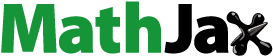
Abstract
This rejoinder addresses the discussants’ specific comments on the article “Narrative Restrictions and Proxies” (Section 2) as well as more general comments on the approach to robust Bayesian inference that we have proposed in previous work (Section 1).
1 General Comments on Robust Bayesian Inference
1.1 Is Posterior Sensitivity Quantitatively Important?
A key motivation underlying the robust Bayesian approach to inference is that, in set-identified models, a component of the prior is never updated and so may affect posterior inference. Specifically, the prior for the reduced-form parameter is updated, whereas the conditional prior for the orthonormal matrix Q that maps reduced-form errors into structural shocks (given
) is not updated. In their discussions, Kilian and Rubio-Ramírez argue that this posterior sensitivity is often not quantitatively important in practice, citing work by Inoue and Kilian (Citation2022). We disagree with this claim, for two main reasons.
First, the results in Inoue and Kilian (Citation2022) do not prove that posterior sensitivity is not important. Inoue and Kilian (Citation2022) compare the (joint) prior and posterior distributions of the impulse responses. When these distributions differ substantively, they interpret this as evidence that the prior is not driving posterior inference. However, since the impulse responses are functions of both and Q, differences between the prior and posterior for the impulse responses can simply reflect the fact that the prior for
has been updated. Assessing whether the conditional prior for Q is driving posterior inference requires assessing whether changes in this prior change the posterior; that is, in set-identified models, prior informativeness can only be gauged by assessing posterior sensitivity to the choice of prior rather than by comparing prior and posterior distributions. The robust Bayesian approach to inference in Giacomini and Kitagawa (Citation2021) provides a means of assessing global sensitivity to the choice of conditional prior for Q and can thus assist in assessing its informativeness (other formal approaches to assessing posterior sensitivity are also discussed in Giacomini et al. Citation2021b). Using these methods, the empirical applications in Giacomini and Kitagawa (Citation2021), Giacomini, Kitagawa, and Read (Citation2021a, Citation2021b, Citation2022) and Read (forthcoming) demonstrate cases where posterior conclusions are clearly sensitive to the choice of conditional prior.
Second, we agree with the more general point in Inoue and Kilian (Citation2022) that in some cases the identified set can be narrow and thus standard Bayesian inference will deliver similar results to robust Bayesian inference. However, it is difficult to know whether this will be the case in any particular application without knowing how wide the underlying identified set is; that is, in order to know whether the identifying restrictions are sufficiently informative to make the choice of conditional prior quantitatively unimportant, it is necessary to apply robust Bayesian tools (or to otherwise characterize the identified set).
1.2 Can one Test Joint Hypotheses?
Inoue and Kilian (Citation2016, forthcoming) argue that users of SVARs are often interested in hypotheses that involve multiple parameters, in which case pointwise confidence or credible intervals are not informative. In his discussion, Kilian suggests that it would be useful to extend the robust Bayesian approach to inference proposed in Giacomini and Kitagawa (Citation2021) to allow researchers to conduct joint inference in a way that is robust to the choice of prior for Q. This is a useful suggestion, and we explain how to do this below.
Assume that one is interested in assessing the posterior evidence for a hypothesis that involves multiple impulse responses. For example,
could state that the impulse response of output to a monetary policy shock is larger at the two-year horizon than at the one-year horizon. To gauge the strength of the evidence for
in the standard (single-prior) Bayesian setting, a researcher could simply report the posterior probability assigned to
by computing the proportion of draws from the posterior where
is true. The analogue of this in the robust Bayesian setting would be to report the posterior lower and upper probabilities of
. If the posterior lower (upper) probability of the hypothesis is x, the hypothesis receives at least (most) posterior probability x given any conditional prior for Q within the class of conditional priors under consideration. The posterior lower and upper probabilities provide a natural means for researchers to conduct prior-robust posterior inference about hypotheses that depend on multiple impulse responses.
Let be an indicator variable equal to one when
is true given the parameters
and equal to zero otherwise.Footnote1 Given a value of
, the identified set for
, which we denote by
, is either {0} (when
is never true for any value of Q within its identified set), {1} (when
is always true for any value of Q within its identified set) or {0, 1} (when
is only sometimes true). The posterior lower and upper probabilities assigned to
can be expressed in terms of the posterior for
(
).Footnote2 The posterior lower probability,
, is the posterior probability (with respect to
) that
is equal to {1}:
(1)
(1) and the posterior upper probability,
, is the posterior probability that
intersects {1}:
(2)
(2)
To assist applied researchers, we describe a general algorithm for computing posterior lower and upper probabilities in an SVAR when the hypothesis of interest potentially relates to multiple impulse responses.
Algorithm 1:
Let be an indicator variable equal to one when the hypothesis
is true given the parameters
and equal to zero otherwise. Let
be M draws of
from
such that the identified set is nonempty.
Step 1: For each m, obtain K draws of Q,
, from a uniform distribution over its identified set and compute
for
.Footnote3
Step 2: Let
if
and
otherwise. Let
if
and
otherwise.
Step 3: Approximate the posterior lower and upper probabilities assigned to
by:
To illustrate, we consider the application in GKR, which estimates the effects of a monetary policy shock in the United States by applying narrative and sign restrictions, as in Antolín-Díaz and Rubio-Ramírez (Citation2018). We consider the hypothesis that the impulse response of output is lower two years after a positive shock than it is after one year. Under the standard approach to Bayesian inference, the posterior probability of this hypothesis is 91.5%, so there appears to be reasonably strong evidence that output falls by more after two years than after one year. Under the robust Bayesian approach to inference, the posterior lower probability is 21.4% and the posterior upper probability is 100%. The conclusion that output falls by more at the two-year horizon than at the one-year horizon is therefore sensitive to the choice of conditional prior for Q.Footnote4
1.3 Is the “Plausibility of Restrictions” Statistic Meaningful?
In a set-identified SVAR, the identified set for Q may be empty. In this case, the imposed identifying restrictions are inconsistent with the joint distribution of the data, as summarized by the reduced-form parameters. Giacomini and Kitagawa (Citation2021) therefore propose reporting the posterior probability that the identified set is nonempty as a measure of the “plausibility” of the identifying restrictions. In his discussion, Kilian questions the validity of this statistic. The main thrust of his argument is that adding restrictions will always (weakly) decrease the posterior plausibility, so the statistic will penalize the use of additional restrictions even when these have a valid economic motivation.
Conceptually, the statistic can be motivated by the “Law of Decreasing Credibility” (Manski Citation2003): if there is a tradeoff between the strength of the assumptions and their credibility, reporting the posterior plausibility statistic can help communicate with an audience that may place a different degree of credibility on the imposed assumptions. In practice, we acknowledge that there are some nuances associated with the interpretation of this statistic, which are not expounded in Giacomini and Kitagawa (Citation2021). We discuss them below.
First, consider a thought experiment where the sample size diverges and the posterior for collapses toward its true value,
. For values of
sufficiently close to
and so long as the identifying restrictions are correct, the identified set will be nonempty and the posterior plausibility will be one. The posterior plausibility of the restrictions should therefore converge to one asymptotically when the identifying restrictions are correct. In contrast, when the identifying restrictions are refutable and incorrect, the identified set may be empty for values of
in a neighborhood around
, and the posterior plausibility will not necessarily converge to one. In this sense, the posterior plausibility may be informative about whether the identifying restrictions are true or not. However, the interpretation of this statistic is muddied in finite samples. To illustrate, consider the case where the posterior for
assigns probability mass to values of
far from
(e.g., due to the prior being concentrated far from
). In this case, it is possible that the identified set could be empty with high posterior probability despite the identifying restrictions being correct. Care should therefore be taken when interpreting the posterior plausibility, particularly when the sample is small and/or when the posterior for
is dominated by the prior.
When questioning the usefulness of the posterior plausibility statistic, Kilian also notes that the point of identifying restrictions (and narrative restrictions in particular) is to restrict the reduced-form parameter space of the model and hence the identified set. We think it is important to clarify this point. The identified set is the set of structural parameters that share the same value of the reduced-form parameters. Imposing identifying restrictions (weakly) tightens this set. However, it does not necessarily restrict the reduced-form parameter space. For example, when there are r zero restrictions, s sign restrictions and the restrictions constrain a single column of Q, the identified set is never empty so long as , where n is the dimension of the SVAR. So long as this condition is satisfied, adding identifying restrictions will not constrain the reduced-form parameter space (because the identified set is never empty), but it will (weakly) narrow the identified set. When
, the identifying restrictions may yield an empty identified set and imposing additional restrictions may, but will not necessarily, restrict the reduced-form parameter space.
1.4 Comments on the Class of Priors
Given a likelihood function, the robust Bayesian approach in Giacomini and Kitagawa (Citation2021) takes as inputs a prior for and a class of conditional priors for Q given
. As Kilian notes, one can summarize this class of priors analogously to how one can summarize the class of posteriors (e.g., by computing sets of prior means or quantiles). However, Kilian argues that these statistics do not lend themselves to judging the “economic content” of the class of priors. We disagree with this, on the basis that one can always compute the prior lower and upper probabilities for any hypothesis of interest, including for hypotheses relating to multiple impulse responses; in Algorithm 1, simply replace the draws of
from the posterior with draws of
from the prior (assuming this is proper).
More fundamentally, we question the value of assessing the implicit prior (or class of priors) for the impulse responses under the conventional approach to Bayesian inference in set-identified SVARs. Under the conventional approach, researchers impose a prior on the reduced-form parameters (e.g., diffuse normal-inverse-Wishart or Minnesota), while direct prior information about the structural parameters of interest (e.g., impulse responses) is imposed via the identifying restrictions. This approach therefore operates under the assumption that the “economic content” of the researcher’s prior information about the structural parameters is exhausted through the imposition of identifying restrictions. Accordingly, we see little compelling reason for researchers to examine the implicit prior (or class of priors) for the structural parameters; if the researcher had access to additional prior information about these parameters, it would make sense to impose it using additional identifying restrictions or via a prior for the (structural) parameters of interest, as discussed in Baumeister and Hamilton (Citation2015, Citation2022).Footnote5
Related to this, we have previously claimed that the uniform prior for Q is typically chosen because it is computationally convenient, in the sense that there are fairly simple algorithms that can be used to obtain draws from this distribution subject to sign and/or zero restrictions (e.g., Rubio-Ramírez et al. Citation2010; Arias et al. Citation2018). In his discussion, Rubio-Ramírez argues that this prior is in fact chosen because the prior assigns equal prior density to observationally equivalent models. While this is true when the model is parameterized in its orthogonal reduced form, it is not necessarily true in other parametrizations, since transformations or marginalizations to the parameters of interest cannot preserve uniformity of the distribution.Footnote6 Regardless of the motivation for imposing the uniform prior for Q, the fact remains that this prior is never updated, is typically not chosen to reflect actual prior information (outside of restrictions on its support reflecting the identifying assumptions) and can be crucial in driving posterior inference. See also Baumeister and Hamilton (Citation2022) for further discussion on this topic.
2 Comments on “Narrative Restrictions and Proxies”
2.1 Merits of Narrative Proxies over Narrative Restrictions
In his discussion, Plagborg–Møller draws out some benefits of using the narrative proxy (NP) approach to identification given information about shock signs over the approach that imposes this information using narrative restrictions (NR). One suggested benefit is that the NP approach allows for the shock signs to be measured with error, whereas the NR approach assumes that the shock sign is known with certainty. As an example, he asks whether we really know that there was a positive monetary policy shock in the United States in October 1979 (as imposed in Antolín-Díaz and Rubio-Ramírez Citation2018) rather than, say, September 1979. One point worth noting is that the NR approach allows for this type of uncertainty (i.e., about the timing of the narrative information); for example, the researcher could impose that the monetary policy shock was positive in October or September 1979 by rejecting values of Q such that the shock was negative in both periods. Of course, this still assumes that it is known with certainty that the shock was positive in one of these periods, so the point about knowing the narrative information with certainty still applies at some level.
Plagborg–Møller also explains that the proxy approach allows for nonclassical measurement error; for example, if the sign of the structural shock is only revealed in periods where the shock is relatively large in magnitude, the NP approach remains valid. In contrast, the NR approach (implicitly) assumes that the information about the shock signs arrives independently of other information about the shocks. We acknowledge this benefit of the NP approach and suggest that it would be useful for researchers to think more about the mechanism that generates the information that they use to impose NR—what we like to call the “narrative generating mechanism.” However, we would also argue that—at least to some extent—this idea is already embedded in the way that most researchers impose NR. For example, Antolín-Díaz and Rubio-Ramírez (Citation2018) only impose shock-sign restrictions alongside restrictions on the historical decomposition (e.g., in October 1979 the monetary policy shock was positive and the “overwhelming” contributor to the observed change in the federal funds rate). Nevertheless, this still implicitly assumes that the joint narrative information about the shock sign and historical decomposition arrives independently of other information about the shocks.
It would be useful for further research to explore the implications of ignoring additional narrative information (e.g., about the size or contribution of shocks) when using the NP approach based on shock signs relative to incorporating the full set of narrative information using the NR approach. We believe that Plagborg-Møller’s suggested permutation-based approach to inference will be a useful tool when making such a comparison.
2.2 Combining Narrative and Proxy-based Restrictions
Assume we have access to a valid proxy for the last structural shock in some SVAR system. The proxy can be used to point-identify the impulse responses to the shock as well as the coefficients in the structural equation corresponding to that shock.Footnote7 Given the realizations of the data, the proxy can therefore be used to recover the posterior distribution of the shock in each period.
Rubio-Ramírez notes that, when the proxy is based on information about shock signs, there is no guarantee that the posterior distribution of the shock in each period will be consistent with the information about the sign of the shock used to construct the proxy. For example, even in a period where the shock was known to be positive, the posterior distribution for the shock in this period may place positive posterior probability on negative values. Rubio-Ramírez therefore makes the interesting suggestion that one could impose shock-sign narrative restrictions in addition to the proxy exogeneity restrictions so that the posterior distribution is fully consistent with the available narrative information, which may help to more precisely estimate the parameters of interest. Related to this, Plagborg–Møller suggests that it would be useful to investigate the gains from combining the NP approach with other types of identifying information. Combining NR with proxy-based restrictions should be straightforward using either conventional approaches (i.e., by combining the approaches in Arias et al. Citation2021; Antolín-Díaz and Rubio-Ramírez Citation2018) or robust Bayesian approaches (i.e., by combining the approaches in Giacomini et al. Citation2022, Citation2021a).
An open question is whether the combination of narrative and proxy-based restrictions would lead to more informative inference. When there is a single proxy for a single shock, so the proxy variable point-identifies the impulse responses to the shock, the imposition of additional NR may restrict the reduced-form parameter space; this will be the case whenever the posterior distribution of the shocks disagrees with the information about the shock signs with positive posterior probability. When there are multiple proxies for multiple shocks, so the impulse responses to the shocks are set-identified in the absence of additional zero restrictions, the imposition of additional NR may tighten the identified set and/or restrict the reduced-form parameter space.
Acknowledgments
The views in this article are the authors’ and do not reflect the views of the Federal Reserve Bank of Chicago, the Federal Reserve System or the Reserve Bank of Australia. We thank our discussants—Lutz Kilian, Mikkel Plagborg-Møller and Juan Rubio-Ramírez—for their insightful discussions of our article.
Additional information
Funding
Notes
1 The indicator function could also be a function of the data separately from the reduced-form parameters, such as when is a hypothesis about the values of structural shocks or the historical decomposition in specific periods. We leave this potential dependence on the data implicit.
2 Theorem 1 of Giacomini and Kitagawa (Citation2021) expresses the posterior lower and upper probabilities that some subvector or transformation, , of the structural parameters lies within a region D in terms of the posterior for
. Replacing
in their Theorem 1 with
and D with {1} yields the expressions in (1) and (2).
3 Methods for checking whether the identified set for Q is empty and for drawing from a uniform distribution over the identified set for Q are described in Giacomini and Kitagawa (Citation2021) and Giacomini et al. (Citation2021b). If multiple hypotheses are of interest, the same draws of Q can be used to evaluate a different indicator function corresponding to each hypothesis.
4 The results are based on 1000 draws of from its posterior such that the conditional identified set is nonempty and 100,000 draws of Q at each draw of
.
5 When specifying a prior for the structural parameters, it remains the case that a component of the prior will not be updated, so it may still be desirable to use robust Bayesian methods to assess posterior sensitivity to the choice of prior. When partially credible prior information about the structural parameters is available, the approach in Giacomini et al. (Citation2019) can be used to assess posterior sensitivity to perturbations of the prior within some neighborhood around the “benchmark” prior.
6 As a stark example, consider imposing a point-mass prior for a single value of and a conditionally uniform prior for Q given
. The implied prior for the impulse responses will in general not be uniform, despite the fact that all impulse responses with positive prior density are observationally equivalent, since they share the same value of
.
7 Baumeister and Hamilton (Citation2022) discuss this point. For alternative intuition, consider the model’s orthogonal reduced-form. Given a valid proxy variable for the last structural shock, the last column of Q is point-identified (e.g., Arias et al. Citation2021; Giacomini et al. Citation2022). Since . the last row of
is a function of
and the last column of Q, so the coefficients in the last structural equation are also point-identified.
References
- Antolín-Díaz, J., and Rubio-Ramírez, J. F. (2018), “Narrative Sign Restrictions for SVARs,” American Economic Review, 108, 2802–2829. DOI: 10.1257/aer.20161852.
- Arias, J. E., Rubio-Ramírez, J. F., and Waggoner, D. F. (2018), “Inference Based on Structural Vector Autoregressions Identified with Sign and Zero Restrictions: Theory and Applications,” Econometrica, 86, 685–720. DOI: 10.3982/ECTA14468.
- Arias, J. E., Rubio-Ramírez, J. F., and Waggoner, D. F. (2021), “Inference in Bayesian Proxy SVARs,” Journal of Econometrics, 225, 88–106.
- Baumeister, C., and Hamilton, J. D. (2015), “Sign Restrictions, Structural Vector Autoregressions, and Useful Prior Information,” Econometrica, 83, 1963–1999. DOI: 10.3982/ECTA12356.
- Baumeister, C., and Hamilton, J. D. (2022), “Advances in Using Vector Autoregressions to Estimate Structural Magnitudes,” unpublished manuscript.
- Giacomini, R., and Kitagawa, T. (2021), “Robust Bayesian Inference for Set-identified Models,” Econometrica, 89, 1519–1556. DOI: 10.3982/ECTA16773.
- Giacomini, R., Kitagawa, T., and Read, M. (2021a), “Identification and Inference Under Narrative Restrictions,” arXiv: 2102.06456 [econ.EM].
- Giacomini, R., Kitagawa, T., and Read, M. (2021b), “Robust Bayesian Analysis for Econometrics,” Centre for Economic Policy Research Discussion Paper DP16488.
- Giacomini, R., Kitagawa, T., and Read, M. (2022), “Robust Bayesian Inference in Proxy SVARs,” Journal of Econometrics, 228, 107–126.
- Giacomini, R., Kitagawa, T., and Uhlig, H. (2019), “Estimation Under Ambiguity,” cemmap Working paper CWP24/19.
- Inoue, A., and Kilian, L. (2016), “Joint Confidence Sets for Structural Impulse Responses,” Journal of Econometrics, 192, 421–432. DOI: 10.1016/j.jeconom.2016.02.008.
- Inoue, A., and Kilian, L. (2022), “The Role of the Prior in Estimating VAR Models with Sign Restrictions,” unpublished manuscript.
- Inoue, A., and Kilian, L. (forthcoming), “Joint Bayesian Inference about Impulse Responses in VAR Models,” Journal of Econometrics.
- Manski, C. F. (2003), Partial Identification of Probability Distributions, New York: Springer-Verlag.
- Read, M. (forthcoming), “The Unit-effect Normalisation in Set-identified Structural Vector Autoregressions,” Reserve Bank of Australia Research Discussion Paper.
- Rubio-Ramírez, J. F., Waggoner, D. F., and Zha, T. (2010), “Structural Vector Autoregressions: Theory of Identification and Algorithms for Inference,” The Review of Economic Studies, 77, 665–696. DOI: 10.1111/j.1467-937X.2009.00578.x.