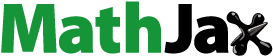
Abstract
In this article, we propose new tests of equal predictive ability between nested models when factor-augmented regressions are used to forecast. In contrast to the previous literature, the unknown factors are not estimated by principal components but by the common correlated effects (CCE) approach, which employs cross-sectional averages of blocks of variables. This makes for easy interpretation of the estimated factors, and the resulting tests are easy to implement and they account for the block structure of the data. Assuming that the number of averages is larger than the true number of factors, we establish the limiting distributions of the new tests as the number of time periods and the number of variables within each block jointly go to infinity. The main finding is that the limiting distributions do not depend on the number of factors but only on the number of averages, which is known. The important practical implication of this finding is that one does not need to estimate the number of factors consistently in order to apply our tests.
1 Introduction
Evaluating a time series models’ ability to forecast is one method of determining its usefulness. In the recent years, the predictive ability of factor-augmented regression models has attracted considerable attention, so much so that there is by now a separate strand of the forecasting literature devoted to such models. The reason for this development is that if the number of predictors is large and the comovement of those predictors is generated by a relatively small number of unobserved common factors, one can forecast a particular series by estimates of those factors rather than by the original predictors, with the benefit of significant dimension reduction.1
In most studies, the predictive content of the estimated factors is evaluated by comparing the mean squared forecast error (MSE) of point forecasts based on a model that includes estimated factors with one that does not include factors, such as a simple autoregressive model. Only if the estimated factors are able to bring about a reduction in MSE are they deemed useful for forecasting purposes. The evidence provided sofar is, however, mixed and far from conclusive, and this has in turn led to a lively discussion on whether factor-augmented models are really as useful as initially thought (see, e.g., Eickmeier and Ziegler Citation2008).
A drawback of pair-wise MSE comparisons that may well partly explain the inconclusive empirical evidence is that they ignore the uncertainty coming from the estimation of those MSEs. This is reflected in the empirical literature where researchers often apply already existing tests for equal forecast accuracy to their factor-based forecasts (see Stock and Watson Citation1999, Citation2002b; Camba-Mendez and Kapetanios Citation2005; Schumacher Citation2007; Ludvigson and Ng Citation2009, Citation2011; Kim and Swanson Citation2014; McCracken and Ng Citation2016 to mention a few). But then this means that the estimated factors are treated as though they were observed regressors, and it is not clear whether such a treatment is asymptotically justified (see Cheng and Hansen Citation2015). However, it is not until recently that the effect of the estimation of the factors has been investigated. Empirical researchers therefore have little or no option but to ignore it. The following quotation, taken from (Grover and McCracken Citation2014, p. 185), illustrates the sentiment in the literature: “[n]either the results in Diebold and Mariano (1995) nor those in West (1996) are directly applicable to situations where generated regressors are used for prediction. Even so, we follow the literature and use standard normal critical values.”
The present article is motivated by the above discussion. The purpose is to develop tests for predictability that account for the fact that the tested factors are not the true factors but just estimates, a problem that has received surprisingly little attention given the size of the literature. In fact, as far as we are aware, there is just one other article, namely Gonçalves, McCracken, and Perron (Citation2017), in which the predictive ability of the factors is tested using the well-known out-of-sample ENC-F and MSE-F test statistics (see, e.g., Inoue and Kilian Citation2005; McCracken Citation2007; Clark and McCracken Citation2001). We now position ourselves relative to this other article.
One of the assumptions in Gonçalves, McCracken, and Perron (Citation2017) that we would like to draw special attention to is that the number of factors, henceforth denoted r, is known. Gonçalves, McCracken, and Perron (Citation2017) did not justify this assumption; however, we note that it is very common in the literature.2 A common argument is that r can be consistently estimated and that this should leave the resulting forecast unaffected; however, there are no proofs, and even if consistency is enough, the estimation of r is likely to be very important in small samples (Leeb and Pötscher Citation2005). In fact, r has proven to be a very difficult object to estimate, and the resulting forecast can be very sensitive in this regard, to the point that many researchers have chosen not to rely on estimates, but to instead work with fixed numbers (see, e.g., Stock and Watson Citation2002a, Citation2002b; Citation2009, De Mol, Giannone, and Reichlin Citation2008; Chen, Dolado, and Gonzalo Citation2014; Cheng and Hansen Citation2015).
Another assumption in Gonçalves, McCracken, and Perron (Citation2017) is that the factors are estimated by applying the method of principal components (PC) to the full set of predictors. Typically, the variable to be forecasted is a macroeconomic variable, such as inflation or output, or a financial variable, such as excess returns on stocks or bonds, and in these cases it is standard to organize the predictors into blocks of variables, such as consumption, money aggregates, prices, exchange rates and so on, each of which contains a large number of series.3 The blocks are selected based on the definitional and behavioral similarities of the series that they contain, and it is not difficult to find evidence of their relevance for both factor estimation and forecasting (see, e.g., Hallin and Liška Citation2011; Ludvigson and Ng Citation2011; Moench, Ng, and Potter Citation2013). PC based on all the predictors ignores the blocks, which means that it does not take full advantage of the structure of the data (see Hallin and Liška Citation2011; Moench, Ng, and Potter Citation2013, for discussions). Another drawback of PC is that the estimated factors can be difficult to interpret (see, e.g., Ludvigson and Ng Citation2011; Castle, Clements, and Hendry Citation2013; Moench, Ng, and Potter Citation2013; McCracken and Ng Citation2016), and in the forecasting context accuracy it is not everything but one would also like to say something about the factors that matter. A common approach is to try to label the PC factors according to their relationship with the underlying series (see, e.g., Ludvigson and Ng Citation2009, Citation2011; McCracken and Ng Citation2016). However, such labels are only suggestive, as each factor estimate is influenced to some degree by all the series in the dataset.
In the present article, we take the same test statistics as in Gonçalves, McCracken, and Perron (Citation2017) but instead of using PC based on the full set of predictors to estimate the factors, we use a version of the very popular common correlated effects (CCE) approach of Pesaran (Citation2006). While initially proposed as a means to allow for interactive effects in panel data regressions, as Karabiyik and Westerlund (Citation2021) pointed out, CCE can be used also for forecasting purposes. The idea, laid out in detail in Section 2 of the present article, is to take the cross-sectional average of all the series within each block of predictors, and to use these as estimators of the factors. The main attractions of the approach are that it does not rely on correct specification of r, it accounts for the block structure of the data, and it is easy to interpret. It is also very easy to implement, which, by analogy to the forecast combination literature where the simple average tends to outperform more sophisticated combinations (see, e.g., Stock and Watson Citation2004), is expected to lead to good small-sample properties. The main restriction is that the number of averages used in the estimation, henceforth denoted m, cannot be smaller than r, which is analogous to the common assumption in the model selection literature that the researcher is able to set an upper bound for the dimension of the model (see, e.g., Bai and Ng Citation2002).
The asymptotic results of this article, which are presented in Section 3, depend critically on whether m = r or m > r. On the one hand, if m = r, which is tantamount to assuming that r is known, the feasible ENC-F and MSE-F test statistics based on the CCE factor estimates are asymptotically equivalent to their infeasible counterparts based on the true factors, and therefore the asymptotic distributions are unaffected by the estimation of the factors. If, on the other hand, m > r, so that the number of factors is over-specified, the effect of the estimation of the factors is nonnegligible, and therefore the asymptotic distributions are no longer the same as for the infeasible test statistics. Interestingly enough, however, the distributional effect is not detrimental in any way, but actually beneficial. Specifically, the redundant m – r factor estimates affect the asymptotic distributions in the same way as if there were just as many additional true factors present. This means that the critical values do not depend on r, but only on the known value of m, which is very useful in applied work, as r need not be known or estimated accurately in order to apply our tests. This is illustrated in Section 4 where we test the predictive ability of the factors in the most recent vintage of the popular FRED-MD dataset.
The article is concluded in Section 5. All proofs are provided the supplementary material, which also contains the results of a large-scale Monte Carlo study, as well as some additional theoretical results and implementation details. The Monte Carlo results confirm that the new tests perform well even when m > r, and that they do so under a variety of empirically relevant data-generating processes. Another finding is that the relatively simple and user-friendly CCE-based tests tend to perform at least as good as the main competitor based on PC.
2 Setup
Suppose that there are M predictors available, henceforth denoted , with
time series observations on each. The predictors can be divided into m blocks, and it is going to be convenient to assume that the number of series within each block is given by
and that m is finite.4 Let us denote by
the set that indexes the series contained in block
. It is convenient to think of the blocks as panel data variables and to think of the predictors within each block as the cross-sectional units of the panel dataset. In terms of the above notation, the predictor panel data variable is given by
, where
is the ith predictor in the bth block. Let us further introduce the time series variable
. This is the variable to be forecasted. The data-generating process considered for
and
is the same as in the bulk of the previous literature (see, e.g., Stock and Watson Citation2002a, Citation2002b; Bai and Ng Citation2006; Breitung and Eickmeier Citation2011; Gonçalves, McCracken, and Perron Citation2017), and is given by
(1)
(1)
(2)
(2) where
,
,
is a vector of variables that are known to be important for forecasting
, such as yt
and its lags,
is a vector of common factors,
is an error term that is unpredictable given the information available at time t,
is a matrix of factor loadings, and
is a vector of errors that are largely idiosyncratic.
The hypothesis of interest is that the factors in are irrelevant for forecasting yt
, which is tantamount to testing
.5 If
was observed, then this hypothesis could be tested using the usual ENC-F and MSE-F test statistics. These are two of the workhorses of the time series literature, and we will therefore use them here as a basis for the new CCE tests. In order to describe the ENC-F and MSE-F statistics, we divide the sample of T observations into an in-sample part containing the first R observations and an out-of-sample part containing the last
observations. Define the restricted and unrestricted residuals as
and
, respectively, where
(
) is the ordinary least-square (OLS) slope estimator in a regression of
onto
(
) for
. Let
be the estimated error variance based on the unrestricted residuals. In this notation,
(3)
(3)
(4)
(4) where the subscript “f” indicates that the tests are based on the true factors. In this article, we follow Inoue and Kilian (Citation2005), and formulate our null hypothesis in terms of the coefficient restriction imposed. However, one can also formulate the null in terms of the difference in expected loss from the restricted and unrestricted forecasting models. For example, for
, the null hypothesis can be stated as
, where
(
) is the population forecast error obtained by evaluating
(
) at the probability limit of
(
).6 But under our conditions,
(
) is consistent for
(
), and therefore the two formulations are equivalent. This equivalence is interesting, because it gives some intuition behind the construction of the test statistic. The basic idea is to test H0 by checking if the variances of the restricted and unrestricted forecasting errors are asymptotically equivalent. If H0 is true, then the two sets of errors should be asymptotically equivalent, and so MSE-Ff
will tend to be close to zero, whereas if H0 is false, said equivalence breaks down, and so MSE-Ff
will be relatively large. The intuition behind ENC-Ff
is slightly more involved and can be found in Clark and McCracken (Citation2013).
The problem with ENC-Ff
and MSE-Ff
is that is unobserved. This means that before a test of H0 can be mounted, the unknown factors have to be estimated somehow. Gonçalves, McCracken, and Perron (Citation2017) took the eigenvectors corresponding to the r largest eigenvalues of the sample covariance matrix of
. This is the PC approach. For reasons given in Section 1, however, in this article, we use the cross-sectional average of
. Let us therefore define
(5)
(5) where
for a generic matrix
. The resulting feasible versions of
and
are given by
and
, respectively, where
and
is obtained by regressing
onto
for
. Define
. The CCE-based test statistics that we will be considering in this article are given by
(6)
(6)
(7)
(7)
where the subscript “
” indicates that factors are estimated. It is important to note the ease with which these test statistics are computed. In fact, they are just as simple as their infeasible counterparts. Note in particular how, unlike PC, with CCE there is no need reestimate
for every
.7 The tests considered here are therefore not only very user-friendly but also fast, and the Monte Carlo results reported in the supplementary material confirm this.
The conditions that we will be working under when evaluating the asymptotic distributions of and
are stated in Assumptions U, Z, IP, E, IND, LAM, ALP, and PI. Here and throughout this article,
and
signify convergence in probability and weak convergence in measure, respectively,
is the integer part of x,
is the Frobenius (Euclidean) norm of A, and
is the vectorization operator that stacks the columns of A on top of one another. If B is also a matrix, then
denotes the block-diagonal matrix that takes A (B) as the upper left (lower right) block. Also,
means
. As above, vectors and matrices are written in bold.
Assumption
U. ,
,
, and
, where
is the sigma-algebra generated by
.
Assumption
Z.
and
are positive definite with probability one (wp1) for all
.
and
as
, where
(8)
(8)
and
are positive definite.
(c)
.
Assumption E.
, where
is such that
and
with
being the element of R that sits in row i and column n. Also,
with
, where
is independent across both t and i with
, and
.
The following matrices are positive definite:
(9)
(9)
(10)
(10)
Assumption
IP.(11)
(11) is strong mixing with coefficients of size
with b > 4 and
, and
is positive definite.
Assumption IND. and ut
are independent of
for all t, s and i.
Assumption LAM. is a nonrandom matrix, such that
as
and
, where
and
is full rank for all N, including
, and
. If m = r, then
.
Assumption ALP. , where
.
Assumption PI. as
.
Some comments on the above assumptions are in order. Assumption U is not very restrictive and allows for general types of (unconditional) heteroscedasticity (as in, e.g., Stock and Watson Citation2002a; Bai and Ng Citation2006; Gonçalves and Perron Citation2020).
Assumption Z imposes only minimal conditions on . In fact, the only requirements are that the variables in
cannot be collinear and that some moments exist. The variables can be both deterministic and stochastic, and the stochastic variables are not required to be serially uncorrelated, provided that they are stationary, nor exogenous. Of course, if some of the variables are predetermined, then ut
must be serially uncorrelated, as usual. Serial correlation is not ruled out, though, but then
cannot be predetermined.
Assumption E allows weak dependence in over both i and t, and is similar to the conditions in Pesaran and Tosetti (Citation2011). The dependence over i is assumed to be spatial in nature with R being the so-called network matrix, which is a convenient way of relaxing the otherwise so common cross-section independence assumption without for that matter having to resort to abstract high-level moment conditions. The forms of serial dependence that can be permitted under Assumption E are very general, although they cannot be strong, as in the presence of unit roots. Assumption E also allows general forms of heteroscedasticity across the cross-section, but not across time, which is similar to (Gonçalves, McCracken, and Perron Citation2017, assump. 2). Time heteroscedasticity is possible but then only for
, and at the expense of having to impose more restrictive conditions on ut
, as we show in the supplemental material. As usual,
must be independent of
and ut
, and this is where Assumption IND comes in.
Assumption IP ensures that satisfies an invariance principle. This condition is basically the same as in McCracken (Citation2007), and Clark and McCracken (Citation2001), and is used to ensure that the estimation of
in the infeasible test statistics does not have a dominating effect in the asymptotic theory. The condition is not necessary, but it simplifies the proofs.
As explained in Section 1, the null hypothesis of interest is given by . However, we do not want to restrict our attention to the null, but would also like to study the effect of the estimation of the factors on local power. We therefore follow Inoue and Kilian (Citation2005), and assume that
is local-to-zero. However, we do not restrict
to be different from zero, which means that Assumption ALP covers both the null and local alternative hypotheses. Hence, in what follows, we test
versus
.
Assumption PI requires that , which implies
. The condition that
therefore ensures that
is nonzero even asymptotically. This is intuitive, as
and
are based on comparing the restricted and unrestricted out-of-sample residuals, and there are only P observations on these. Hence, for
and
to be well defined, P cannot be too small (relative to T). If, on the other hand,
, then
, which is also not allowed, as there are only R observations available for the initial estimation of
, and therefore R cannot be too small (relative to T). By requiring that
(12)
(12)
Assumption PI ensures that R is sufficiently large.
We have saved Assumption LAM to last. We will discuss this assumption at length, because it illustrates how the rank of impacts the asymptotic distribution
and
when there is uncertainty over r. We begin by inserting (2) into EquationEquation (5)
(5)
(5) , giving
(13)
(13) which highlights the relevance of
for the estimation of
. This is where Assumption LAM comes in. If m = r,
is full rank and invertible, which means that EquationEquation (13)
(13)
(13) can be rewritten as follows:
(14)
(14)
Because under Assumption E, we have
uniformly in t, and hence
is consistent for
. In practice we never observe
. However, since
, it is enough if we know
, because
is subsumed in the estimation of
. The challenge in the case when m = r is therefore to show that the effect of
is negligible also in the tests, which we do in Section 3.
The case when m > r is more problematic. One reason for this is that is no longer invertible. However, we still need an equivalent of EquationEquation (14)
(14)
(14) , because it determines the object that is being estimated. The way we approach this issue is by introducing the following rotation matrix, which is chosen such that
and that is going to play the same role as
under m = r:
(15)
(15) where
and
. If m = r, we define
. We further introduce
with
if m = r. By premultiplying
by
, we obtain
(16)
(16)
where
and
with
and
being the partitions of
. If m = r, then
,
and
, and so we are back in EquationEquation (14)
(14)
(14) . Hence, since
and
, when m > r we are no longer estimating
but rather
.8 The fact that
is included in this object suggests that asymptotically CCE should be able to account for the unknown factors even if m > r. By ensuring the existence of
, Assumption LAM makes this possible. However, we also note that because of the presence of
, the asymptotic distribution theory will depend on whether m = r or m > r. In Section 3, we elaborate on this.
Remark 1.
Note that in EquationEquation (2)(2)
(2)
is time-invariant. This means that we do not allow the loadings to be breaking. The main reason for neglecting breaks is threefold. First, we can show that the proposed tests are unaffected by “small” breaks that shrink to zero at rate
, which is in agreement with the finding of Stock and Watson (Citation2002a, Citation2009) that local-to-zero breaks has no effect on the estimated PC factors. Second, as we demonstrate in Section 4, the condition that the loadings are time-invariant is testable. Third, provided that m is large enough, the proposed tests are invariant also to “big,” non-shrinking, breaks. The intuition, which draws on the work of Chen, Dolado, and Gonzalo (Citation2014), and Breitung and Eickmeier (Citation2011), goes as follows. Suppose that at time
the loadings change from
to
. Let
be the resulting time-varying version of
with
being the indicator function for the event A taking the value one when A is true and zero otherwise. This means that the common component of
can be written as
(17)
(17) where
and
. Hence, the model with breaking loadings can be written equivalently as a model without break but with 2r factors. In the supplemental material, we show that the asymptotic distributions of our tests (without modification) are unaffected by this change provided that
satisfies Assumption LAM, such that
. We also use Monte Carlo simulations as a means to investigate the effect of big breaks in small samples. According to the results, the effect almost nonexistent, just as expected.
3 Asymptotic Analysis
By adding and subtracting appropriately, and
, can be written as follows:
(18)
(18)
(19)
(19)
Gonçalves, McCracken, and Perron (Citation2017) showed that when is the PC estimator based on taking r as known, the feasible and infeasible test statistics are asymptotically equivalent. The authors do this by verifying that all the terms that appear on the right-hand side of each of EquationEquations (18)
(18)
(18) and Equation(19)
(19)
(19) are negligible, except the first. Our analysis is similar in that it is based on evaluating the limiting behavior of each of the terms on the right-hand side of EquationEquations (18)
(18)
(18) and Equation(19)
(19)
(19) . The results are, however, materially different.
Let us start by considering , the last term on the right-hand side of EquationEquation (19)
(19)
(19) . This term is simplest and will allow us to develop some intuition behind the results. Readers that are not interested in intuition should go directly to Lemma 2 and the analysis that follows it. We begin by noting that by the definitions of
and
,
(20)
(20)
Consider . It is convenient to partition
, where
and
conforms with
and
, respectively.9 While
is not necessarily square under Assumption LAM, it has full column rank, which means that we can compute its Moore–Penrose inverse, henceforth denoted
. In the proof of Lemma 1 in the supplemental material, we show that this inverse is given by
, such that
. It follows that
(21)
(21) where the last equality is obtained by letting
and
, and noting that
by EquationEquation (16)
(16)
(16) . By using this and the fact that
can be written as
, where
is invertible (see the proof of Lemma 1), EquationEquation (20)
(20)
(20) becomes
(22)
(22)
Since is obtained from a correctly specified regression model,
uniformly in t (see Corradi, Swanson, and Olivetti Citation2001), and by Assumption ALP the order of
is the same. Hence, since
, we obtain
(23)
(23) uniformly in t. The second term on the right-hand side of EquationEquation (22)
(22)
(22) is therefore negligible. Lemma 1 is concerned with the remaining first term. It can be seen as the CCE equivalent to (Gonçalves, McCracken, and Perron Citation2017, lem. 4.1).
Lemma 1.
Under Assumptions U, Z, E, IND, LAM, ALP and PI, as ,
uniformly in t, where
with
and
if m > r, and
if m = r.
Making use of EquationEquation (23)(23)
(23) , Lemma 1 and the fact that
under m > r, EquationEquation (22)
(22)
(22) becomes
(24)
(24) which again holds uniformly in t. Using Lemma 1 and EquationEquation (24)
(24)
(24) , we can now start to develop some intuition about the asymptotic behavior of
, the formal proof of which will appear in Lemma 2. The result in EquationEquation (24)
(24)
(24) implies that this term admits to the following asymptotic representation:
(25)
(25)
where the first term on the right is
, because
and
are. Moreover, since
, we have
. It should therefore be possible to show that
(26)
(26)
Because only depends on
, we know that
is a martingale difference process. A central limit law therefore applies to the first term on the right-hand side, giving
(27)
(27) since
by Assumption PI. Hence,
. Moreover, since
by standard arguments (see Cavaliere, Rahbek, and Taylor Citation2010), we have
. Finally, since
, where
and
are both
, we expect that
(28)
(28)
Lemma 2 provides rigor to these heuristic arguments.
Lemma 2.
Suppose the conditions of Lemma 1 are met with m > r. Then, as ,
By using Lemma 2, EquationEquations (18)(18)
(18) , Equation(19)
(19)
(19) , and Equation(27)
(27)
(27) , and the consistency of
, we can show that
and
admit to the following asymptotic representations when m > r:
(29)
(29)
(30)
(30)
The terms on the right-hand side that depend on are due to the estimation of the factors. Note, however, that since
under m = r, in this case (22) reduces to
(31)
(31)
Hence, what was previously non-negligible is now negligible, suggesting that the right-hand side sums in Lemma 2 should also be negligible. Corollary 1 confirms that this is indeed the case.
Corollary 1.
Suppose the conditions of Lemma 1 are met with m = r. Then, as ,
Corollary 1, EquationEquation (27)(27)
(27) and the consistency of
imply that if m = r, all terms on the right-hand side of EquationEquations (18)
(18)
(18) and Equation(19)
(19)
(19) but the first are negligible, and so
(32)
(32)
(33)
(33) which is the CCE counterpart of the asymptotic equivalence result of Gonçalves, McCracken, and Perron (Citation2017) without restrictions on the relative expansion rate of N and T.10 Hence, if m = r, the effect of the estimation of the factors, which is due to the use of too many estimates, drop out. Knowing
is therefore as good as knowing
itself, at least in large samples.
The asymptotic null distributions of and
are derived in the supplemental material. They are given by
(34)
(34)
(35)
(35) where
and
is a standard Brownian motion on
. If H0 is true,
, and therefore the asymptotic distributions in EquationEquations (34)
(34)
(34) and Equation(35)
(35)
(35) reduce to
and
, respectively, which are free of nuisance parameters. This is convenient because it means that the tests can be implemented using the critical values provided by McCracken (Citation2007), and Clark and McCracken (Citation2001). The main issue here is that since
is
, the critical values depend on the unknown r, and there is no reason to believe that m should be equal to r. Lemma 3 is concerned with the more realistic case when m > r.
Lemma 3.
Suppose that Assumptions U, Z, E, IND, LAM, ALP and PI are met with m > r. Then, as ,
The asymptotic distributions reported in Lemma 3 are free of nuisance parameters, which in view of EquationEquations (29)(29)
(29) and Equation(30)
(30)
(30) means that the distributional effect of the estimation of the factors is also free of such parameters. In fact, since
(36)
(36) where
if m = r and
if m > r, we can show that the asymptotic distribution of
is given by
(37)
(37)
as
. Similarly, since
(38)
(38)
the asymptotic distribution of
is given by
(39)
(39)
Theorem 1 summarizes these results.
Theorem 1.
Under Assumptions U, Z, E, IND, IP, LAM, ALP and PI, as ,
According to Theorem 1, the only terms in the asymptotic distributions of and
that involve
are those that are independent of
, and these terms have exactly the same form as those appearing in the asymptotic distributions of
and
with
replaced by
. This has two important implications.
One implication is that, since the asymptotic null distributions have the same form as before, the critical values can again be taken from McCracken (Citation2007), and Clark and McCracken (Citation2001). The main difference is that the choice of which critical values to use only depends on the known value of m. We want to stress the elegance of this result. Logic from classical theory for regressions in stationary variables dictates that the effect of redundant regressors should be negligible. When m > r, the unrestricted model has m – r factor estimates that are redundant. Yet, their effect is non-negligible. The reason for this seemingly counterintuitive result is that under H0 the factors are irrelevant and therefore the coefficients of all factor estimates are estimated to zero, irrespectively of whether they are redundant under H1. The asymptotic null distributions therefore only depend on the total number of factor estimates. This result is very interesting not only by itself but also for its implications for applied work. Note in particular how the implementation of the CCE-based tests does not require any knowledge of r, provided that .
Another implication of Theorem 1 is that while the asymptotic null distributions are affected by over-specification of the number of factors, the terms that drive local asymptotic power are not. However, this does not mean that power is unaffected by m. Let us illustrate this point using . Let
(40)
(40) and denote by
the α-level critical value obtained from the distribution of
. A direct calculation reveals that the variance of D is given by
(41)
(41)
where the dependence on m again comes from the dimension of
. By using this and Cantelli’s inequality (see, for example, Ghosh Citation2002), the probability of rejecting H0 in large samples is given by
(42)
(42)
where the inequality holds, provided that
. Hence, since
is increasing in m, power is decreasing in m, and it is easy to verify that the derivative of the expression on the right-hand side of EquationEquation (42)
(42)
(42) with respect to m is indeed negative. However, since the second derivative is positive, the reduction in power is decreasing in m. Hence, while there is a “cost” to over-specifying the number of factors, this cost is decreasing in m. We also see that the effect of m is going to be smaller the larger is
. This is intuitive, since
, which means that
is the only term that affects the mean of the asymptotic distribution of
. The power of the test is therefore going to be dominated by
. These predictions are for
; however, we can show that the same is true for
. In the supplementary material, we evaluate the accuracy of these predictions in small samples. The main conclusion is that while power is generally decreasing in m, the effect is not detrimental.
Remark 2.
All the results reported so far are based on the assumption that . In applications involving relatively small values of m, however, we cannot rule out the possibility that m < r. Under-specification of the number of factors does not interfere with the asymptotic null distributions of
and
, because under H0 the factors irrelevant for forecasting
. It may, however, affect local asymptotic power. In particular, the concern is that under-specification may lead to low power relative to that achievable under
. The intuition here is simple. Suppose that there are two factors, but that only one of them is useful for forecasting. This is impossible to detect if the tests are based on just one average that loads only on the irrelevant factor.
4 Empirical Illustration
4.1 Data
In this section, we illustrate the empirical usefulness of our proposed tests using the FRED-MD database of the Federal Reserve Bank of St. Louis (see McCracken and Ng Citation2016, for a detailed description), which builds on the very popular “Stock–Watson dataset.” The database is publicly available and updated on a monthly basis. Here, we use the 2020-07 vintage, which is the most recent vintage available to us. The main reason for our interest in the FRED-MD data is this: “it is important that the variables in the FRED-MD have good predictive [ability] when used in diffusion index forecasting exercises” (McCracken and Ng Citation2016, page 583). This article is not the first to investigate the predictive ability of the factors in the FRED-MD data, but it is the first to do so using tests that accounts for the fact that both the factors and their number are not known.11
The 2020-07 vintage of the FRED-MD dataset consists of 128 monthly time series, which are classified into eight blocks; (i) output and income, (ii) labor market, (iii) housing, (iv) consumption, orders and inventories, (v) money and credit, (vi) interest and exchange rates, (vii) prices, and (viii) stock market. The time span is 1959:1–2020:6. However, we follow the usual convention in the literature and take 1960:1 as the start of the sample. Many of the series are non-stationary, which, as pointed out in Section 2, is not permitted under our assumptions. The data are therefore transformed by taking logs, first or second differences when necessary, as in McCracken and Ng (Citation2016). Because of the differencing, the sample used in the analysis starts in 1960:3 and, because of missing observations in 2020:4–2020:6, the sample ends in 2020:3 for a total of 721 observations per series. Series 64, 66, 101, and 130 (in terms of the identifiers of McCracken and Ng Citation2016, appendix) and VXOCLSx (new to the dataset) have large sections of missing observations in either beginning or end, and are therefore discarded, similarly to Breitung and Eickmeier (Citation2011). The total number of series is therefore given by 123. The variables to be forecasted are INDPRO (industrial production), PAYEMS (non-farm employment), and CPIAUCSL (CPI inflation), similarly to McCracken and Ng (Citation2016).12 All series are screened for outliers.13
4.2 Implementation
In most studies of the Stock–Watson dataset the number of common factors, r, is a key issue. Different approaches have been used; however, most studies estimate six to 10 factors, which seems excessive given the estimates reported in the bulk of the empirical factor model literature (see Stock and Watson Citation2005). Stock and Watson (Citation2005) applied PC and two of the information criteria of Bai and Ng (Citation2002) with which they estimate seven factors, although they mention that the criteria are almost flat for between six and 10 factors. Breitung and Eickmeier (Citation2011) made the same observation. Because of this, they decide not to take the estimated number of factors but to instead report results for between six and nine factors. Bai and Ng (Citation2007) estimated seven factors, but they do mention that there is substantial uncertainty over this number, and that four or five factors might actually be enough.14 In fact, as Breitung and Eickmeier (Citation2011), and Bai and Ng (Citation2007) pointed out, the number of factors might not even be constant but changing as a result of structural breaks in the factor loadings. In other words, there is substantial uncertainty over the appropriate number of factors to use, which means that it is important to use tests that are robust in this regard. The CCE-based tests considered here do not rely on consistent estimation of r, but only that the number of averages, m, is not smaller than r.
The robustness with respect to the number of factors is one reason for considering CCE. Another reason is that it lends itself to easy interpretation of the estimated factors. In the current illustration, there are eight blocks. These have been put together on purpose, because the series they contain share some common feature, or are expected to do so (see Hallin and Liška Citation2011, and Moench, Ng, and Potter Citation2013). They are therefore relevant for estimation of the factors. If we take one average per block, then the estimated factors will not only be economically meaningful, but their number should also be large enough to capture the true number of factors. The first pair of tests that we consider is therefore the “plain vanilla” ENC-F and MSE-F tests based on taking all eight blocks of the FRED-MD dataset as given.
We have already shown that while over-specification of the number of factors does not invalidates our tests, it may to lead to a loss of power. The way researchers usually deal with such problems is to do model selection before computing their test statistics. Model selection is likely to lead to more parsimonious and less noisy model specifications, and hence to more accurate tests. Motivated by this, in addition to the plain vanilla tests, we report ENC-F and MSE-F test results based on using the eigenvalue-based growth ratio (GR) test procedure of Ahn and Horenstein (Citation2013) to select which factor estimates to retain.15
The third test pair is designed to deal with the situation in which there is uncertainty over the relevance of the given block structure. Bonhomme and Manresa (Citation2015) proposed using k-means clustering, which is quite natural given our objective to estimate both the blocks and the cross-sectional averages of those blocks. As pointed out by Ando and Bai (Citation2016, Citation2017), however, k-means supposes that the common component of the panel data is made up of block-specific time fixed effects, which in terms of EquationEquation (2)(2)
(2) means that the loadings are restricted to be either zero or one. They therefore propose an extension that is able to handle more general factor structures, and this is the one we use.16
We therefore consider three versions of the proposed CCE-based ENC-F and MSE-F tests. We would like to point out, though, that neither GR selection nor clustering is necessary in order to implement our tests, and that the reason for why we include them here is to see if there are any advantages to using these more elaborate approaches.
Another three pairs of tests based on PC are considered. The first pair is ENC-F and MSE-F based on PC and the GR procedure to determine the number of factor estimates to use. The second pair again uses PC but this time we apply it in a block-by-block fashion and retain one factor per block (as in, e.g., Castle, Clements, and Hendry Citation2013, and Ludvigson and Ng Citation2011). The third and final pair is the same as the second, except that it uses GR to select among the block-specific factor estimates. As we point out in Section 1, the only proof available of the validity of the PC-based tests requires that r is known, which is not the case here. The results obtained by using these tests should therefore be interpreted with caution. We still include them, though, as PC represents the closet competitor to CCE.
There is ample empirical evidence to suggest that deep lags (and squares) of estimated factors are not very important for the accuracy of the forecast (see, e.g., Bai and Ng Citation2009, Kim and Swanson Citation2014, and Stock and Watson Citation2002b), and our preliminary results confirm this. In this section, we therefore set , leading to a model that is slightly more general than the difficult-to-beat “DI” specification of Stock and Watson (Citation2002b) with only a constant and two PC factors.
4.3 Results
Before we come to the predictability test results, in we report some preliminary results for the full sample, which are intended to check some of the assumptions of Section 2. One of the assumptions is that the data are stationary, which is not an issue as the data are differenced to stationarity. Another assumption is that the loadings are time-invariant. As pointed out in Remark 1, breaking loadings are tantamount to a break in the number of factors from r to 2r. If , the estimated factors capture both the underlying factors and the break, and so there is no problem. If, however,
, the estimated factors need not be consistent anymore, because their number is not large enough to capture the breaking factors, which can in turn lead to tests with low power. In order to shed some light on this issue, we test for structural breaks in the loadings using the sup-LM test statistic of Chen, Dolado, and Gonzalo (Citation2014). According to the results reported in , there is no evidence against the no break null hypothesis, not even at the 10% level, which is consistent with the findings of Bai and Ng (Citation2009), and De Mol, Giannone, and Reichlin (Citation2008).
Table 1 Preliminary empirical results for the full sample.
Another assumption is that the error term in the model for , is at most weakly cross-sectionally correlated. In order get a feeling for the validity of this assumption, we look at the cross-correlations from across all pairs of residuals obtained by regressing
onto
for all
. reports the simple average and the average absolute value of these pair-wise correlations. The average correlations are very small and range from 0.008 to 0.053, which we take as evidence in support of the at-most-weak cross-sectional correlation assumption. The average absolute correlations are naturally higher, although not by much, and vary between 0.077 and 0.112. Regardless of which correlation measure we look at, the lowest value is always obtained by using plain vanilla CCE. This is interesting because it means that the simplest approach is also the one that is best at capturing the co-movement of the predictors.
In this illustration, there are eight blocks, which means that there can be at most eight factors. The fact that the residuals seem to be only weakly cross-sectionally correlated suggests that all the factors have been accounted for and hence that . As a further test of the validity of this condition, we apply the GR procedure, which in addition to being penalty-free does not depend on how the factors are estimated. The maximum number of factors is therefore not bounded by the number of cross-sectional averages. According to , the number of factors is estimated to two, which is in line with the two to three factors found in Bai and Ng (Citation2019), and Gorodnichenko and Ng (Citation2017). The estimated number of factors is therefore much smaller than m.
The results reported so far suggest that there are no major violations of the assumptions of Section 2, which in turn suggests that the CCE-based tests should perform well here. We therefore move on to the predictability test results reported in , which also contains some results on the ratio of the MSE of the unrestricted factor-based forecast relative to the restricted, purely autoregressive, forecast under the null. Stock and Watson (Citation2002a, Citation2002b) use the period 1960:3–1969:12 for the initial estimation, and make the first forecast in 1970:1. In addition to this, here we follow Bai and Ng (Citation2008), and McCracken and Ng (Citation2016), and consider two alternative out-of-sample starting dates, 1990:1 and 2008:1. The main reason for doing so is that while the loadings seem time-invariant, the parameters of the forecasting model may still be subject to structural change. If they are, we would expect to see some time variation in the predictive ability of the factors. Hence, in this illustration, R, the size of the in-sample period, varies from 117 to 573. The estimation of the number of factors and blocks is done once using only the in-sample observations, and is maintained throughout the out-of-sample period.17 This ensures that the tests are not biased toward the factor-based forecasts. That being said, the estimated number of factors turned out to be vary stable, and is estimated to two regardless of the choice of out-of-sample period, just as in the full sample. Clark and McCracken (Citation2001) only report critical values for , which is not enough for the tests based on the cross-sectional averages of all eight blocks. We therefore ended up simulating our own critical values. As usual, the tests are set up as one-sided, to the right (see Clark and McCracken Citation2001, Citation2005, Citation2013).
Table 2 Pseudo out-of-sample predictability test results.
The first thing to note from the results is that the block-based tests generally lead to the strongest evidence against the null. The test values are generally larger for the tests that use all eight block-specific factor estimates than for those that retain only the two GR-selected ones. However, this does not mean that the evidence against the equal predictability null is stronger for the tests that employ more factors, as the critical values are increasing in the number of factor estimates (see Clark and McCracken Citation2001; McCracken Citation2007). As a reflection of this, we see that in terms of significance, there is almost no difference depending on how many block-specific factors that are being retained. GR selection therefore does not lead to any advantage in this regard. We similarly see that there is no direct advantage to using PC over CCE, which is in agreement with the Monte Carlo results reported in the supplemental material.
The weakest evidence is obtained by using either the cluster-based CCE tests or the PC tests based on estimating two factors from the full panel dataset. In fact, these versions of the CCE and PC tests tend to lead to very similar results. This is due to the composition of the clusters, which is largely consistent with existing classifications of the first two PC factors (see Ludvigson and Ng Citation2009, Citation2011).18 Hence, estimating the blocks by clustering and then taking the cross-sectional average of each cluster leads to very similar results as when applying PC to all series and retaining only the GR-selected factor estimates, which is reasonable, since both the clustering and PC are minimum sum-of-squares-based. The fact that the evidence against the null becomes stronger when using the given block structure of the data to estimate the factors supports the idea that the blocks contain information that is valuable for forecasting. It also strengthens the already reported evidence suggesting that m is large enough, as under-specification should make it difficult to reject the equal predictability null.
We have compared the results across the six tests considered. Let us now compare the results based on the variable that is being forecasted. If we ignore the cluster-based CCE tests and the PC tests based on the GR-selected factor estimates, while quite strong also for PAYEMS, the evidence is more overwhelming for INDPRO and CPIAUCSL, which is partly consistent with the results of McCracken and Ng (Citation2016). The fact that the ENC-F tests typically lead to stronger evidence against the null than the MSE-F tests is consistent with their relatively high power documented in the Monte Carlo study of the supplemental material.
If we compare the results for the three out-of-sample periods, then we see that the ENC-F test values are generally decreasing in the length of the in-sample period, P. Similarly to before, however, this does not mean that the evidence against the equal predictability null is getting weaker, as the critical values are decreasing in P, too (see Clark and McCracken Citation2001, and McCracken Citation2007). The conclusions based on the ENC-F test are therefore not affected by the choice of out-of-sample period. However, the same cannot be said about MSE-F. Note in particular how most MSE-F test values for INDPRO and PAYEMS go from positive and significant in the 1970:1–2020:3 out-of-sample period to highly negative in the other periods, which is suggestive of structural breaks (see Clark and McCracken Citation2005; Giacomini and Rossi Citation2009).19 If the coefficient of is breaking, then the factors have some predictive content and the null of equal predictability cannot hold. The asymptotic null distributions of our tests therefore continue to apply even in the presence of breaks. The problem is that breaks can lead to relatively low power when compared to the case without breaks. Clark and McCracken (Citation2005) evaluated the extent of this power loss for the original ENC-F and MSE-F tests. They find that there are cases in which the MSE-F test is relatively more affected, and hence that “the two statistics can lead to different conclusions in the same environment” (p. 11). This is exactly what we see in . More to this point, as noted by a referee of this journal, according to (Clark and McCracken Citation2005, lem. 2), breaks can cause the MSE-F test statistic to diverge to negative infinity, which means that large negative values can actually be taken as evidence in favor of the unrestricted factor-based forecasting model. We therefore simulated two-sided critical values (available upon request). Based on these, most of the negative MSE-F test values reported in for INDPRO and PAYEMS in the 1990:1–2020:3 and 2008:1–2020:3 out-of-sample periods are significant (at the 1% level). Hence, if we account for breaks by making MSE-F two-sided, then both tests provide evidence of predictability of the factors in all three out-of-sample periods considered.
All-in-all, we find that the estimated factors based on the FRED-MD data are useful for forecasting, but that the strength of the evidence depends on whether the information contained in the blocks are accounted for. We also find that the evidence against the null is just as strong for the plain vanilla ENC-F and MSE-F tests as for any of the other, more sophisticated, variants of these tests. The extra effort therefore does not seem to pay off, which is consistent with the Monte Carlo results reported in the supplemental material where again the plain vanilla tests tend to perform at least as well as the other tests. This is reassuring because we have shown in the theoretical analysis of Section 3 that the plain vanilla tests are also asymptotically justified.
5 Conclusion
Factor-augmented predictive regressions are becoming increasingly popular, so much so that there is by now a separate literature devoted to them. Most of this literature is empirical; however, there is also a large and growing body of theoretical work concerned with the development of suitable econometric tools. The present article falls within this last category of works. The purpose is to develop tests that can be used to test the predictive ability of the factors when both the factors and their number, r, are unknown, a problem that seems to have been largely overlooked in the previous literature. The only exception known to us is the article of Gonçalves, McCracken, and Perron (Citation2017), in which the factors are treated as unknown and estimated using PC. However, these tests treat r as known. Moreover, the estimated PC factors are difficult to interpret and they do not make use of the block structure of the data. The proposed tests can be seen as a reaction to this. They use CCE, or block-by-block averaging, which is easy to interpret, it exploits the block structure of the data, and it is very user-friendly and easy to implement. More importantly, the tests do not require any knowledge of r, provided that it is not larger than the number of blocks, m.
Supplemental Material
Download PDF (419.5 KB)Acknowledgments
The authors thank to seminar and conference participants, and in particular Ignace De Vos, David Edgerton, Per Johansson, Rolf Larsson, Johan Lyhagen, Serena Ng, Simon Reese, Lorenzo Trapani, David Harvey, Povilas Lastauskas, Greg Franguridi, an associate editor, and two anonymous referees for many valuable comments and suggestions. Westerlund thank to the Knut and Alice Wallenberg Foundation for financial support through a Wallenberg Academy Fellowship.
Supplementary Materials
The supplement materials include proofs and additional results.
References
- Ahn, S. C., and Horenstein, A. R. (2013), “Eigenvalue Ratio Test for the Number of Factors,” Econometrica, 81, 1203–1227.
- Ando, T., and Bai, J. (2016), “Panel Data Models With Grouped Factor Structure Under Unknown Group Membership,” Journal of Applied Econometrics, 136, 163–191. DOI: 10.1002/jae.2467.
- Ando, T., and Bai, J. (2017), “Clustering Huge Number of Financial Time Series: A Panel Data Approach With High-Dimensional Predictors and Factor Structures,” Journal of the American Statistical Association, 112, 1182–1198. DOI: 10.1080/01621459.2016.1195743.
- Bai, J., and Ng, S. (2002), “Determining the Number of Factors in Approximate Factor Models,” Econometrica, 70, 191–221. DOI: 10.1111/1468-0262.00273.
- Bai, J., and Ng, S. (2006), “Confidence Intervals for Diffusion Index Forecasts and Inference for Factor-Augmented Regressions,” Econometrica, 74, 1133–1150.
- Bai, J., and Ng, S. (2007), “Determining the Number of Primitive Shocks in Factor Models,” Journal of Business & Economic Statistics, 25, 52–60. DOI: 10.1198/073500106000000413.
- Bai, J., and Ng, S. (2008), “Forecasting Economic Time Series Using Targeted Predictors,” Journal of Econometrics, 146, 304–317. DOI: 10.1016/j.jeconom.2008.08.010.
- Bai, J., and Ng, S. (2009), “Boosting Diffusion Indices,” Journal of Applied Econometrics, 24, 607–629. DOI: 10.1002/jae.1063.
- Bai, J., and Ng, S. (2019), “Rank Regularized Estimation of Approximate Factor Models,” Journal of Econometrics, 212, 78–96.
- Bonhomme, S., and Manresa, E. (2015), “Dynamic Factors in the Presence of Blocks,” Econometrica, 84, 1147–1184. DOI: 10.3982/ECTA11319.
- Breitung, J., and Eickmeier, S. (2011), “Testing for Structural Breaks in Dynamic Factor Models,” Journal of Econometrics, 163, 71–84. DOI: 10.1016/j.jeconom.2010.11.008.
- Camba-Mendez, G., and Kapetanios, G. (2005), “Forecasting Euro Area Inflation Using Dynamic Factor Measures of Underlying Inflation,” Journal of Forecasting, 24, 491–503. DOI: 10.1002/for.964.
- Castle, J. L., Clements, M. P., and Hendry, D. F. (2013), “Forecasting by Factors, by Variables, by Both or Neither?” Journal of Econometrics, 177, 305–319. DOI: 10.1016/j.jeconom.2013.04.015.
- Cavaliere, G., Rahbek, A., and Taylor, A. M. R. (2010), “Cointegration Rank Testing Under Conditional Heteroskedasticity,” Econometric Theory, 26, 1719–1760. DOI: 10.1017/S0266466609990776.
- Chen, L., Dolado, J. J., and Gonzalo, J. (2014), “Detecting Big Structural Breaks in Large Factor Models,” Journal of Econometrics, 180, 30–48. DOI: 10.1016/j.jeconom.2014.01.006.
- Cheng, X., and Hansen, B. E. (2015), “Forecasting With Factor-Augmented Regression: A Frequentist Model Averaging Approach,” Journal of Econometrics, 86, 280–293. DOI: 10.1016/j.jeconom.2015.02.010.
- Clark, T. E., and McCracken, M. W. (2001), “Tests of Equal Forecast Accuracy and Encompassing for Nested Models,” Journal of Econometrics, 105, 85–110. DOI: 10.1016/S0304-4076(01)00071-9.
- Clark, T. E., and McCracken, M. W. (2005), “The Power of Tests of Predictive Ability in the Presence of Structural Breaks,” Journal of Econometrics 124, 1–31.
- Clark, T. E., and McCracken, M. W. (2013), “Chapter 20 - Advances in Forecast Evaluation,” in Handbook of Economic Forecasting, eds. G. Elliott and A. Timmermann, Elsevier, pp. 1107–1201.
- Corradi, V., Swanson, N., and Olivetti, C. (2001), “Predictive Ability With Cointegrated Variables,” Journal of Econometrics, 104, 315–358. DOI: 10.1016/S0304-4076(01)00086-0.
- De Mol, C., Giannone, D., and Reichlin, L. (2008), “Forecasting Using a Large Number of Predictors: Is Bayesian Shrinkage a Valid Alternative to Principal Components?” Journal of Econometrics, 146, 318–328. DOI: 10.1016/j.jeconom.2008.08.011.
- Eickmeier, S., and Ziegler, C. (2008), “How Successful Are Dynamic Factor Models at Forecasting Output and Inflation? A Meta-Analytic Approach,” Journal of Forecasting, 27, 237–265. DOI: 10.1002/for.1056.
- Ghosh, B. (2002), “Probability Inequalities Related to Markov’s Theorem,” The American Statistician, 56, 186–190. DOI: 10.1198/000313002119.
- Giacomini, R., and Rossi, B. (2009), “Detecting and Predicting Forecast Breakdowns,” Review of Economic Studies 76, 669–705. DOI: 10.1111/j.1467-937X.2009.00545.x.
- Giacomini, R., and White, H. (2006), “Tests of Conditional Predictive Ability,” Econometrica, 74, 1545–1578. DOI: 10.1111/j.1468-0262.2006.00718.x.
- Gonçalves, S., McCracken, M. W., and Perron, B. (2017), “Tests of Equal Accuracy for Nested Models With Estimated Factors,” Journal of Econometrics, 198, 231–252. DOI: 10.1016/j.jeconom.2017.01.004.
- Gonçalves, S., and Perron, B. (2020), “Bootstrapping Factor Models With Cross Sectional Dependence,” Journal of Econometrics, 218, 476–495. DOI: 10.1016/j.jeconom.2020.04.026.
- Gorodnichenko, Y., and Ng, S. (2017), “Level and Volatility Factors in Macroeconomic Data,” Journal of Monetary Economics, 91, 52–68. DOI: 10.1016/j.jmoneco.2017.09.004.
- Grover, S., and McCracken, M. W. (2014), “Factor-Based Prediction of Industry-Wide Bank Stress,” Federal Reserve Bank of St. Louis Review, 96, 173–194. DOI: 10.20955/r.96.173-194.
- Hallin, M., and Liška, R. (2011), “Dynamic Factors in the Presence of Blocks,” Journal of Econometrics, 163, 29–41. DOI: 10.1016/j.jeconom.2010.11.004.
- Inoue, A., and Kilian, L. (2005), “In-Sample or Out-of-Sample Tests of Predictability: Which One Should We Use?” Econometric Reviews, 23, 371–402. DOI: 10.1081/ETC-200040785.
- Karabiyik, H., and Westerlund, J. (2021), “Forecasting Using Cross-Section Augmented Time Series Regressions,” Econometrics Journal.
- Kim, H. H., and Swanson, N. R. (2014), “Forecasting Financial and Macroeconomic Variables Using Data Reduction Methods: New Empirical Evidence,” Journal of Econometrics, 78, 352–367. DOI: 10.1016/j.jeconom.2013.08.033.
- Leeb, H., and Pötscher, B. (2005), “Model Selection and Inference: Facts and Fiction,” Econometric Theory, 21, 21–59. DOI: 10.1017/S0266466605050036.
- Ludvigson, S., and Ng, S. (2009), “Macro Factors in Bond Risk Premia,” The Review of Financial Studies, 22, 5027–5067. DOI: 10.1093/rfs/hhp081.
- Ludvigson, S., and Ng, S. (2011), “A Factor Analysis of Bond Risk Premia,” in Handbook of Empirical Economics and Finance, eds. D. Gilles and A. Ullah. Chapman and Hall, pp. 313–372.
- McCracken, M. W. (2007), “Asymptotics for Out of Sample Tests of Granger Causality,” Journal of Econometrics, 140, 719–752. DOI: 10.1016/j.jeconom.2006.07.020.
- McCracken, M. W., and Ng, S. (2016), “FRED-MD: A Monthly Database for Macroeconomic Research,” Journal of Business & Economic Statistics, 34, 574–589.
- Moench, E., Ng, S., and Potter, S. (2013), “Dynamic Hierarchical Factor Model,” The Review of Economics and Statistics, 95, 1811–1817. DOI: 10.1162/REST_a_00359.
- Pesaran, M. H. (2006), “Estimation and Inference in Large Heterogeneous Panels With a Multifactor Error Structure,” Econometrica, 74, 967–1012. DOI: 10.1111/j.1468-0262.2006.00692.x.
- Pesaran, M. H., and Tosetti, E. (2011), “Large Panels With Common Factors and Spatial Correlation,” Journal of Econometrics, 161, 182–202. DOI: 10.1016/j.jeconom.2010.12.003.
- Schumacher, C. (2007), “Forecasting German GDP Using Alternative Factor Models Based on Large Dataset,” Journal of Forecasting, 26, 271–302. DOI: 10.1002/for.1026.
- Stock, J. and Watson, M. (1999), “Forecasting Inflation,” Journal of Monetary Economics, 44, 293–335. DOI: 10.1016/S0304-3932(99)00027-6.
- Stock, J. and Watson, M. (2002a), “Forecasting Using Principal Components From a Large Number of Predictors,” Journal of the American Statistical Association, 97, 1167–1179.
- Stock, J. and Watson, M. (2002b), “Macroeconomic Forecasting Using Diffusion Indexes,” Journal of Business & Economic Statistics, 20, 147–162.
- Stock, J. and Watson, M. (2004), “Combination Forecasts of Output Growth in a Seven-Country Data Set,” Journal of Forecasting, 23, 405–430.
- Stock, J. and Watson, M. (2005), “Implications of Dynamic Factor Models for VAR Analysis,” Technical report, National Bureau of Economic Research.
- Stock, J. and Watson, M. (2009), “Forecasting in Dynamic Factor Models Subject to Structural Instability,” in The Methodology and Practice of Econometrics: Festschrift in Honor of David F. Hendry, eds. N. Shephard and J. Castle. Oxford: Oxford University Press, pp. 1–57.