Abstract
The prevalence of tomato diseases and local smallholder farmer knowledge was assessed in five villages in the Morogoro Region of Tanzania during the rainy and dry production seasons. The most commonly occurring foliar diseases in both seasons were early blight (88 of 100 fields), bacterial spot/speck (49), viral diseases (42) and Septoria leaf spot (34). Bacterial spot/speck, tomato yellow leaf curl virus, other viral diseases, and late blight were present in significantly higher numbers of fields during the rainy season than the dry season, while significantly more plants per field were affected by early blight in the rainy season than the dry season. A root health assay was conducted to assess root knot nematode damage and root rot severity, and root knot nematodes were found in 44 of 50 fields surveyed. Farmers used local names for plant diseases, which tended to be associated with the symptomatology of the disease, concepts borrowed from other aspects of life, perceived causal agent or weather conditions. Identification of local names improved communication between farmers and researchers and elucidated how farmers perceived key diseases in the region. Extension materials were developed to improve farmers’ identification and management of key tomato diseases in the region. Farmers can better allocate limited resources to manage key diseases through an improved understanding of prevalent diseases and local plant disease knowledge. This study serves as an example of how plant pathologists can develop a baseline understanding of key regional plant disease constraints through the integration of field surveys and ethnophytopathological studies.
Résumé
La fréquence des maladies de la tomate et les connaissances des petits fermiers locaux ont été évaluées dans cinq villages de la région de Morogoro, en Tanzanie, durant les saisons pluvieuse et sèche. Durant les deux saisons, les maladies foliaires les plus fréquentes étaient l’alternariose (88 champs sur 100), la tache/moucheture bactérienne (49), les maladies virales (42) et la tache septorienne (34). Durant la saison pluvieuse, la tache/moucheture bactérienne, le virus de la frisolée jaune de la tomate, d’autres maladies virales et le mildiou étaient répertoriés dans un nombre considérablement plus élevé de champs que durant la saison sèche. En outre, un nombre considérablement plus élevé de plants par champ était touché par le mildiou durant la saison pluvieuse, comparativement à la saison sèche. Un biotest sur la santé des racines a été effectué pour évaluer le dommage causé par les nématodes cécidogènes et la gravité du pourridié: on a trouvé des nématodes cécidogènes dans 44 des 50 champs inspectés. Les fermiers donnaient des noms familiers aux maladies des plantes, noms qui tendaient à être associés à la symptomatologie de la maladie, à des concepts empruntés à d’autres aspects de la vie, à des agents causaux apparents ou aux conditions climatiques. La définition de ces noms a contribué à améliorer la communication entre les fermiers et les chercheurs et a permis de comprendre comment les fermiers percevaient les principales maladies dans la région. Des documents de vulgarisation ont été produits pour permettre à ceux-ci d’identifier et de gérer plus facilement les principales maladies de la tomate. Les fermiers locaux sont les mieux placés pour répartir des ressources limitées afin de gérer les principales maladies grâce à une meilleure compréhension des plus courantes et à la connaissance des maladies des plantes locales. Cette étude est un exemple de la façon par laquelle les phytopathologistes peuvent développer une compréhension des conditions de base des contraintes liées aux principales maladies régionales des plantes, et ce, grâce à l’intégration des enquêtes sur le terrain et des études ethnophytopathologiques.
Introduction
Tomato (Solanum lycopersicum L.) is an important vegetable crop globally and is a key economic crop for smallholder farmers in Tanzania. Fresh vegetables rank as one of the top 10 Tanzanian agricultural commodities in terms of both production quantity and value (UNFAO, Citation2012). Tomato, along with cabbage and onion, is one of the most commonly grown fresh vegetable crops in Tanzania (Luzi-Kihupi et al., Citation2012). Tomatoes are grown for local consumption and domestic markets, with only a small portion destined for export. In 2012, Tanzanian farmers produced 390 000 metric tons of tomatoes on 32 000 hectares (UNFAO, Citation2012).
The Morogoro Region is the third largest geographic region in Tanzania. It is a major agricultural production area (Paavola, Citation2008) and part of the so-called ‘breadbasket’ of the country (de Cleene Citation2014). Rainfall patterns in Morogoro are bimodal, with the heaviest rains occurring from March to May and lighter rains from October to December. Mean annual precipitation is highly variable, from 600–4000 mm per year, and climate change patterns may be shifting rainfall to more of a unimodal pattern (Paavola, Citation2008). Tomatoes are produced during both the rainy and dry seasons in Morogoro, but production is threatened by climate change (Paavola, Citation2008). Other key constraints to tomato production include diseases, insect pests, lack of access to improved germplasm, and poor soils (Minja et al., Citation2011).
Diseases are a key constraint to vegetable production in tropical countries and tomato crops are affected by a wide range of pathogens in Tanzania. Reported bacterial diseases include bacterial wilt (Ralstonia solanacearum (Black et al., Citation1999)), bacterial canker (Clavibacter michiganensis subsp. michiganensis (Black et al., Citation1999)), bacterial speck (Pseudomonas syringae pv. tomato (Shenge & Mabagala, Citation2007)), and bacterial spot (Xanthomonas euvesicatoria, X. vesicatoria, X. arboricola (not reported elsewhere on tomato), X. perforans and a fifth undetermined Xanthomonas species (Shenge et al., Citation2010; Mbega et al., Citation2012)). Fungal and oomycete diseases include early blight (Alternaria spp. (Maerere et al., Citation2010)), Septoria leaf spot (Septoria lycopersici (Maerere et al., Citation2010)) and late blight (Phytophthora infestans (Ojiewo et al., Citation2011)). Root knot nematodes, predominately Meloidogyne javanica and M. incognita, also constrain tomato production across the country (Nono-Womdim et al., Citation2002). One of the most commonly reported viral diseases of tomato in Eastern Africa is Tomato yellow leaf curl virus (TYLCV) (Chiang et al., Citation1997; Czosnek & Laterrot, Citation1997). Determining the most prevalent tomato diseases and identifying those that cause the greatest losses to smallholder farmers in the Morogoro Region allows for prioritization of farmers’ limited disease management resources.
Current disease and pest management strategies in the Morogoro Region and nearby regions rely heavily on the use of fungicides and insecticides (Maerere et al., Citation2006, Citation2010; Ngowi et al., Citation2007; Lekie et al., Citation2014). These pesticides are often sprayed too frequently, used for non-target pests (such as using an insecticide for a fungal disease) and applied without personal protective equipment. Results from a survey of pesticide usage in northern Tanzania (Ngowi et al., Citation2007) indicated that farmers used improvised tank mixes and pesticides for non-target pests and experienced symptoms of pesticide poisoning after application. Farmer education, particularly in the area of integrated pest management (IPM), is needed to reduce misuse of pesticides. In IPM, a combination of strategies is emphasized, with chemical pesticides used when warranted and often to complement cultural, biological and physical pest control measures. Successful IPM programmes hinge on farmers’ ability to correctly identify pests and diseases; yet researchers often overlook farmers’ local disease knowledge in this regard.
Local farmer knowledge needs to be taken into consideration when developing disease management strategies. Ethnophytopathology is the study of local plant disease knowledge (Bentley et al., Citation2009). It is necessary for plant health practitioners to understand how farmers identify, name and manage plant diseases in order to communicate efficiently with them, identify misconceptions and knowledge gaps, and suggest appropriate disease management practices (Thurston, Citation1990; Bentley et al., Citation2009). The findings of key, early studies of local plant disease knowledge indicate that farmers often attribute disease symptoms to environmental conditions, such as precipitation, sun and temperature, and these attributions to environmental conditions impact how farmers name and describe these diseases (Bentley, Citation1989; Trutmann et al., Citation1996). Local management practices, such as the spacing and timing of plantings, can develop based on these perceptions of environmental causes of diseases, and in turn, farmers can impact disease incidence without direct knowledge of the causal agents of diseases (Trutmann et al., Citation1996). As farmers learn about plant diseases through development programmes or extension agents, disease naming systems used by farmers (Bentley et al., Citation2009) and disease management strategies can reflect the influence of these educational programmes. Ethnophytopathological studies usually focus on one agricultural region on either a single crop (Trutmann et al., Citation1996; Mele et al., Citation2001; Nyeko et al., Citation2002; Segura et al., Citation2004; Poubom et al., Citation2005; Manu-Aduening et al., Citation2007; Adam et al., Citation2015) or a single disease (Nagaraju et al., Citation2002; Nathaniels et al., Citation2003; Nyankanga et al., Citation2004). Multi-regional (Sileshi et al., Citation2007; Bentley et al., Citation2009) and multi-crop studies (Kiros-Meles & Abang, Citation2008; Obopile et al., Citation2008) are less commonly performed. Ethnophytopathological studies are used to identify farmers’ names for diseases (Bentley et al., Citation2009), disease prioritization (Poubom et al., Citation2005), perceived disease aetiology (Trutmann et al., Citation1996), perceived losses from diseases (Kiros-Meles & Abang, Citation2008) and local management practices (Adam et al., Citation2015). There are currently no reports of local disease knowledge in tomato production in the Morogoro Region of Tanzania.
We sought to improve the ability of smallholder tomato farmers in the Morogoro Region of Tanzania to monitor and manage the health of their tomato crops. We first needed to identify and prioritize the occurrence of tomato diseases in the region in order to offer management recommendations to help reduce farmers’ reliance on chemical pesticides. The objectives of this research were to (i) identify the prevalent tomato diseases of the Morogoro Region; (ii) develop a root health bioassay to determine soilborne disease incidence and severity; and (iii) gather information on local tomato disease knowledge.
Materials and methods
Research sites
Surveys were conducted during the dry and rainy seasons in five villages in the Morogoro Region of Tanzania (): Msufini (6°17ʹ29.16′′S, 37°28ʹ19.92′′E), Mabana (6°28ʹ17.94′′S, 37°25ʹ34.62′′E), Msongozi (7°4ʹ10.20′′S, 37°20ʹ39.12′′E), Mlali (6°57ʹ39.60′′S, 37°32ʹ11.64′′E) and Kibagala (7°6ʹ12.78′′S, 37°35ʹ9.96′′E). These villages were selected following consultation with local agricultural officials. The baseline and dry season disease surveys were conducted in August–September 2013 and the rainy season disease survey in May–June 2014. Aggregate field characteristics (varieties grown, years of tomato cropping, tomato growth stage evaluated and rotational crops) are summarized in .
Table 1. Characteristics of tomato production in 100 fields (50 per season) included in the dry and rainy season disease surveys in the Morogoro Region of Tanzania, 2013–2014. Data were obtained by informal interviews of farmers managing each surveyed field.
Baseline survey and local plant disease knowledge
Twenty farmers in each village completed a written baseline survey individually with a trained enumerator to gather demographic data and identify tomato production practices, including those targeting disease management, disease occurrence and pesticide usage. Baseline survey data were summarized using the SPSS Statistics package (IBM Corporation, Armonk, New York). These methods were reviewed and determined to be exempt by The Ohio State University Institutional Review Board under protocol numbers 2013E0502 and 2014E0219.
To determine local plant disease knowledge, activities were conducted in each village to collect local names for tomato diseases and identify farmers’ knowledge of key diseases. Focus groups of farmers (6–12 individuals) were shown photographic images (Trutmann et al., Citation1996) of 18 tomato diseases, one disorder and one arthropod pest (). Local disease names were given in Kiswahili (or a local mother tongue) and translated into English for analysis. Farmers also provided descriptions of the symptoms. Disease names were categorized based on the farmers’ naming system (Bentley et al., Citation2009), which included names based on symptomatology, causal agents, words borrowed from other aspects of life (i.e. a human disease or household items) or weather. Farmers were also asked to identify five diseases they felt were most common in the dry production season and five in the rainy production season using the same set of 20 cards.
Table 2. Farmer descriptions of tomato diseases in the Morogoro Region of Tanzania. Farmers described tomato diseases and provided a local name for each one. Farmers’ local names for diseases were based on the perceived causal agent, symptoms of the disease, words borrowed from other aspects of life, or the weather. The number of villages that used each naming classification is shown in parentheses.
Tomato disease survey
Ten tomato fields were selected randomly in each village, which were distributed widely across each village. Within each field, three randomly selected 2-m2 quadrats were surveyed for diseases, and incidence and severity were estimated. Foliar disease severity was rated on a 1–4 scale: 1: no disease, 2: >0–25% disease (low severity), 3: >25–50% disease (moderate severity) and 4: >50% disease (high severity) (Maerere et al., Citation2010). Wilt diseases were rated on a 0–4 scale severity scale (Grattidge & O’Brien, Citation1982). Disease incidence was described in terms of incidence by village, which was the proportion of fields in a village with disease present, or incidence by field, which was the proportion of diseased plants in a field out of the total number surveyed in a field in which the disease was present. A subsample of plants (1–3 plants per field) with virus-like symptoms, leaf mottling and distortion, were tested for Tobacco mosaic virus (TMV), Pepino mosaic virus (PepMV), Cucumber mosaic virus (CMV), Impatiens necrotic spot virus (INSV) and Tomato spotted wilt virus (TSWV) using lateral flow (immunoassay) devices (Immunostrip, Agdia, Elkhart, IN). TYLCV was identified based on symptoms and rated on a 0–4 severity scale (Lapidot & Friedmann, Citation2002). Diseased samples were microscopically examined in the field using a CellScope (Skandarajah et al., Citation2014). Samples with diseases of unknown aetiology were examined at Sokoine University of Agriculture (Morogoro Town, Tanzania). Farmers who managed the surveyed fields were also asked about their pesticide usage and cropping history in an informal interview. Disease survey data were analysed using SPSS (IBM Corporation, Armonk, NY) and Minitab (Minitab Inc. State College, PA) using Pearson’s chi-square test to determine seasonal differences in incidence by village and a two-sample t-test to determine seasonal differences in incidence by field with an α = 0.10.
Root health bioassay
The presence and severity of soilborne pathogens and diseases were underestimated in field surveys as many farmers did not allow destructive sampling. Therefore, a modified root health bioassay (Gugino et al., Citation2009) was conducted to assess the prevalence and density (galls per g root) of root knot nematodes and the severity of root rot in each village surveyed. Soils were collected from all disease survey fields, air-dried and stored at ambient lab temperature (24–26°C) for up to two months, and then assayed for pathogens and diseases. A 150 mL sample of soil was placed in the bottom half of a 500 mL plastic soda bottle with holes added for drainage. Five replicates were used for each field, giving a total of 50 samples per village. Tomato ‘Moneymaker’ seeds were planted in the soil and seedlings were grown for 5 weeks under ambient environmental conditions (average minimum temperature: 18°C, average maximum temperature: 30°C) in a screenhouse. Tomato ‘Moneymaker’ was selected as a susceptible variety. Seedling roots were washed in water and examined for diseases. Root rot was rated on a scale of 0–4 (0: no root rot; 1: >0–25% root discoloured; 2: >25–50% root discoloured; 3: >50–75% root discoloured; 4: >75–100% root discoloured) and data were converted to the midpoint of root rot per cent severity for analysis. The number of root knot nematode galls per root system was counted. Fresh and dry shoot biomass, shoot length and dry root biomass were recorded from all samples.
Root rot severity and plant growth data were analysed by analysis of variance using the Minitab software package (Minitab Inc., State College, PA). Means were separated using Tukey’s HSD with a family-wise error rate of 0.05. Binary and proportional odds logistic regression models were fitted to the nematode data to estimate the odds of this pathogen being present and its population density (based on galls per g root) exceeding certain levels in each village. For the former analysis, each soil sample from each field was coded as either 0 (absence) or 1 (presence), and the model was fitted using the LOGISTIC procedure of SAS (Allison, Citation1999; Derr, Citation2013). PROC LOGISTIC was also used to fit the proportional odds model to the nematode data grouped into ordinal categories based on root gall counts. The categories were: 1 (low): <100 galls per g of dry root; 2 (moderate): 100–500 galls per g of dry root; and 3 (high): >500 galls per g of dry root. Contrast statements were used to estimate odds ratios and compare nematode presence and density among villages.
Results
Baseline survey results of farmer demographics and tomato production
Farmer participants in the baseline survey (n = 101) consisted of 73 males and 28 females, 97% of whom had completed primary school. Most farmers (45.5% of respondents) were between 34–49 years of age, followed by younger (age 18–33, 30.7%) and older (age 50+, 23.8%) farmers. Farmers had a median of six years of experience growing tomatoes. Tomatoes were grown on 0.4–7.3 hectares, with a median of 0.4 hectare, and were most often rotated with rice or maize (66% of respondents). Legumes (24%) or other vegetables (30%) were sometimes included in tomato rotations. Farmers produced mainly determinate, plum-shaped, processing type tomatoes, including ‘Onyx’ (55% of respondents), ‘Cal J’ (29%), ‘Tanya’ (28%), ‘Rio Grande’ (25%), ‘Mwanga’ (17%), a hybrid variety (9%), ‘Roma’ (5%) and ‘Tengeru 97‘ (1%). Farmers often obtained seeds from agrodealers (87% of respondents) or saved their own seed (24%). Tomato varieties were selected based on fruit size (60% of respondents), transportability (56%), fruit keeping quality (53%), disease resistance (25%) and insect resistance (25%). Farmers reported that tomato profitability was constrained by diseases (80% of respondents), insects (72%), market access (57%), drought (29%) and poor seed quality (20%).
Pesticide usage
Most of the interviewed farmers (94% of respondents, n = 101) stated in the baseline survey that they used fungicides to manage tomato diseases. Farmers identified 25 different fungicide trade names, representing 12 combinations of active ingredients. A little over half of the identified products (14 of 25) contained mancozeb. The five most commonly applied fungicides were Ivory 72 (mancozeb + metalaxyl, 38% of respondents), Linkmil (mancozeb + metalaxyl, 32%), Farmerzeb (mancozeb, 25%), Ridomil (metalaxyl + mancozeb, 21%) and Bayfidan (triadimenol, 13%). Approximately a third of farmers (34%) included insecticides in the pesticides applied to manage tomato diseases, while 13% applied foliar fertilizers to combat diseases. A moderate percentage of farmers (19%) included some form of cultural control (roguing, thinning, water management, etc.) in their disease management strategies.
Pesticide usage information was collected during the disease survey from 87 farmers who managed the surveyed fields, including some farmers who also participated in the baseline survey. Seventy-four farmers (85%) used fungicides to manage tomato diseases. Of these 74 farmers, 13 used more than one fungicide on their tomato crops. Two respondents stated they were currently using insecticides to manage tomato diseases at the time of the disease survey. Farmers provided 18 different fungicide trade names, representing eight different chemistries. Twelve of these fungicides contained mancozeb as an active ingredient. The most commonly applied fungicides were Linkmil (23% of respondents), Ivory M72 (22%) and Ebony (18%, the specific chemistry varied, consisting of either mancozeb alone or in combination with metalaxyl).
Local disease knowledge
Farmer groups provided 98 local tomato disease names during the ethnophytopathological activities (). Forty-six of these names (47% of responses) described the symptoms of the disease, 30 (31%) were words borrowed from other aspects of life, 17 (17%) attributed the disease to a causal agent, and five (5%) described the weather associated with the disease ( and ). For diseases attributed to a causal agent type (bacteria, fungi, virus or mite), farmers attributed the correct causal agent for 10 of 17 names (59%). Farmer groups in several villages correctly described late blight, early blight, anthracnose and Fusarium wilt as fungal diseases and bacterial wilt as a bacterial disease. Several groups also correctly attributed mite damage to insect damage. Several groups of farmers incorrectly attributed bacterial spot/speck, TSWV, pith necrosis and bacterial canker to fungal causal agents. Rasta, a viroid disease, was attributed to aphids in one village. A causal agent was never attributed to nine diseases (root knot nematode, Septoria leaf spot, leaf mould, TYLCV, cucumber mosaic virus, Verticillium wilt, bacterial speck, physiological leaf roll and powdery mildew) by the respondents in any of the villages surveyed.
Table 3. Local names for tomato diseases provided by farmers in response to a survey in the Morogoro Region of Tanzania. The disease(s) to which each name refers and its English translation are shown.
During the baseline survey, farmers self-identified the five most common diseases in their tomato crops during both the rainy and dry seasons (). For the rainy season, the most common responses (192 responses from 52 farmers) were late blight, root knot nematode, TYLCV, bacterial wilt and bacterial spot, whereas the most common dry season responses (288 responses from 68 farmers) included late blight, root knot nematode, bacterial spot, anthracnose and TYLCV.
Fig. 2 Farmer-identified key diseases for the rainy and dry growing seasons in the Morogoro Region of Tanzania. Farmers were asked to identify the most important tomato diseases in each growing season (up to five for each season) from a set of pictures of 18 commonly occurring diseases, one abiotic disorder and one insect pest. The percentage of respondents stating each disease is shown.
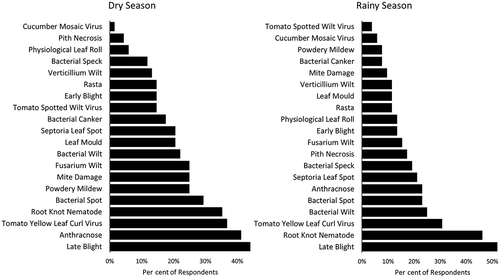
Prevalent tomato diseases
The diseases most commonly encountered during the dry season survey were early blight (42 of 50 fields), bacterial spot/speck (18), Septoria leaf spot (13), viral diseases (13) and grey mould (12 fields) ( and ). During the rainy season, the most common diseases were early blight (46 of 50 fields), bacterial spot/speck (31), viral diseases (29), Septoria leaf spot (21) and TYLCV (18). Incidence by village and incidence by field varied among diseases and growing seasons (). The mean incidence by village of Septoria leaf spot (P = 0.139, chi-square, df = 1) and early blight (P = 0.357) did not differ significantly between the rainy and dry seasons. However, mean incidences by village for bacterial spot/speck (P = 0.016), TYLCV (P < 0.0001), viral diseases (P = 0.004), and late blight (P < 0.0001) were significantly higher in the rainy season, while grey mould (P = 0.008) incidence was significantly higher in the dry season. Mean incidences by field of Septoria leaf spot (P = 0.354, df = 17), bacterial spot/speck (P = 0.189, df = 43), TYLCV (P = 0.74, df = 2) and viral diseases (P = 0.157, df = 38) did not differ significantly between growing seasons based on a two-sample t-test. Mean incidence by field of early blight was significantly higher during the rainy season than during the dry season (P = 0.053, df = 72), while mean incidence by field of grey mould was significantly higher during the dry season than during the rainy season (P = 0.015, df = 11). The severity of most diseases was low to moderate in both seasons (). Viruses were identified from five fields during the dry season (PVY in one field in Mlali and two in Msufini, and TMV in one field in Msongozi) and one during the rainy season (PVY in Mlali) using serological assays. Two new tomato diseases in Tanzania were reported from this survey: tomato pith necrosis caused by Pseudomonas cichorii (Testen et al., Citation2015b), and tomato big bud caused by a 16SrII-C subgroup phytoplasma (Testen et al., Citation2015a).
Table 4. Incidence and severity of tomato diseases during the dry (August–September 2013) and rainy (May–June 2014) seasons in five villages in the Morogoro Region of Tanzania. Disease incidence is shown by village (proportions of fields in each village in which the disease was present) and by field (percentage of diseased plants in fields where disease was present). Foliar disease severity was rated on a 1–4 scale: 1: no disease, 2: >0–25% disease (low severity), 3: >25–50% disease (moderate severity) and 4: >50% disease (high severity). Disease incidence and severity values are means of observations for 10 fields in each village.
Fig. 3 (Colour online) Incidence of tomato diseases in five villages in the Morogoro Region of Tanzania during dry and rainy production seasons. The radius of each circle, semicircle, or sector indicates the number of fields in which the disease was found in each village (max = 10), while circle colour indicates the disease.
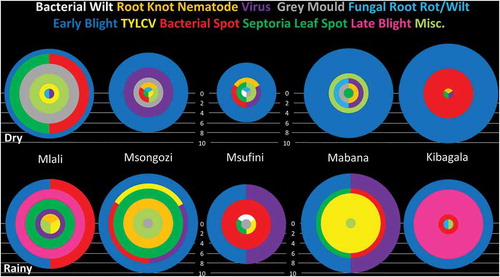
Root health bioassay
Root knot nematodes were detected in 44 of 50 soils using the tomato seedling root health bioassay (). Mean root rot severity was highest in Mabana and significantly higher than in Msongozi and Msufini. Mean shoot dry biomass, shoot length, and root dry biomass were significantly higher in tomato seedlings grown in soils from Kibagala than in soils from the other four villages (). Villages from which soils were collected also had a significant effect on the incidence (P = 0.048, )) and density (galls per g of dry root tissue, P = 0.007, )) of root knot nematodes. The odds of finding root knot nematodes ()) in Mabana were 3.3 times higher than in Msufini (P = 0.017), 3.7 times higher than in Mlali (P = 0.0083), 4.0 times higher than in Msongozi (P = 0.005) and 2.5 times higher than in Kibagala (P = 0.067). Similarly, the odds of finding this nematode at moderate to high density (> 100 galls per g of dry root) versus low density (< 100 galls per g dry root) ()) in Mabana were 3.4 times higher than in Msufini (P = 0.003), 3.0 times higher than in Mlali (P = 0.009), 3.1 times higher than in Msongozi (P = 0.006) and 4.3 times higher than in Kibagala (P < 0.001). This indicates that the risk of finding root knot nematode at higher population densities was highest in Mabana compared with all the other villages.
Table 5. Health of tomato seedlings grown in soils collected from 10 tomato fields in each of five villages in the Morogoro region of Tanzania, 2014. Seedlings were rated for root rot and root knot nematode (Meloidogyne spp.) incidence and severity. Shoot dry mass and length and root dry mass were determined.
Fig. 4 Odds ratios for comparisons of root knot nematode presence (A) and density (B) between pairs of villages in the Morogoro Region of Tanzania. Dots indicate estimated odds ratios, while vertical lines indicate the 95% confidence intervals around the point estimates. For those comparisons in which the confidence intervals do not intercept the dotted line, differences between the villages are not significant (P > 0.05). Estimates in A were obtained from the fit of a binary logistic regression model to presence/absence of nematodes data from a soil health assay (see text for details), whereas those in B were estimated through proportional odds logistic regression analysis of nematode gall density data, with 1: < 100 galls per dry g root, 2: 100–500 galls per dry g root, and 3: >500 galls per dry g root.
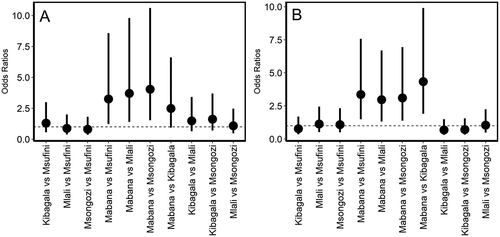
Discussion
While it was generally understood that diseases were a constraint to tomato production in the Morogoro Region of Tanzania (Maerere et al., Citation2010) when this study was undertaken, a systematic survey of multiple villages for prevalent diseases had not been conducted and information on farmers’ knowledge and disease management practices was not available. This dual approach provided information on both the most prevalent diseases and how local farmers perceive and understand plant diseases. The individual approaches have limitations, but the dual approach provides a broader picture of regional plant health in order to provide the best management options for farmers.
Field surveys of prevalent diseases and farmers’ rankings of diseases did not always agree. Early blight was the most prevalent disease identified in field surveys, and bacterial spot/speck, Septoria leaf spot and viral diseases, including TYLCV, were also commonly encountered. Farmers’ rankings of disease incidence could be influenced by actual disease incidence, management difficulty, or damage inflicted, but our methodology did not allow us to parse this information from farmers’ responses. Farmers did not rank early blight or Septoria leaf spot highly as common diseases in the region. Root knot was identified as a key disease in the region through the use of a root health assay, and farmers also identified this as a key disease. Although farmers reported late blight as a common disease in both production seasons, we only found this disease during the rainy season survey in multiple fields in two villages. Late blight is a potentially devastating disease in the region given conducive environmental conditions, such as during the rainy season. Bacterial wilt is another potentially devastating disease, but it was only identified in a few fields in Msufini.
Local knowledge of plant diseases must be considered when conducting surveys and making management recommendations. A critical aspect of ethnophytopathological knowledge is farmers’ disease naming systems, and it is important for researchers and extension agents to not only recognize local disease names, but also understand the meanings of these names (Bentley et al., Citation2009). We categorized local disease names as those based on symptoms, words borrowed from other aspects of life, causal agents and weather. Diseases were named most often based on the symptoms, as was the case in other studies (Bentley et al., Citation2009). The manner in which farmers name diseases can indicate how these diseases are perceived. For example, the local name for late blight was ‘fulifuli’, a Kiswahili word referring to cloudy, drizzly weather. This suggests that farmers correctly associated this disease with weather conditions conducive for its development. Farmers in one village provided the name of ‘fat disease’, due to leaf distortion, for CMV-infected tomatoes and indicated this disease was associated with aphids, indicating probable farmer knowledge of the viral vector.
Farmer naming strategies can also indicate if farmers differentiate between diseases with similar symptoms. For example, farmers in several villages usually provided different names for bacterial spot, Septoria and early blight, which indicates that they perceived subtle differences in the symptoms of these diseases. Names given to diseases may change as farmers receive formal education; these names suggest the influence of previous development projects and extension agents (Bentley et al., Citation2009). In Tanzania, farmers attributed wilts to either bacterial or fungal causes, likely indicating previous education on these diseases, but farmers did not always identify the correct causal agent of diseases. Knowing local disease names improves communication between farmers and researchers and benefits researchers by providing insight into farmers’ understanding of these diseases. Improved understanding of local plant disease knowledge can help researchers to identify and implement appropriate participatory research methods to improve disease management, such as participatory variety selection trials (Testen et al., Citation2016) or farmer field schools (Nelson et al., Citation2001).
Developing a better understanding of the most prevalent diseases in a region and farmers’ current management strategies is essential for improving disease management practices. This knowledge is especially important in limited resource environments in which farmers may only have resources to address specific diseases in their fields. Farmers relied heavily on pesticides to manage diseases, with few farmers indicating the use of cultural practices. These findings are similar to other studies in Tanzania, which indicated that farmers made frequent pesticide applications, including tank mixes, often without protective equipment (Ngowi et al., Citation2007; Maerere et al., Citation2010). We identified evidence of pesticide misuse that could be corrected through farmer education. According to the baseline survey, approximately one-third of farmers interviewed used insecticides to manage diseases and some also used foliar fertilizers to manage diseases. Early blight was found in the majority of fields despite the widespread use of mancozeb-based fungicides, potentially indicating that these fungicides are not being applied appropriately (such as using incorrect rates or incompatible tank mixes) or are of poor quality (old chemicals or adulteration; Lekie et al., Citation2014).
Information from disease surveys and ethnophytopathological studies can be combined to develop appropriate tools to assist farmers in identifying and managing key diseases. These tools can provide integrated disease management strategies to reduce farmers’ sole reliance on chemical pesticides. We developed disease identification and management cards (examples shown in Fig. S1) that contained pictures and descriptions of disease symptoms and management suggestions written in Kiswahili at a detail level relevant to farmers. English text was translated into Kiswahili by three Tanzanian researchers with years of experience working with Tanzanian farmers. Cards were developed for 11 diseases: bacterial spot, bacterial speck, bacterial wilt, bacterial canker, late blight, early blight/Alternaria stem canker, root knot nematodes, Septoria leaf spot, TYLCV, general tomato viruses and Fusarium wilt. These cards were distributed to 100 farmers in the five villages. These extension materials made an impact on farmers’ disease management practices. We visited Msufini, Mabana and Msongozi one year after the end of the project and asked farmers if they had changed their disease management practices and if yes, how had the management practices changed. Seventeen of 26 farmers interviewed indicated that they had changed their disease management practices. Thirteen of those 17 farmers indicated a reduction in spraying pesticides and eight of the 17 farmers indicated that they scouted for diseases. Nine of 26 respondents indicated no change.
This study serves as an example of how plant pathologists can develop a baseline understanding of key regional plant disease constraints through the integration of field surveys and ethnophytopathological studies. The inclusion of local knowledge is essential in these surveys, both to improve communication and identify appropriate plant health solutions for farmers. The methods outlined in this study provide a framework for future studies to assess and address plant health problems on smallholder vegetable farms.
TCJP 1414881 Figure S1
Download TIFF Image (19.3 MB)Acknowledgements
We thank the farmers and extension agents who participated in this project.
Supplemental material
Supplemental data for this article can be accessed online here: https://doi.org/10.1080/07060661.2017.1414881.
Additional information
Funding
References
- Adam RI, Sindi K, Badstue L. 2015. Farmers’ knowledge, perceptions and management of diseases affecting sweet potatoes in the Lake Victoria Zone region, Tanzania. Crop Prot. 72:97–107.
- Allison PD. 1999. Logistic regression using the SAS system: theory and application. Cary (NC): SAS Institute Inc.
- Bentley JW. 1989. What farmers don’t know can’t help them: the strengths and weaknesses of indigenous technical knowledge in Honduras. Agric Human Values. 6:25–31.
- Bentley JW, Boa ER, Kelly P, Harun-Ar-Rashid M, Rahman AKM, Kabeere F, Herbas J. 2009. Ethnopathology: Local knowledge of plant health problems in Bangladesh, Uganda and Bolivia. Plant Pathol. 58:773–781.
- Black R, Seal S, Abubakar Z, Nono-Womdim R, Swai I. 1999. Wilt pathogens of Solanaceae in Tanzania: Clavibacter michiganensis subsp. michiganensis, Pseudomonas corrugata, and Ralstonia solanacearum. Plant Dis. 83:1070.
- Chiang B-T, Nakhla MK, Maxwell DP, Schoenfelder M, Green SK. 1997. A new Geminivirus associated with a leaf curl disease of tomato in Tanzania. Plant Dis. 81:111–211.
- Czosnek H, Laterrot H. 1997. A worldwide survey of tomato yellow leaf curl viruses. Arch Virol. 142:1391–1406.
- de Cleene S. 2014. Agricultural growth corridors – unlocking rural potential, catalyzing economic development. In: Köhn D, editor. Finance food. Berlin Heidelberg: Springer Berlin Heidelberg; p. 67–87.
- Derr RE. 2013. Ordinal response modeling with the LOGISTIC procedure. In: SAS Institute Inc., editors. Proceedings of the SAS global forum 2013 conference. Cary (NC): SAS Institute Inc.
- Grattidge R, O’ Brien RG. 1982. Occurrence of a third race of Fusarium wilt of tomatoes in Queensland. Plant Dis. 66:165–166.
- Gugino BK, Idowu OJ, Shindelbeck RR, Van Es HM, Wolfe DW, Moebius-Clune BN, Thies JE, Abawi GS. 2009. Cornell soil health assessment training manual, edition 2.0. Geneva (NY): Cornell Univ.
- Kiros-Meles A, Abang MM. 2008. Farmers’ knowledge of crop diseases and control strategies in the Regional State of Tigrai, northern Ethiopia: implications for farmer–researcher collaboration in disease management. Agric Hum Values. 25:433–452.
- Lapidot M, Friedmann M. 2002. Breeding for resistance to whitefly-transmitted geminiviruses. Ann Appl Biol. 140:109–127.
- Lekie E, Ngowi A, London L. 2014. Farmers’ knowledge, practices, and injuries associated with pesticide exposure in rural farming villages in Tanzania. BMC Public Health. 14:389.
- Luzi-Kihupi A, Kashenge-Killenga S, Bonsi C 2012. A review of crop improvement research in Tanzania with specific focus on maize, rice, and horticultural crops. Morogoro, Tanzania: iAGRI Technical Report. http://iagri.org/student-portal/choosing-your-research-topic/.
- Maerere AP, Sibuga KP, Bulali JEM, Mwatawala MW, Kovach J, Kyamanywa S, Mtui HD, Erbaugh M. 2010. Deriving appropriate pest management technologies for smallholder tomato (Solanum lycopersicum Mill.) growers: a case study of Morogoro, Tanzania. J Anim Plant Sci. 6:663–676.
- Maerere AP, Sibuga KP, Mwajombe KK, Bulali J, William MN, Mwinyipembe L, Mbwambo J, Shayo J. 2006. Baseline survey report of tomato production in Mvomero District, Morogoro Region, Tanzania. Morogoro (Tanzania): Sokoine University of Agriculture.
- Manu-Aduening J, Lamboll R, Mensah G, Gibson R. 2007. Farmers’ perceptions and knowledge of cassava pests and diseases and their approach to germplasm selection for resistance in Ghana. Ann Appl Biol. 151:189–198.
- Mbega ER, Mabagala RB, Adriko J, Lund OS, Wulff EG, Mortensen CN. 2012. Five species of Xanthomonads associated with bacterial leaf spot symptoms in tomato from Tanzania. Plant Dis. 96:760.
- Mele PV, Cuc NT, Huis AV. 2001. Farmers’ knowledge, perceptions and practices in mango pest management in the Mekong Delta, Vietnam. Int J Pest Manag. 47:7–16.
- Minja RR, Ambrose J, Ndee A, Swai IS, Ojiewo CO. 2011. Promising improved tomato varieties for Eastern Tanzania. Afr J Hortic Sci. 4:24–30.
- Nagaraju N, Venkatesh HM, Warburton H, Muniyappa V, Chancellor TCB, Colvin J. 2002. Farmers’ perceptions and practices for managing tomato leaf curl virus disease in southern India. Int J Pest Manag. 48:333–338.
- Nathaniels NQR, Sijaona MER, Shoo JAE, Katinila N. 2003. IPM for control of cashew powdery mildew in Tanzania. I: Farmers’ crop protection practices, perceptions and sources of information. Int J Pest Manag. 49:25–36.
- Nelson R, Orrego R, Ortiz O, Tenorio J, Mundt C, Fredrix M, Vien NV. 2001. Working with resource-poor farmers to manage plant diseases. Plant Dis. 85:684–695.
- Ngowi AVF, Mbise TJ, Ijani ASM, London L, Ajayi OC. 2007. Smallholder vegetable farmers in Northern Tanzania: pesticides use practices, perceptions, cost and health effects. Crop Prot. 26:1617–1624.
- Nono-Womdim R, Swai IS, Mrosso LK, Chadha ML, Opena RT. 2002. Identification of root-knot nematode species occurring on tomatoes in Tanzania and resistant lines for their control. Plant Dis. 86:127–130.
- Nyankanga RO, Wien HC, Olanya OM, Ojiambo PS. 2004. Farmers’ cultural practices and management of potato late blight in Kenya Highlands: implications for development of integrated disease management. Int J Pest Manag. 50:135–144.
- Nyeko P, Edwards-Jones G, Day RK, Raussen T. 2002. Farmers’ knowledge and perceptions of pests in agroforestry with particular reference to Alnus species in Kabale district, Uganda. Crop Prot. 21:929–941.
- Obopile M, Munthali DC, Matilo B. 2008. Farmers’ knowledge, perceptions and management of vegetable pests and diseases in Botswana. Crop Prot. 27:1220–1224.
- Ojiewo CO, Swai IS, Oluoch MO, Silue D, Nono-Womdim R, Hanson P, Black L, Wang TC. 2011. Participatory cultivar evaluation, selection, and release of late blight resistant tomato cultivars in Tanzania. Acta Hortic. 911:199–206.
- Paavola J. 2008. Livelihoods, vulnerability and adaptation to climate change in Morogoro, Tanzania. Environ Sci Policy. 11:642–654.
- Poubom CFN, Awah ET, Tchuanyo M, Tengoua F. 2005. Farmers’ perceptions of cassava pests and indigenous control methods in Cameroon. Int J Pest Manag. 51:157–164.
- Segura HR, Barrera JF, Morales H, Nazar A. 2004. Farmers’ perceptions, knowledge, and management of coffee pests and diseases and their natural enemies in Chiapas, Mexico. J Econ Entomol. 97:1491–1499.
- Shenge KC, Mabagala RB. 2007. First report of bacterial speck of tomato caused by Pseudomonas syringae pv. tomato in Tanzania. Plant Dis. 91:462.
- Shenge KC, Mabagala RB, Mortensen CN. 2010. Current status of bacterial -speck and -spot diseases of tomato in three tomato-growing regions of Tanzania. J Agric Ext Rural Dev. 2:84–88.
- Sileshi GW, Kuntashula E, Matakala P, Nkunika PO. 2007. Farmers’ perceptions of tree mortality, pests and pest management practices in agroforestry in Malawi, Mozambique and Zambia. Agrofor Syst. 72:87–101.
- Skandarajah A, Reber CD, Switz NA, Fletcher DA. 2014. Quantitative imaging with a mobile phone microscope. PloS One. 9:e96906.
- Testen AL, Baysal-Gurel F, Mamiro DP, Miller SA. 2015a. First report of tomato big bud caused by a 16SrII-C phytoplasma in Tanzania. Plant Dis. 99:1854.
- Testen AL, Mamiro DP, Mtui HD, Nahson J, Mbega ER, Francis DM, Miller SA. 2016. Introduction and evaluation of tomato germplasm by participatory mother and baby trials in the Morogoro Region of Tanzania. HortScience. 51:1467–1474.
- Testen AL, Nahson J, Mamiro DP, Miller SA. 2015b. First report of tomato pith necrosis caused by Pseudomonas cichorii in Tanzania. Plant Dis. 99:1035.
- Thurston HD. 1990. Plant disease management practices of traditional farmers. Plant Dis. 74:96–101.
- Trutmann P, Voss J, Fairhead J. 1996. Local knowledge and farmer perceptions of bean diseases in the central African highlands. Agric Hum Values. 13:64–70.
- UNFAO. 2012. Food and Agriculture Organization of the United Nations Statistics (FAOSTAT). Rome (Italy): UNFAO.