Abstract
The in-depth study of plant metabolic networks and their changes in response to biotic stimuli is an emerging field of metabolomics which has a great potential to provide new insights into plant physiology, plant–pathogen interactions, crop protection and breeding. In spite of the development of metabolomics in numerous applications, its application to the study of plant–pathogen interactions is still in its infancy. Here we describe an integrated methodology for the study of plant–pathogen interactions, ranging from experimental design and execution, to analyses of samples and data, and their biological interpretation. As a study case, the investigation of interactions in the pathosystem potato (Solanum tuberosum L.) and Rhizoctonia solani using metabolomics is presented. Chemical analyses were performed by integrating Fourier transform-ion cyclotron resonance/mass spectrometry (FT-ICR/MS) with gas chromatography/mass spectrometry (GC/MS). The use of two analytical platforms enabled the study of alterations in the content of infected plants for a large number of metabolites belonging to chemical groups with diverse physicochemical properties, such as amino acids, fatty acids, and carboxylic acids, as well as carbohydrates, and terpenoid and steroidal glycoalkaloids. The biological interpretation of data was performed using the powerful software Cytoscape whose role in metabolomics will be highlighted, and BioCyc databases. The principle behind such an approach is to reveal changes in the global metabolic network of the plant in response to pathogen invasion and subsequently to correlate those changes to gene expression.
Résumé
L'étude approfondie des réseaux métaboliques des plantes et de leurs changements en réponse aux stimuli biotiques est un domaine émergent de la métabolomique qui a le potentiel d'ouvrir de nouvelles perspectives dans les domaines de la physiologie végétale, des interactions plante-agent pathogène, de la protection des cultures et de la sélection. Malgré l'avancement de la métabolomique dans de nombreuses applications, son application dans le domaine des interactions plante-agent pathogène en est encore à ses balbutiements. Nous décrivons dans cet article une méthodologie intégrée s'appliquant à l'étude des interactions plante-agent pathogène variant du concept expérimental et de l'exécution à l'analyse des échantillons et des données ainsi que de leur interprétation biologique. Comme étude de cas, nous présentons l'investigation des interactions dans le pathosystème pomme de terre (Solanum tuberosum L.) et Rhizoctonia solani sous l'angle de la métabolomique. Des analyses chimiques ont été faites en intégrant le spectromètre de masse à résonnance cyclotonique ionique à haut champ et transformée de Fourier (FT-ICR/MS) au couplage entre la chromatographie en phase gazeuse et la spectrométrie de masse (CG/SM). L'utilisation des deux plates-formes analytiques a permis l'étude des changements dans le contenu des plantes infectées, et ce, pour un grand nombre de métabolites appartenant à des groupes chimiques possédant différentes propriétés physicochimiques comme les acides aminés, les acides gras, les acides carboxyliques de même que les hydrates de carbone, les terpénoïdes et les glycoalkaloïde stéroïdiens. L'interprétation biologique des données a été effectuée à l'aide du puissant logiciel Cytoscape, dont le rôle en métabolomique sera mis en évidence, et des bases de données BioCyc. La raison principale qui sous-tend une telle approche est de révéler les changements qui se produisent dans le réseau métabolique global de la plante en réaction à l'invasion d'un agent pathogène et, ultérieurement, de corréler ces changements à l'expression génique.
Introduction
Plants are composed of a vast number of primary and secondary metabolites (Dixon, Citation2001; Berger et al., Citation2007) with diverse physicochemical properties in a wide range of concentrations, which make the comprehensive analysis of their metabolomes a very challenging task (Hegeman, Citation2010). To date, approximately 10% of the secondary metabolites have been identified, which is indicative that our knowledge on plant metabolism and its regulation is still largely fragmented. The robust and comprehensive monitoring of plant metabolomes facilitates the in-depth understanding of a plant's physiology which is a prerequisite for the development of applications in plant breeding, biotechnology, and crop protection.
Metabolomics is an integrated tool of systems biology which enables the qualitative and quantitative profiling of metabolites of a biological system serving as a linkage between genotypes and phenotypes (Fiehn et al., Citation2000). Its development has provided a new dimension for the investigation of biological systems (Kell, Citation2004; Schauer & Fernie, Citation2006; Dunn, Citation2008; Hall et al., Citation2008; Bundy et al., Citation2009; Kaddurah-Daouk & Krishnan, Citation2009; Vinayavekhin et al., Citation2010; Aliferis & Jabaji, Citation2011; Simpson et al., Citation2011). Additionally, the development of powerful analytical platforms has greatly expanded our analytical capabilities.
Here we present principles for the application of metabolomics for the dissection of interactions in plant–pathogen pathosystems using the potato (Solanum tuberosum L.)-Rhizoctonia solani Kühn anastomosis group 3 (AG3) as a model. The process of metabolomics for the study of plant–pathogen interaction is displayed in . Metabolomics analysis consists of three distinct experimental phases. The first phase is sample preparation, the second is chemical analyses and the final step is data mining and integration using chemometrics. All steps are tightly interrelated, but particularly the first two, since the various analytical methods require certain properties of the analyte and thus determine the way the sample should be prepared.
Fig. 1. Representative steps for the study of plant–pathogen interactions applying metabolomics. FT-ICR/MS, Fourier transform-ion cyclotron resonance-mass spectrometry; GC, gas chromatography, HCA, hierarchical cluster analysis; LC, liquid chromatography; MS, mass spectrometry; Orthogonal PLS-DA, orthogonal partial least squares-discriminant analysis; PCA, principal components analysis.
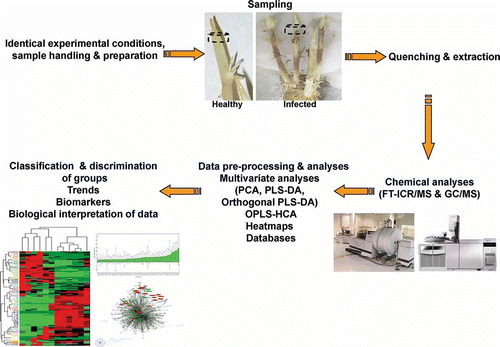
Experimental conditions and sample handling for metabolomics
Due to the rapid turnover of intracellular metabolites and since the composition of plant metabolomes is influenced by environmental conditions and stress factors (Guy et al., Citation2008; Shulaev et al., Citation2008), it is important to have reliable and reproducible protocols for sampling and sample treatment. Focusing on the study of plant–pathogen interactions, precise sampling of target regions is essential. The metabolite composition varies not only from organ to organ but even within the same organ. Additionally, accurate sampling is required in order to avoid inclusion of plant parts that have deteriorated as result of the pathogen invasion and could make the interpretation of the results problematic. Precise sampling also reduces the variability between biological replications which is important for the detection of reliable biomarkers applying metabolomics.
Quenching of metabolism and extraction of samples
To capture the changes that occur in the potato's metabolomes in response to R. solani invasion, samples should be subjected to robust global metabolite profiling. Immediate quenching of the cellular metabolism after sampling is essential in order to avoid undesirable changes in the metabolomes that could greatly affect the validity of the biological interpretation of the data. Samples are directly placed at −80 °C until further processing. Freeze-drying is the most common and suitable method to dry plant material prior to extraction.
In general, metabolomics studies should be designed to detect as many metabolites as possible in plant samples; thus the extraction protocol is crucial as it determines the quantitative and qualitative composition of the recorded metabolic profiles (Lin et al., Citation2007; Wu et al., Citation2008). Efficient protocols enable the extraction of the metabolites of interest and their subsequent analyses and identification. Organic solvents that can extract diverse groups of metabolites as well as mixtures of organic solvents with water are commonly used in metabolomics extraction protocols. In the present study, quenching of the cell metabolism was performed by liquid N2 and the extraction of the samples using a mixture of methanol:ethyl acetate (50:50, v/v).
Instrumentation
The choice of the analytical instrument is essential for metabolomics since the applied analytical protocols and our capabilities in monitoring metabolomes depend on the employed analyzer. The selection of the analyzer depends on many factors such as the available instrumentation, the expertise, the nature and composition of the analyzed samples, and the goals of the research. Unfortunately, it seems that there is not yet a universal extraction protocol suited for all kinds of biological questions and for the different metabolomics protocols. However, for the different analytical methods, there seems to be a trend towards certain protocols being widely accepted for metabolomics (Fiehn et al., Citation2007). Generally, nuclear magnetic resonance spectroscopy (NMR) and mass spectrometry (MS) analyzers are the most commonly employed in metabolomics (Dettmer et al., Citation2007; Dunn, Citation2008; Lindon & Nicholson, Citation2008; Wishart, Citation2008; Allwood et al., Citation2009; Aliferis & Chrysayi-Tokousbalides, Citation2011; Simpson et al., Citation2011). Widely used methods have been formalized into protocols, for GC/MS based metabolomics (Lisec et al., Citation2006), and NMR based metabolomics (Kim et al., Citation2011).
Here, chemical analyses were performed integrating Fourier transform-ion cyclotron resonance/mass spectrometry (FT-ICR/MS) with gas chromatography/mass spectrometry (GC/MS). Such approach facilitated the detection in infected plant tissues of a large number of primary and secondary metabolites belonging to amino, fatty and carboxylic acids, carbohydrates, and terpenoid and steroidal glycoalkaloids which compose a large portion of the potato sprout's metabolomes.
Data analyses and visualization
A large number of freely available software and databases for the analyses and visualization of metabolomics data have been developed and have been recently reviewed (Aliferis & Chrysayi-Tokousbalides, Citation2011).
The sequential steps of metabolomics data analyses include the pre-processing, statistical analyses and biological interpretation of results (). The pre-processing of data (i.e. spectra alignment and baseline correction) follows the construction of data matrices which will be subjected to statistical analyses for the detection of trends and biomarkers. Metabolomics data are multivariate, thus multivariate analyses (MVA), such as principal components analysis (PCA) and partial least squares-discriminant analysis (PLS-DA), are the most common employed statistical analyses for metabolomics. In addition to MVA, hierarchical cluster analysis (HCA) and heatmaps provide a powerful visualization of large datasets. In our research, MVA was performed using the software Simca P+ 12 (Umetrics, MKS Instruments Inc., Sweden) and HCA and heatmaps were created using the software MATLAB (Mathworks Inc., Natick, MA, USA). Such analyses enabled the detection of differences between the metabolomes of control and infected plant tissues, with the most profound differences to be detected in their content in various alkaloids including de novo synthesized potato phytoalexins.
Plant metabolism is a dynamic system and changes in the levels of any metabolite are expected to affect that of others. The listing of metabolic differences between infected and non-infected plants is inadequate to provide a holistic picture of the changes that take place in the global metabolic network of the plant. Therefore, it is crucial in metabolomics studies to get the whole picture of all possible biosynthetic pathways. The visualization of a potato sprout metabolome was achieved using the software Cytoscape (Shannon et al., Citation2003) and the curated potatoCyc metabolic library (). The software offers search and visualization tools for genes, enzymes and metabolic networks. Such an approach enabled the robust visualization of changes in more than 300 identified potato sprout metabolites in response to pathogen invasion and revealed relationships between metabolome and proteome.
Fig. 2. Partial view of the potato sprout metabolome (a) and selected sub-networks of amino acids and glycoalkaloids (b, c). For the construction of the metabolic network the software Cytoscape v2.6.3 and the curated potatoCyc database were used. Nodes (highlighted in shaded areas) represent metabolites, enzymes or chemical reactions. For metabolites, the size of the nodes is proportional to their relative content in the metabolic profiles.
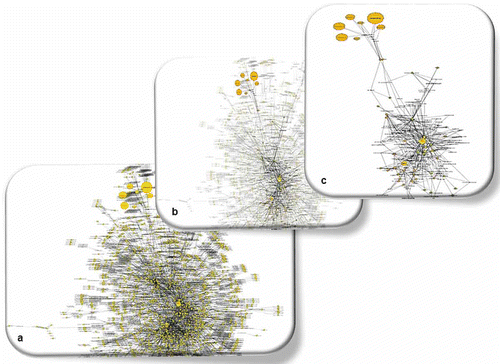
Conclusions
In the last decade, plant metabolomics provided critical insights into molecular and biochemical events that occur in only a handful of examples pertaining to mutualistic and pathogenic plant–microbe interactions. Fluctuations of metabolites of potato caused by fungal infection have been largely unexploited with most of the previous studies limited to their partial and fragmented monitoring. We embarked on this study to examine changes in potato sprout metabolites using a combination of more than one analytical platform with robust chemometric analyses. A network of possible connected metabolic pathways was built which allowed us to monitor the perturbations of potato sprout metabolites in response to the pathogen. One of the challenges that metabolomics studies of plant–microbe interactions is faced with is the presence of fungal-derived metabolites that could make the interpretation of results difficult. The use of fungal metabolite profiles is useful in order to identify fungal-derived metabolites that could lead to misinterpretation of results. The construction of comprehensive metabolite databases for fungal pathogens is expected to help with overcoming this obstacle.
In conclusion, the understanding of plant defence mechanisms through high-throughput metabolomics could lead to the discovery of biomarkers that could be exploited in biomarker-driven plant breeding, and applications in biotechnology and/or crop protection.
Acknowledgements
The work has been funded by the Natural Sciences and Engineering Research Council of Canada (NSERC).
Notes
This paper was a contribution to the symposium entitled ‘Contributions of genomics to plant pathology’ held during the Canadian Phytopathological Society Annual Meeting in Vancouver, British Columbia, June 2010.
References
- Aliferis , K. and Chrysayi-Tokousbalides , M. 2011 . Metabolomics in pesticide research and development: review and future perspectives . Metabolomics , 7 : 35 – 53 .
- Aliferis , K.A. and Jabaji , S. 2011 . Metabolomics – a robust bioanalytical approach for the discovery of the modes-of-action of pesticides: a review . Pest. Biochem. Physiol. , 100 : 105 – 117 .
- Allwood , J.W. , Erban , A. de Koning , S. 2009 . Inter-laboratory reproducibility of fast gas chromatography-electron impact-time of flight mass spectrometry (GC-EI-TOF/MS) based plant metabolomics . Metabolomics , 5 : 479 – 496 .
- Berger , S. , Sinha , A.K. and Roitsch , T. 2007 . Plant physiology meets phytopathology: plant primary metabolism and plant–pathogen interactions . J. Exp. Bot. , 58 : 4019 – 4026 .
- Bundy , J.G. , Davey , M.P. and Viant , M.R. 2009 . Environmental metabolomics: a critical review and future perspectives . Metabolomics , 5 : 3 – 21 .
- Dettmer , K. , Aronov , P.A. and Hammock , B.D. 2007 . Mass spectrometry-based metabolomics . Mass Spectrom. Rev. , 26 : 51 – 78 .
- Dixon , R.A. 2001 . Natural products and plant disease resistance . Nature , 411 : 843 – 847 .
- Dunn , W.B. 2008 . Current trends and future requirements for the mass spectrometric investigation of microbial, mammalian and plant metabolomes . Physiol. Biol. , 5 : 1 – 24 .
- Fiehn , O. , Kopka , J. , Dormann , P. , Altmann , T. , Trethewey , R.N. and Willmitzer , L. 2000 . Metabolite profiling for plant functional genomics . Nat. Biotech. , 18 : 1157 – 1161 .
- Fiehn , O. , Robertson , D. , Griffin , J. , van der Werf , M. , Nikolau , B. Morrison , N. 2007 . The metabolomics standards initiative (MSI) . Metabolomics , 3 : 175 – 178 .
- Guy , C. , Kaplan , F. , Kopka , J. , Selbig , J. and Hincha , D.K. 2008 . Metabolomics of temperature stress . Physiol. Plant. , 132 : 220 – 235 .
- Hall , R.D. , Brouwer , I.D. and Fitzgerald , M.A. 2008 . Plant metabolomics and its potential application for human nutrition . Physiol. Plant. , 132 : 162 – 175 .
- Hegeman , A.D. 2010 . Plant metabolomics – meeting the analytical challenges of comprehensive metabolite analysis . Brief. Funct. Genomics , 9 : 139 – 148 .
- Kaddurah-Daouk , R. and Krishnan , K.R.R. 2009 . Metabolomics: a global biochemical approach to the study of central nervous system diseases . Neuropsychopharmacology Rev. , 34 : 173 – 186 .
- Kell , D.B. 2004 . Metabolomics and systems biology: making sense of the soup . Curr. Opin. Microbiol. , 7 : 296 – 307 .
- Kim , H.K. , Choi , Y.H. and Verpoorte , R. 2011 . NMR-based plant metabolomics: where do we stand, where do we go? . Trends Biotech. , 29 : 267 – 275 .
- Lin , C. , Wu , H. , Tjeerdema , R. and Viant , M. 2007 . Evaluation of metabolite extraction strategies from tissue samples using NMR metabolomics . Metabolomics , 3 : 55 – 67 .
- Lindon , J.C. and Nicholson , J.K. 2008 . Spectroscopic and statistical techniques for information recovery in metabonomics and metabolomics . Annu. Rev. Analyt. Chem. , 1 : 45 – 69 .
- Lisec , J. , Schauer , N. , Kopka , J. , Willmitzer , L. and Fernie , A.R. 2006 . Gas chromatography mass spectrometry based metabolite profiling in plants . Nat. Protoc. , 1 : 387 – 396 .
- Schauer , N. and Fernie , A.R. 2006 . Plant metabolomics: towards biological function and mechanism . Trends Plant Sci. , 11 : 508 – 516 .
- Shannon , P. , Markiel , A. Ozier , O. 2003 . Cytoscape: a software environment for integrated models of biomolecular interaction networks . Genome Res. , 13 : 2498 – 2504 .
- Shulaev , V. , Cortes , D. , Miller , G. and Mittler , R. 2008 . Metabolomics for plant stress response . Physiol. Plant. , 132 : 199 – 208 .
- Simpson , A.J. , McNally , D.J. and Simpson , M.J. 2011 . NMR spectroscopy in environmental research: from molecular interactions to global processes . Prog. Nucl. Mag. Res. Spec. , 58 : 97 – 175 .
- Vinayavekhin , N. , Homan , E.A. and Saghatelian , A. 2010 . Exploring disease through metabolomics . ACS Chem. Biol. , 5 : 91 – 103 .
- Wishart , D.S. 2008 . Quantitative metabolomics using NMR . Trends Analyt. Chem. , 27 : 228 – 237 .
- Wu , H. , Southam , A.D. , Hines , A. and Viant , M.R. 2008 . High-throughput tissue extraction protocol for NMR- and MS-based metabolomics . Analyt. Biochem. , 372 : 204 – 212 .