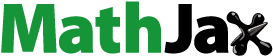
Abstract
A huge literature on modeling cross-sectional dependence in panels has been developed using interactive effects (IE). One area of contention is the hypothesis concerned with whether the regressors and factor loadings are correlated or not. Under the null hypothesis that they are conditionally independent, we can still apply the consistent and robust two-way fixed effects estimator. As an important specification test we develop an LM test for both static and dynamic panels with IE. Simulation results confirm the satisfactory performance of the LM test in small samples. We demonstrate its usefulness with an application to a total of 22 datasets, including static panels with a small T and dynamic panels with serially correlated factors, providing convincing evidence that the null hypothesis is not rejected in
1 Introduction
The pervasive evidence in favor of the presence of strong cross-sectional dependence (CSD) in panels (e.g., Pesaran 2015) has prompted the development of rigorous econometric methodology for explicitly modeling CSD, mainly through multiplicative interactive effects (IE). Two leading approaches have received considerable attention. The first approach proposed by Bai (Citation2009), on the basis of the principal component (PC) estimation, estimates the factors and the main parameters in an iterative manner. This has been extended by Moon and Weidner (Citation2015), Fernandez-Val and Weidner (Citation2016), and Charbonneau (Citation2017). The second approach, referred to as the common correlated effects (CCE) estimator advanced by Pesaran (Citation2006), treats factors as nuisance terms by removing their effects through proxying them by the cross-section averages of the dependent and independent variables. A few extensions have been developed by Kapetanios, Pesaran, and Yamagata (Citation2011), Chudik and Pesaran (Citation2015), Westerlund and Urbain (2015), and Juodis (Citation2022). See Chudik and Pesaran (Citation2015) for an extended survey.
The conventional wisdom is that the two-way fixed effects (FE) estimator would be inconsistent in the presence of IE, due to ignoring the correlation between the regressors and factors/loadings (e.g., Bai Citation2009). However, if the regressors are uncorrelated with the factor loadings (even if still correlated with factors), then the two-way FE estimator is still unbiased. This has been noted earlier by Coakley, Fuertes, and Smith (Citation2006), Sarafidis and Wansbeek (Citation2012), and Westerlund (Citation2019a). Kapetanios, Serlenga, and Shin (Citation2023) formally establish that the FE estimator is consistent and asymptotically normally distributed under this situation while proposing a robust heteroscedasticity and autocorrelation consistent (HAC) variance estimator to deal with the presence of IE in error components. In what follows, we refer to this estimator as the “robust FE estimator”.
A number of specification tests have been proposed for testing the presence of CSD or IE in panels, see Pesaran (2015), Sarafidis, Yamagata, and Robertson (Citation2009), Bai (Citation2009), Castagnetti, Rossi, and Trapani (Citation2015), and Westerlund (2019b). As discussed above, however, the rejection of the null hypothesis by these tests does not always imply that the FE estimator is inconsistent. Surprisingly, the literature has been, in general, silent on investigating whether regressors are uncorrelated with factor loadings. This is the crucial hypothesis to be tested because if it is not rejected, then we can still apply the robust FE estimator as well as the PC estimator, though the consistency of the latter requires that the estimated number of factors is equal to or larger than the true one. Furthermore, under this situation, the FE estimator can be easily applied to static panels with a small number of time periods or dynamic panels with serially correlated factors.
In order to fill this gap, Kapetanios, Serlenga, and Shin (Citation2023) developed a Hausman test that determines whether the regressors and factor loadings are correlated or not. It focuses on testing the null hypothesis that the difference between the FE and PC estimators is zero in population. This procedure is intuitive since we care about the cost of using the potentially inaccurate FE estimator. Within the context of the factor augmented panel data model, where both the dependent variable and regressors share overlapping sets of factors, this is equivalent to testing that regressors and loadings are conditionally independent. However, such a Hausman test requires the use of a well-specified PC estimator for its implementation (e.g., the Stata commands by Kripfganz and Sarafidis Citation2021), suggesting that it may still be cumbersome from a practical perspective.
As a main contribution in this article we propose an LM test that does not require the use of the PC estimator at all. After applying the two-way within transformation to the panel data model with IE, we only use the FE estimator to obtain the residuals and construct an LM test statistic for determining the validity of the orthogonal moment conditions between transformed regressors and residuals under the null hypothesis. Further, by a portmanteau characterization of the LM test, we propose the use of the first principal component of the regressors, in constructing the LM test statistic. We show that this approach can provide a valid test that is well behaved under the null and the alternative hypotheses (see Theorem 1 and the supporting simulation evidence in Section S4.4 in the online supplement). Importantly, we also establish that the validity of the LM test does not require consistent estimation of individual slope parameters even for small T.
Further, Kapetanios, Serlenga, and Shin (Citation2023) consider only the static panel data model with large T, while the current paper extends the LM test to the dynamic panel data model as well as the static panel data model with small T. In addition, we note that the performance of the Hausman test proposed by Kapetanios, Serlenga, and Shin (Citation2023) is very sensitive to model misspecification.
Next, for a dynamic panel data model with IE and serially correlated factors, we suggest the use of an autoregressive distributed lag (ARDL) approximation in constructing the LM test statistic. We establish that the corresponding LM test follows the distribution under the null hypothesis whereas it diverges under the alternative hypothesis.
It is important to emphasize that our approach is designed to develop a crucial specification test that is missing in the literature rather than provide a model building strategy, analogous to conducting a serial correlation test. The proposed LM test then complements the popular CD test by Pesaran (2015) and the refined CD* test recently advanced by Pesaran and Xie (2022). Given the pervasive evidence in favor of strong CSD, we suggest applying the LM test to determine whether the form of IE invalidates the consistency of the FE estimator or not. However, it is worth emphasizing that both CD and CD* tests are not always satisfactory. The CD test fails to reject the null hypothesis of weak error CSD when loadings have zero means, implying that it displays very poor power when applied to cross-sectionally demeaned data. Furthermore, Juodis and Reese (Citation2021) show that the application of the CD test to regression residuals obtained from models with IE, involves a bias, resulting in erroneous rejections under the null hypothesis (e.g., Mastromarco, Serlenga, and Shin Citation2016). Thus, the CD* test is developed to correct the asymptotic bias of the CD test using the estimates of factor loadings and error variances, though its performance crucially relies upon a tuning parameter (the optimal number of factors). On the contrary, we can establish the validity of the LM test under weaker conditions, irrespective of the presence of CSD or selecting the correct number of factors, as a tool for testing the consistency of the FE estimator for the panel data models with IE.
Via Monte Carlo experiments, we find that the finite sample performance of the LM test is satisfactory even under pronounced parameter heterogeneity, serial and weak cross-sectional error correlation. Furthermore, its performance remains satisfactory for static panels with a small number of time periods and for dynamic panels with serially correlated factors. The LM test is also robust to model misspecification, unlike the Hausman test proposed by Kapetanios, Serlenga, and Shin (Citation2023) that tends to display severe size distortions in the presence of neglected regressors.
We demonstrate the usefulness of the LM test with an application to a total of 22 datasets employed in the literature. At the 5% (10%) significance the LM test does not reject the null hypothesis in 12 (10) out of 14 datasets under the static panels whilst the null is marginally rejected only once out of five datasets under the dynamic framework. Furthermore, the LM test does not reject the null hypothesis for all three static panels with a small T. These results provide convincing evidence that the null hypothesis of conditional independence between factor loadings and the regressors is not rejected for many datasets.
The article proceeds as follows. Section 2 develops the LM test for the null hypothesis of the conditional independence between the regressors and factor loadings for static and dynamic panel data models with IE and presents the asymptotic theory. Section 3 investigates the finite sample performance of the LM test. Section 4 presents empirical evidence. Section 5 offers concluding remarks. Mathematical proofs and additional analytic/simulation results are relegated in the online supplement.
2 The LM Test
Consider a heterogeneous panel data model with interactive effects:
(1)
(1) where yit is the dependent variable of the ith cross-sectional unit in period t,
is the
vector of covariates with
the
vector of parameters, and
is an idiosyncratic error.
is an
vector of unobserved factors and
is an
vector of heterogeneous loadings.
For consistent estimation of , two main approaches have been proposed to account for unobserved factors. The CCE estimator advanced by Pesaran (Citation2006), imposes that
share the same factors,
:
(2)
(2) where
an r × k matrix of heterogeneous loadings and
are idiosyncratic errors. CCE approximates
by the cross-section averages of the dependent and independent variables. The DGP condition in (2) simplifies the analysis considerably, but can be viewed as restrictive (see Remark 4). Next, Bai (Citation2009) allows
to be arbitrarily correlated with both
and
, and proposes an iterative PC approach that estimates the factors/loadings together with
.
A number of specification tests have been proposed to test the presence of CSD or IE in panels. The most popular test is the cross-section dependence (CD) test proposed by Pesaran (2015, 2021) that is increasingly used as an ex-post diagnostic tool. The CD test may be used as a model-selection tool, with a reduction in the absolute value of the CD statistic typically being interpreted as an indication of an improved model specification. Bai (Citation2009) advances a Hausman test for testing the null hypothesis of (two-way) additive fixed effects (i.e., ) against the alternative of the multiplicative IE as
(3)
(3)
Under the null hypothesis, Bai (Citation2009) derives that where
is the variance estimator provided by the two-way FE estimation and
is the variance estimator that accommodates unknown forms of heteroscedasticity and autocorrelation in errors. Westerlund (2019b) proposes an alternative Hausman test, denoted HW, by replacing the PC estimator with the CCE estimator, see Section S2 in the Online Supplement for details.
The conventional wisdom is that if the CD test rejects the null hypothesis of weak error CSD, then the FE estimator is biased/inconsistent due to the endogeneity arising from the correlation between the regressors and unobserved factors/loadings. Notice, however, that if the regressors are conditionally independent from loadings, the two-way FE estimator is still consistent for the panel data model with IE. This has been noted earlier by Coakley, Fuertes, and Smith (Citation2006), Sarafidis and Wansbeek (Citation2012), and Westerlund (Citation2019a). Under this situation, Kapetanios, Serlenga, and Shin (Citation2023) formally establish that the FE estimator is consistent and follows an asymptotic normal distribution and propose the robust FE estimator using the HAC variance estimator to deal with the presence of IE in error components, that is shown to be robust to the presence of heteroscedastic and serially-correlated disturbances as well as parameter heterogeneity.
In empirical applications below we apply both CD test and Bai’s Hausman test to several datasets employed in the literature. Surprisingly, we find the conflicting result that the CD test strongly rejects the null hypothesis of weak error CSD while the HB test rarely rejects the null of (FE) additive-effects in most datasets. A careful inspection reveals that if factor loadings and the regressors are conditionally independent, then the HB test becomes inconsistent. To investigate this issue, we examine the powers of both CD and HB tests for the heterogeneous panel data with IE in Section S2 in the Online Supplement. We consider the two experiments. Under Experiment 1 we generate the loadings to be independent from the regressors while they are correlated under Experiment 2. In both Experiments we maintain that the regressors are correlated with factors. As expected, the CD test results display that the null of weak residual CSD is strongly rejected for all the data generating process (DGP) under both Experiments. Under Experiment 1, however, the HB test does not display any power and its rejection probability is close to and sometimes lower than the nominal size, especially in the presence of serially correlated errors. This is because the FE estimator is still consistent under Experiment 1. The HB test is consistent only under Experiment 2. This confirms the limitation of applying the HB test in practice.
This suggests that the null hypothesis of the conditional independence between the regressors and factor loadings emerges as an influential but underappreciated feature of the panel data model with IE. Surprisingly, the literature has been silent on investigating this important issue. Pesaran (Citation2006) implicitly assumes that the factor loadings in (1) and
in (2), are uncorrelated, see also Sarafidis, Yamagata, and Robertson (Citation2009). Westerlund and Urbain (Citation2013) questions the assumption of uncorrelated factor loadings while Bai (Citation2009) shows via simulations that the CCE estimator is biased when
is correlated with both
and
. In this regard, we consider the null and alternative hypotheses as follows:
(4)
(4)
(5)
(5)
This can be regarded as the important misspecification test because if the null hypothesis (4) is not rejected, then the (robust) two-way FE estimator is still valid to employ in the panel data with IE.
Notice that in (1) can be expressed equally as
(6)
(6) where
,
and
with
and
. Using (6), we rewrite (1) as
(7)
(7)
Applying the two-way within transformation to (7), we obtain:
(8)
(8) where
and
. Similarly for
and
. Under the null hypothesis, (4), it is easily seen by
and assuming independence of
from all other random quantities in the model that
is uncorrelated with the transformed composite error,
, provided that
are strictly exogenous with respect to
because
(see also Section 5 in Hsiao Citation2018). Therefore, under the null hypothesis (4), we obtain the following
vector of moment conditions:
(9)
(9)
The conventional panel data without IE satisfies this condition under the maintained assumption that the regressors are exogenous. Now, our main contribution lies in showing that this moment condition can also be satisfied for the panel data model with IE under our null hypothesis.
We obtain an individual two-way FE estimator of and the mean group (MG) estimator by
(10)
(10)
Notice that is unbiased but inconsistent for
whereas
is
-consistent for
. We have:
. Clearly,
and
. Although
, we still have
. Hence,
is unbiased but inconsistent. Next, we have:
such that the MG estimator is
consistent (see Kapetanios, Serlenga, and Shin Citation2023). We conducted (unreported) simulation exercises, finding that the biases of the individual and MG estimators are mostly negligible. The variance of the MG estimator declines with N, but that of the individual estimator does not decline with T. Conversely, if
and
are correlated, both estimators are biased and inconsistent due to
.
Stacking (8) over t, we have:
(11)
(11) where
and
. Under the moment conditions in (9), it is easily seen that
(12)
(12)
where
. Under H1 we have
, from which we have:
(13)
(13)
Using the difference between (12) and (13), we can construct the LM test statistic as follows:
(14)
(14) whereas the test diverges under H1.
To develop an operational version of the LM test, we replace by the FE residuals given by
(15)
(15)
The corresponding statistic is identical to zero since are orthogonal to
, that is,
. We propose replacing
by a fitted value, denoted
, and obtaining
in a simple manner. We first estimate factors, denoted
, obtained by
times the T × r eigenvectors corresponding to the r largest eigenvalues of the covariance matrix of the regressors,
. Next, by a portmanteau characterization of the LM test, we propose to employ only the first estimated factor corresponding to the largest eigenvalue, though the true number of factors, r is larger than one (see the proof of Theorem 1 and the supporting simulation evidence in Section S4.4 in the Online supplement.) Then, the final version of the LM test is constructed as follows:
(16)
(16) where
with
, and
.
We present Assumption A containing the regularity conditions.
Assumption A.
(i) and
for
and for some
. Let
be the σ-field of all stochastic elements in the panel data model, apart from
. Then,
.
(ii) has finite
moments for some
.
(iii) has finite mean,
and positive definite variance,
. Let
be the σ-field of all stochastic elements apart from
. Then, under the null hypothesis (4), we have:
(17)
(17)
Under the alternative hypothesis (5), , and
is a spatial martingale difference process (see Definition 1 in the online supplement).
(iv) have finite
moments.
and
exist for all
. Furthermore, the elements of
and
have finite
moments for all
and for some
uniformly over i. Finally,
.
Assumption A is much weaker than what is usually found in the factor literature (e.g., Bai Citation2009; Karabiyik, Reese, and Westerlund Citation2017; Cui et al. Citation2023; Cui, Sarafidis, and Yamagata Citation2022), mainly because the LM test requires the PC extraction from regressors only, not the residuals from the main regression, (1). Further, we do not require that the unobserved factors are consistently estimated by PC, thus, reducing the need for restrictive assumptions. This is a substantial relaxation since we only require existence of
moments of
instead of eighth moments, usually imposed in this literature. In Section S.4.3 in the Online Supplement, we show via further simulations that if the higher moments (greater than 3) do not exist, then the FE estimator outperforms the PC estimator in all sample sizes. For simplicity we make a zero conditional expectation assumption for the idiosyncratic errors
, though it can be easily extended to the case with a serial and (weak) cross-sectional correlation at the expense of slightly more convoluted proofs (see Assumption A’ used for the dynamic panel data model in the Online Appendix and the simulation evidence in Section 3). We impose a zero conditional expectation condition in (17) under the null hypothesis, that is weaker than independence of
across i. It is slightly stronger than (4) but makes the theoretical derivations much simpler. The first three parts of Assumption A(iv) are standard identification conditions on the existence of moments and their relevant inverses, and needed to derive the asymptotic distribution of the LM test for fixed T, though it also holds as
. The final part of A(iv) is a regularity condition ensuring no pathological cases of perfect multicollinearity of the regressors. This condition is simply satisfied for the case where (2) holds, if
is positive definite uniformly over i and t, though we do not need to impose this condition for the static model. Finally, we slightly restrict our definition of the null hypothesis in line with the technical proofs. Under the alternative hypothesis, we require that the sum of the expectations of the product of the regressors and the factor components, over panel units, explodes.
We have the main theoretical result in Theorem 1.
Theorem 1.
Consider the static heterogeneous panel data model with IE given by (1). Under Assumption A and under the null hypothesis, (4), as , the LMX test statistic in (16) asymptotically follows the
distribution, where k is the number of regressors. Under the alternative hypothesis, (5), the LMX test is consistent.
Remark 1.
Theorem 1 holds for finite T as well as for . Initially, we place our work within a macroeconometric literature that views an effective estimation of
as a main aim. For large T, it is natural to maintain an assumption that the regressors are correlated with factors,
(representing the common policy or globalization trend) to avoid any omitted variables bias while it remains an important issue to test whether the regressors are correlated with the heterogeneous loadings. Moreover, there is a growing literature in microeconometrics that focuses on estimating causal effects by expanding models using factor components. Examples include estimating causal/counterfactual effects using synthetic controls (e.g., Arkhangelsky et al. Citation2021) or cautioning about the causal interpretation of the two-way FE estimator in difference-in-difference setups (e.g., Athey and Imbens Citation2022). In this regard our specification test can be regarded as a significant contribution in this literature with large N and small T, as it can provide support for the use of consistent and robust FE estimator under the null hypothesis.
Remark 2.
Notice that the LMX test remains valid in the absence of a factor structure in yit. In this case the null hypothesis, (4), makes no sense since there are no loadings. But, we can still entertain the moment condition in (9) under which it is easily seen that the LM test, based on extracting a principal component that is well defined in the absence of a factor structure, follows a distribution (see the simulation evidence in Section 3). Hence, we can establish the validity of the LM test under weak conditions, as a tool for testing the consistency of the two-way FE estimation, irrespective of the presence of IE in the model.
Remark 3.
We may construct the FE residuals using the MG estimator such as . We can still show that the corresponding LM statistic follows a limiting
distribution under the null hypothesis (4), though there are complex technical issues related to establishing consistency of the HAC variance estimator used in constructing the LM statistic. Thus, we focus on the LMX test based on the individual FE estimator given by
. The main advantage of this approach lies in that the validity of the LM test does not require consistent estimation of
for small T. Furthermore, as we employ the individual FE estimator from the transformed regression (8), not the MG estimator, our approach does not need to make a random coefficient assumption about
(say, the
vector of
generated as
, where
is independent across i with
and
), implying that our main results hold irrespective of whether slope parameters are homogeneous or heterogeneous (see the proof of Theorem 1 in the Online Supplement).
Remark 4.
It is important to emphasize that we do not have to impose the DGP condition for in (2) in Theorem 1.
may have no factor or can contain different factors from those in yit. For example, we can consider the general case where yit and
share a subset of common factors
while they are subject to further specific factors,
and
such that
and
(see Monte Carlo evidence in Section 3). The only condition required is A(iii). However, if we consider the dynamic panel data, we need to impose a more specific structure such as (2) (see Assumption A’ in the Online Appendix).
Remark 5.
We can generalize our testing approach through providing links with the monograph of Godfrey (Citation1989) on the LM testing approach. Consider the model,
(18)
(18) where
can be any set of regressors that satisfy the usual regularity conditions. Suppose that the estimated model is given by
(19)
(19)
which is misspecified by missing
such that
with
. By applying a two-way within transformation, we obtain the estimated model by
and
where
. Let
be a set of variables, designed to capture some model misspecification.
can overlap partly or wholly with
, or be a proxy for
. For example, we have:
and
. Define
that is a composite parameter depending on a number of model parameters such as regression coefficients and correlations between
,
and
. Notice that
is part of the score function of the regression model,
(20)
(20)
evaluated at
. If
, we can use (19) to carry out a consistent estimation of
, rather than using the more complex model, (20) that requires the construction of
, as a means of approximating (18). It would be cumbersome to construct
in some instances, as is the case for
and
. So we formally test the null hypothesis,
, using the test statistic given by
where
and
. This is not a test of whether adding
to (19) improves the model fit. Such a test would be based on a panel version of the F-test, and would reject the null,
, as long as
. What we test here is whether we can consistently estimate
by (19), coupled with the FE estimation. Notice that the two null hypotheses (
and
) overlap under many circumstances. For example,
requires
under (1) and (2), if N = 1. The fact that they do not overlap in our setting is closely linked to our null hypothesis that
is conditionally independent from
.
Remark 6.
Another useful property of the LM test is its relative robustness to model misspecification under the null hypothesis. To formalize this we augment the model (18) to and complete this specification by assuming that
follows (2) and setting
where
is iid across i with finite mean,
and variance,
.
are independent of
and
for all i, j and t. Suppose that we retain (19) as the estimated model. Then, we examine if
(21)
(21) which determines whether the LM test retains its validity as a test of the null hypothesis, (4) under the model misspecification. If
and
are uncorrelated (i.e.,
and
are uncorrelated), it is straightforward to show that
such that (21) holds since
. On the other hand, if
and
are correlated, then
. Hence,
As contains the term
, (21) does not hold. Then, the LM test diverges with probability approaching one even under the null hypothesis.
Remark 7.
An extension of the model (1) to cover nonstationary factor models is feasible. For the case of I(1) factors (with I(0) errors, and
), it is easily seen that Theorem 1 holds under Assumption A and the null hypothesis, (4). We explored this issue in a limited Monte Carlo study. The results, not reported for brevity, are available upon request.
Remark 8.
Consider another modification, arising from weak loading correlations. Define two sets of units. The first is the set of unit indices, denoted , for which (17) holds while the complement is the set of unit indices, denoted
, for which (17) does not hold. Denote the cardinality of
by
. We refer to this setting as a weak factor case with exponent ϖ. We then have:
As long as , it follows that
where
This implies that the presence of weak loading correlation () does not affect the LM test inference. On the other hand, if
,
But,
implying
. Corollary 1 summarizes the above discussions.
Corollary 1.
Consider the panel data model with IE given by (1) under a weak factor setting with exponent ϖ. Under Assumption A, if , then the LMX test statistic in (16) follows the
distribution where k is the number of the regressors. If
, the LMX test is consistent.
2.1 Extension to Dynamic Panels with Large T
The homogeneous panel data model with lagged dependent variables and IE has been analyzed by Moon and Weidner (Citation2015, 2017), who propose a quasi maximum likelihood (QML) estimator. Song (Citation2013) extends the iterative PC analysis of Bai (Citation2009) for dynamic panels under parameter heterogeneity, but provides an asymptotic theory for the individual estimator only. Chudik and Pesaran (Citation2015) extend the CCE approach to heterogeneous dynamic panels with IE, and propose a CCEMG estimator by augmenting the model with a sufficient number of lagged cross-sectional averages. Via Monte Carlo simulations, they demonstrate that the CCEMG estimator and the MG estimator based on Song’s (Citation2013) individual PC estimator perform better than Bai’s IPC estimator and the QML estimator by Moon and Weidner (Citation2015). The (bias-corrected) CCEMG estimator of the autoregressive parameters on the lagged dependent variable still exhibits significant bias, mainly due to the Nickel bias of order . Recently, Norkute et al. (Citation2021) develop two instrumental variable (IV) estimators for dynamic panel data models with exogenous covariates and a multifactor error structure, when both N and T are large, by projecting out the common factors from the exogenous covariates and constructing instruments based on defactored covariates. For a homogeneous model they propose a two-step IV estimator, which is not subject to either the small T Nickel bias or asymptotic biases, unlike the QML estimator by Moon and Weidner (Citation2015). For a heterogeneous model they propose a mean group IV estimator, which is shown to outperform the CCEMG estimator by Chudik and Pesaran (Citation2015).
Consider the heterogeneous dynamic panel data model with IE:
(22)
(22) where
. If the null hypothesis, (4) is not rejected, then the (modified) two-way FE estimator will still be valid to apply to the dynamic panel data with IE. Notice that only the MG estimator is consistent for dynamic panels if the parameters are heterogeneous (Pesaran and Smith Citation1995).
We first consider the case with serially uncorrelated factors, . To show that the dynamic two-way FE estimator of ρij and
from (22) is unbiased under the null, we should ensure that
for
and
. It is clear by (9) that
. Consider
. Under Assumption A,
. Further, it is easily seen that
if factors,
are serially uncorrelated. Hence, under the null, it is valid to apply the FE estimator to the dynamic panel data model with IE. We then construct the LMX test using the dynamic FE residuals given by
(23)
(23) where
and
are the two-way dynamic FE estimator obtained from (22). By Theorem 1, it is easily seen that
under the null hypothesis, (4).
Next, to deal with the challenging case where are serially correlated, we suppose that
follow an infinite order vector autoregressive (VAR) process:
where
and
is an
vector of iid errors. Now, the dynamic FE estimator of ρij and
from (22) is biased even under the null, (4) because
for
due to
. This suggests that the LMX test constructed using the FE residuals in (23), suffer from size distortions.
In this regard, for a valid inference, we propose the use of the following autoregressive distributed lag (ARDL) approximation of (22):
(24)
(24)
This representation follows since yit and are generated by a state space model where
is the unobserved state. By Theorem 1.2.1 of Hannan and Deistler (Citation1989), the ARDL representation formalizes the usual projection argument:
Then, it is easily seen that
for
and
for
. Next, we propose the finite order ARDL approximation of (24):
(25)
(25) where we suggest using
or
(e.g., Lewis and Reinsel Citation1988; Hannan and Deistler Citation1989; Chudik and Pesaran Citation2015). Under the null hypothesis, (4), the two-way dynamic ARDL estimator of δij and
from (25), denoted
and
, will be unbiased. Hence, when constructing the proper LMX test, we propose the use of the following ARDL residuals:
(26)
(26)
Assumption B.
The lag polynomial, in (22) has the roots outside the unit circle such that
for some
. The VAR lag polynomial,
satisfy
where
denotes spectral norm, and
. The idiosyncratic errors,
and
are serially uncorrelated and mutually independent.
To show that the LMX test constructed, using the ARDL residuals in (26), follows the distribution asymptotically under the null hypothesis, (4), we need to strengthen Assumption A, replaced by Assumption A’ in the Online Supplement. We also consider a setting with
and impose the DGP condition in (2). These refinements are needed to accommodate the case with serially correlated factors.
Theorem 2.
Consider the dynamic heterogeneous panel data model with IE given by (22) and (2), the ARDL representation, (24) and the ARDL approximation, (25). Then, under Assumption A’ and B and under the null hypothesis, (4), as , the LMX test statistic in (16), constructed using the ARDL residuals in (26), follows a
distribution where k is the dimension of
. Under the alternative hypothesis, (5), the LMX test is consistent.
Remark 9.
Theorems 1 and 2 do not require any particular relative rates for N and T for the validity of the LM test. For large N and T, we only need to show that the dominant term of the LM test statistic tends to a normal variate while the remaining terms tend to zero. This is shown to be theoretically less demanding than deriving convergence rates for the pooled or MG estimators that would involve handling asymptotic biases.
Remark 10.
In practice we suggest to apply the LMX test with the ARDL residuals to the dynamic panel data with IE, irrespective of whether factors are serially correlated or not. See Section S4.5 in the Online Supplement for the comprehensive simulation results. In the presence of serially correlated factors, the dynamics given by in (22), do not capture the full dynamics of the panel data model due to neglecting the dynamics embedded in
. In this regard, if the LMX test does not reject the null hypothesis, then we suggest the ARDL model, (25) as a proper dynamic specification.
Remark 11.
It is not straightforward to find the one-to-one relationship between the parameters in (22) and (25). If we are still interested in consistently estimating the parameters of the model, (22) under the null hypothesis, then we may consider applying the IV estimation, similarly to Norkute et al. (Citation2021). For convenience we focus on the simple dynamic panel model with IE:
or in the matrix notation,
where
and
. We consider the (internal) IV matrix given by
, which satisfies the orthogonality condition,
under the null. Given
,
can be consistently estimated by
, which is referred to as the IVFE estimator. We find via simulations that
and
and their MG counterparts show negligible biases though the bias of ρi is higher than that of βi in small samples. But, the LMX test constructed using the IVFE residuals given by (S.50), tends to over-reject the null for large N, especially if the serial correlation of the factors is pronounced. See Section S4.6 in the Online Supplement.
3 Monte Carlo Simulations
W examine the size and power performance of the LMX statistic in (16) under both static and dynamic panel data frameworks.
3.1 Monte Carlo Design
As a benchmark, we generate the data with two regressors (k = 2) and two factors (r = 2):
(27)
(27)
(28)
(28)
We allow serial correlation and weak cross-sectional correlation in by generating
with
and
. We generate
and
(the simulation results for serially correlated factors are qualitatively similar and available upon request). To test the validity of the LM test, we generate the factor loadings,
and
under the following two experiments:
Experiment 1 (independent factor loadings):
, and
such that
Experiment 2 (correlated factor loadings):
, and
We explore the performance of the LMX test under the parameter heterogeneity by generating for
. We have also constructed DGPs with the different number of regressors and factors; namely for
and
. See Section S3 in the Online Supplement for a full description. We examine the following three cases for
: Case 1: Weak heterogeneity with
; Case 2: Medium heterogeneity with
; Case 3: Strong heterogeneity with
. We consider the following combinations of
. We set the number of replications at R = 1000.
3.2 The Performance of the LMX Statistic
We evaluate the size of the test under Experiment 1 and the power under Experiment 2. If r = 0, we only report the size of the test. reports the size performance under Experiment 1 with
, respectively. The size of the LMX test is close to the nominal level (5%) for all sample sizes and its performance is shown to be invariant to the number of regressors, the number of factors and the different strengths of parameter heterogeneity.
Table 1 The size of the LMX test under Experiment 1.
In we present the power performance. Overall, the LMX test is reasonably powerful even for T = 30, but it becomes consistent as the sample size increases. Even as the parameter heterogeneity gets stronger and/or the number of regressors and factors increases, the power of the LMX test remains satisfactory in most cases.
Table 2 The power of the LMX test under Experiment 2.
We find that the finite sample performance of the LMX test is satisfactory in all cases considered, even under strong heterogeneity. Overall simulation results demonstrate that the LMX test is robust to serial correlation and weak cross-sectional correlation in errors as well as the slope heterogeneity.
Section S.4.1 in the Online Supplement explores the performance of the LM test when both factors and loadings are generated by a zero mean process. The performances of the LM test reported in Tables S.5 and S.6 are qualitatively similar to those in and . Furthermore, in Section S4.2, we report additional simulation results for the following cases: Case A with homogeneous parameters, β = 1 for all i and iid errors; Case B with homogeneous parameters and serially and weakly cross-sectionally correlated errors; Case C with heterogeneous parameters, and iid errors; Case D with heterogeneous parameters, and serially and weakly cross-sectionally correlated errors. The simulation results for Cases A–C are qualitatively similar to those reported here for Case D.
Further, we evaluate the size and power performance the LMX test in panels with small T (T = 3, 5) in conjunction with (), using DGPs with one regressor and two factors (see Section S.3.1.2 for details) and set
. We consider two cases:
Case A
with iid error, .
Case B
with serially and weakly cross-sectionally correlated error generated by with
and
.
reports the size and power performance of the LMX test for T = 3, 5. The size of the LMX test is close to the nominal level (5%) for all cases considered while its performance is shown to be invariant to the number of factors. The size performance of the LMX test is also robust to serial and weak cross-sectional error correlation. Given the fixed T (as small as T = 3), the power of the LMX test rises monotonically with N. Overall, the power of the LMX test remains satisfactory.
Table 3 The size and power of the LMX test with small T and k = 1.
3.3 Robustness to Misspecification
To investigate the size performance of the LMX test in the presence of model misspecification, we generate the DGP using three regressors (k = 3) and two factors (r = 2), but apply the LMX test as if k = 1:
(29)
(29)
We allow serial correlation and weak cross-sectional correlation in by generating
with
and
. We generate
and
. We generate the factor loadings only under Experiment 1 (independent factor loadings) as follows:
;
,
;
;
such that
We explore the size performance of the LMX test by generating for k = 1, 2, 3, and examine the following three cases for
: Case 1: weak heterogeneity with
; Case 2: medium heterogeneity with
; Case 3: strong heterogeneity with
. We consider the combinations of
, and set the number of replications at R = 1000.
The results in display that the size of the LMX test is mostly close to the nominal level (5%) in all cases considered, confirming that it is robust to model misspecication. For comparison, we report the size performance of the Hausman test proposed by Kapetanios, Serlenga, and Shin (Citation2023), which is severely oversized in the presence of neglected regressors, leading to an incorrect rejection of the null hypothesis in all cases.
Table 4 The size of the LMX and tests in the presence of the model misspecification.
3.4 Extension to Heterogeneous Dynamic Panel Data Models with Large T
We generate the heterogeneous dynamic panel data with a single regressor (k = 1) and two factors (r = 2):
(30)
(30)
We allow weak error cross-sectional correlation by generating with
and
. We generate
, and serially correlated factors as
where
and
. We generate the factor loadings under the following two settings:
Experiment 1 (independent factor loadings):
,
such that
Experiment 2 (correlated factor loadings):
and
.
We explore the performance of the LMX test under parameter heterogeneity by generating and
. We examine the following three cases for
: Case 1: weak heterogeneity with
and
; Case 2: medium heterogeneity with
and
; Case 3: strong heterogeneity with
and
. To explicitly take into account the presence of serially correlated factors, we use the ARDL approximation, (25) and construct the LMX test using the ARDL residuals given by (26). We consider the combinations of
, and set the number of replications at R = 1, 000.
reports the size and power performance under Experiments 1 and 2, respectively. The size of the LMX test is close to the nominal level (5%) for all sample sizes and its performance is invariant to the number of factors and the different strengths of parameter heterogeneity. Under Experiment 2 the LMX test is reasonably powerful in small samples, but it becomes consistent as the sample size increases. Even if the parameter heterogeneity gets stronger and/or the number of factors increases, the power of the LMX test remains satisfactory in most cases. This suggests that the size and power performance of the LMX tests, using the ARDL residuals, is satisfactory for the heterogeneous dynamic panel data with IE, irrespective of whether the factors are serially correlated or not.
Table 5 The size and power of the LMX test for the heterogeneous dynamic panel data.
In Section S4.5 in the Online Supplement we have conducted comprehensive simulation exercises, covering the following 4 cases: Case A with serially uncorrelated factors and the dynamic FE residuals given by (23); Case B with serially uncorrelated factors and the ARDL residuals given by (26); Case C with serially correlated factors with and the dynamic FE residuals; Case D with serially correlated factors and the ARDL residuals. The performance of the LMX test under Case 1 is satisfactory while the simulation results for Case 2 are qualitatively similar to those for Case 1. This suggests that the performance of the LMX test using either dynamic FE residuals or ARDL residuals is satisfactory, only if the factors are serially uncorrelated. Under Case 3, however, the LMX test suffers from severe size distortions that worsen as the number of factors rises. This clearly suggests that we need to deal with serially correlated factors through the use of the ARDL approximation for correct inference. The simulation results under Case 4 are qualitatively similar to those for Case 2. Based on this evidence we propose the use of the ARDL residuals when constructing the LMX test in practice.
4 Empirical Applications
To investigate the empirical relevance of the null hypothesis of (conditional) independence between regressors and factor loadings in the heterogeneous panel data model with IE, we apply the LMX test to a total of 22 datasets (17 under a static panel data framework including 3 panels with small T and 5 under a dynamic panel data framework). In what follows we briefly describe the datasets used in the applications (see Section S5 in the Online Supplement for a full description).
4.1 Static Panel Data Framework
4.1.1 Cobb-Douglas Production Function
We estimate the production function in five different cases: the OECD members (N = 26 and T = 41, Mastromarco, Serlenga, and Shin Citation2016), the Italian regions (N = 20 and T = 21), the 48 U.S. States (N = 48 and T = 17, Munnell Citation1990), the aggregate sectoral data for manufacturing from developed and developing countries (N = 25 and T = 25, Eberhardt and Teal Citation2020), and the manufacturing industries across the OECD countries (N = 82 and T = 26, Eberhardt, Helmers, and Strauss Citation2013).
4.1.2 Gravity Model of Bilateral Trade Flows
We estimate a gravity model of the bilateral trade flows, where bilateral trade flow is a function of GDP, countries’ similarity, relative factor endowment, the real exchange rate as well as the trade union and common currency dummies. We estimate a model for the 91 pairs of EU14 countries from 1960 to 2008 (N = 91 and T = 49, Serlenga and Shin Citation2007).
4.1.3 Gasoline Demand Function
To evaluate the price and income elasticities of gasoline demand, we estimate the gasoline demand function using the quarterly data for the 50 U.S. States over the period 1994–2008 (N = 50 and T = 60, Liu Citation2014).
4.1.4 Housing Prices
We estimate the income elasticity of real housing prices from 1975 to 2010 using two datasets for the 49 U.S. States (N = 49 and T = 36, Holly, Pesaran, and Yamagata Citation2010) and the 384 Metropolitan Statistical Areas (N = 384 and T = 36, Baltagi and Li Citation2014).
4.1.5 Technological Spillovers on Productivity
We consider two applications. First, we estimate the effects of domestic and foreign R&D on total factor productivity (TFP) controlling for the human capital. We use a balanced panel of 24 OECD countries over the period 1971–2004 (N = 24 and T = 34), see Coe, Helpman, and Hoffmaister (Citation2009) and Ertur and Musolesi (Citation2017). Next, we explore the channels through which technological investments affect the productivity performance of industrialized economies by estimating the productivity effects of R&D and Information and Communication Technologies (ICT), controlling for the inputs accumulation as labor and (non-ICT) capital. We use a balanced panel of 49 high-tech OECD industries over the period 1977–2006 (N = 53 and T = 30, Pieri, Vecchi, and Venturini Citation2018).
4.1.6 Health Care
We estimate the relationship between healthcare expenditure and income after controlling for public expenditure over total health expenditure. We consider a panel of 167 countries covering the period 1995–2012 (N = 167 and T = 18, Baltagi et al. Citation2017).
4.1.7 Demographic and Business Cycle Volatility
We estimate the impact of the age composition of the labor force on business cycle volatility. We employ a balanced panel dataset for 51 countries over the period 1957–2000 (N = 51 and T = 44, Everaert and Vierke Citation2016).
4.1.8 Carbon Emissions and Trade
We explore the nexus between carbon emissions and trade using a balanced panel of 32 OECD countries over the period 1990–2013 (N = 32 and T = 24, Liddle Citation2018).
4.1.9 Health Care with Small T
We use a balanced panel data for 140 countries from 1993 to 1997 (N = 140, T = 5, Greene Citation2004) and regress a composite measure of health care delivery on per capita public and private health care expenditure. Further, we consider a balanced panel data for 2084 individuals from 1995 to 1999 (N = 2084, T = 5, Winkelmann Citation2004). We aim to evaluate the impact of the 1997 health care reform in Germany by estimating the regression of the number of doctor visits on the logged gross income.
4.1.10 Wage Equation with Small T
We estimate a reduced form of the wage equation by employing the balanced panel data (N = 595 and T = 7, Cornwell and Rupert Citation1988).
4.2 Dynamic Panel Data Framework
4.2.1 Economic Growth
We explore dynamic economic growth. First, we estimate the growth equation proposed by Islam (Citation1995). We employ a balanced dataset of 87 countries from 1960 to 2007 (N = 87 and T = 48, Ditzen Citation2018). Next, we use a balanced panel of 47 countries over 1961–2003 (N = 47 and T = 43) and estimate the dynamic effects of temperature shocks on aggregate output growth, see Dell, Jones, and Olken (Citation2012) and Vos and Everaert (Citation2021). Lastly, we estimate a dynamic growth model to identify the effects of public debt on economic growth. We use a balanced panel data of 33 countries from 1972 to 2010 (N = 33 and T = 42, Chudik et al. Citation2017).
4.2.2 Energy Intensities and Urbanization
We estimate the dynamic impact of urbanisation on total energy usage by using a balanced panel of 24 Chinese provinces from 1987 to 2011 (N = 24 and T = 25, Ma Citation2015).
4.2.3 Income Inequality and Natural Resources
We analyse the dynamic relationship between natural resource endowments and income inequality using a balanced panel data of 17 OECD countries from 1981 to 2014 (N = 17 and T = 33, Kim, Chen, and Lin Citation2020).
presents the main estimation and test results for the heterogeneous static panel datasets. The LMX test can reject the null hypothesis (4) at the 5% significance only two times for the gravity model of bilateral trade flows and the income elasticity of real housing prices for MSA, while rejecting the null at the 10% significance two more times for the U.S. production function and the income elasticity of real housing prices for U.S. States, out of 14 datasets. These results provide convincing evidence that the null hypothesis of conditional independence between factor loadings and the regressors is not rejected in the majority of cases, suggesting that it is still valid to apply the consistent and robust FE estimator to the panel data with IE.
Table 6 Empirical applications for the static heterogeneous panel data.
Next, we turn to the results for the CD test proposed by Pesaran (2015) and the bias-corrected CD* test recently advanced by Pesaran and Xie (Citation2021), both of which test the null hypothesis of weak residual CSD against the alternative of strong CSD. Both CD tests are applied to the two way FE residuals. We report the CD* test results computed using one factor only though the results are qualitatively similar when employing the different number of factors. The CD (CD*) test rejects the null hypothesis 10 (12) times out of 14 datasets. We also consider the Hausman tests proposed by Bai (Citation2009) and Westerlund (2019b), denoted HB and HW, which test the null hypothesis of additive two-way effects against the alternative hypothesis of the multiplicative IE. The HB test marginally rejects the null hypothesis only once for the gravity model of bilateral trade flows whilst the HW test rejects two more times for UNIDO production function and the income elasticity of real housing prices for MSA. Apparently, these results are in conflict, since the CD test indicates the presence of CSD while the Hausman tests reveal the absence of IE in most panels. We have already shown that the HB and HW tests become inconsistent if factor loadings and the regressors are conditionally independent (see Monte Carlo simulations in Section S2 in the Online Supplement). In this regard, these conflicting results indicate that non-rejection of the null hypothesis by the Hausman tests is mainly due to the (observed) conditional independence between the regressors and loadings in the panel data with IE, rather than the absence of IE, under which the FE estimator is consistent. Furthermore, notice that for the three datasets where the HB and HW tests can reject the null of additive effects, we can also reject our null hypothesis. Combined together, this provides a strong support for the utility and importance of applying our proposed LM specification test in practice.
Next, presents the test results for the dynamic heterogeneous panel data. Out of the five datasets, the LMX test can reject the null hypothesis marginally only once for the effects of public debt on economic growth. Moreover, the CD (CD*) test rejects the null hypothesis of weak residual CSD at the 1% significance in 5 (4) cases. Notice, however, that the theoretical properties of both CD tests have not been established yet for the dynamic panel data model.
Table 7 Empirical applications for the dynamic heterogeneous panel data.
reports the LM test results for static panel data with small T. We also find that the LMX test does not reject the null hypothesis for all three datasets we consider.
Table 8 Empirical applications for heterogeneous panel data with small T.
Finally, following the suggestion by an anonymous referee, we examine whether the LMX test results are invariant to the case where the correct model specification is dynamic, but the practitioner applies a static model specification, even though we have applied the same model specification as in the original studies. We now consider the dynamic specifications for the gravity model of the bilateral trade flows, the income elasticity of real housing prices, the R&D production function, and the demographic and business cycle volatility, where we add the lagged dependent variable to the original static specifications. We then apply the LM test statistic constructed using the ARDL residuals from the approximation, (25). We find that the null hypothesis is rejected for the gravity and the housing income elasticity models whereas the null is not rejected for R&D production function and volatility (these results are available upon request), confirming that the LM test results are the same under both the static and dynamic panel data frameworks.
Combining all these results, we may conclude that our proposed LM test will make an essential specification test, given the pervasive evidence in favor of strong CSD. Therefore, we suggest applying the LM test to determine the form of IE that can validate the use of the FE estimator. Following the suggestion by an anonymous referee, we add practical guidelines for step-by-step model specifications in Section S7 in the Online Appendix. In the case where the null hypothesis of conditional independence is not rejected, the FE estimation can still produce consistent estimation and robust inference in a variety of cross-sectionally correlated panels, albeit less efficient than the PC estimator, though we emphasize that the FE estimator is invariant to any complex issues related to selecting the true number of unobserved factors (Moon and Weidner Citation2015), and to employing inconsistent initial estimates which may not guarantee the convergence of the iterative PC estimator (Hsiao Citation2018).
5 Conclusions
A large strand of the literature on panel data has focused on analyzing CSD, based on the error components model with IE, which is implicitly understood to bias the two-way FE estimator, due to the potential endogeneity arising from the correlation between regressors and factors/loadings (e.g., Bai Citation2009).
In this article we have built upon the notion that if the regressors and factor loadings are conditionally independent, then the panel data model with IE can still be consistently estimated by the two-way FE estimator. This suggests that the null hypothesis of conditional independence between the regressors and loadings emerges as an influential feature of the panel data modeling with IE. We have proposed an easy way of verifying the validity of such an important misspecification hypothesis through the v test, that only requires the use of the FE estimator. Crucially, our proposed test can be easily applicable to either static panels with a small number of time periods or dynamic panels with serially correlated factors. In this regard the LM test can make a valuable addition to the toolkit of applied researchers as it provides a support for the use of the consistent and robust FE estimator under the null hypothesis.
Finally, we apply the LM test to a number of existing panel datasets, and find substantial evidence that the regressors and factor loadings are likely to be conditionally independent in practice. This suggests that the FE estimator can still provide a simple but robust strategy in a variety of cross-sectionally correlated panels including a growing literature in microeconometrics that focuses on estimating causal/counterfactual effects or policy evaluations.
We note a couple avenues for further researches. First, given the pervasive empirical evidence supporting the conditional independence between the regressors and factor loadings, it will be worthwhile to develop the simple consistent estimator for heterogeneous dynamic panel data model with IE under weaker conditions. Next, we can extend our testing approach to high-dimensional and/or multi-dimensional panels, for example, Cameron, Gelbach, and Miller (Citation2011), Kapetanios, Serlenga, and Shin (Citation2023) and Choi, Lin, and Shin (Citation2021). For example, consider the three-dimensional heterogeneous panel data model of Kapetanios, Serlenga, and Shin (Citation2023), given by where yijt is the dependent variable observed across three indices, i being the origin unit, j the destination unit at period t and
is the
vector of covariates with
being an
vector of parameters. Further, uijt is given by
, where
and
are vectors of unobserved global, origin-specific and destination-specific factors with
and
being the corresponding vectors of heterogeneous loadings, and
are idiosyncratic errors. If loadings and the regressors are conditionally independent, then we conjecture that the appropriate within estimator retains consistency. In this case an extended LM test can be obtained by
where
is the corresponding residual and
is the fitted value of the regressors when regressed on the first principal components of
and
. Clearly, a number of alternative extensions can be envisaged. Notice, however, that there are both conceptually and technically challenging issues to be addressed carefully.
Supplementary Materials
The online supplement contains all technical proofs, additional simulation results the full description of the datasets and the empirical specifications, and practical guidelines.
No potential conflict of interest was reported by the authors.
onlinesupplement.zip
Download Zip (1.6 MB)Acknowledgments
We are mostly grateful for the insightful comments by the editor, the associate editor and two anonymous referees as well as Jia Chen, David Kang, Rui Lin, Hashem Pesaran, Vasilis Sarafidis, Ron Smith, Michael Thornton, Takashi Yamagata, Chaowen Zheng and the seminar participants at the Rimini Centre for Economic Analysis, University of East Anglia, Athens University of Economics and Business, Lancaster University Management School, University of York, the 9th Italian Congress of Econometrics and Empirical Economics, University of Cagliari, Italy, 21–23 January 2021, and the KER International Conference, Seoul, 27–28 July 2021 for helpful comments. The usual disclaimer applies.
Additional information
Funding
References
- Arkhangelsky, D., Athey, S., Hirshberg, D. A., Imbens, G. W., and Wager, S. (2021), “Synthetic Difference in Differences,” American Economic Review, 111, 4088–4118. DOI: 10.1257/aer.20190159.
- Athey, S., and Imbens, G. W. (2022), “Design-based Analysis in Difference-In-Differences Settings with Staggered Adoption,” Journal of Econometrics, 226, 62–79. DOI: 10.1016/j.jeconom.2020.10.012.
- Bai, J. (2009), “Panel Data Models with Interactive Fixed Effects,” Econometrica, 77, 1229–1279.
- Bai, J., and Ng, S. (2002), “Determining the Number of Factors in Approximate Factor Models,” Econometrica, 70, 191–221. DOI: 10.1111/1468-0262.00273.
- Baltagi, B. H., Lagravinese, R., Moscone, F., and Tosetti, E. (2017), “Health Care Expenditure and Income: A Global Perspective,” Health Economics, 26, 863–874. DOI: 10.1002/hec.3424.
- Baltagi, B. H., and Li, J. (2014), “Further Evidence on the Spatio-Tempral Model of House Prices in the United States,” Journal of Applied Econometrics, 29, 515–522. DOI: 10.1002/jae.2372.
- Cameron, A. C., Gelbach, J. B., and Miller, D. L. (2011), “Robust Inference with Multiway Clustering,” Journal of Business & Economic Statistics, 29, 238–249. DOI: 10.1198/jbes.2010.07136.
- Castagnetti, C., Rossi, E., and Trapani, L. (2015), “Testing for no Factor Structures: On the Use of Hausman-Type Statistics,” Economics Letters, 130, 66–68. DOI: 10.1016/j.econlet.2015.02.030.
- Charbonneau, K. B. (2017), “Multiple Fixed Effects in Binary Response Panel Data Models,” Econometrics Journal, 20, S1–S13. DOI: 10.1111/ectj.12093.
- Choi, I., Lin, R., and Shin, Y. (2021), “Canonical Correlation-based Model Selection for the Multilevel Factors,” Journal of Econometrics, 233, 22–44. DOI: 10.1016/j.jeconom.2021.09.008.
- Chudik, A., Mohaddes, K., Pesaran, M. H., and Raissi, M. (2017), “Is There a Debt-Threshold Effect on Output Growth?” The Review of Economics and Statistics, 99, 135–150. DOI: 10.1162/REST_a_00593.
- Chudik, A., and Pesaran, M. H. (2015), “Common Correlated Effects Estimation of Heterogeneous Dynamic Panel Data Models with Weakly Exogenous Regressors,” Journal of Econometrics, 188, 393–420. DOI: 10.1016/j.jeconom.2015.03.007.
- Coakley, J., Fuertes, A.-M., and Smith, R. (2006), “Unobserved Heterogeneity in Panel Time Series Models,” Computational Statistics and Data Analysis, 50, 2361–2380. DOI: 10.1016/j.csda.2004.12.015.
- Coe, D. T., Helpman, E., and Hoffmaister, A. W. (2009), “International R&D Spillovers and Institutions,” European Economic Review, 53, 723–741. DOI: 10.1016/j.euroecorev.2009.02.005.
- Cornwell, C., and Rupert, P. (1988), “Efficient Estimation with Panel Data: An Empirical Comparison of Instrumental Variables Estimators,” Journal of Applied Econometrics, 3, 149–155. DOI: 10.1002/jae.3950030206.
- Cui, G., Hayakawa, K., Nagata, S., and Yamagata, T. (2023), “A Robust Approach to Heteroskedasticity, Error Serial Correlation and Slope Heterogeneity in Linear Models with Interactive Effects for Large Panel Data,” Journal of Business & Economic Statistics, 41, 862–875. DOI: 10.1080/07350015.2022.2077349.
- Cui, G., Sarafidis, V., and Yamagata, T. (2022), “IV Estimation of Spatial Dynamic Panels with Interactive Effects: Large Sample Theory and an Application on Bank Attitude towards Risk,” The Econometrics Journal, 226, 62–79. DOI: 10.1093/ectj/utac026.
- Dell, M., Jones, B. F., and Olken, B. A. (2012), “Temperature Shocks and Economic Growth: Evidence from the Last Half Century,” American Economic Journal: Macroeconomics, 4, 66–95. DOI: 10.1257/mac.4.3.66.
- Ditzen, J. (2018), “Estimating Dynamic Common-Correlated Effects in Stata,” The Stata Journal, 18, 585–617. DOI: 10.1177/1536867X1801800306.
- Eberhardt, M., Helmers, C., and Strauss, H. (2013), “Do Spillovers Matter When Estimating Private Returns to R&D?” The Review of Economics and Statistics, 95, 436–448. DOI: 10.1162/REST_a_00272.
- Eberhardt, M., and Teal, F. (2020), “The Magnitude of the Task Ahead: Macro Implications of Heterogeneous Technology,” Review of Income and Wealth, 66, 334–360. DOI: 10.1111/roiw.12415.
- Ertur, C., and Musolesi, A. (2017), “Weak and Strong Cross-Sectional Dependence: A Panel Data Analysis of International Technology Diffusion,” Journal of Applied Econometrics, 32, 477–503. DOI: 10.1002/jae.2538.
- Everaert, G., and Vierke, H. (2016), “Demographics and Business Cycle Volatility: A Spurious Relationship?” Journal of Applied Econometrics, 31, 1467–1477. DOI: 10.1002/jae.2519.
- Fernandez-Val, I., and Weidner, M. (2016), “Individual and Time Effects in Nonlinear Panel Models with Large N, T,” Journal of Econometrics, 192, 291–312. DOI: 10.1016/j.jeconom.2015.12.014.
- Godfrey, L. G. (1989), “Misspecification Tests in Econometrics: The Lagrange Multiplier Principle and Other Approaches. Econometric Society Monographs. Cambridge: Cambridge University Press.
- Greene, W. (2004), “Distinguishing between Heterogeneity and Inefficiency: Stochastic Frontier Analysis of the World Health Organization’s Panel Data on National Health Care Systems,” Health Economics, 13, 959–980. DOI: 10.1002/hec.938.
- Hannan, E. J., and Deistler, M. (1989), “The Statistical Theory of Linear Systems,” Statistical Papers, 30, 239–242.
- Holly, S., Pesaran, M. H., and Yamagata, T. (2010), “A Spatio-Temporal Model of House Prices in the USA,” Journal of Econometrics, 158, 160–173. DOI: 10.1016/j.jeconom.2010.03.040.
- Hsiao, C. (2018), “Panel Models with Interactive Effects,” Journal of Econometrics, 206, 645–673. DOI: 10.1016/j.jeconom.2018.06.017.
- Islam, N. (1995), “Growth Empirics: A Panel Data Approach,” The Quarterly Journal of Economics, 110, 1127–1170. DOI: 10.2307/2946651.
- Juodis, A. (2022), “A Regularization Approach to Common Correlated Effects Estimation,” Journal of Applied Econometrics, 37, 788–810. DOI: 10.1002/jae.2899.
- Juodis, A., and Reese, S. (2021), “The Incidental Parameters Problem in Testing for Remaining Cross-section Correlation,” Journal of Business & Economic Statistics, 40, 1191–1203. DOI: 10.1080/07350015.2021.1906687.
- Kapetanios, G., Pesaran, M. H., and Yamagata, T. (2011), “Panels with Non-stationary Multifactor Error Structures,” Journal of Econometrics, 160, 326–348. DOI: 10.1016/j.jeconom.2010.10.001.
- Kapetanios, G., Serlenga, L., and Shin, Y. (2023), “Estimation and Inference for Multi-Dimensional Heterogeneous Panel Datasets with Hierarchical Multi-Factor Error Structure,” Journal of Econometrics, 220, 504–531. DOI: 10.1016/j.jeconom.2020.04.011.
- ———(2023), “Testing for Correlation between the Regressors and Factor Loadings in Heterogeneous Panels with Interactive Effects,” Empirical Economics, 64, 2611–2659.
- Karabiyik, H., Reese, S., and Westerlund, J. (2017), “On the Role of the Rank Condition in CCE Estimation of Factor-Augmented Panel Regressions,” Journal of Econometrics, 197, 60–64. DOI: 10.1016/j.jeconom.2016.10.006.
- Kim, D.-H., Chen, T.-C., and Lin, S.-C. (2020), “Does Oil Drive Income Inequality? New Panel Evidence,” Structural Change and Economic Dynamics, 55, 137–152. DOI: 10.1016/j.strueco.2020.08.002.
- Kripfganz, S., and Sarafidis, V. (2021), “Instrumental-Variable Estimation of Large-t Panel-Data Models with Common Factors,” Stata Journal, 21, 659–686. DOI: 10.1177/1536867X211045558.
- Lewis, R. A., and Reinsel, G. C. (1988), “Prediction Error of Multivariate Time Series with Mis-specified Models,” Journal of Time Series Analysis, 9, 43–57. DOI: 10.1111/j.1467-9892.1988.tb00452.x.
- Liddle, B. (2018), “Consumption-based Accounting and the Trade-Carbon Emissions Nexus,” Energy Economics, 69, 71–78. DOI: 10.1016/j.eneco.2017.11.004.
- Liu, W. (2014), “Modeling Gasoline Demand in the United States: A Flexible Semiparametric Approach,” Energy Economics, 45, 244–253. DOI: 10.1016/j.eneco.2014.07.004.
- Ma, B. (2015), “Does Urbanization Affect Energy Intensities across Provinces in China? Long-Run Elasticities Estimation Using Dynamic Panels with Heterogeneous Slopes,” Energy Economics, 49, 390–401. DOI: 10.1016/j.eneco.2015.03.012.
- Mastromarco, C., Serlenga, L., and Shin, Y. (2016), “Modelling Technical Efficiency in Cross Sectionally Dependent Stochastic Frontier Panels,” Journal of Applied Econometrics, 31, 281–297. DOI: 10.1002/jae.2439.
- Moon, H. R., and Weidner, M. (2015), “Linear Regression for Panel with Unknown Number of Factors as Interactive Fixed Effects,” Econometrica, 83, 1543–1579. DOI: 10.3982/ECTA9382.
- ———(2017), “Dynamic Linear Panel Regression Models with Interactive Fixed Effects,” Econometric Theory, 33, 158–195.
- Munnell, A. (1990), “How Does Public Infrastructure Affect Regional Economic Performance,” New England Economic Review, 34, 11–33.
- Norkute, M., Sarafidis, V., Yamagata, T., and Cui, G. (2021), “Instrumental Variable Estimation of Dynamic Linear Panel Data Models with Defactored Regressors and a Multifactor Error Structure,” Journal of Econometrics, 220, 416–446. DOI: 10.1016/j.jeconom.2020.04.008.
- Pesaran, M., and Smith, R. (1995), “Estimating Long-Run Relationships from Dynamic Heterogeneous Panels,” Journal of Econometrics, 68, 79–113. DOI: 10.1016/0304-4076(94)01644-F.
- Pesaran, M. H. (2006), “Estimation and Inference in Large Heterogeneous Panels with a Multifactor Error Structure,” Econometrica, 74, 967–1012. DOI: 10.1111/j.1468-0262.2006.00692.x.
- ———(2015), “Testing Weak Cross-Sectional Dependence in Large Panels,” Econometric Reviews, 34, 1089–1117.
- ———(2021), “General Diagnostic Tests for Cross-Sectional Dependence in Panels,” Empirical Economics, 60, 13–50.
- Pesaran, M. H., and Xie, Y. (2021), “A Bias-Corrected CD Test for Error Cross-Sectional Dependence in Panel Data Models with Latent Factors,” arXiv Working Paper 2109.00408.
- Pieri, F., Vecchi, M., and Venturini, F. (2018), “Modelling the Joint Impact of R&D and ICT on Productivity: A Frontier Analysis Approach,” Research Policy, 47, 1842–1852. DOI: 10.1016/j.respol.2018.06.013.
- Sarafidis, V., and Wansbeek, T. (2012), “Cross-Sectional Dependence in Panel Data Analysis,” Econometric Reviews, 31, 483–531. DOI: 10.1080/07474938.2011.611458.
- Sarafidis, V., Yamagata, T., and Robertson, D. (2009), “A Test of Cross Section Dependence for a Linear Dynamic Panel Model with Regressors,” Journal of Econometrics, 148, 149–161. DOI: 10.1016/j.jeconom.2008.10.006.
- Serlenga, L., and Shin, Y. (2007), “Gravity Models of Intra-EU Trade: Application of the CCEP-HT Estimation in Heterogeneous Panels with Unobserved Common Time-Specific Factors,” Journal of Applied Econometrics, 22, 361–381. DOI: 10.1002/jae.944.
- Song, M. (2013), “Asymptotic Theory for Dynamic Heterogeneous Panels with Cross-sectional Dependence and Its Applications,” Mimeo, Columbia University.
- Vos, I. D., and Everaert, G. (2021), “Bias-Corrected Common Correlated Effects Pooled Estimation in Dynamic Panels,” Journal of Business & Economic Statistics, 39, 294–306. DOI: 10.1080/07350015.2019.1654879.
- Westerlund, J. (2019a), “On Estimation and Inference in Heterogeneous Panel Regressions with Interactive Effects,” Journal of Time Series Analysis, 40, 852–857. DOI: 10.1111/jtsa.12432.
- ———(2019b), “Testing Additive versus Interactive Effects in Fixed-T Panels,” Economics Letters, 174, 5–8.
- Westerlund, J., and Urbain, J. P. (2013), “On the Estimation and Inference in Factor-Augmented Panel Regressions with Correlated Loadings,” Economics Letters, 119, 247–250. DOI: 10.1016/j.econlet.2013.03.022.
- ———(2015), “Cross-Sectional Averages versus Principal Components,” Journal of Econometrics, 185(2):372–377.
- Winkelmann, R. (2004), “Health Care Reform and the Number of Doctor Visits: An Econometric Analysis,” Journal of Applied Econometrics, 19, 455–472. DOI: 10.1002/jae.764.