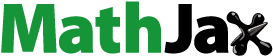
Abstract
We investigate information processing in the stochastic process driving stock’s volatility (volatility discovery). We apply fractionally cointegration techniques to decompose the estimates of the market-specific integrated variances into an estimate of the common integrated variance of the efficient price and a transitory component. The market weights on the common integrated variance of the efficient price are the volatility discovery measures. We relate the volatility discovery measure to the price discovery framework and formally show their roles on the identification of the integrated variance of the efficient price. We establish the limiting distribution of the volatility discovery measures by resorting to both long span and in-fill asymptotics. The empirical application is in line with our theoretical results, as it reveals that trading venues incorporate new information into the stochastic volatility process in an individual manner and that the volatility discovery analysis identifies a distinct information process than that based on the price discovery analysis.
1 Introduction
The proliferation of trading venues within many developed security markets has been a new trend in the financial equity market. The U.S., for instance, features 11 exchanges, 85 alternative trading systems (ATS) and more than 200 dealers.Footnote1 The market fragmentation phenomenon experienced in the U.S. highlights the importance of the microstructure of trading venues, as traditional listing exchanges have created markets within markets, changed markets’ pricing structures, and set up specialized microstructures to attract specific trading clienteles (Menkveld, Citation2014; O’Hara, Citation2015; Menkveld, Citation2016). Retail brokers have responded to this new market setting by routing their orders to multiple trading platforms (Battalio, Corwin, and Jennings, Citation2016). Consequently, the way traders and markets learn and disseminate information is also dispersed across markets (O’Hara, Citation2015).
In this context of a decentralized system in which there are multiple prices for one homogeneous security, the study of information processing among trading venues becomes highly complex and, at the same time, of great significance for both market participants and regulators. In this article, we take a different angle to the price discovery literature and investigate information processing in the stochastic process driving stock’s volatility.Footnote2 The economic reasoning of the volatility discovery framework hinges on the fact that economic agents do not process volatility related news instantaneously and on the relevance of the informational content driving the volatility process. As for the former, recent evidence in Lochstoer and Muir (Citation2022) shows that financial agents have sticky expectations about volatility that generates initial underreaction and delayed overreaction to volatility news. As for the volatility informational content, French and Roll (Citation1986) document that volatility is mainly caused by private information which affects prices when informed investors trade. Differently from lagged returns, the volatility process contains important information that produces some degree of predictability in future returns (see, for instance, Ng and Ludvigson, Citation2007; Bollerslev, Tauchen, and Zhou, Citation2009). More specifically, Bollerslev et al. (Citation2013) find that the implied and realized variation fractionally cointegrate and this long-run equilibrium relationship predicts returns over interdaily horizons. Finally, the information contained in the volatility process has compelled traders to use derivative markets to trade volatility (Ni, Pan, and Poteshman, Citation2008). All in all, the volatility process reveals information that is not present in lagged returns and hence it is not captured by the price discovery framework. This means that the volatility discovery analysis can be a valuable tool to identify the source (what market) of this information.
The price discovery literature has identified the unobserved efficient price by extracting commonalities within the transaction prices of a homogeneous security. In this context, prices are cointegrated, and the vector error correction (VEC) model has become the workhorse of price discovery analyses. We rely on a similar intuition and exploit the evidence that estimates of the integrated variance (realized measures) are long memory processes and cointegrated in order to identify the latent integrated variance of the efficient price using the fractionally cointegrated vector autoregressive (FCVAR) model of Johansen and Nielsen (Citation2012).Footnote3 We then investigate how different markets impound information into the integrated variance of the efficient price.
We make the following methodological and theoretical contributions: First, we build upon the continuous-time theory and characterize the volatility discovery phenomenon, leading to the derivation of the volatility discovery measures. Second, we show that recovering the efficient price by means of a permanent and transitory decomposition (building block of any price discovery measure) generally does not suffice to identify the integrated variance of the efficient price. Third, we resolve the issue of the generated regressor problem in the FCVAR framework when using realized measures. We employ double asymptotics to establish the limiting distribution of the volatility discovery measures, enabling correct inferences on the volatility discovery process.
Our empirical application investigates the volatility discovery mechanism for 30 of the most actively traded stocks in the U.S over a large sample period of 7 years. The tick-by-tick quotes consists of firms from different industries that are traded in three trading venues. We document that markets indeed trade toward the efficient integrated variance, that is, market specific integrated variances are fractionally cointegrated. Our results reveal that trading venues incorporate new information into the stochastic volatility process in an individual manner. In line with our theoretical results, the price discovery analysis identifies distinct market leaders than those based on the volatility discovery measures. Concurrently with this work, Dimpfl and Elshiaty (Citation2021) reformulate our model to discrete time and study volatility discovery in cryptocurrencies markets. More specifically, they employ a stochastic volatility model fitted to daily returns to obtain daily volatility estimates and compute the volatility discovery measures using the FCVAR model. Forte and Lovreta (Citation2019) also apply FCVAR model. They find that the CDS implied equity volatility and a equity options based index (VSTOXX) are fractionally cointegrated. Finally, Baule, Frijns, and Tieves (Citation2017) deviate from our approach and adapt Hasbrouck’s (Citation1995) information share measure to stationary processes to study volatility discovery for options and bank-issued options (warrants).
The remainder of the article proceeds as follows. Section 2 introduces the volatility discovery framework. Next, Section 3 shows how to identify the integrated variance of the efficient price as a function of both price and volatility discovery measures. Section 4 derives the asymptotic properties of the volatility discovery measures and Section 5 presents an empirical application. Section 6 offers concluding remarks. The supplementary material presents the proofs, a simulation exercise, and additional empirical results.
2 The Volatility Discovery Framework
Asset price theory postulates that the efficient log-price of a financial security at time t must follow a semimartingale process defined in some filtered probability space (see, among others, Andersen et al., Citation2003; Barndorff-Nielsen et al., Citation2011). Additionally, econometrics and finance literature have widely considered the volatility process of the efficient price a stochastic process, such that the efficient price can be written as
(1)
(1) where a is a predictable locally bounded drift, σm is a càdlàg volatility process denoted as the efficient stochastic volatility process, and, without loss of generality, W is a Brownian motion. It is important to note that mt is driven by two separate stochastic processes: W and σm, which reflect new information arrival to the price and volatility processes, respectively.Footnote4 Furthermore, the efficient price is considered a latent process not only due to trading frictions but also as a result of market fragmentation.
Our framework consists of investigating a homogeneous asset traded in S different markets. In general terms, the S-dimensional log-price process is modeled as a Brownian semimartingale defined in some filtered probability space . The S-dimensional daily log-price process then reads
(2)
(2) where
is a predictable locally bounded drift,
is a càdlàg volatility matrix with
denoting the càdlàg spot covariance matrix, and W is a S-dimensional vector of Brownian motions. The quadratic variation of P is defined as
(3)
(3)
where
with n denoting the number of intraday observations such that
and T being the total number of trading days, such that
. Because the price process in (2) does not consider jumps, the diagonal elements of
are the market-specific integrated variances (IV). The IV is central to the pricing of financial instruments, portfolio allocation, and risk management. In a fragmented market context, not only market prices but also their stochastic volatilities are expected to gather information at different speeds, following market characteristics such as market design, trading costs, trading clientele, liquidity, and the presence of informed traders.
The price discovery literature typically adopts the VEC model to approximate the dynamics of (2), identify the single common stochastic trend seen as the efficient price, and obtain consistent estimates of the price discovery measures. Among the most popular price discovery measures are Booth, So, and Tseh’s (Citation1999) component share measure and variants of Hasbrouck’s (Citation1995) information share (IS) measure.Footnote5
To investigate the dynamics of the market-specific IVs, we first acknowledge the fact that these IVs are tied to a long-run equilibrium and track a common latent stochastic process seen as the integrated variance of the efficient price. Second, we need to define feasible estimates of market-specific IVs originated from (3). We generically denote these estimates as realized measures (RM), which are consistent estimators computed with ultra-high-frequency data. There are several consistent estimators for the IV (see Mcaleer and Medeiros (Citation2008) for a comprehensive survey of realized measures estimators), thus, at this point, we do not distinguish among these different estimators, apart from requiring them to be consistent estimates of the market-specific IVs. Third, as opposed to security prices, which are known to be integrated of order one, , processes, there is well-documented evidence that RMs are persistent and characterized by a long memory. The long memory feature of RMs follows from early work on the conditional volatility of daily financial returns (Baillie, Bollerslev, and Mikkelsen, Citation1996) and on modeling RMs (Andersen and Bollerslev, Citation1997; Andersen et al., Citation2003; Corsi, Citation2009). In particular, Andersen et al. (Citation2003) note that The slow hyperbolic autocorrelation decay symptomatic of long memory is evident…. This stylized fact of RMs implies that these series are fractionally integrated of some order d. A process zt is said to be fractionally integrated of order d if
, where ηt is an integrated of order zero (stationary) process. It follows that
is the fractional difference operator defined in terms of the binomial expansion
.
One way to accommodate these characteristics is to approximate the daily dynamics of RMs using the fractionally cointegrated vector autoregressive (FCVAR) model of Johansen (Citation2008) and Johansen and Nielsen (Citation2012),
(4)
(4) where
is a
vector that concatenates the element-wise logarithmic of the daily market-specific RMs,
is a S × S consistent estimator of
is the usual lag operator for fractional processes, d and b are the fractional order of the RMs and the degree of fractional cointegration, respectively, and
is iid with a mean zero and positive-definite covariance matrix
. The parameters in the FCVAR framework have the same common interpretation as the parameters in the standard VEC model. In that, α corresponds to the speed of adjustment and β is the cointegrating vector so that
is integrated of order d – b and represents the long-run equilibrium relation. It is relevant to note two important aspects of the approximation in (4). First, because the RMs are not expected to diverge over time, the rank of
should equal S – 1 in that the RM’s share a single common fractional stochastic trend. We see this stochastic trend as an estimate of the logarithm of the IV of the efficient price. Second, we expect deviations between the RMs in the different markets to be transient, that is, a short memory (covariance stationary) process driven by the trading-venue-specific characteristics, such as cost structure, different degrees of transparency, the speed of order execution and different trader groups, among other features. This stylized fact is accommodated by assuming d = b in (4), which implies that
is an
process. Finally, we allow the RMs to present a fractional order in the range of
, implying that these measures may be nonstationary but mean reverting.Footnote6 It is possible to extend the FCVAR approximation in (4) to the estimates of the market-specific spot volatilities at a cost of a larger measurement error on their estimates. Nevertheless, our simulation study shows that fitting a FCVAR model to the daily RMs still allows us to capture the partial adjustment dynamics that occurs on the spot volatilities.Footnote7
Multivariate random walk decompositions are central to constructing any price discovery measure, as they decompose observed prices into two components: the efficient price—the stochastic trend—and an
process that is associated with the portion of information that has no permanent impact on prices. In the same way that the random walk decomposition constitutes the basic building block of price discovery analysis, the fractional counterpart of the Granger representation theorem serves as a building block for volatility discovery analysis. By decomposing the RMs into a long memory term common to all markets and market-specific
terms, we can identify the efficient price’s IV and investigate how different venues incorporate information and adjust to the long-run equilibrium. Johansen (Citation2008) and Johansen and Nielsen (Citation2012) provide the fractional counterpart of the Granger representation theorem and decompose the process Rt into
and
components:
(5)
(5) where
is a truncated version of the fractional difference operator of order d; Xt is an
stochastic term; and
and
are the orthogonal complements of α and β, respectively, with
, and
denoting a
vector of ones. Similarly to the price discovery case, if
and
, the matrix
has common rows. Section 5.1 provides strong empirical support for the cointegrating vector akin to
in the FCVAR setup.Footnote8 Hence, a similar interpretation as that for the price discovery analysis holds in the volatility discovery setup, implying that Rt shares a single fractional stochastic trend that is integrated of order d, given by
(6)
(6)
where ψ accounts for the common row of
. Notably,
possesses the long-term persistence and slow hyperbolic decay discussed in Andersen et al. (Citation2003), and it can be seen as the natural estimate of the logarithm of the efficient price’s IV. Given that
can be recovered by
, we can investigate how it is tied to innovations to the RMs of the different markets.
In view of the Granger representation in (6), is essentially a normalization factor, meaning that
plays the key role on identifying the fractional stochastic trend. In turn, we define
as a measure of volatility discovery. The elements of
show how innovations from the logarithm of the market-specific RMs contribute to
. As the element of
for a given market increases, the importance of this market for the volatility discovery process increases.
The economic interpretation of is therefore given by its ability to identify where (in what markets) the informational content driving the efficient volatility is revealed. In the context of highly fragmented markets,
has the potential to guide what markets should be used when computing the realized measures, meaning that the markets with the highest volatility discovery measures should be preferred to markets with low importance to the volatility discovery mechanism. Moreover, similar to the price discovery analysis, the volatility discovery framework can be a valuable component of a comprehensive toolbox for assessing market quality. By combining the insights from volatility discovery measures with other relevant indicators, we can gain a deeper understanding of market dynamics, information efficiency, and overall market quality. Because the information content of the volatility process has predictive power for future returns and volatility (see, for instance, Patton and Sheppard, Citation2015; Baltussen, van Bekkum, and van der Grient, Citation2018), knowing the source of this information has the potential to improve derivatives pricing and out-of-the sample forecasts.
3 Identification of the Efficient Price’s IV
The discussion posed in the previous section relies on discrete-time approximations to introduce the volatility discovery framework. This section assumes a parametric structure to (2) and to the daily dynamics of its quadratic variation, such that we can work out the identification of the IV of the efficient price. Next, we provide a theoretical bridge between the price and volatility discovery measures and disentangle the role played by them on impounding information to the IV of the efficient price. The proofs for the theoretical results are available on the supplementary material.
Our first goal is to write the IV of the efficient price as a function of both price and volatility discovery measures. To this extent, we need to specify a data-generating process for the general market-specific price process in (2) and the daily dynamics for the market-specific IVs. The price process is such that the drift parameter has reduced rank, that is, prices cointegrate, and the diffusion matrix is stochastic. Assumption 1 provides the technical conditions.
Assumption 1.
The log-prices on any given day of an asset that trades on S multiple venues follow the S-dimensional Brownian semimartingale
(7)
(7) where γ and δ have dimensions
is a
matrix of rank S – 1 with all eigenvalues having negative real parts, meaning that the eigenvalues of
lie in the left open half-plane of the complex plane and at zero, and
is a càdlàg process with
for all
, where
denotes the
th element of a matrix.
The process in (7) is Markovian and the discrete-time parameters obtained from its discretization coincide with those from the reduced-rank Gaussian Ornstein-Uhlenbeck (OU) process in Kessler and Rahbek (Citation2004). In that, Kessler and Rahbek’s (Citation2004) Theorem 1 holds automatically, meaning that the subspace in spanned by the columns of the discrete-time speed-of-adjustment parameters is the same of those spanned by γ.
Next, we establish a multivariate random walk decomposition of the process in (7). To decompose (7) into long- and short-run components, we employ the identity where
and
, where
and
are the orthogonal complements of γ and δ, respectively. The first and second terms in this identity are orthogonal and hence satisfy the Gonzalo and Granger’s (Citation1995) definition of permanent and transitory components. More specifically,
returns the stochastic trend common to all markets, that is, a common Brownian semi-martingale with initial value given by
, whereas
is an I(0) component that admits a continuous-time Wold representation. Proposition 1 formalizes the results.
Proposition 1.
Let Assumption 1 holds. The permanent and transitory (P-T) decomposition then reads
(8)
(8) where
is the stochastic trend and
.
The P-T decomposition generalizes the results in Kessler and Rahbek (Citation2001) to the case of a stochastic covariance matrix. The common stochastic trend, ft, driving the price process is a Brownian semi-martingale and is used as a price discovery measure (the component share measure). Furthermore, according to Proposition 1, the IV of ft is given by
.
Next, we investigate the relationship between the quadratic variation of Pt and . To this extent, we start by writing the daily quadratic variation of Pt in (7) as Footnote9
(9)
(9)
In the fragmented markets setting and in the absence of jumps in (7), the diagonal elements of (9) are the market-specific IVs, which we denote as . The empirical estimators of the quadratic variation usually adopted in the realized measures literature, for example, the realized variance estimator, are consistent estimators of
. Therefore, in the context of an homogeneous asset traded on multiple markets, the RMs estimate the market-specific IVs (and covariances) rather than the IV of the efficient price. The price discovery literature advocates using ft from the P-T decomposition to identify the IV of the efficient price. However, this identification strategy has an important caveat:
only takes into consideration the weights given by
and hence provides no information about any potential learning and temporal feedback mechanisms embedded into Σt in Assumption 1. More concretely, in order to identify the partial adjustments dynamics in the market-specific IVs, it is necessary to specify a parametric data-generating process for these quantities.
As briefly discussed in Section 2, much evidence from both the empirical and theoretical realized measures literature point toward the importance of taking into account the high degree of persistence and the long memory property in the integrated variance and realized measures (see, among others, Andersen et al., Citation2003; Corsi, Citation2009; Bollerslev, Patton, and Quaedvlieg, Citation2016; Shi and Yu, Citation2022). When fitting ARFIMA models to the RMs, statistically significant long memory parameter estimates typically fall within the range of . Consequently, RMs can exhibit either stationary or mean-reverting nonstationary processes (Rossi and de Magistris, Citation2014 and Shi and Yu, Citation2022). Alternatively, the long memory feature of RMs can be addressed using Corsi’s (Citation2009) heterogeneous autoregressive (HAR) model. The HAR family of models are based on a restricted autoregressive (AR) model that captures the persistence of the RMs by considering the RM in the previous day, its average over the previous week, and its average over 22 days. Baillie et al. (Citation2019) reconcile both approaches and estimate an ARFIMA model with the restrictions implied by the HAR model. Their findings demonstrate the benefits of incorporating the long memory parameter into the HAR model to explain higher-order dynamics. To capture the long memory feature of the IV of the efficient price as well as the fact that market-specific IVs do not diverge and are tied to a long-run equilibrium, we assume the dynamics of the daily market-specific logarithmic IVs follow a FCVAR process. Assumption 2 formalizes their data-generating process.
Assumption 2.
The daily logarithmic IVs of a given asset that trades on multiple venues follow a FCVAR model as
(10)
where
, α and β have dimensions
, and
denotes the element-wise logarithmic function.Footnote10
The errors
are iid process with
and
.
The matrix of cointegrating vectors is
, such that
.
Assumption 2 ensures that the market-specific IVs cointegrate and share a single common fractional stochastic trend with long memory order d. This common factor corresponds to the logarithm of the IV of the efficient price and reads
(11)
(11)
Alternatively, we could reformulate Assumption (2) in terms of primitive assumptions regarding both the efficient spot volatility and market-specific and efficient price IVs. Specifically, the fractional Ornstein-Uhlenbeck process of Comte and Renault (Citation1998) models the logarithmic of the spot volatility as a long memory process of order d. Consequently, the logarithmic of the spot volatility associated with the efficient price reads
(12)
(12) where ϖ is the drift parameter,
is the volatility (volatility of volatility) parameter, and
is a fractional Brownian motion (fBm).Footnote11 It is important to note that the fBm process in (12) does not imply an arbitrage opportunity because the price process in Assumption 1 is a semi-martingale. The logarithmic of the market-specific spot volatility in (12) inherits the long memory property from the fBm process, as its (pseudo) spectral density has a pole in zero that is a function of d:
as
, where
and
. Importantly, Proposition 2.2 in Comte and Renault (Citation1998) show that
, and σm share the same long memory order d. Additionally, Proposition 1 in Rossi and de Magistris (Citation2014) establishes that the integrated variance also possesses the same long memory degree d as
, that is,
is a long memory process of order d. This long memory feature of
is equivalent to the fractionally integrated property of the IV of the efficient price implied by Assumption 2. Moreover, because the market-specific integrated variances are tied to a long run equilibrium they can then be expressed as a function of
and an I(0) zero-mean covariance stationary process
, such that
with
. This specification establishes a connection with two important conditions implied by Assumption 2. First, it satisfies the definition of fractional cointegration with
, that is,
and
and
, are I(d) processes and
is an I(0) process. Second, it is consistent with Johansen and Nielsen’s (Citation2012) Granger representation theorem as implied by Assumption 2, as
and
share
as the common fractional stochastic trend. Finally, the main advantage of employing Assumption 2 over the set of primitive assumptions discussed earlier is that it allows for the identification of
using d and α (and consequently
). Additionally, it does not require any constraints on the parameters to ensure that estimates of
are positive, as the FCVAR is defined in terms of the logarithm of the market-specific IVs. Next, we characterize the IV of the efficient price as a function of the price and volatility discovery measures, that is
and
, respectively.
Theorem 1.
Let Assumptions 1 and 2 hold. Let , k(s) be a
vector that has its sth row equal to one and all the remaining entries equal to zero,
be a
matrix with
denoting a S × S matrix that has the (s, s)th entry equal to one and all the remaining entries equal to zero,
be a
elimination matrix such that, for any symmetric matrix A,
, and
be the element-wise logarithm. Then,
if
,
(13)
if
with
,
(14)
if
and
with
,
(15)
Theorem 1.1 carries two important implications. First, it shows how the price and volatility discovery mechanisms emerge as different sources on impounding information to the IV of the efficient price. Unlike fully encompasses the informational content and error correction mechanisms presented in Σt through
. Consistent with empirical evidence that demonstrate investor trade based on volatility information (e.g., Ni, Pan, and Poteshman, Citation2008), incorporating
instead of
is expected to enhance trading decisions, while leveraging
identifies what are the sources of information impacting
. Second, the identification of the IV of the efficient price using ft critically hinges on the condition
with
(Theorem 1.1). Failure to satisfy this condition renders any price discovery measure relying on estimates of
inconsistent. This has significant implications for any variation of Hasbrouck’s (Citation1995) IS measure, as such measures give the relative contribution of each market to the IV of the efficient price.
To further appreciate the limitation of using instead of
, we shall discuss the case in which the restriction
or
with
with
holds, that is, Theorem 1.1. In the very special case of
with
, a single market is fully responsible for impounding information to both Pt and
, meaning that the remaining exchanges are irrelevant with respect to information processing. Such scenario appears to be rather unlikely to hold in practise, as trading platforms fiercely compete for order flow and high-frequency traders operate across markets (see, for instance, O’Hara, Citation2015). Second, the two last terms on the right-hand side of (13) would collapse to zero if the diffusion matrix in (7) is constant, which rules out the presence of stochastic volatility altogether. In such case, there is neither intra- nor inter-daily learning with respect to the volatility processes across the different trading platforms.
Another possible scenario consists of examining the case where price and volatility discovery processes do not happen exclusively in one market, but holds. In such case, Theorem 1.1 shows that
remains a function of both price and volatility discovery measures. More specifically, the cross-quadratic variation is zero and
in (15) shows how
is related to elements of the daily quadratic variation of the transitory component in Proposition 1.
4 Estimation and Inference
We now turn our attention to the estimation and inference tools for the volatility discovery measures. We extend the Johansen and Nielsen’s (Citation2012) results on consistency and the asymptotic normality of the maximum likelihood (MLE) estimator to the context of double asymptotics (mixed case), that is, where both long span and infill asymptotics hold. This approach is necessary because we need to replace the latent market-specific IVs by their consistent intraday-based estimates in the FCVAR models. Because consistency of these realized measures are established for , consistency and asymptotic normality of the FCVAR-based volatility discovery measures require both
. This approach is consistent with previous work on estimating diffusion processes based on discretely observed data in which the asymptotic properties of the estimators depend on both
(see, for instance, Prakasa Rao, Citation1983; Tang and Chen, Citation2009). Specifically, in the context of extremum estimators similar to ours, Phillips and Yu (Citation2009) use the feasible central limit theorem for realized variances in Barndorff-Nielsen and Shephard (Citation2002) as the first step in their two-stage estimation procedure to estimate the parameters in the drift function of a diffusion process.
To obtain the log-likelihood function of the FCVAR model in (10), first note that Assumption 2.2 does not imply that the errors are Gaussian. This means that the estimation strategy based on the Gaussian likelihood in Johansen and Nielsen (Citation2012) can be interpreted in the context of quasi MLE estimation. In addition to Assumption 2, we need Assumption 3 to ensure the limiting behavior of the likelihood function for the FCVAR model.Footnote12
Assumption 3.
The initial values , are uniformly bounded with
for
and
for
.
Assumption 3 concerns the initial values in which the likelihood function is conditioned on and essentially determines that is well-defined for any
. Given Assumptions 2.1, 2.2, and 3, we follow Johansen and Nielsen (Citation2012) and define the Gaussian likelihood function conditional on
and
:
(16)
(16) where
is a
vector of free parameters and
are the corresponding residuals.Footnote13
The latent nature of the IVs means that we cannot compute the MLE estimator directly from (16). Therefore, we replace in (16) by their consistent high-frequency based estimates Rt. Assume prices are observed at regular spaced intervals, such that there is n intraday prices in a given day. Define
as the realized covariance estimator of
, such that
and
denotes the element-wise logarithm. The feasible conditional log-likelihood function then reads
(17)
(17) where
. Note that the notation in (17) includes the number of intraday observations n, which serves to highlight the fact that Rt are consistent estimates of the logarithm of the IVs as
. As a result,
also depends on n. More precisely, we impose the following assumptions on the RMs and feasible conditional log-likelihood function.
Assumption 4.
As
, where Πt is a positive-definite matrix (Barndorff-Nielsen and Shephard, Citation2004).
, with
and
denoting the Euclidean norm, and Λ is a compact set.
The initial values
are uniformly bounded with
for
and
for
.
Assumption 4.1 provides information on the rate of convergence of the RMs estimates, which is relevant for establishing the limiting distribution of in the mixed asymptotics case. The limiting result in Barndorff-Nielsen and Shephard (Citation2004) is obtained under the assumption of “no-leverage,” which corresponds to the case where
is random but independent of the Brownian motion in (7) and achieves the optimal convergence rate. Assumption 4.2 imposes a Lipschitz type smoothness condition to the feasible conditional log-likelihood function. Assumption 4.3 relates to the initial values and it is analogous to Assumption 3.
The estimator we consider is in the class of extremum estimators and hence it is necessary to show that feasible and infeasible conditional log-likelihood functions and their gradients converge uniformly in probability as . It then follows that, for a fixed
, the conditional MLE estimator based on (17) reduces to a reduced rank regression similar to the standard VEC case. The likelihood function can be simplified by concentrating out the set of parameters
. This allows us to estimate d through the numerical optimization of the profile likelihood function. As a result,
is the MLE estimator of λ. The limiting distribution of the MLE estimator for λ determines the asymptotic distribution underlining the LR-based cointegration rank test, which is used to determine the number of cointegrating vectors in the FCVAR model (see Johansen and Nielsen, Citation2012 for more details). Finally, we could use the MLE estimates of α to compute the estimates of the volatility discovery measures:
. Theorem 2 formalizes the limiting distribution of the volatility discovery measures. To show that, define
as
, where
such that
and
hold. Next, note that
is a vector-valued continuously differentiable function and define
. Also, let
and
be the first-order derivative of
and
, respectively, with respect to
and evaluated at a vector
that is between
and
be a
deterministic matrix such that
, and
.
Theorem 2.
Let Assumptions 1, 2.1, 2.2, 3, and 4 hold and suppose with
. If d > 1∕2, assume also that
with
. If
as
, then
;
The limiting distribution in Theorem 2 allows us to construct single hypothesis tests on the elements of and to formulate a Wald test for joint zero constraints on the
. Specifically, the Wald statistic has the usual limiting chi-squared distribution under the null hypothesis.
In the two-market setting, testing single zero restrictions on the elements of is equivalent to testing for weak exogeneity of one of the IVs with respect to the cointegrating vector β. This follows because the null hypothesis
implies
and the volatility discovery only occurs in market 2. Similarly, the null hypothesis
is equivalent to test the null hypothesis
and implies that volatility discovery happens exclusively in market 1. The rejection of both individual null hypotheses (
and
) suggests that volatility discovery occurs in the two markets. Finally, because Assumption 2 implies a FCVAR with zero autoregressive components, testing for weak exogeneity implies testing for Granger causality (strong exogeneity).
In the general S-dimensional market setting, testing multiple joint S – 1 zero constraints on the elements of allows us to examine whether a single market is solely responsible for impounding information into the efficient IV. For instance, if S = 3, rejecting both null hypotheses
and
, while failing to reject the null hypothesis
imply finding statistical evidence that market 1 is the single driver for the volatility discovery process. However, this procedure neglects the multiple testing issue to correct for Type I error. We address this issue in our empirical application by applying the Holm-Bonferroni procedure. This procedure involves sorting the multiple tests p-values in ascending order and sequentially comparing them against the significant level scaled by a function of the number of tests.
5 Empirical Application
Our data consist of 30 of the most actively traded assets in the U.S. extracted from the TAQ database. We consider the two major listing exchanges in the U.S.—Nyse (N) and Nasdaq (T)—and one of the most liquid trading venues, Arca (P). This sample selection reflects a balance between capturing relevant market information and avoiding estimation difficulties associated with a large number of markets. All stocks are simultaneously traded in at least two of the three markets covered in this analysis and represent a broad set of industries. We use tick-by-tick quotes from a sample period of 7 years, from January 2007 to December 2013, which captures the implementation of the National Market System regulation (Reg NMS) and the market fragmentation phenomenon.Footnote14Table S.3 in the supplementary material displays the details. We compute the daily RMs using Barndorff-Nielsen et al.’s (Citation2011) realized kernel estimator because it provides consistent estimates of the market IV measures under time-dependent and endogenous market microstructure noise, irregularly spaced data, and jumps. These features ultimately allow us to take advantage of mid-quotes observed at a tick-by-tick frequency.
5.1 Model Specification
We use the log RMs to estimate bivariate and trivariate FCVAR models.Footnote15 We consider four market combinations: Nasdaq-Arca, Nasdaq-Nyse, Arca-Nyse, and Nasdaq-Arca-Nyse. This choice accommodates assets that are not simultaneously traded on the three exchanges and ultimately yields a richer and broader sample, which is particularly important for handling firms that are listed on Nasdaq but are not traded on Nyse. We choose the lag length, κ, as the minimum value that makes the LM test for serial correlation on the residuals at the first 10 lags nonsignificant at the 5% significance level.Footnote16
To evaluate whether the RMs at different trading venues are fractionally cointegrated, we implement the sequential likelihood ratio (LR) test for the cofractional rank of Johansen and Nielsen (Citation2012). For each two-market combination, the RMs are fractionally cointegrated and share a single common fractional stochastic trend if . As for the three-market case, if
, then the RMs share a single fractional stochastic trend. presents the p-values associated with the LR cofractional rank test.Footnote17 The null is not rejected at the 5% significance level in all but five stocks, suggesting that the RMs are fractionally cointegrated and share the IV of the efficient price as a common fractional stochastic trend. As for the long memory feature of the RMs, we find that
is statistically significant at 1% significance level and usually greater than 0.5, confirming the RMs’ long memory characteristic (nonstationary and mean reverting). In line with Assumption 2.3, provides evidence that
and
hold for the two- and three-market combinations respectively, indicating that the market stochastic volatilities are expected to be equal in equilibrium. To appreciate that, recall that β is not uniquely defined, in that we normalize the left-hand
block of
to be the identity matrix and find that the
right-hand block of
equals
. In summary, provides the necessary preliminary results to ensure that Assumption 2 holds and both identification of the IVm and inference on the volatility discovery measures are valid.
Table 1 Cofractional rank test and estimates of the cointegrating vector.
5.2 Volatility Adjustment
We now turn our attention to the parameter estimates that determine the volatility discovery mechanism. We focus on the estimates of volatility discovery measures , which is normalized such that
.Footnote18 Recall that the market associated with the highest element of
leads the volatility discovery process. In view of the Granger representation in (6) and Assumption 2, the logarithm of the integrated variance of the efficient price is given by a weighted sum of the innovations in the different market-specific integrated variances, with weights given by the elements of
. In that, it is natural to interpret these weights as nonnegative, such that
with
and
. In finite sample analysis, it is still possible for some elements of the estimates of
to turn out to be negative. In these cases, we should not reject the null hypothesis that these volatility discovery measures are equal to zero.
presents the estimates of for each market combination, along with the significance levels (one-sided test) of the null hypothesis that a single market does not contribute to the volatility discovery process, that is,
with
.Footnote19 For the three-market setting, we provide the corresponding p-value for the null hypotheses that two volatility discovery measures are equal to zero. These p-values are denoted as
, where
and
. For example,
represents the p-value associated with the null hypothesis
.
Table 2 Volatility discovery measures.
Considering the first market combination, pointwise analyses show that holds for 19 of the 28 assets. This suggests that Arca is more important than Nasdaq. A more precise analysis is obtained by examining the hypothesis tests derived from Theorem 2. At the 5% significance level, we find that the volatility discovery mechanism occurs exclusively at Arca for 12 of the 28 stocks. This means that we fail to reject the null hypothesis
, while simultaneously rejecting the null hypothesis
. Similar analysis offers statistical evidence that the Nasdaq is the single driver for 5 assets only. In order to address the issue of multiple testing, we apply the Holm-Bonferroni correction procedure at the 5% significance level. We find that that Arca and Nasdaq are the single drivers of the volatility discovery process for 9 and 4 stocks, respectively.Footnote20
Results from the second market combination also suggest that Nasdaq is less important. We find that holds for 13 of the 19 stocks, indicating that Nyse leads the volatility discovery process. At the 5% significance level, we find statistical evidence that Nyse is the unique contributor for 9 of the 19 assets, which also implies that Nasdaq does not Granger-cause the Nyse for these stocks. Considering the same significance levels, we find that Nasdaq is the sole contributor for 6 stocks only. These findings remain consistent when applying the Holm-Bonferroni correction procedure.
Regarding the Arca-Nyse market combination Arca and Nyse lead in 11 and 10 of the 21 markets, respectively. When applying the hypothesis tests, we find that Arca and Nyse individually drive volatility discovery for 8 and 9 stocks, respectively, at the 5% significance level. Controlling for multiple tests does not alter the qualitative findings.
The two-market setting shows Nasdaq as the least important exchange to the volatility discovery process, whereas Nyse appears as the most efficient one. To obtain a more precise answer to which market drives the volatility discovery process, the natural step forward in our analysis is to extend it to a three-market setting. The final set of results in displays the results. Pointwise analyses reveal a stronger role of Nyse when compared to the two-market setting. Nyse dominates in 12 out of the 19 stocks, while Arca and Nasdaq leads in only 5 and 2 stocks, respectively. With joint hypothesis tests at the 5% significance level, we reject the null hypotheses and
and fail to reject the null hypothesis
for 8 of the 19 stocks, indicating that Nyse is the sole contributor to the volatility discovery process. Similarly, our analysis shows that Arca and Nasdaq are the sole contributor for 1 and 0 stocks, respectively. The Holm-Bonferroni correction procedure confirms Nyse as the single driver in 7 stocks.
Overall, our findings in indicate that Nasdaq plays a less significant role compared to Nyse, which emerges as the leading exchange in impounding information for the efficient integrated variance. Using the limiting distribution established in Theorem 2, we find statistical evidence suggesting that a single market typically acts as the sole driver of the volatility discovery process.
We further expand our empirical application to investigate the implications of our theoretical framework, which highlights the presence of two distinct sources of information within the price and volatility discovery mechanisms. Having documented the secondary role of Nasdaq for the volatility discovery mechanism, we next examine whether the same feature holds in the price discovery analysis. We follow the price discovery literature and adopt the VEC model as a discrete approximation of the observed prices in the three market combinations discussed previously.Footnote21 Our choice of price discovery measure is the component share (CS), which is invariant to the sampling frequency, meaning that we can consistently estimate it from prices sampled at any sampling intervals (Dias, Fernandes, and Scherrer, Citation2020). As there is a tradeoff between the choice of sampling interval and the size of the standard errors of the CS measures, we use mid-quotes aggregated at 10 sec to balance out the negative effect of market microstructure noise and the positive effect of having information at the highest possible frequency.
In general, the price discovery results in suggest that Nasdaq leads the price discovery mechanism when compared to both Arca and Nyse. We find that Nasdaq leads in 22 of the 28 stocks for the Nasdaq-Arca market combination and 14 of the 19 stocks for the Nasdaq-Nyse market combination. The Arca-Nyse combination shows generally more balanced results, with Arca leading in 13 of the 21 stocks. The results from the Nasdaq-Arca-Nyse market combination are robust to the two-market setting, with Nasdaq leading in 12 of the 19 stocks. In contrast with the volatility discovery analysis, we find that all markets contribute to the price discovery process irrespectively of the market combination, that is, we reject the null hypotheses for all
at the 5% significance level for all but two stocks. This result holds when controlling for the multiple testing issue.
Table 3 Price discovery measures.
Overall, our results suggest that the volatility and price discovery mechanisms do not necessarily occur in the same trading venue. This finding is in line with Theorem 1, which shows that the price and volatility discovery measures capture how markets incorporate news into two distinct efficient stochastic processes: the efficient price and the efficient stochastic volatility.
6 Conclusions
In the current context of market fragmentation, we develop a theoretical framework to investigate how distinct markets contribute to the efficient price’s IV. The economic rationale supporting the volatility discovery analysis rests on the premise that the efficient volatility process is a separate stochastic process. Just as the price discovery measure, the volatility discovery analysis contributes to assess market efficiency and investor protection—reliable and transparent information when making investment decisions using volatility.
The volatility discovery framework builds on evidence that the RMs of a homogeneous asset are tied to a long run equilibrium and share a common factor: the efficient stochastic volatility. We exploit RMs’ long memory feature and adopt the FCVAR model to identify and estimate how innovations to market volatilities contribute to the efficient volatility process. We show the role played by both price and volatility discovery measures on the identification of the integrated variance of the efficient price. Next, we establish the limiting distribution of the volatility discovery measures and discuss how to make inference on market leadership. Finally, our empirical application corroborate our theoretical findings: RMs are tied to a long run equilibrium; markets incorporate information distinctively; and volatility and price discovery do not necessarily occur in the same trading venue.
Supplementary Materials
It contains the proofs for Proposition 1 and Theorems 1 and 2. It also presents a review of the price discovery measures, a simulation exercise, data details, and additional empirical results (SuppVD.pdf file).
SuppVD.pdf
Download PDF (452 KB)Acknowledgments
The authors are grateful to the Editor Prof. Atsushi Inoue, an anonymous associate editor, two anonymous referees, Marcelo Fernandes, Asger Lunde, Carsten Tanggaard, Pedro Valls and seminar participants at several workshops and conferences. We thank for the opportunity to run the computational analysis on the High Performance Computing Cluster supported by the Research and Specialist Computing Support service at the University of East Anglia (UEA).
Disclosure Statement
The authors report there are no competing interests to declare.
Notes
2 The finance and econometrics literature has long viewed volatility as a separate stochastic process (see the excellent survey on stochastic volatility models in Shephard and Andersen (Citation2009)).
3 Well-documented evidence is found in the literature to show that estimates of integrated variance (e.g., realized variance) depict long memory features, that is, these estimates are characterized as highly persistent and presenting an autocorrelation function that decays at a hyperbolic rate (Andersen and Bollerslev, Citation1997; Andersen et al., Citation2003; Corsi, Citation2009, among others).
4 The exact parametric functional form of the stochastic volatility process σm is not relevant to our analysis, and hence we only stress that σm is driven by a different (possibly correlated) Brownian motion from W.
5 See supplementary material for an overview on the price discovery framework.
6 This is consistent with previous empirical findings, such as those of Rossi and de Magistris (Citation2014), among others, who find estimates of d greater than 0.5.
7 See supplementary material for the details.
8 Similarly to the orthogonal complements of the parameters in the VEC model, α and β are not unique and hence and
are also not fully identified. Without loss of generality, we impose the normalizations
and
.
9 The drift component is slower moving that the diffusion component, resulting in zero quadratic variation. As a result, the parameterization of the drift in Assumption 1 does not impact the quadratic variation of observed prices, which remains the same as in (3).
10 In line with the empirical evidence in Section 5, we simplify the exposition and set κ = 0 and d = b.
11 The fBm is defined as , where Γ is the Gamma function. The fBm process can also be written in terms of the Hurst index H rather than d, such that
. Comte, Coutin, and Renault (Citation2012) proposes an affine class of long memory volatility processes that involve fractional integration of the square root of the spot volatility process, rather than its logarithm as in (12). Additionally, the fractional OU in (12) can be written in terms of
(Rossi and de Magistris, Citation2014).
12 Assumptions 2 and 3 are equivalent to Assumptions 1–4 in Johansen and Nielsen (Citation2012).
13 Johansen and Nielsen’s (Citation2012) Theorem 4 yields that the likelihood function has a strict minimum at the true vector of the parameters and converges uniformly to its deterministic limit.
14 The Reg NMS in 2007 allows the entry of new trading venues that are linked together and compete for order flow, liquidity, and trades.
15 Estimation results for the FCVAR models are obtained using the computer program by Nielsen and Popiel (Citation2014).
16 In addition to the requirement that residuals should be a white noise process, we choose κ so that the roots of the characteristic polynomials lie outside the transformed unit circle, (see Johansen, Citation2008 for a theoretical discussion on identification).
17 We do not report the p-values when the null hypothesis is because we strongly reject the null for the 30 assets in all market combinations.
18 Tables S.5 and S.6 in the supplementary material report the estimates of α and the LM-test for serial correlation in the residuals, respectively.
19 Because we find κ = 0 for nearly all stocks (see Table S.4 in the supplementary material), the hypothesis tests below can be most often interpreted as Granger causality tests.
20 See Table S.7 in the supplementary material for the p-values of the volatility discovery measures.
21 See supplementary material for an overview of the price discovery analysis and the estimation details.
References
- Andersen, T. G., and Bollerslev, T. (1997), “Heterogeneous Information Arrivals and Return Volatility Dynamics: Uncovering the Long-Run in High Frequency Returns,” The Journal of Finance, LII, 975–1005. DOI: 10.2307/2329513.
- Andersen, T. G., Bollerslev, T., Diebold, F. X., and Labys, P. (2003), “Modeling and Forecasting Realized Volatility,” Econometrica, 71, 579–625. DOI: 10.1111/1468-0262.00418.
- Baillie, R. T., Bollerslev, T., and Mikkelsen, H. O. (1996), “Fractionally Integrated Generalized Autoregressive Conditional Heteroskedasticity,” Journal of Econometrics, 74, 3–30. DOI: 10.1016/S0304-4076(95)01749-6.
- Baillie, R. T., Calonaci, F., Cho, D., and Rho, S. (2019), “Long Memory, Realized Volatility and Heterogeneous Autoregressive Models,” Journal of Time Series Analysis, 40, 609–628. DOI: 10.1111/jtsa.12470.
- Baltussen, G., van Bekkum, S., and van der Grient, B. (2018), “Unknown Unknowns: Uncertainty About Risk and Stock Returns,” Journal of Financial and Quantitative Analysis, 53, 1615–1651. DOI: 10.1017/S0022109018000480.
- Barndorff-Nielsen, O. E., Hansen, P. R., Lunde, A., and Shephard, N. (2011), “Multivariate Realised Kernels: Consistent Positive Semi-Definite Estimators of the Covariation of Equity Prices with Noise and Non-synchronous Trading,” Journal of Econometrics, 162, 149–169. DOI: 10.1016/j.jeconom.2010.07.009.
- Barndorff-Nielsen, O. E., and Shephard, N. (2002), “Econometric Analysis of Realized Volatility and Its Use in Estimating Stochastic Volatility Models,” Journal of the Royal Statistical Society, Series B, 64, 253–280. DOI: 10.1111/1467-9868.00336.
- —– (2004), “Econometric Analysis of Realised Covariation: High Frequency based Covariance, Regression and Correlation in Financial Economics,” Econometrica, 72, 885–925.
- Battalio, R., Corwin, S. A., and Jennings, R. (2016), “Can Brokers have It All? On the Relation between Make-Take Fees and Limit Order Execution Quality,” The Journal of Finance, 71, 2193–2238. DOI: 10.1111/jofi.12422.
- Baule, R., Frijns, B., and Tieves, M. E. (2017), “Volatility Discovery and Volatility Quoting on Markets Foroptions and Warrants,” Journal of Future Markets, 28, 758–774.
- Bollerslev, T., Osterrieder, D., Sizova, N., and Tauchen, G. (2013), “Risk and Return: Long-Run Relations, Fractional Cointegration, and Return Predictability,” Journal of Financial Economics, 108, 409–424. DOI: 10.1016/j.jfineco.2013.01.002.
- Bollerslev, T., Patton, A. J., and Quaedvlieg, R. (2016), “Exploiting the Errors: A Simple Approach for Improved Volatility Forecasting,” Journal of Econometrics, 192, 1–18. DOI: 10.1016/j.jeconom.2015.10.007.
- Bollerslev, T., Tauchen, G., and Zhou, H. (2009), “Expected Stock Returns and Variance Risk Premia,” Review of Financial Studies, 22, 4463–4492. DOI: 10.1093/rfs/hhp008.
- Booth, G. G., So, R. W., and Tseh, Y. (1999), “Price Discovery in the German Equity Index Derivatives Markets,” The Journal of Futures Markets, 19, 619–643. DOI: 10.1002/(SICI)1096-9934(199909)19:6<619::AID-FUT1>3.0.CO;2-M.
- Comte, F., Coutin, L., and Renault, E. (2012), “Affine Fractional Stochastic Volatility Models,” Annals of Finance, 8, 337–378. DOI: 10.1007/s10436-010-0165-3.
- Comte, F., and Renault, E. (1998), “Long Memory in Continuous-Time Stochastic Volatility Models,” Mathematical Finance, 8, 291–323. DOI: 10.1111/1467-9965.00057.
- Corsi, F. (2009), “A Simple Approximate Long-Memory Model of Realized Volatility,” Journal of Financial Econometrics, 7, 174–196. DOI: 10.1093/jjfinec/nbp001.
- Dias, G. F., Fernandes, M., and Scherrer, C. M. (2020), “Price Discovery in a Continuous-Time Setting,” Journal of Financial Econometrics, 19, 985–1008. DOI: 10.1093/jjfinec/nbz030.
- Dimpfl, T., and Elshiaty, D. (2021), “Volatility Discovery in Cryptocurrency Markets,” The Journal of Risk Finance, 22, 313–331. DOI: 10.1108/JRF-11-2020-0238.
- Forte, S., and Lovreta, L. (2019), “Volatility Discovery: Can the CDS Market Beat the Equity Options Market?” Finance Research Letters, 28, 107–111. DOI: 10.1016/j.frl.2018.04.015.
- French, K. R., and Roll, R. (1986), “Stock Return Variances. The Arrival of Information and the Reaction of Traders,” Journal of Financial Economics, 17, 5–26. DOI: 10.1016/0304-405X(86)90004-8.
- Gonzalo, J., and Granger, C. (1995), “Estimation of Common Long-Memory Components in Cointegrated Systems,” Journal of Business & Economic Statistics, 13, 27–35. DOI: 10.2307/1392518.
- Hasbrouck, J. (1995), “One Security, Many Markets: Determining the Contributions to Price Discovery,” The Journal of Finance, 50, 1175–1198. DOI: 10.1111/j.1540-6261.1995.tb04054.x.
- Johansen, S. (2008), “A Representation Theory for a Class of Vector Autoregressive Models for Fractional Processes,” Econometric Theory, 24, 651–676. DOI: 10.1017/S0266466608080274.
- Johansen, S., and Nielsen, M. Ø. (2012), “Likelihood Inference for a Fractionally Cointegrated Vector Autoregressive Model,” Econometrica, 80, 2667–2732.
- Kessler, M., and Rahbek, A. (2001), “Asymptotic Likelihood Based Inference for Co-integrated Homogenous Gaussian Diffusions,” Scandinavian Journal of Statistics, 28, 455–470. DOI: 10.1111/1467-9469.00248.
- —– (2004), “Identification and Inference for Multivariate Cointegrated and Ergodic Gaussian Diffusions,” Statistical Inference for Stochastic Processes, 7, 137–151.
- Lochstoer, L. A., and Muir, T. (2022), “Volatility Expectations and Returns,” Journal of Finance, 77, 1055–1096. DOI: 10.1111/jofi.13120.
- Mcaleer, M., and Medeiros, M. C. (2008), “Realized Volatility: A Review,” Econometric Reviews, 27, 10–45. DOI: 10.1080/07474930701853509.
- Menkveld, A. J. (2014), “High-Frequency Traders and Market Structure,” Financial Review, 49, 333–344. DOI: 10.1111/fire.12038.
- —– (2016), “The Economics of High-Frequency Trading: Taking Stock,” Annual Review of Financial Economics, 8, 1–24.
- Ng, S., and Ludvigson, S. (2007), “The Empirical Risk-Return Relation: A Factor Analysis Approach,” Journal of Financial Economics, 83, 171–222. DOI: 10.1016/j.jfineco.2005.12.002.
- Ni, S., Pan, J., and Poteshman, A. (2008), “Volatility Information Trading in the Option Market,” The Journal of Finance, 58, 1059–1091. DOI: 10.1111/j.1540-6261.2008.01352.x.
- Nielsen, M. Ø., and Popiel, M. K. (2014), “A Matlab Program and User’s Guide for the Fractionally Cointegrated var Model,” Technical Report, QED working paper 1330.
- O’Hara, M. (2015), “High Frequency Market Microstructure,” Journal of Financial Economics, 116, 257–270. DOI: 10.1016/j.jfineco.2015.01.003.
- Patton, A. J., and Sheppard, K. (2015), “Good Volatility, Bad Volatility: Signed Jumps and The Persistence of Volatility,” The Review of Economics and Statistics, 97, 683–697. DOI: 10.1162/REST_a_00503.
- Phillips, P. C., and Yu, J. (2009), “A Two-Stage Realized Volatility Approach to Estimation of Diffusion Processes with Discrete-Data,” Journal of Econometrics, 150, 139–150. DOI: 10.1016/j.jeconom.2008.12.006.
- Prakasa Rao, B. L. S. (1983), “Asymptotic Theory for Non-Linear Least Squares Estimator for Diffusion Processes,” Statistics: A Journal of Theoretical and Applied Statistics, 14, 195–209. DOI: 10.1080/02331888308801695.
- Rossi, E., and de Magistris, P. S. (2014), “Estimation of Long Memory in Integrated Variance,” Econometric Reviews, 33, 785–814. DOI: 10.1080/07474938.2013.806131.
- Shephard, N., and Andersen, T. G. (2009), “Stochastic Volatility: Origins and Overview,” in Handbook of Financial Time Series, eds. T. Mikosch, J.-P. Kreiß, R. A. Davis, and T. G. Andersen, pp. 233–254, Berlin: Springer.
- Shi, S., and Yu, J. (2022), “Volatility Puzzle: Long Memory or Antipersistency,” Management Science, 69, 3861–3883. DOI: 10.1287/mnsc.2022.4552.
- Tang, C. Y. and S. X. Chen (2009), “Parameter Estimation and Bias Correction for Diffusion Processes,” Journal of Econometrics, 149, 65–81. DOI: 10.1016/j.jeconom.2008.11.001.