004 (Plot 5) and 2005 (Plot 11). The efficacy was tested at these plots by comparing spray regimes based on the forecast model with calendar spray regimes. Carrot ‘Enterprise’ was used at the MCRS validation plots because this cultivar produces a thick canopy compared with other available carrot cultivars. Excess foliar growth can increase shading and senescence of leaves (Couper, Citation2001), and promote carpogenic germination of sclerotia (Sun & Yang, Citation2000; Kora et al., 2005a). Validation plots in commercial fields were also established in 2004 (Plot 6–10) and 2005 (Plots 12–16). Each plot consisted of 50 × 40 m sections within each carrot field and was located a minimum of 25 m from the edge of the field along all borders. A total of 10 plots were established with five in each year. Plots were 0.7 to 4.7 km from the Muck Crops Research Station. Seeding dates and cultivars of all plots are summarized in .
Table 1. Type, number, location, year, cultivar, and planting and harvest dates for all epidemiology and validation research plots from 1999–2005
All MCRS plots were direct seeded at approximately 90 seeds m−1 of row on raised trapezoid beds, 86 cm apart from centre-to-centre using a precision seeder. In each bed, three rows of carrot seeds were sown approximately 5 cm apart. The plots were maintained following Ontario standard management practices (Anonymous, Citation2004), with the exception that fungicides were only applied according to the experimental design. Soil fertilization included pre-plant incorporation of 60 kg ha−1 nitrogen, 20 kg ha−1 phosphorus and 40 kg ha−1 potassium. Pre-emergence (Gesegard at 5 L ha−1; Novartis Crop Protection Canada Inc., Guelph, ON) or post-emergence (Lorox at 750 g ha−1; DuPont Canada Inc. Mississauga, ON) broad-leaf herbicides were used in combination with hand weeding for weed control.
All commercial plots were selected within commercial carrot fields (). These were direct seeded by the growers using precision seeders (45 to 48 seeds m−1 of row) on raised trapezoid beds 72 cm apart from centre-to-centre. In each bed, two or three rows of carrot seeds were sown. Plots were maintained by the growers following Ontario standard management practices.
Scouting for apothecia, crop factors and disease incidence was conducted along pre-determined diamond-shaped patterns with sides of 20 and 24 m within all plots except Plots 5 and 11. Eight sample areas were established in 1999 and 2000, and five in 2004 and 2005. Each sample area was located at random intervals along a diamond-shaped counter-clockwise scouting pattern and consisted of a 2 m (1999 and 2000) or 5 m (2004 and 2005) section of carrot bed that extended from the middle of one furrow to the middle of the next furrow. A furrow was defined as the area extending between the middles of two adjacent raised carrot beds (Kora et al., 2005a).
Crop phenological stages specified by the forecast model, specifically canopy closure and foliar senescence, were monitored at weekly intervals from the first week of July until harvest at MCRS in all years. Canopy closure was monitored from the first week of August until harvest in 2004 and from the first week of July until harvest in all other years at all commercial field plots. Canopy coverage was rated visually using a standard area diagram to estimate the percentage of soil covered by the carrot foliage (Kora, Citation2003). Canopy closure was defined when the percentage of the soil covered by the carrot foliage was above 95% when viewed from above. Digital imaging software was used to confirm the accuracy of this method (APS Assess, American Phytopathological Association, St. Paul, MN, USA). The per cent of leaf lodging was determined by counting the number of senesced, collapsed leaves per plant, lodged on the soil. Counts were conducted on 10 plants per sampling area and a mean value was reported for each sample area.
Assessment of microclimate
Air temperature (T), relative humidity (RH), rainfall, leaf wetness duration of topmost, exposed leaves and lower, shaded leaves under the canopy (LWD), soil T and soil moisture (M) were recorded between 8 July and 15 October using sensors and microloggers located within each carrot plot. Unless otherwise noted, all sensors and microloggers were obtained from Campbell Scientific, Inc. (Logan, UT, USA).
Atmospheric variables of air T and RH were measured at a height of c. 10 cm above the carrot canopy. Air T and RH were measured using a thermistor probe (Model HMP 35C) housed in a protective case. Rain was measured in increments of 0.25 mm using a tipping bucket rain gauge (Model TE525). Leaf wetness was estimated using flat electronic impedance grid sensors (Model 237) coated with light green latex paint (Gillespie & Kidd, Citation1978). Leaf wetness sensors were mounted on individual wooden stakes and positioned at a 45° angle to the canopy surface and under the canopy, 50 and 10 cm above the soil surface, respectively.
Soil variables, including soil T and soil M, were measured at 5 cm below the soil surface. Soil T was measured using a thermistor probe (Model 107B) embedded in a plastic waterproof coating and buried horizontally 5-cm deep in the soil. Soil T at each validation plot was determined using a Red Flag temperature data logger (Datatherm Inc. Pickering, ON, Canada) buried horizontally at a depth of 5 cm. In 1999, soil M was measured twice a week by gravimetric determinations. Per cent gravimetric water content was converted to per cent volumetric water content based on the specific bulk density of the organic soil (0.21 g cm−3) determined for each plot (Kora, Citation2003). In 2000, volumetric soil M was measured using a ThetaProbe sensor Type ML2x (Delta-T Devices Ltd. Cambridge, UK) buried horizontally in soil so that the centre of the cylindrical sensor was c. 5 cm below the surface. Percentage soil M data obtained from the gravimetric method and the ThetaProbe were converted into soil matric potential (SMP) using a standard soil water desorption curve generated for each epidemiology plot (Richards, Citation1965; Kora, Citation2003). Sensors were connected to a Micrologger Model CR 21X programmed to sample at one-minute intervals and record averaged or summed (rain gauge) readings over 15-minute intervals. Monitoring instrumentation was calibrated prior to installation each year.
Assessment of inoculum of S. sclerotiorum
The occurrence of apothecia and ascospores was monitored in all plots. The total number of apothecia present in each sample area was counted at every sampling date. The mean number of apothecia m−2 from each sample area was used as an estimate of apothecia population on respective sampling dates for each plot.
The presence of airborne inoculum within the plots was monitored using Petri plates of 90-mm diameter containing 20 mL Sclerotinia semi-selective medium (SSM) as spore traps (Steadman et al., Citation1994; Steadman & O'Keefe, Citation1998; Kora et al., 2005a). The medium consisted of 39 g of PDA L−1 deionized water amended with 25 mg L−1 of PCNB (75% pentachloronitrobenzene), 150 mg L−1 penicillin G, 150 mg L−1 streptomycin sulphate and 50 mg L−1 bromophenol blue. One open Petri plate containing SSM was exposed in each sampling area of the plot for three consecutive hours, between 09:00 to 14:00 h. The plates were placed on top of the carrot bed under the canopy at a 45° angle, facing upwind to the direction of prevailing winds. Following exposure, the exposed plates were incubated at 20 ± 1 °C in the dark and evaluated after three to five days for the presence of colony forming units (CFU). Each CFU was considered to be derived from a single germinating ascospore of S. sclerotiorum and was readily identified based on the characteristic yellow halo that formed around the developing colonies. Colonies were observed for the formation of characteristic sclerotia to confirm identification.
Assessment of disease
The incidence of SRC on foliage was defined as the percentage of plants in the sample area that had at least one diseased petiole. Disease incidence was determined by identifying symptoms including water-soaked, dark olive-green lesions, actively growing mycelia or sclerotia of S. sclerotiorum on carrot leaves. Diseased tissues were sampled regularly during the growing season and cultured to confirm the presence of S. sclerotiorum. Disease incidence in storage represented the percentage of roots in the sample with at least one lesion of SRC.
Statistical analyses used to develop the forecast model
Independent variables were derived from the measurements of each microclimate factor averaged or summed over selected periods coinciding with pathogen and disease sampling dates. These variables included daily mean, minimum and maximum air and soil T, daily mean RH, and daily mean SMP averaged over three-, two- and one-week periods, and cumulative rainfall during three-, two- and one-week periods, preceding the sampling dates. The weekly mean SMP, calculated as the mean of the SMP measurements on the day of sampling and one week prior to sampling (Hunter et al., 1984), and cumulative days with LWD of >15 and >20 h on exposed and shaded leaves during the week preceding sampling dates, were also tested. Means of SMP in 1999 were calculated from single daily measurements recorded twice a week. Dependent variables included weekly observations of the number of apothecia, number of CFUs and incidence of SRC.
Critical microclimate variables for the occurrence of apothecia, ascospores and disease in carrot crops were assessed using a step-by-step evaluation process. A Pearson correlation analysis was used to examine linear correlations among all variables. Correlation analyses were performed in a two-step process: (1) individually for each epidemiology plot, and then (2) for pooled crops. Variables within microclimate factor groups that had relatively higher coefficients of correlation (P < 0.05) on a crop or pooled crop basis were selected for further assessment. Statistical analyses were performed using the PROC CORR procedure of SAS (Statistical Analyses System) for Windows, Ver. 8.1 (SAS Institute Inc., Citation1999).
Risk algorithms
Preliminary algorithms were developed as empirical qualitative predictors (Madden & Ellis, Citation1988) of the risk of S. sclerotiorum or SRC based on the results of the variable selection process from four carrot plots in 1999 and 2000. These algorithms were designed as single season prediction models to predict the likelihood of the occurrence of initial inoculum (e.g. presence of apothecia or ascospores) and the start of SRC epidemics. These models were based on cumulative weather conditions summarized in the form of arbitrary, ordinal values that represented the overall suitability of environmental conditions for pathogen or disease development. Risk factors selected for the algorithms were crop and microclimate variables that were most closely correlated with the respective dependent variables. Risk points (0–3) were assigned to each risk factor to estimate the relative weight that the factor contributed to the model. A seven-day severity index (SDSI) was computed by multiplying the crop and microclimate risk points for any given day, and then summing the daily scores over the seven preceding days (Langston et al., 2002).
Determining the efficacy of the forecast model
Spray regimes were tested for their efficacy in field plots planted in a randomized complete block design with four treatments and six blocks in both 2004 (Plot 5) and 2005 (Plot 11). Treatment plots consisted of two adjacent rows of carrots, 10 m in length. Adjacent treatments within blocks were separated by two guard rows that received no fungicide applications, to reduce interplot effects. The first 5 m of each treatment area was defined as the sampling area. In each sampling area, the occurrence of inoculum of S. sclerotiorum, percentage soil shaded by the canopy, and the number of senescing and lodged leaves was recorded weekly. The second 5 m of each plot was left undisturbed during the growing season and carrots in this portion of the plot were used to determine the incidence of disease incidence at harvest, yield and disease incidence following six months in storage. These sections were left undisturbed until harvest to prevent scouting activities from interfering with disease development. Blocks were separated from each other by 1.5 m of bare soil. Carrots harvested from the undisturbed sections were placed in plastic containers in temperature-controlled Filacell storage at 2 ± 1 °C and 95 ± 2% RH after harvest in both years. In 2005, carrot roots were also individually stored in Deepot D60LW plastic tree seedling cells (Stuewe & Sons Inc., Oregon, USA) to determine disease progress throughout the storage period without disturbing the carrots, and to prevent secondary spread of infection from one carrot to another.
Four fungicide spray regimes were tested to time the application of the fungicide boscalid (Lance WDG, BASF, Toronto, Canada). In Citation2004, boscalid was applied at 220.5 g active ingredient (a.i.) ha−1, which is the current recommended rate for the use of boscalid in the management of carrot leaf blights in the United States (BASF, 2004). In 2005, this rate was doubled (441 g a.i. ha−1) to attain better disease control as this was within the range of the recommended rate for the control of diseases caused by S. sclerotiorum in other crops in the United States (BASF, 2004). The four treatments included: (1) an untreated control where no fungicide was applied; (2) a calendar-timed spray regime where fungicides were applied every two weeks, beginning when the canopy was 95% closed; (3) a spray regime based on the forecast model with an inoculum threshold of 5 CFU plate−1 (Model 1); and (4) a spray regime based on the forecast model with a mean SMP at a depth of 5 cm > −30 kPa recorded over the previous two weeks (Model 2).
A higher threshold level for ascospores (>10 CFU plate−1) was originally established as an indication of the onset of significant amounts of deposited ascospores (Kora et al., 2005a). However, the threshold value for the Forecast 1 spray regime was reset at 5 CFU plate−1 to ensure the treatment would receive at least one fungicide application in each year of the study.
Determining the accuracy of the risk algorithm
To determine the accuracy of the risk algorithm in predicting the quantity of inoculum of S. sclerotiorum, SDSI values from the forecast model were compared with measured levels observed in commercial carrot plots. At each plot the SDSI was calculated using measurements of SMP at a depth of 5 cm, canopy closure and soil T at a depth of 5 cm. Soil matric potential data were collected at the MCRS in both years.
Statistical methods used for model validation
Analysis of variance (ANOVA) was used to determine if significant differences existed among treatments for number of apothecia observed, total number of CFU plate−1 observed in the growing season, per cent canopy closure, disease incidence pre-harvest, carrot yield and disease incidence following six months in storage. ANOVA was also used to determine if significant differences existed among the CFU values detected at the established risk levels in commercial plots. Means separation was conducted using Tukey's HSD test.
Disease progress was analyzed using linear regression to determine the fit to five temporal population growth models used commonly in the analysis of plant disease epidemics: linear, exponential, monomolecular, logistic and Gompertz (Zadoks & Schein, Citation1979). The model with the highest coefficient of determination (r2) and lowest mean square of error after transformation of data was chosen to describe the disease progress curve.
Spearman's rank correlation was used to determine correlations between SDSI values calculated by the two models of the risk algorithm and the observed daily mean CFU plate−1 and apothecia m−2 from commercial plots. The coefficient of determination (rs) for Spearman's rank correlation was used to determine the relative strength of the risk algorithm in predicting the occurrence of inoculum because both the SDSI values from the risk algorithm and measurements of inoculum were ordinal and non-parametric. After inoculum thresholds were established, statistical analyses were conducted using Statistix 7.0 (Analytical Software, Tallahassee, USA).
Results
Influence of microclimate on apothecia development
Apothecia were detected in three of four of the carrot plots in 1999 and 2000 (Plots 1–3). The initial appearance of apothecia ranged from 4 August to 15 September, or 65 to 110 days after seeding (DAS) with a mean of 88 DAS, and always occurred after the crop canopy closed. In general, the development of apothecia was correlated with air T, soil T and soil M variables in the 1999 epidemiology Plot 1, and occasionally with soil T in pooled crops (). Correlations were more consistent across soil T and soil M variables, therefore, only these variables are discussed and further analyzed.
Table 2. Correlations among the number of apothecia (APO), number of colony forming units (CFU) of deposited ascospores, and incidence of Sclerotinia rot of carrot (SRC) and variables of air temperature (T), soil T and soil matric potential (SMP) in individual and pooled epidemiology carrot plots in Bradford Marsh, Ontario in 1999 and 2000
In the 1999 epidemiology crop (Plot 1), occurrence of apothecia was negatively correlated with the daily mean, minimum and maximum soil T over the three-, two- and one-week periods preceding sampling (). Daily mean and maximum soil T yielded the highest coefficients of correlation in all assessed intervals, and these were further analysed. Daily mean soil T averaged 18.7 and 19.2 °C, and daily maximum soil T averaged 23.3 and 23.9 °C, during one and two weeks preceding the initial detection of apothecia, respectively. On a seasonal basis, apothecia were associated with daily mean and daily maximum soil T ranging from 13 to 21 °C and 16 to 25 °C during the preceding one to two weeks, respectively. Higher numbers of apothecia were associated with daily mean and daily maximum soil T between 16 to 20 °C and 21 to 25 °C, respectively.
In the 1999 epidemiology crop (Plot 1), occurrence of apothecia was positively correlated with daily mean SMP over three-, two- and one-week periods, and the weekly mean SMP preceding sampling (). On a seasonal basis, apothecia were associated with daily mean SMP ranging from −750 to −10 kPa during the preceding one and two weeks (data not shown). Higher incidence of apothecia presence was associated with SMP of −30 to −10 kPa.
No correlations were observed between soil T and soil M variables and apothecia in other plots (). Values of daily mean SMP of 0 to −40 kPa, daily mean soil T of <25 °C and daily maximum soil T of <30 °C summed over seven-day intervals were selected as important microclimate variables to develop the risk algorithm for predicting the occurrence of apothecia.
Influence of microclimate on ascospore development
Ascospores were detected as CFUs on SSM plates in all four carrot plots in 1999 and 2000. The initial appearance of ascospores ranged from 18 July to 16 August, or 48 to 86 DAS with a mean of 70 DAS, and occurred before or after the crop canopy closed. In general, development of ascospores was most consistently correlated with SMP variables in individual and pooled crops (), therefore, only these variables are discussed and further analysed.
Occurrence of ascospores was positively correlated with daily mean SMP over three-, two- and one-week periods, and with weekly mean SMP preceding sampling (). The weekly mean SMP in the week prior to ascospore detection averaged −20 kPa, and over two weeks averaged −40 kPa. On a seasonal basis, ascospores were associated with daily mean SMP during one to two weeks ranging from −75 to −10 kPa, and a higher concentration of ascospores (>10 CFU plate-1) occurred at −30 to −10 kPa (data not shown). Daily mean SMP of 0 to −40 kPa summed over seven-day intervals was the most important factor to develop risk algorithms for predicting the occurrence of ascospores.
Influence of microclimate on disease development
Sclerotinia rot was detected in three of the four carrot plots in 1999 and 2000 (Plots 2–4). The initial appearance of disease ranged from 18 August to 18 September, or 80 to 126 DAS with a mean of 106 DAS. Disease occurred after senescing leaves started to collapse and healthy leaves started to lodge in the furrow, and after ascospores appeared in the field. In general, development of disease was consistently correlated with variables of air and soil T in all individual and pooled crops, and occasionally with soil M in Plot 1 ().
In almost all plots, disease incidence was negatively correlated with daily mean, minimum and maximum air and soil T during the three-, two- and one-week periods preceding sampling (). Higher correlation coefficients were obtained for daily maximum air and soil T in most intervals examined, therefore, only these variables were identified for further analysis. Analysis of pooled means indicated that daily maximum air T over two- and one-week periods prior to disease detection averaged 26.7 °C and 26.2 °C, respectively. Similarly, daily maximum soil T over two- and one-week periods prior to disease detection averaged 25.0 °C and 25.4 °C, respectively. The means of these variables differed by c. ± 1 °C across intervals and sampling dates within respective microclimate factors, with no discriminating pattern. Therefore, no critical value or period range was identified in association with disease development. On a seasonal basis, daily mean and maximum air T over one to two weeks prior to disease detection ranged from 5 to 19 °C and 12 to 31 °C, respectively. Higher incidence of disease was associated with daily mean and maximum air T between 12 to 18 °C and 17 to 27 °C, respectively. The daily mean and maximum soil T during one to two weeks preceding disease ranged from 8 to 20 °C and 13 to 25 °C, respectively (data not shown).
Disease incidence in one crop in 1999 (Plot 2) was positively correlated with daily mean SMP during three-, two- and one-week periods preceding disease assessment (). Daily mean SMP over the three-, two- and one-week periods prior to initial detection of disease across all sampling dates ranged from −40 to −90 kPa with no discriminating pattern. Therefore, no critical value or period range could be identified in association with disease development.
Variables of RH and rainfall were not correlated with the number of apothecia, number of deposited ascospores, or disease incidence, therefore, these data were not reported.
Development of risk algorithms
Several crop and microclimate risk factors were selected to construct algorithms for predicting the risk of apothecia and ascospores in carrot crops. These factors were per cent coverage of soil by the carrot canopy, daily mean SMP and daily maximum or mean soil T. A risk point from a scale of 0 to 3 was assigned to describe the expected contribution of each selected sub-range of crop and microclimate factors for any given day (), with a higher point indicating a higher contribution. The final step for constructing the risk algorithms was to generate severity index values for seven-day intervals based on the risk points and risk factors using the following models:
Table 3. Crop and microclimate risk factors, factor sub-ranges, and corresponding multiplier values of risk points used to calculate risk for the occurrence of apothecia and ascospores a of S. sclerotiorum
where SDSI is the seven-day severity index, CG is the daily risk point of the canopy growth sub-range; SMP is the daily risk point of the SMP sub-range; and ST is the daily risk point of the soil T sub-range. The primary difference between the models was the inclusion of soil temperature in Model 1.
Calculation of SDSI was started when the carrot canopy was approaching 95% soil coverage. The critical crop threshold for the SDSI to accrue values >0 was canopy coverage >95%. The critical microclimate thresholds for the SDSI to accrue values >0, after the crop threshold was satisfied, were mean daily SMP of −40 kPa and mean soil T of <25 °C. Values of SDSIs for ascospore prediction were designed to incorporate canopy growth and SMP parameters.
Validation of the forecast model
Efficacy of spray regimes
The threshold for leaf senescence and lodging was met at the MCRS plots prior to 95% canopy closure in both years (Plots 5, 11). Peak levels of inoculum occurred earlier in the growing season and the magnitude of the measured inoculum was greater in 2004 than 2005.
In 2004, the disease progress curves for all spray regimes followed a monomolecular growth model from 29 July to 30 September (R2 ≥ 0.94; P < 0.01). No SRC was observed at the MCRS plot from 4 October 2004 until harvest (21 October 2004). In 2005, SRC was observed from 5 August to 23 August. Disease symptoms were not observed from 29 August 2005 to 28 September 2005. Disease was again observed on 5 October 2005 until harvest on 21 October 2005.
In 2004, spray regimes had no significant effect on area under the disease progress curve (AUDPC), number of apothecia m−2, CFU plate−1, total yield, marketable yield or disease incidence post-harvest following six months in storage (data not shown). As indicated above, disease was not observed at harvest in 2004, probably because of the dry conditions in October of that year.
Following Model 1 (one spray) and Model 2 (three sprays) resulted in a reduction of four and two fungicide applications compared with the calendar spray regime (five sprays) in 2004, respectively. However, none of the regimes had an effect on disease development.
In 2005, the application of fungicides resulted in a significant reduction in disease incidence at harvest and AUDPC () compared with the non-treated check. All fungicide regimes were equally effective. Model 1 called for two fungicide applications compared to five for the calendar spray regime and Model 2. Spray regimes had no effect on number of apothecia m−1, CFU plate−1, total yield or marketable yield (data not shown). Disease was not observed post-harvest following six months in storage.
Fig. 1. Effect of fungicide application on (a) percentage of carrots with symptoms of SRC at the time of harvest and (b) area under the disease progress curve (AUDPC) in 2005 (Plot 11). Four spray regimes were tested: check received no fungicide application, Model 1 followed timing of fungicide application with a threshold of >5 CFU plate−1, Model 2 followed timing of fungicide application with a threshold of > −30 kPa for two-week mean soil matric potential (SMP). Calendar timed spray regime received fungicide applications from the start of canopy closure at two-week intervals. Bars with the same letter are not significantly different at P ≤ 0.05, Tukey's HSD test.
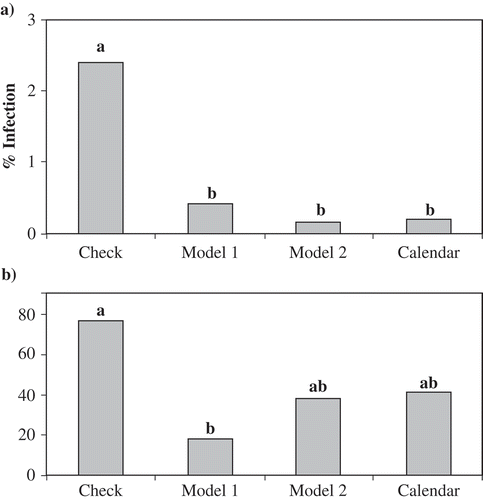
Accuracy of the risk algorithm
The percentage of soil shaded by the carrot canopy and soil T at a depth of 5 cm were field-specific factors used in the risk algorithm to determine the SDSI for each field. Soil T at a depth of 5 cm was found to be relatively uniform among all five commercial plots in both years. Soil T at a depth of 5 cm was below 19 °C for most of the 2004 growing season and, as a result, the daily risk point for soil T was often at the maximum of 3.
The percentage of carrots showing symptoms of foliar SRC varied among the field plots in this study. Disease severity was higher in 2004 than 2005. In 2004, four of five field plots had more than 50% of carrots showing symptoms of SRC at one point during the growing season, while none had more than 50% in 2005. The highest disease incidence in 2005 was 35.9%.
Disease progress data from each field plot best fit either the logistic or monomolecular models. In 2004, disease progress followed a logistic model at all plots (R2 ≥ 0.91; P < 0.01). In 2005, disease progress followed a logistic model at Plot 12 (R2 = 0.95; P < 0.01), Plot 14 (R2 = 0.88; P < 0.01) and Plot 16 (R2 = 0.81; P < 0.01), and a monomolecular model at Plot 13 (R2 = 0.90; P < 0.01) and Plot 15 (R2 = 0.83; P < 0.01).
Disease was observed and peaked at different times in 2004 and 2005. In 2004, scouting did not begin until 9 August due to weather conditions. Disease was first observed between 9 August and 24 August, but may have started earlier in the growing season. Disease severity peaked on 28 September 2004 at all plots. From 7 October until harvest, no symptoms of disease were observed in 2004. In 2005, disease was first observed between 18 August and 24 August and disease severity was highest at harvest.
Both risk algorithms were accurate in their prediction of CFU plate−1. In 2004, the calculated SDS values from Model 1 and Model 2 were correlated with both CFU plate−1 (rs = 0.79, P < 0.01) and apothecia m−2 (rs = 0.58, P < 0.01) (). At all commercial plots in 2004, SDSI values from both models were correlated with CFU plate-1 (rs ≥ 0.75, P < 0.05). However, SDSI values from both models were correlated with apothecia m−2 for only two of five plots (Plots 7, 10) ().
Table 4. Correlations between predicted severity values for inoculum of S. sclerotiorum and detected inoculum in commercial plots in 2004 and 2005
In 2005, the combined data for all plots showed that SDSI values from Model 1 and Model 2 were correlated with CFU plate−1 (rs = 0.49, P < 0.01; rs = 0.46, P < 0.01), respectively. When data for individual plots were examined, SDSI values from both models were correlated with CFU plate−1 at three of five plots (Plots 12, 13, 15). Apothecia were observed at only two of the five plots (Plots 13, 15) and SDSI values from both models were not correlated with apothecia m−2 ().
When data were combined from all 10 commercial plots in the study, the SDSI were correlated with apothecia m−2 and CFU plate−1. The SDSI values from Model 1 were correlated with observed inoculum (ascospores: rs = 0.63, P < 0.01; apothecia: rs = 0.38, P < 0.01) and the results were similar with Model 2 (ascospores: rs = 0.61, P < 0.01; apothecia: rs = 0.37, P < 0.01) ().
Establishment of thresholds for the risk algorithm
To establish threshold values for Model 1 of the risk algorithm, the predicted SDSI values and detected levels of ascospores were examined and three ranges of inoculum were detected (data not shown). When the SDSI values ranged from 0 to 32, ascospores were detected, but at levels below the critical threshold established by Kora et al. (2005a). When the SDSI ranged from 33 to 44, the number of ascospores detected was above the critical threshold of 10 CFU plate−1. When the SDSI ranged from 45 to 63, ascospore numbers exceeded the critical threshold in a larger proportion of the observations. These ranges in SDSI values represented three levels of relative risk of high spore level: ‘Low’ indicating that disease risk was present, but management strategies were not yet required; ‘Medium’ indicating that risk of disease development was present and management strategies could be required; and ‘High’ indicating that risk of disease development was likely and management strategies should be implemented. Two thresholds were established in the risk algorithm, one at 33 ≤ SDSI ≤ 44 and one at SDSI ≥ 45. Significantly more ascospores were detected at the ‘Medium’ risk level than the ‘Low’ risk level. At the ‘High’ risk level, significantly more ascospores were detected than at both the “Low’ and ‘Medium’ risk levels ().
Fig. 2. Mean number of ascospores observed at different risk levels calculated from the risk algorithms. CFU plate−1 = mean number of CFU observed from all commercial field plots in both 2004 and 2005. a, Model 1 includes factors of canopy closure, soil matric potential and soil temperature. b, Model 2 includes factors of canopy closure and soil matric potential. Risk levels for Model 1 are: Low = SDSI < 33, Medium = 32 < SDSI < 45, High = SDSI >44; risk levels for Model 2 are: Low = SDSI < 11, Medium = 10 < SDSI < 14, High = SDSI >13. Prior to analysis, CFU plate−1 was log transformed. Bars with the same letter are not significantly different at P ≤ 0.05, Tukey's HSD test.
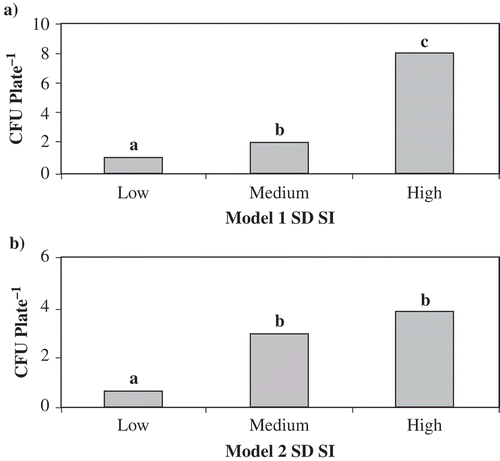
To establish threshold values for Model 2 of the risk algorithm, the predicted SDSI values and detected levels of ascospores were again examined. Large values >10 CFU plate−1 were detected only when SDSI from the risk algorithm were >15. Ascospores were detected at SDSI < 11 and when the SDSI was 0, therefore, some risk of SRC was present. When the SDSI was >15, 32% of mean CFU values (6 of 19) were >10 CFU plate−1. Three ranges of inoculum were observed. When the SDSI ranged from 0–10, ascospores were detected, but at levels below 10 CFU plate−1. When the SDSI ranged from 11–14, the number of ascospores detected was above the critical threshold in some observations. When the SDSI ranged from 15 to 21, ascospore numbers exceeded the critical threshold in a larger proportion of the observations. Three ‘risk levels’ were established for Model 2: ‘Low’ at SDSI < 11, ‘Medium’ at 11 ≤ SDSI ≤ 14, and ‘High’ at SDSI ≥ 14. Significantly more ascospores were detected at the ‘Medium’ risk level than the ‘Low’ risk level, but there was no significant difference detected between the ‘High’ and ‘Medium’ risk levels ().
Periods of reduced disease incidence in 2004 and 2005
Symptoms of SRC were not visible during all periods of the epidemic. In 2004, disease progress slowed during periods of dry weather starting on 9 September. During 14–28 September 2004, white mycelium of S. sclerotiorum was no longer visible on petioles, and plants were diagnosed with SRC based on the presence of water-soaked lesions and sclerotia. After 28 September 2004, petioles previously water-soaked were dry and no external signs of disease were present. Most infected petioles had senesced and abscised from the crown.
At the MCRS plot in 2005, disease symptoms were visible from 5 to 23 August, but symptoms were no longer visible from 29 August until 28 September 2005. Disease symptoms were detected again on 5 October 2005. Petioles with symptoms of SRC dried up and abscised from the plant. No period of dry weather was associated with this reduction in disease. The disappearance of disease symptoms did not occur at commercial plots in 2005. However, disease was observed in commercial plots later in the growing season than in the MCRS plot.
Disease was not observed in stored carrots from the commercial plots in either year. No correlation was observed between disease incidence pre- and post-harvest.
Discussion
This research builds on previous research on the epidemiology of SRC (Kora et al., 2005a). The two disease-forecasting models were developed based on risk algorithms that predicted the occurrence of inoculum. Soil moisture and occasionally soil temperature were the variables most closely associated with the development of apothecia and ascospores. Applying the fungicide boscalid according to forecast Model 1 resulted in a decreased number of fungicide applications from five to one in 2004 and from five to two in 2005 and provided equivalent disease control to using calendar-based sprays. Predicted inoculum values from the model were correlated with the observed inoculum values at commercial field sites.
The SRC forecast models developed in this study are similar to other models for disease caused by S. sclerotiorum in other crops. Three common variables included in forecast models for bean, canola and carrot are the history of disease caused by S. sclerotiorum, soil moisture and crop susceptibility (Hunter et al., 1984; Turkington, Citation1988; Bom & Boland, Citation2000; Kora, Citation2003). In bean, soil matric potential was a better predictor than rainfall, and one- or two-week periods of soil matric potential > −30 kPa in the top 3–4 cm of soil was associated with the occurrence of disease (Hunter et al., 1984). In the SRC forecast model, one suggested microclimatic threshold is a mean soil matric potential > −30 kPa for the previous two weeks. However, the use of soil matric potential as the sole microclimatic variable resulted in an excess of fungicide sprays in this study in 2005. Including soil T data increased the correlation between SDSI and CFU plate−1 by a small amount. The availability of soil moisture appears to be more important for carpogenic germination at temperatures over 18 °C (Sun & Yang, Citation2000). Disease suppression was achieved with Model 1, Model 2 and the calendar spray regime in 2005. The most efficient spray regime was Model 1, which included soil temperature as a variable. Spray timing based on Model 1 resulted in the lowest number of fungicide applications with equivalent efficacy to calendar spray regimes in both years. These forecast models involve labour-intensive weekly measurements of airborne inoculum using SSM plates. Plates cannot be used when it is raining or when high winds are present (Steadman et al., Citation1994) and require a pest management scout to place, retrieve and evaluate the plates throughout the season. There is also a lag time of three to five days from the time plates are collected before the number of CFU on plates can be counted. The optimum time for fungicide application may be missed. Thus, the use of SSM plates in a disease forecast model is feasible, but not practical.
The data presented here show that applying the risk algorithm can substitute for the use of SSM plates. Colony-forming units collected on SSM plates were correlated with SDSI values from the risk algorithm at eight of 10 plots using both Model 1 and Model 2. When data from both years were combined, Model 1 more accurately predicted CFU plate−1 than Model 2.
Testing of the risk algorithm relied on the use of environmental data from both a centralized location and from the field plots. Field-specific data for soil matric potential was not used in the risk algorithms in this study. It was recorded at the MCRS, which was within 4.7 km from commercial plots in both years. Ideally, all microclimatic data should be collected at individual fields. However, it may be possible to collect data from a centralized location if the soil type is similar and the fields are within a 5 km radius.
The use of two thresholds can help accommodate growers with conservative and liberal disease management strategies. Growers with a history of significant losses due to SRC may choose to apply fungicides when the SDSI reaches 33, while growers who are less concerned with SRC may wait until the SDSI reaches 45 using Model 1 of the risk algorithm. Where soil T data cannot be readily attained, Model 2 can be used with a moderate threshold when SDSI values are ≥11 and a high risk threshold when SDSI values ≥14. Spray regimes based on the forecast model were as effective as sprays timed on a calendar schedule in both years of this study. However, in 2004, none of the spray regimes were effective in reducing disease development. This may have been related to the lower rate of boscalid used in this year. Therefore, rates of boscalid were increased in 2005 and did provide disease control when applied according to Model 1.
There are a number of methods, other than the use of SSM plates, to detect airborne inoculum of S. sclerotiorum. Spore trapping methods, such as adhesive-coated glass microscope slides, traditionally rely on microscopic identification of fungal propagules. However, ascospores of S. sclerotiorum are nondescript and similar to spores of a number of other fungal species and, therefore, are difficult to identify based on morphological characteristics (McCartney et al., Citation1997; Freeman et al., Citation2002). Selective media plates can be used in vacuum samplers to determine the concentration of spores; however, vacuum sampler devices can be costly (McCartney et al., Citation1997). Forecast models for canola have incorporated the collection and culturing of petals to predict disease caused by S. sclerotiorum with relatively high success (Turkington, Citation1988). Petal tests determine the percentage of petals infected by ascospores of S. sclerotiorum and are similar to SSM plates in that both are designed to indicate the presence of airborne spores.
Effective methods for trapping and quantifying airborne ascospores of S. sclerotiorum and conidia of Botrytis squamosa Walker have recently been developed (Carisse et al., Citation2009; Rogers et al., Citation2009). In these studies, spores were trapped using wax-coated plastic tapes or rotating arm traps, and quantified using quantitative polymerase chain reaction (qPCR) methods. Relationships between spore numbers detected using traditional microscopic and qPCR methods indicated that results from the qPCR method were more sensitive or accurate, or both, for detecting inoculum of these pathogens and that the qPCR method may have potential use in inoculum-based forecasting models.
The number of apothecia has also been used as an indication of the prevalence of the pathogen. Despite rigorous scouting, relatively few apothecia were detected in all years of this study in any field plots. Apothecia were often numerous (>10) within a small area (<1 m2) and were not evenly distributed throughout the field plots that were scouted. The distribution of apothecia is likely clustered and not random throughout a carrot field. In bean and soybean, apothecia were spatially aggregated and could be described by a negative binomial distribution (Boland & Hall, 1988a, 1988b). Due to this clustered distribution, scouting can produce inaccurate estimates of total apothecia in a field. The relatively small size of apothecia can also make them difficult to detect, especially when fertilizer pellets that are relatively the same size and colour are present. In bean, the association between disease incidence and the frequency and distribution of apothecia was inconsistent (Schwartz & Steadman, Citation1978). Significant relationships between the presence of apothecia and disease incidence were reported for bean, soybean and rapeseed, but intensive scouting was required (Gugel & Morrall, Citation1986; Boland & Hall, 1988a, 1988b). Intensive scouting for apothecia is not feasible for IPM programmes for carrot.
Crop history may also be an important variable for predicting the development of SRC. A forecast model for rapeseed included a risk point table that incorporated the number of years since the last rapeseed crop and the disease incidence in the last host crop of S. sclerotiorum (Twengstrom et al., Citation1998). The SRC forecast model includes a variable for the history of disease caused by S. sclerotiorum in the expert system described below. Incorporating a more detailed analysis of disease history into the model for SRC may improve the model's ability to predict disease in fields where carrot is planted in long rotations. In Ontario, the majority of carrot crops are grown in relatively short rotation (e.g. two years), thus, rotation likely does not effectively reduce the number of sclerotia of S. sclerotiorum (McDonald & Vander Kooi, Citation1999; McDonald et al., Citation2001), and the current SRC model is adequate.
Forecasting SRC throughout the growing season is important because weekly disease incidence can fluctuate. Periods of reduced disease incidence occurred in both 2004 and 2005. Increased leaf abscission may have been a factor in the reduction of disease incidence. Leaf abscission was observed in blueberry plants that were severely infected by Septoria leaf spot, and was likely involved in reducing the incidence of disease symptoms (Ojiambo & Scherm, Citation2005). A similar mechanism of leaf abscission in response to S. sclerotiorum was observed in carrot (Foster et al., Citation2008).
To integrate the forecast models into a disease management programme, a preliminary expert system was developed (). The expert system would be activated when: (1) there is a history of SRC in the field, (2) after full closure of the canopy, (3) at least 70 to 80% of carrot plants had one to two collapsed senescing leaves and one to three healthy leaves lodged in the furrow, and (4) ascospores are present within the crop. The presence of ascospores is determined either from the microclimate suitability for apothecia or ascospore development using risk algorithms. The start of SRC would be predicted and an action recommendation would be prompted if all four conditions were met in a sequential fashion.
Fig. 3. Diagram of an expert system prototype based on field history, host and weather proposed to predict outbreaks of Sclerotinia rot of carrot and advise growers in making disease management decisions. This diagram depicts the sequential order of satisfying the critical thresholds of risk factors for disease initiation, and respective actions (spray or no spray) for each outcome (yes or no).
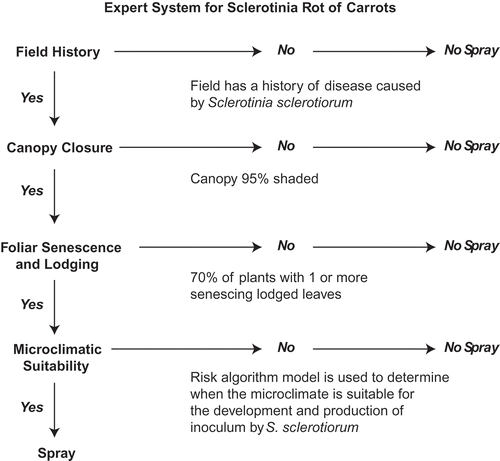
This model was made available to carrot growers on organic soil in Bradford Marsh, Ontario, starting in the 2006 growing season through the integrated pest management programme. Management options, other than the use of fungicides, are currently available to growers in Ontario. For instance, irrigation could be timed based on the forecast model. Trimming of carrot foliage (Kora et al., 2005b) is a cultural practice where the carrot canopy is trimmed to prevent canopy closure and hence the development of apothecia to reduce SRC. Trimming could also be timed according to the forecast model. Further field evaluation will be required to identify which model is more accurate, and to adapt the expert system to crops grown on mineral soils.
Acknowledgements
The authors wish to thank Kevin Vander Kooi and the staff at the Muck Crops Research Station for technical support. Financial support for this project was provided by the National Sciences and Engineering Research Council and the Sustainable Production Systems Program of the Ontario Ministry of Agriculture and Food and Rural Affairs and the University of Guelph Partnership.
References
- Anonymous . 2004 . Vegetable Production Recommendations. Publication 363. Ontario Ministry of Agriculture and Food , Toronto, ON : Queen's Printer for Ontario .
- Basf . 2004 . Lance WDG Fungicide. Approved Lance Crop Label , Toronto, ON : BASF Canada Inc .
- Boland , G.J. and Hall , R. 1988a . Numbers and distribution of apothecia of Sclerotinia sclerotiorum in relation to white mold of white bean (Phaseolus vulgaris) . Can. J. Bot. , 66 : 247 – 252 .
- Boland , G.J. and Hall , R. 1988b . The spatial distribution and number of apothecia of Sclerotinia sclerotiorum in relation to Sclerotinia stem rot of soybean . Plant Pathol. , 37 : 329 – 336 .
- Boland , G.J. and Hall , R. 1994 . Index of plant hosts of Sclerotinia sclerotiorum . Can. J. Plant Pathol. , 16 : 93 – 108 .
- Bom , M. and Boland , G.J. 2000 . Evaluation of disease forecasting variables for Sclerotinia stem rot (Sclerotinia sclerotiorum) of canola . Can. J. Plant Sci. , 80 : 889 – 898 .
- Carisse , O.D. , Tremblay , M. , Levesque , C.A. , Gindro , K. , Ward , P. and Houde , A. 2009 . Development of a blight TaqMan real-time PCR assay for quantification of airborne conidia of Brotrytis squamosa and management of botrytis leaf blight of onion . Phytopathology , 99 : 1273 – 1280 .
- Clarkson , J.P. , Phelps , K. , Whipps , J.M. , Young , C.S. , Smith , J.A. and Watling , M. 2004 . Forecasting Sclerotinia disease on lettuce: toward developing a prediction model for carpogenic germination of sclerotia . Phytopathology , 94 : 268 – 279 .
- Clarkson , J.P. , Phelps , K. , Whipps , J.M. , Young , C.S. , Smith , J.A. and Watling , M. 2007 . Forecasting Sclerotinia disease on lettuce: a predictive model for carpogenic germination of Sclerotinia sclerotiorum sclerotia . Phytopathology , 97 : 621 – 631 .
- Couper , G. 2001 . The biology and control of sclerotinia within carrot crops in north east Scotland , Aberdeen, UK : Ph.D. Thesis, University of Aberdeen .
- Foster , A.J. , Mcdonald , M.R. and Boland , G.J. 2008 . Disease progression of sclerotinia rot of carrot (Sclerotinia sclerotiorum) from shoot to root pre- and post-harvest . Can. J. Plant Pathol. , 30 : 206 – 213 .
- Freeman , J. , Ward , E. , Calderon , C. and Mccartney , A. 2002 . A polymerase chain reaction (PCR) assay for the detection of inoculum of Sclerotinia sclerotiorum . Eur. J. Plant Pathol. , 108 : 877 – 886 .
- Geary , J.R. 1978 . Host–parasite interactions between the cultivated carrot (Daucus carota) and Sclerotinia sclerotiorum (Lib.) de Bary , Norwich, UK : Ph.D. Thesis, University of East Anglia .
- Gillespie , T.J. and Kidd , G.E. 1978 . Sensing duration of leaf moisture retention using electrical impedance grids . Can. J. Plant Sci. , 58 : 179 – 187 .
- Gugel , R.K. and Morrall , R.A.A. 1986 . Inoculum–disease relationships in Sclerotinia stem rot of rapeseed in Saskatchewan . Can. J. Plant Pathol. , 8 : 89 – 96 .
- Hughes , G. , Mcroberts , N. and Burnett , F.J. 1999 . Decision-making and diagnosis in disease management . Plant Pathol. , 48 : 147 – 153 .
- Hunter , J.E. 1981 . Proposal for a forecasting system for white mold of snap bean . Rep. Bean Improve. Coop. , 24 : 122 – 123 .
- Hunter , J.E. , Pearson , R.C. , Seem , R.C. , Smith , C.A. and Palumbo , D.R. 1984 . Relationship between soil moisture and occurrence of Sclerotinia sclerotiorum and white mold disease on snap beans . Prot. Ecol. , 7 : 269 – 280 .
- Koch , S. , Dunker , S. , Kleinhenz , B. , RöHrig , M. and Von Tiedemann , A. 2007 . A crop loss-related forecasting model for Sclerotinia stem rot in winter oilseed rape . Phytopathology , 97 : 1186 – 1194 .
- Kora , C. 2003 . Etiology, Epidemiology and Management of Sclerotinia Rot of Carrot Caused by Sclerotinia sclerotiorum (Lib.) de Bary , Ontario, , Canada : Ph.D. Thesis, University of Guelph, Guelph .
- Kora , C. , Mcdonald , M.R. and Boland , G.J. 2003 . Sclerotinia rot of carrot: a unique case of phenological adaptation and bicyclic development of Sclerotinia sclerotiorum . Plant Dis. , 87 : 456 – 470 .
- Kora , C. , Mcdonald , M.R. and Boland , G.J. 2005a . Epidemiology of sclerotinia rot of carrot caused by Sclerotinia sclerotiorum . Can. J. Plant Pathol. , 27 : 245 – 258 .
- Kora , C. , Mcdonald , M.R. and Boland , G.J. 2005b . Lateral clipping of canopy influences the microclimate and development of apothecia of Sclerotinia sclerotiorum in carrots . Plant Dis. , 89 : 549 – 557 .
- Langston , D.B. , Jr , Phipps , P.M. and Stipes , R.J. 2002 . An algorithm for predicting outbreaks of Sclerotinia blight of peanut and improving the timing of fungicide sprays . Plant Dis. , 86 : 118 – 126 .
- Madden , L.V. and Ellis , M.A. 1988 . “ How to develop plant disease forecasters ” . In Experimental techniques in plant disease epidemiology , Edited by: Kranz , J. and Rotem , J. 191 – 208 . New York : Springer-Verlag .
- Mccartney , H.A. , Fitt , B.D.L. and Schmechel , D. 1997 . Sampling bioaerosols in plant pathology . J. Aerosol Sci. , 28 : 349 – 364 .
- Mcdonald , M.R. and Boland , G.J. 2004 . Forecasting diseases of Sclerotina spp. in eastern Canada: fact or fiction? . Can. J. Plant Pathol , 26 : 480 – 488 .
- Mcdonald, M.R., Traquair, J., Boland, G.J., & Ripley, B. (2001). Improved quality and storability of onions and carrots through sustainable management of soil-borne diseases. Ontario Research Enhancement Program, Report: OREP-1999/25. Agriculture and Agri-Food Canada http://res2.agr.gc.ca/London/orep/ (http://res2.agr.gc.ca/London/orep/)
- Mcdonald , M.R. and Vander Kooi , K. 1999 . “ Evaluation of various field and post-harvest fungicide and calcium treatments for the control of Sclerotinia on carrots in storage, 1998–1999 ” . In In Muck vegetable cultivar trial and research report No. 49, 1999 , 23 – 24 . Kettleby, ON : Department of Plant Agriculture, University of Guelph .
- Mclaren , D.L. , Conner , R.L. , Platford , R.G. , Lamb , J.L. , Lamety , H.A. and Kutcher , H.R . 2004 . Predicting diseases caused by Sclerotinia sclerotiorum on canola and bean – a Western Canadian perspective . Can. J. Plant Pathol. , 26 : 489 – 497 .
- Ojiambo , P.S. and Scherm , H. 2005 . Survival analysis of time to abscission of blueberry leaves affected by Septoria leaf spot . Phytopathology , 95 : 108 – 113 .
- Richards , L.A. 1965 . “ Physical condition of water in soil ” . In Methods of Soil Analysis , Edited by: Black , C.A. 128 – 129 . Madison, WI : American Society of Agronomy .
- Rogers , S.L. , Atkins , S.D. and West , J.S. 2009 . Detection and quantification of airborne inoculum of Sclerotinia sclerotiorum using quantitative PCR . Plant Pathol. , 58 : 324 – 331 .
- SAS Institute Inc . 1999 . SAS/STAT User's Guide, Release 7 and 8 , Cary, NC : SAS Institute, Inc .
- Steadman , J.R. 1979 . Control of plant diseases caused by Sclerotinia species . Phytopathology , 69 : 904 – 907 .
- Steadman , J.R. , Marinkowska , J. and Rutledge , S. 1994 . A semi-selective medium for the isolation of Sclerotinia sclerotiorum . Can. J. Plant Pathol. , 16 : 68 – 70 .
- Steadman , J.R. and O'Keefe , D. 1998 . “ The blue plate test: a simple method to indicate the potential for white bean mold in dry beans and soybeans ” . In In Proceedings of the 10th International Sclerotinia Workshop , 9 – 12 . Fargo, ND : North Dakota State University, Department of Plant Pathology .
- Sun , B. and Yang , X.B. 2000 . Light, temperature, and moisture effects on apothecium production of Sclerotinia sclerotiorum . Plant Dis. , 84 : 1287 – 1293 .
- Schwartz , H.F. and Steadman , J.R. 1978 . Factors affecting sclerotia populations of, and apothecium production by, Sclerotinia sclerotiorum . Phytopathology , 68 : 383 – 388 .
- Thomas , P. 1984 . Sclerotinia stem rot checklist . In: Canola Growers Manual (pp. 1053–1055). Canola Council of Canada, Winnipeg, Manitoba ,
- Turkington , T.K. 1988 . Using ascospore infestation of petals to forecast Sclerotinia stem rot of rapeseed , Saskatchewan, , Canada : M.Sc. Thesis, University of Saskatchewan, Saskatoon .
- Turkington , T.K. and Morrall , R.A.A. 1993 . Use of petal infestation to forecast Sclerotinia stem rot of canola: the influence of inoculum variation over the flowering period and canopy density . Phytopathology , 83 : 682 – 689 .
- Turkington , T.K. , Morall , R.A.A. and Gugel , R.K. 1991 . Use of petal infestation to forecast stem rot of canola: evaluation of early bloom sampling, 1985–1990 . Can. J. Plant Pathol. , 13 : 50 – 59 .
- Twengstrom , E. , Sigvald , R. , Svensson , C. and Yuen , J. 1998 . Forecasting Sclerotinia stem rot in spring sown oilseed rape . Crop Prot. , 17 : 405 – 411 .
- Yuen , J. , Twengström , E. and Sigvald , R. 1996 . Calibration and verification of risk algorithms using logistic regression . Eur. J. Plant Pathol. , 102 : 847 – 854 .
- Zadoks , J.C. and Schein , R.D. 1979 . Epidemiology and plant disease management , New York : Oxford University Press .