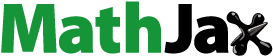
ABSTRACT
Replication in the social sciences is a necessary but oft-forgotten reality. In the Australian context, cross-cultural replication is an uncommon and relatively scarce practice. This study provides the first detailed analysis of the Corrections Mental Health Screen (Male) in an Australian setting. Using data for a national sample of 620 male police detainees interviewed as part of the Drug Use Monitoring in Australia (DUMA) program, our objective is to examine the scale’s item-level characteristics in a cross-cultural context, as well as to explore the consequences of a violation of the assumption of unidimensionality. Our results confirm that all 12 items of the CMHS-M are significantly correlated and exhibit strong internal consistency, although several items are relatively uninformative in these Australian data. The item measuring prior experience of psychiatric hospitalisation is particularly uninformative, while items which measure general psychological and antisocial traits (such as the lack of empathy, impulsivity, and interpersonal conflict) are likely to confound measurement in criminal justice settings. A two-dimensional model proves to be a superior fit and has implications for the estimation of the relationship between mental health, crime, and race.
Introduction
Replication, including cross-cultural replication, is critical for conceptual testing and theoretical generalisation in psychology and criminology, as it is in all fields of scientific enquiry. It is especially important where abstract theoretical constructs and their correlates are to be generalised from quantitative instruments and where the resulting estimates are used as a foundation for policy debate and program development (Payne and Wong Citation2018). Unfortunately, the practice of cross-cultural replication is not as common as it should be – at least not in an Australian context – and the so-called ‘replication crisis’ in psychology offers a stark reminder of the possible consequences (Loken and Gelman Citation2017).
In this study, we explore data collected by the Australian Institute of Criminology’s (AIC) Drug Use Monitoring in Australia (DUMA) program – a program which for two decades collected a comprehensive suite of data from police detainees at the entry point of the Australian criminal justice system. Modelled from the original Arrestee Drug Abuse Monitoring (ADAM) program (Golub and Johnson Citation2002; Department of Justice Citation2002) in 1999, the DUMA study has interviewed more than 60,000 offenders, mostly about their drug use and crime, but also about a wide range of other intersectional issues using instruments developed overseas (mostly in the US). Given the well-documented mediating effect of substance misuse on criminal behaviour within psychiatric populations (Alden et al. Citation2007; Arseneault et al. Citation2000; Lindqvist and Allebeck Citation1990; Monahan et al. Citation2001; Smith and Trimboli Citation2010), it is not surprising that instruments intended to estimate the prevalence of psychiatric disorders featured regularly in the DUMA survey. The most recent of these (Forsythe Citation2013; Forsythe and Gaffney Citation2012) was a pilot of the Corrections Mental Health Screen (CMHS) (Ford et al. Citation2007, Citation2009), though remarkably, none of the mental health instruments implemented in DUMA since its inception in 1999 (including the CMHS) have been the subject of detailed item-level analysis.
It is here that the present study makes a valuable and novel contribution to the international debate about the measurement of mental health in the criminal justice system: an important debate that ultimately concerns the efficiency and effectiveness of delivering psychological assessment and treatment services in the criminal justice sector. Specifically, we present the first ever Item Response Theory (IRT) analysis of the male version of the CMHS (CMHS-M). Our approach is motivated by the hypothesis that the CMHS-M is not a unidimensional scale, as would be otherwise assumed, but a multi-dimensional scale comprised of two separate constructs. Empirically, we predict that a two-dimensional model of the CMHS-M will provide a superior fit to these data and that the isolation of the antisocial behavioural indicators is important when such tools are used to draw inferences about the prevalence and correlates of mental health and crime. Not only does our use of Australian data offer the first cross-cultural item-level analysis of the CMHS-M, but our analysis has important implications further afield – particularly in the nexus between psychological and criminological theory where antisocial behaviour, lack of empathy, low self-control, and impulsivity (Gottfredson and Hirschi Citation1990) have each been foundational behavioural indicators.
Background and context
Like many complex phenomena, mental health is not easily reduced to a single scale or prevalence measure. Indeed, given that the experience of psychopathology is mediated by a combination of individualised biological, environmental, and situational factors, a full diagnostic interview schedule is essential for accurate diagnosis and treatment planning (Schneider et al. Citation2022). In the criminal justice sector, however, psychological and psychiatric services are a costly and finite resource (Ogloff et al. Citation2007) and the implementation of full diagnostic procedures is impractical (and arguably unnecessary) for the large number of criminal justice clients who do not require mental health related services or interventions. It is here that screening instruments like the CMHS-M play an important role, serving as tools designed and calibrated to triage offenders based on their experience of acute psychiatric symptoms, highlighting only those with the most immediate need for more a comprehensive diagnostic assessment (Ford et al. Citation2007, Citation2009). These screens are not substitutes for a proper diagnostic interview, but they are intended to make for a more efficient allocation of diagnostic services in hard-to-access populations. In the DUMA study, the CMHS-M was not designed to be used for diagnostic triaging (Forsythe Citation2013; Forsythe and Gaffney Citation2012), but rather to develop a baseline of data from which the prevalence and correlates of mental health and psychiatric disorders among detainees in the criminal justice system could be estimated and compared.
Psychiatric disorders in the justice system – prevalence and consequences
Worldwide estimates of psychiatric disorder within prison populations are estimated at between 32 and 94 percent (Andersen Citation2004; Wildeman and Andersen Citation2020) but the true population prevalence of psychiatric disorders in the Australian criminal justice system is unknown. The Australian Institute of Health and Welfare (Australian Institute of Health and Welfare Citation2019) estimates that two out of every five Australian prisoners (40%) report having had a mental disorder at some point during their lives (see also Butler et al. Citation2011). In custodial centres and arrestee populations, there appears to be an even greater prevalence, with estimates between 49 percent (Forsythe and Gaffney Citation2012) and 76 percent (Baksheev, Thomas, and Ogloff Citation2010). Similar to international work, the Australian literature is consistent only in its agreement that mental health and psychiatric disorders are more prevalent than in the general population,Footnote1 and more widespread than can be serviced by current programs within existing resources.
The importance of measuring the prevalence of mental health and psychiatric disorders in the criminal justice sector is underscored by an expanding literature which correlates both diagnosed and undiagnosed disorders with poorer short- and long-term criminal justice and community outcomes (White and Whiteford Citation2006). Chang et al. (Citation2015) found that ex-prisoners with common mental health problems, such as bipolar disorder, and alcohol and drug use disorders, were more likely to commit violent offences after their release (see also Whiting, Lichtenstein, and Fazel Citation2021). Hobbs et al. (Citation2006) highlighted that released prisoners were between two and three times more likely than the general population to have substantial contact with the mental health system (including hospitalisation). Kariminia et al. (Citation2007) highlighted an increased risk of suicide and drug overdose death within recently released prisoners, with 86 percent of suicides, and 97 percent of deaths due to overdose occurring after release from prison. More recently, the advent of the COVID-19 pandemic has once again reminded us of the need for accurate screening tools to understand the mental health of criminal justice-involved individuals (Hewson et al. Citation2020; Johnson et al. Citation2021; Tozzo, D’angiolella, and Caenazzo Citation2020).
Australian attempts to measure the prevalence of mental health problems on entry into the criminal justice system are relatively few in number. Schulte, Mouzos, and Makkai (Citation2005) trialled the use of the Kessler 10 Psychological Distress Scale ([K-10] (Kessler et al. Citation2002)) among detainees in the DUMA program, finding that 30 percent met the threshold for psychiatric assistance. In a similar police custodial context, J. R. Ogloff et al. (Citation2007) conducted a validation study of the Brief Jail Mental Health Screen ([BJMHS] Steadman and Naples (Citation2005)) in Melbourne, Victoria, and found that 15 percent of detainees surveyed were receiving psychiatric medication prescribed by a physician while incarcerated. In another study, Forsythe and Gaffney (Citation2012) used the CMHS to estimate the prevalence of mental disorders in the DUMA program. They found that almost half of all detainees were likely to be experiencing a diagnosable mental health disorder at the time of arrest. Butler and Allnut (Citation2003) screened detainees in New South Wales using the Composite International Diagnostic Interview (CIDI-A) to measure 1-month and 12-month prevalence rates of psychiatric disorders with prevalence rates estimated to be 76 and 84 percent, respectively. However, each of these investigations was carried out in isolation, and the results from one-off investigations are potentially not generalizable to different criminal justice populations across Australia.
The Corrections Mental Health Screen (CMHS)
The CMHS was originally designed in the United States following a comprehensive study of undetected psychiatric impairment among samples of incarcerated adults (Ford et al. Citation2007). From a battery of 56 items there emerged two gender-specific screening tools – a 12-item instrument for adult males (CMHS-M) and a smaller, eight-item instrument for adult women (CMHS-F). Separate diagnostic interviews were used to validate the instruments and demonstrate their ability to correctly predict (predictive accuracy) current Axis I psychiatric disorders with greater precision than existing instruments (i.e., incremental validity). In this original development study, a predictive accuracy between 83 and 100 percent was achieved (Ford et al. Citation2007). The 12 items that make up the CMHS-M are listed in . Each item is coded dichotomously (yes/no) and a selection of cut-scores were nominated by the authors depending on the intended use of the instrument (Ford et al. Citation2007, Citation2009). As a baseline, a score of six or more affirmative responses (out of 12) was recommended as the optimal point of sensitivity (accurate prediction of true positives) and specificity (accurate prediction of true negatives).
Table 1. CMHS-M Items and questions.
Two years later, a second validation study was conducted in which Ford et al. (Citation2009) reported again on the CMHS-M and CMHS-F. This time, 990 incarcerated inmates were randomly selected and interviewed at reception to jail in the US. Comprehensive diagnostic interviews with a random subsample were later used for validation purposes and the results indicated predictive accuracy rates for Axis I and Axis II psychiatric disorders of between 73 and 80 percent – lower than in the original study, but still exceeding the outcomes of other similar jail-based brief mental health screening instruments.
Though not a validation study, one of the few contemporary applications of the CMHS was in the Australian DUMA program when the male and female instruments were implemented as a method for estimating the prevalence of psychiatric and mental health disorders among the Australian police detainee population. Elsewhere, Forsythe (Citation2013) provides a comprehensive discussion arguing in favour of the CMHS as a more appropriate custodial-based alternative to the K-10 which was twice implemented in the DUMA study. The reasons for favouring the CMHS included: (1) in addition to its validation with incarcerated populations, it was designed to be administered by non-clinical staff; (2) it was designed to also identify previously undiagnosed or detected disorders, meaning that it had the capacity to measure unmet mental health need; (3) the questions were relatively neutral and not likely to lead to the level of upset or confusion that had been previously reported by DUMA interviewers with the K10; and (4) there was a male and female instrument which, in light of contemporary studies, seemed appropriate for the DUMA study (Forsythe Citation2013, 11).
Classical Test Theory (CTT), Item Response Theory (IRT) and the CMHS-M
The development and analysis of the CMHS has, to date, relied on procedures broadly classified under the umbrella of Classical Test Theory (CTT). Though widely used, CTT is a philosophy of measurement which situates the test (not the items or the respondents) as the principal focal point, and this results in several limitations. The first is an over-reliance on Cronbach's alpha as evidence of unidimensionality. In fact, Cronbach's alpha only measures internal consistency, which is necessary but not a sufficient condition for unidimensionality (Cortina, Citation1993). Second, circular dependency is another widely recognised problem of CTT because the quality of a scale or screening tool is determined by the strength of the covariance of the individual item-responses, yet the individual item-responses are, at the same time, dependent on the quality of the instrument (Dodeen Citation2004; Fan Citation1998). Third, a related limitation of CTT is the problem of item equivalence, which emerges when the total sum of the manifest items are used to approximate the underlying latent trait (Streiner Citation2010). This summative approach assumes that all manifest variables perform equally well across the distribution of the latent trait and, therefore, deserve equal weight in the measurement model. Consequently, a sample’s observed and true scores are said to be linearly dependent and the scale’s measurement error is assumed to be equal across all levels of the latent trait-two assumptions which are rarely met in practice because on many scales the manifest items often have varying degrees of importance and difficulty.
IRT, by contrast, quantifies the relative degree of measurement precision across different levels of the latent trait and has emerged over the last 50 years as a complement (not an alternative) to traditional CTT procedures (Dodeen Citation2004; Fan Citation1998). Contemporary applications of IRT have grown rapidly, as computational capacities have increased to accommodate the complex mathematical formulae at its foundation. This technical sophistication also offers several distinct advantages, not least of which is that it makes no assumption about item-equivalence or the equality of measurement, and so can be used to determine the relative reliability of a scale across the full range of the trait that is being measured (Streiner Citation2010). Similarly, IRT provides item-level data that are not sample or context dependent (Dodeen Citation2004; Fan Citation1998). Therefore, IRT provides useful insight into how informative each item is (the item differentiation parameter) and where across the full range of the latent trait each item is most influential (the item difficulty parameter). For these reasons, IRT has emerged as a theoretically grounded approach to the development of scales and tests – a method which models the probabilistic distribution of responses at the item level, rather than in CTT where the primary focus is on test or scale-level information.
Mental health and antisocial behaviour – single or separate constructs?
Perhaps the two most important issues for CTT, as it relates to this study, is the assumption of unidimensionality and the implication that, quantitatively, each item contributes equally to measurement of latent mental health. In the summative use of the CMHS-M, for example, this means that two individuals will be assessed as having an equal level of mental health disorder, so long as they endorse the same number of items (but not necessarily the same items). Imagine, however, that these two respondents each endorsed four items, but the first respondent endorsed four items that are direct measures of psychiatric symptomology, while the second endorsed four items which measure antisocial conduct, lack of empathy, impulsivity, and low self-control. If the scale does not represent true conceptual unidimensionality (as opposed to just quantitative unidimensionality) there is a risk of significant measurement error and although IRT goes some way to addressing this item imbalance it still assumes unidimensionality.
In the case of the CMHS-M, there is a mix of manifest items. Read carefully, some measure the personal experience of moods, feelings, and worries, including the historical experience of, or affective response to, past traumatic events. One item measures a respondent’s actual experience of psychiatric hospitalisation, while others tap into the potential behavioural consequences of an acute or chronic psychiatric experience. Several items are less specific to mental health symptomology but measure more general behavioural experiences which are elsewhere recognised as correlates of crime. Lack of empathy (see Jolliffe and Murray Citation2012), impulsivity and low self-control (see the meta review by de Ridder et al. Citation2012; Gottfredson and Hirschi Citation1990) conscientiousness and agreeableness (Jones, Miller, and Lynam Citation2011), for example, are each measured in the CMHS-M without specific connection to a prior or current psychiatric experience. Some of the specific items imply behavioural dysfunction in the workplace or in personal relationships and there is a long history of criminological theory and research that would identify these as analogous behaviours (Gottfredson and Hirschi Citation1990) and predict a high prevalence in criminal justice populations, with or without other underlying mental health symptoms.
This is not to say that these traits are not experienced by people with acute or chronic mental health problems, or that they aren’t highly correlated with other mental health indicators. Indeed, they often are. Instead, we argue that in a criminal justice setting there is a risk that mental health scales such as the CMHS-M risk confounding these general psychological traits with other indicators of poor mental wellbeing. The risk of this is that in a unidimensional framework an offender who endorses all non-specific antisocial behaviours and traits will be quantitatively assessed as having the same latent mental health as their peers who endorsed an equal number of items measuring actual psychiatric symptoms. This is a potentially important source of measurement error which could confound the screening of mental health and the estimation of its correlation with crime.
Current study
In the current study we explore the test- and item-level properties of the CMHS-M, as administered in the Australian DUMA study with a sample of 620 adult male police detainees. We are motivated by three research questions: (1) are all 12 items on the CMHS-M sufficiently informative to justify their continued use in the Australian context? (2) does a two-dimensional model provide a superior fit to the response patterns in the CMHS-M data?; and (3) how does the two-dimensional measurement of mental health affect substantive conclusions about the correlation between mental health, crime, race, and age?
This cross-cultural comparative analysis of the CMHS-M is the first of its kind and we expect to find some general and local differences which require further conceptual analysis. Specifically, we believe that there is an important conceptual overlap between the general behavioural traits that have been used to characterise antisocial or deviant behaviour, and those which are used in the CMHS-M to screen for probable psychiatric disorder. In criminal justice populations, those items which capture such traits as impulsivity and low self-control may confound the unidimensional assumption. The consequence is a potential over-estimation of mental health disorder and its correlates (demographic and criminal history) in criminal justice settings. We predict that the CMHS-M data will be more effectively modelled under a two-dimensional framework where antisocial behaviour is a construct that is correlated with, but best measured separately from, mental health and psychiatric symptomology. We also predict that the failure to account for this multidimensionality can lead to estimation errors that can affect the substantive conclusions made about the nexus between mental health and crime.
Methodology
Data and measures
We draw our data from the AIC’s DUMA study (Australian Institute of Criminology Citation2015). The main methodological components of DUMA were modelled from the original Arrestee Drug Abuse Monitoring (ADAM) program in the United States (Golub and Johnson Citation2002). DUMA combines an interviewer-administered self-report survey with voluntary urinalysis and has operated quarterly or biennially since 1999. DUMA collects criminal justice information and alcohol and drug use self-report data along with a set of quarterly addendum that cover an issue(s) of policy importance (Makkai and McGregor Citation2001). Participation in the DUMA study was voluntary and informed consent procedures, together with the research instrument, were regularly reviewed and approved by the AIC’s Human Research Ethics Committee. Access to the data for the purposes of this study has been facilitated by the Australian Data Archive. In this study, we draw on the self-report data collected from eight police stations and watch-houses in the fourth quarter of 2010. During this time 620 adult male detainees agreed to be interviewed and completed all questions of the CHMS (77% of all eligible detainees).
A descriptive summary of these data, including the univariate means and bivariate polychoric correlations for each CMHS-M item, are provided in . The internal consistency of the 12 items was reasonably strong, yielding a Kuder-Richardson (KR20) coefficientFootnote2 of 0.82. For the correlates of mental health presented later in this paper, race is measured dichotomously as Indigenous and non-Indigenous based on self-reported identification. Indigenous detainees are those who identified as either Aboriginal or Torres Strait Islander. Age is also self-reported and dichotomised into two groups of young adult offenders (aged 18–20 years) and all other offenders aged 21 years or over. Prior criminal history is a linear continuous variable measuring the number of charges received in the past 12 months (not including the current charges for which the detainees was in custody). Some detainees had no recent criminal history, while the maximum number of prior charges was 117 ( = 3.1, sd = 9.4). Finally, prior imprisonment was self-reported and is measured dichotomously for those who had been incarcerated at least once in the past 12 months, zero if otherwise.
Table 2. Descriptive statistics, correlations and IRT model outcomes.
Analytical approach
Our approach to the analysis was divided into three stages. First, we used a generalised latent variable model to estimate item discrimination and difficulty parameters for each of the CMHS-M’s 12 manifest items. Our approach is analogous to an Item Response Theory model and was implemented in Stata v15 (StataCorp Citation2017). As is standard practice, we commenced our modelling with the most parsimonious specification (a single parameter Rasch model (Rasch Citation1980)) and then tested the viability of a two-parameter model using a nested model Likelihood Ratio test.Footnote3 The composition of the CMHS-M and the contribution of each item was explored using graphical representations of the IRT model as item characteristic curves (ICCs, ) and the test Information Function (TIF) ().
Figure 1. 1(a)-(b): Item Characteristic Curves Test Information Function (b), CMHS-M.
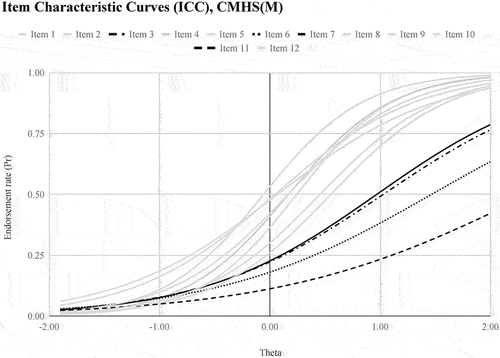
Figure 2. Test Information Function, CMHS-M. Source: Australian Institute of Criminology, DUMA [Computer File].
![Figure 2. Test Information Function, CMHS-M. Source: Australian Institute of Criminology, DUMA [Computer File].](/cms/asset/3c7dcd11-78db-4c25-91f0-8db8a124d565/rjcj_a_2174577_f0002_b.gif)
In the second stage, a two-dimensional model (see De Boeck and Wilson Citation2004) was estimated using a generalised structural equation framework and the model fit was compared to the unidimensional model using the Akaike Information Criteria (AIC), the Bayesian Information Criteria (BIC) and a Likelihood Ratio test. A comparison of two latent mental health specifications was conducted post-hoc using the linear prediction of each latent variable. A Pearson correlation coefficient compared the linear correlation, while Gwet’s AC1 coefficient of agreement was used to test the concordance of their quartile categorisation.
In the final stage of the analysis, we explore the univariate correlations between latent mental health and two demographic (race and age) and two criminal history (frequency of recent offending and recent imprisonment) covariates. Each covariate is modelled against three alternative operationalisations of the CMHS-M: the simple summative score, the unidimensional latent prediction, and the two-dimensional latent prediction. Standardised (beta) coefficients are compared both in terms of size and direction of change.
Results
The two-parameter logistic IRT model is provided in the final two columns of . The single-parameter assumption of equal discrimination is rejected by the Likelihood Ratio test, confirming a preference for the model in which item difficulty (parameter b) and discrimination (parameter a) can vary for each item (X2(11) = 74.33, p < .001). This preference is also confirmed by an improvement in the Comparative Fit Index, from 0.94 in the (single-parameter) Rasch model to 0.96 the two-parameter model. Looking first at the difficulty parameters, we note that the majority of the 12 items are clustered around the value of zero. To interpret this, we must first recall that each of the 12 items of the CMHS are manifest indicators of a theoretical (criminal justice) population distribution of psychiatric disorder. This population distribution is not observed, but is estimated from the response patterns and probabilities of the 12 items. Essentially, mental disorder is an unmeasured latent construct which has mean of zero and a variance of one. Zero is the point which divides these detainees into two groups: those with above average mental disorder and those with below average. At the individual item level, the difficulty parameter represents the point in the distribution of latent mental disorder above which 50 percent of the detainees are predicted to answer ‘yes’. Like in any scale or test, some items are ‘harder’ and less likely to be endorsed unless the respondent has high levels of mental disorder. Other items are ‘easier’ or more commonly endorsed by detainees even if their latent mental disorder is low. In our data, all but four items have a difficulty parameter that falls within a relatively narrow range. The four remaining items appear somewhat anomalous. Item 11, for example, has an estimated difficulty parameter of b = 2.29. This indicates that it is rare for this item to be endorsed, even for detainees with considerably high rates of latent mental health disorder.
By itself, item difficulty is not a major concern for the development of tests and scales. Indeed, most tests will likely include questions that are somewhat harder or easier than others and this is an important feature if a test is designed to have predictive accuracy across the full range of the latent variable. In the case of the CMHS-M, however, where dichotomised triaging and screening are the principal objective, it may be more important for the scale to be very informative (and more accurate) within a narrow range and so very difficult or very easy items may be of limited utility. For this reason, further exploration of items 3, 6, 7, and 11 is warranted, since these items are unlikely to be affirmed even at high levels of the latent trait.
The differentiation parameters (parameter a) are more important in the context of this analysis. Specifically, item-level discrimination parameters in a two-parameter model reflect how well each item discriminates between detainees across the population distribution of latent mental disorder. Put simply, items with low discrimination (depicted by logistic functions with a shallow gradient) are said to provide less information to the scale because they are less highly correlated with the underlying latent trait. Conversely, items with high discrimination (depicted by a steep logistic function) are very informative at the point of difficulty since the probability of endorsement changes rapidly across a narrow band of the latent trait. Using the analogy of a test designed to measure a student’s math ability, ‘good’ questions have high discrimination because they are rarely answered correctly by students with a latent level of math ability that is below the item’s difficulty parameter. Poorly designed questions have low discrimination because the shallow logistic function suggests that a correct answer could likely be given by students with a much wider range of ability in math. Where the objective is to differentiate students based on their competence in math, a test comprised of multiple questions with high discrimination and variable difficulty would be important. For the present study, having low discrimination means that the manifest variable may be endorsed even if latent mental disorder is relatively low, or not endorsed even if latent disorder is high.
For the CMHS-M, all 12 items are statistically associated with latent mental disorder. The endorsement of each provides a better prediction of latent mental disorder than simply flipping a coin, however not all items are equally useful or informative. The most informative were Item 2 (a = 2.33), Item 1 (a = 2.27) and Item 4 (a = 2.14); the least informative were Item 11 (a = 0.87), Item 6 (a = 1.01), Item 7 (a = 1.25), Item 3 (a = 1.25) and Item 5 (a = 1.38).
To aid our interpretation of the difficulty and discrimination parameters, presents the ICCs for the unconditional two parameter IRT model. These ICCs are easily interpreted as logistic regression curves. The relative position of each ICC reflects its difficulty parameter; its gradient represents the discrimination parameter. Here, a visual inspection reveals the anomalous nature of items 11, 6, 7 and 3 (bolded), each being both more difficult and less discriminatory of the underlying latent disorder that is to be operationalised by the CMHS-M. The Test Information Curve (TCC, ) shows the relative informativeness and accuracy of the overall scale, where the peak in the TCC represents the location on the latent construct where the difficulty parameter of the majority of items coalesce.
We note that for the two-parameter model the overall item fit statistics tell a mixed story. The approximated CFI is acceptable (CFI = 0.96), but the limited information test, M2 (see Maydeu-Olivares and Joe Citation2006), suggests that there are items whose response probabilities are not well predicted by the latent trait (M2 = 185.5, p < 0.001). Evidence of this misfit can be seen in the relatively weak discrimination of some items in the CMHS-M and raises an important question about whether CMHS-M screen is operationalising a single unidimensional latent trait, as is implied by its use as a summative triaging tool, or some multidimensionality that is worth exploring. To investigate this, we return to the question wording in and conclude that items 3, 5, 6, and 7 are behavioural indicators which operationalise general psychological or antisocial traits rather than specific psychiatric symptoms. We note that all four items were among the least informative in the two-parameter unidimensional IRT model and suggest that, perhaps, the CMHS-M functions more efficiently on two dimensions rather than one.
To test this, we model the data using two correlated latent variables: MW (mental wellbeing) and AB (general antisocial behavioural traits) (see ). We then compare the statistical fit of the two-dimensional model against the original unidimensional specification from . The results show a superior fit on all criteriaFootnote4. The two-dimensional specification produced a lower AIC (8086.6 vs. 8122.7) and BIC (8197.3 vs. 8229.0) and the degree of improvement was statistically significant (LR X2(2) = 39.53, p < .001). We also reproduce the limited information statistic, CFI, for both the MW and AB domains and confirm that these models produce an improved fit to the underlying data (MW CFI = 0.98, AB CFI>0.99). Finally, we compared the latent mental health scales () that were predicted from the response patterns of the unidimensional and two-dimensional models. The two latent variables were highly correlated (p = .9972) and, when classified into quartiles, had a very high agreement rate (Gwet’s AC1 = 92.19). Still, some offenders had lower mental health scores in the two-dimensional model than would have been otherwise estimated.
Table 3. Unidimensional and Two-dimensional model diagnostics and comparative outcomes.
Our final question was whether this new measure of latent mental health, as derived from the two-dimensional model, is likely to alter our understanding of the demographic and criminal history correlates of mental health in the criminal justice system. presents the standardised univariate coefficients for two demographic and two criminal history covariates by each of the three models of mental health. The second column is the unadjusted summative score of the CMHS-M. The third and fourth columns are the predicted distributions of latent mental health as measured by the unidimensional and two-dimensional models, respectively. Standardised coefficients are used so that the relative effect size of each covariate can be compared between separately estimated regression models. The results indicate that for the simple summative score of the CMHS-M, the average score for Indigenous offenders was significantly higher than for non-Indigenous offenders (beta = 0.094, p = 0.02). The same was true for the unidimensional model, though to a lesser extent (beta = 0.084, p = 0.04). Importantly, the difference between Indigenous and non-Indigenous offenders diminishes further in the two-dimensional model (beta = 0.076, p = 0.06) and was no longer statistically significant. An equality of coefficients test shows that the coefficient differences between Model 1 and Model 2 (X2(1) = 6.17, p < 0.05) and between Model 2 and Model 3 (X2(1) = 7.69, p < 0.05) were statistically significant. By age, the youngest offenders (aged 18–20 years) scored significantly lower on all three mental health measures, when compared to offenders aged 21 years or older. However, the relative effect size was highest in the two-dimensional model (−0.121, p<=.001), a coefficient difference (between Model 2 and Model 3) that was statistically significant (X2(1) = 9.01, p < 0.05). This suggests that the age effect is underestimated when general antisocial traits are not modelled separately. With regard to the criminal history covariates, both prior imprisonment and the frequency of prior offending are significantly correlated with all three measures of mental health. However, in both cases the effect size was higher when correlated with the summative and unidimensional measures of mental health. Perhaps the most notable result here is that the correlation with the frequency of prior offending diminishes from what might be qualitatively described by Goulden (Citation2006) as a ‘moderate’ (beta = 0.111, p < .001) to ‘small, but meaningful’ (beta = 0.086, p = 0.04). The difference between the coefficients was also statistically significant (X2(1) = 15.45, p < 0.05).
Table 4. Univariate demographic and criminal history covariates of three measures of mental health (standardised coefficients).
Discussion
At the outset of this study, we remarked that the CMHS-M had not been the subject of any detailed item-level analysis, nor any cross-cultural analysis, despite having appeared in several key Australian studies of mental health among police detainees. This raised several questions about the measurement of mental health in the criminal justice system and, more importantly, the conclusions that have since been drawn about the nexus between mental health, crime, and their demographics correlates. As McNemar aptly noted, ‘all measurement is befuddled by error’ (McNemar Citation1946, 249). If left unchecked, this error may have considerable implications for the theories we build and the policies we seek to influence with our data and models. We conclude this analysis with evidence that the CMHS-M is not a unidimensional scale, despite its strong internal consistency and its intended use as a summative triaging tool in the criminal justice sector. Although its multidimensionality may not have significant ramifications for the crude pre-screening of probable mental health disorders, it is likely to have important implications for its use (and the use of other similar tools) in operationalising mental health prevalence and its correlates.
Our conclusions are supported by an iterative IRT modelling procedure. IRT is an increasingly popular complement to CTT which yields new insights into the item-level characteristics of latent variable models and scales that have been constructed using traditional indicators of interval consistency such as Cronbach's alpha. Compared to CTT, IRT offers several advantages. Specifically, IRT does not assume the equality of measurement precision between individuals across all levels of the latent trait, but instead allows measurement precision to vary at different levels of the latent trait and depending on the order in which items are endorsed. In this study, the IRT analysis revealed that despite acceptable internal consistency (alpha = 0.82), four items on the CMHS-M (Items 3, 5, 6 and 7) likely confound the measurement of mental wellbeing with general personality and antisocial traits such as the lack of empathy, impulsivity and low-self-control. For a general population sample, these traits may indeed be highly correlated with poor mental health, not because they are solely indicative of mental health problems per se, but because they often are symptomatic of such. We argue that the potential for measurement error is likely to be more highly concentrated in criminal justice populations because impulsivity and low self-control are traits that are more prevalent (Caspi et al. Citation1994; Moffitt et al. Citation2011) and not necessarily symptomatic of psychiatric disorder. Gottfredson and Hirschi (Citation1990), in their General Theory of Crime, situate the population distribution of low self-control as the single most important explanation for individual and aggregate level crime rates. They make no specific claims about low self-control being a consequence or symptom of an underlying mental health problem, but they do predict low levels of self-control among those who are prolific or imprisoned offenders. Elsewhere in psychology, self-control has been used to represent a dizzying array of trait-like behaviours, such as the ability to exert control over, or supress thoughts, temptations and urges. As an organising framework, self-control has been broadly classified as representing ‘an individual’s capacity to actively exert control over impulsive responses’ (Hagger, Zhang, Kangro, et al. Citation2021) and is generally applicable in a wide arrange of behavioural domains. Importantly, there is mixed evidence of the relationship between trait self-control and psychiatric disorder. It is true that the two phenomena can co-occur in psychiatric populations, but low self-control is a sufficient but not a necessary condition of mental health disorder (Billen et al. Citation2019; Kim, Richards, and Oldehinkel Citation2022).
Consequently, we investigated a two-dimensional model of the CMHS-M data which allows a general antisocial behavioural dimension to be modelled as a separate but correlated construct. Consistent with our prediction, the two-dimensional treatment of these data provided a better fit and produced a measure of latent mental health that was different in a number of important ways. Specifically, we find that the difference between Indigenous and non-Indigenous offenders is likely over-estimated when mental health scales include manifest items that are general measures of antisocial personality or behaviour. In fact, the racial differential changed from statistically significant to non-significant: an important finding in a country where Indigenous Australians are among the world’s most over-incarcerated minority population (Australian Bureau of Statistics Citation2022). Similarly, and as predicted, the relative gap between young offenders and older offenders increased when antisocial behaviour was modelled as a separate construct, while the correlation between mental health and the frequency of prior offending was reduced from ‘moderate’ to ‘small’.
To be sure, these changes in effect size were small and this was not unexpected given that the two-dimensional model, although a better fit to the data, still produced a measure of latent mental health that was mostly concordant (in terms of the relatively ordering of offenders) with its unidimensional alternative. That said, by extracting the antisocial behavioural items into a second construct and allowing it to correlate with latent mental health, we have reduced the potentially spurious influence of past criminal behaviour. This appears to have an important influence on the conclusions one might draw about racial differences in mental health, as well as the apparent strength of the relationship between mental health and the frequency of recent offending. These differences are qualitatively important as the unadjusted findings might have pointed to the need for specific mental health interventions with Indigenous people or, worse still, continued to fuel misperceptions about the strength of the relationship between mental health and offending frequency. For critical scholars, these data reaffirm that measurement error is not ignorable, and that cross-cultural comparative analysis is essential to help shape the policy and public discourse about mental health and crime.
Notwithstanding the superior fit of a two-dimensional model for the CMHS-M, there is still some evidence of misfit. Specifically, while the Comparative Fit Indices are superior, the global goodness of fit statistic for the MW scale still suggests there is room for improvement. In our view, item 11 requires separate clarification as, this item measures a respondent’s experience of psychiatric hospitalisation and has a comparatively low rate of endorsement, even among those with high levels of latent mental disorder. It seems that for these Australian detainees, the experience of psychiatric hospitalisation is relatively rare and not an experience that is highly correlated with the severity or level of one’s latent disorder. We suggest this finding is likely a methodological consequence of the use of the term ‘psychiatric hospital’ in a jurisdiction where there are few private psychiatric hospitals (68 nation-wide, see Australian Institute of Health and Welfare Citation2022) and where the self-reported admission of past hospitalisation may be considered socially undesirable. It may also speak to the relative differences between the health and mental health care systems of the two countries. To be sure, the Australian and US systems differ considerably in both the structure and complexity of their public and private health insurance arrangements. In Australia, for example, the cost of most mental health related general practitioner, psychologist, and specialist consultations are reimbursed through the national health insurer, Medicare (McIntyre et al. Citation2017), and this may provide for a significant early intervention and treatment opportunity. Relative to the US, this may limit the prevalence of both voluntary and involuntary overnight separations and long-stay hospitalisations.
Of course, it remains to be seen whether a two-dimensional specification of the CMHS-M would improve the instrument’s predictive validity and reliability against clinical diagnoses. Unfortunately, the DUMA study does not collect any external diagnostic indicators against which these alternative specifications can be compared. Further, while the DUMA data is the most comprehensive sample of police detainees available in Australia, it is not a random sample of the Australian police detainee or criminal justice population. Therefore, the IRT parameters and latent variables estimated in this study may not be generalizable to broader criminal justice populations. Notwithstanding these limitations, our results have demonstrated the necessity of cross-cultural replication and future work is needed to explore the implications of these findings in further detail.
We conclude with some thoughts about the practical and policy implications of this research. Specifically, we have argued strongly in favour of cross-cultural comparative analysis, especially because the US-developed and designed CMHS-M, in its original formulation, likely misrepresents the difference between racial groups in the Australian criminal justice system. This happens to be a difference in the estimated mental wellbeing of First Nations Australians in police custody and suggests that the level of mental disorder would be incorrectly estimated (as higher), and flagged as a statistically significant, but for the evidence that this cross-cultural analysis has provided. Whether this finding would generalise to First Nations or other minority racial population in other countries or jurisdictions remains to be seen, however, our results foretell of the risks we take in simply adopting and using instruments designed out of a local context. In some respects, we have avoided making any specific predictions about the generalisability of this finding to other countries for precisely the same reason we believe this Australian analysis is important. We suspect, however, that the inclusion of questions about antisocial behaviour and interpersonal conflict will likely have similar implication elsewhere – even in the US where the scale was developed.
To be fair, the difference between models (in terms of the standardised parameters) are small – some might say too small to be of practical use in real world application. This is true. However, we reflect upon the apparent importance and weight given to ‘statistically significant’ findings, especially when it comes to prioritising resources in the criminal justice setting. That the best fitting two-dimensional model changes the difference between Aboriginal and non-Aboriginal detainees from statistically significant to non-statistically significant should not be dismissed, even if the substantive difference is small. There is a long history of critical scholarship that has pointed to the power of statistics to perpetuate racial stereotypes, often by showing statistically significant differences between racial groups using poorly developed instruments that reify racial prejudice (see, for example, Drouhot and Garip Citation2021; Lever Citation2009). This paper is but a first step in the necessary Australian journey of examining internationally developed instruments and tools in criminal justice research. We suggest other countries follow suit.
Disclosure statement
No potential conflict of interest was reported by the authors.
Notes
1. Butler et al. (Citation2006) compared 12-month prevalence estimates of psychiatric morbidity between arrestee and community samples. The prevalence for the community sample was 31%, compared to 80% within the arrestee sample.
2. The KR20 coefficient is a generalised equivalent to Cronbach’s alpha for binary items.
3. We have not included a scaling constant (typically D = 1.7). In brief, scaling constants are sometimes (though much less frequently today) used to transform the logistic ogive to the normal ogive so that logistic IRT parameters are comparable on a single, normal, scale. Camilli (Citation2017) provides a simulation study which shows that scaling does not, in fact, reproduce unbiased estimates and has shown that the best choice of scaling is to not scale at all.
4. We also explored these data using a principal components analysis of the polychoric correlations presented in . The PCA analysis confirmed the presence of two factors, which together explained 60 percent of the variance in the data. As expected, items 3, 5, 6 and 7, loaded onto a second factor while all other items loaded onto the first.
References
- Alden, A., P. Brennan, S. Hodgins, and S. Mednick. 2007. “Psychotic Disorders and Sex Offending in a Danish Birth Cohort.” Archives of General Psychiatry 64 (11): 1251–1258. doi:10.1001/archpsyc.64.11.1251.
- Andersen, H. S. 2004. “Mental Health in Prison Populations. A Review – with Special Emphasis on a Study of Danish Prisoners on Remand.” Acta psychiatrica Scandinavica 110 (s424): 5–59. doi:10.1111/j.1600-0447.2004.00436_2.x.
- Arseneault, L., T. E. Moffitt, A. Caspi, P. J. Taylor, and P. A. Silva. 2000. “Mental Disorders and Violence in a Total Birth Cohort: Results from the Dunedin Study.” Archives of General Psychiatry 57 (10): 979–986. doi:10.1001/archpsyc.57.10.979.
- Australian Bureau of Statistics. (2022). Prisoners in Australia. Retrieved from https://www.abs.gov.au/statistics/people/crime-and-justice/prisoners-australia/2021#post-release-changes
- Australian Institute of Criminology. (2015). About DUMA. Retrieved from http://aic.gov.au/about_aic/research_programs/nmp/duma/about.html
- Australian Institute of Health and Welfare. 2019. The Health of Australia’s Prisoners 2018. Canberra: Australian Institute of Health and Welfare.
- Australian Institute of Health and Welfare. (2022). Mental Health—Psychiatric Facilities in Australia. Australian Institute of Health and Welfare.https://org/aihw.gov.au/mental-health/topic-areas/facilities
- Baksheev, G. N., S. D. Thomas, and J. R. Ogloff. 2010. “Psychiatric Disorders and Unmet Needs in Australian Police Cells.” Australian & New Zealand Journal of Psychiatry 44 (11): 1043–1051. doi:10.1080/00048674.2010.503650.
- Billen, E., C. Garofalo, J. K. Vermunt, and S. Bogaerts. 2019. “Trajectories of Self-Control in a Forensic Psychiatric Sample: Stability and Association with Psychopathology, Criminal History, and Recidivism.” Criminal Justice and Behavior 46 (9): 1255–1275. doi:10.1177/0093854819856051.
- Butler, T., and S. Allnut. 2003. Mental Health Among NSW Prisoners. Retrieved from Sydney: NSW Corrections Health Service.
- Butler, T., Andrews, G., Allnutt, S., Sakashita, C., Smith, N. E., & Basson, J. (2006). Mental disorders in Australian prisoners: a comparison with a community sample. Australian & New Zealand Journal of Psychiatry, 40(3): 272–276.
- Butler, T., D. Indig, S. Allnutt, and H. Mamoon. 2011. “Co-Occurring Mental Illness and Substance Use Disorder Among Australian Prisoners.” Drug and Alcohol Review 30 (2): 188–194. doi:10.1111/j.1465-3362.2010.00216.x.
- Camilli, G. 2017. “The Scaling Constant D in Item Response Theory.” Open Journal of Statistics 7 (05): 780. doi:10.4236/ojs.2017.75055.
- Caspi, A., T. E. Moffitt, P. A. Silva, M. Stouthamer-Loeber, R. F. Krueger, and P. S. Schmutte. 1994. “Are Some People Crime-Prone? Replications of the Personality-Crime Relationship Across Countries, Genders, Races, and Methods*.” Criminology 32 (2): 163–196. doi:10.1111/j.1745-9125.1994.tb01151.x.
- Chang, Z., H. Larsson, P. Lichtenstein, and S. Fazel. 2015. “Psychiatric Disorders and Violent Reoffending: A National Cohort Study of Convicted Prisoners in Sweden.” The Lancet 2 (10): 981-900. doi:10.1016/S2215-0366(15)00234-5
- Cortina, J. M. 1993. “What is Coefficient Alpha? An Examination of Theory and Applications.” The Journal of Applied Psychology 78 (1): 98.
- De Boeck, P., and M. Wilson, edited by. 2004. Explanatory Item Response Models: A Generalized Linear and Nonlinear Approach Vol. 10. New York: Springer.
- de Ridder, D., G. Lensvelt-Mulders, C. Finkenauer, F. Marijn Stok, and R. Baumeister. 2012. “Taking Stock of Self-Control: A Meta-Analysis of How Trait Self-Control Relates to a Wide Range of Behaviors.” Personality and Social Psychology Review 16 (1): 76–99. doi:10.1177/1088868311418749.
- Dodeen, H. 2004. “The Relationship Between Item Parameters and Item Fit.” Journal of Educational Measurement 41 (3): 261–270. doi:10.1111/j.1745-3984.2004.tb01165.x.
- Drouhot, L. G., and F. Garip. 2021. “What’s Behind a Racial Category? Uncovering Heterogeneity Among Asian Americans Through a Data-Driven Typology.” RSF: The Russell Sage Foundation Journal of the Social Sciences 7 (2): 22–45. doi:10.7758/rsf.2021.7.2.02.
- Fan, X. 1998. “Item Response Theory and Classical Test Theory: An Empirical Comparison of Their Item/Person Statistics.” Educational and Psychological Measurement 58 (3): 357–381. doi:10.1177/0013164498058003001.
- Ford, J. D., R. L. Trestman, V. Wiesbrock, and W. Zhang. 2007. “Development and Validation of a Brief Mental Health Screening Instrument for Newly Incarcerated Adults.” Assessment 14 (3): 279–299. doi:10.1177/1073191107302944.
- Ford, J. D., R. L. Trestman, V. Wiesbrock, and W. Zhang. 2009. “Validation of a Brief Screening Instrument for Identifying Psychiatric Disorders Among Newly Incarcerated Adults.” Psychiatric Services 60 (6): 842–846. doi:10.1176/ps.2009.60.6.842.
- Forsythe, L. 2013. Measuring Mental Health in Criminology Research: Lessons from the Drug Use Monitoring in Australia Program. Retrieved from Canberra: Australian Institute of Criminology.
- Forsythe, L., and A. Gaffney. 2012. “Mental Disorder Prevalence at the Gateway to the Criminal Justice System.” Trends and Issues in Crime and Criminal Justice(438)(438) 438: 1–8.
- Golub, A., and B. D. Johnson. 2002. “Arrestee Drug Abuse Monitoring (ADAM) Program.” In Encyclopedia of Crime and Punishment, edited by D. Levinson, 63–65. Thousand Oaks, California: SAGE Publications, Inc.
- Gottfredson, M. R., and T. Hirschi. 1990. A General Theory of Crime. Redwood City: Stanford University Press.
- Goulden, K. J. 2006. “Effect Sizes for Research: A Broad Practical Approach.” Journal of Developmental & Behavioral Pediatrics 27 (5): 419–420. doi:10.1097/00004703-200610000-00013.
- Hagger, M. S., C. Q. Zhang, E. M. Kangro, Ries, F., Wang, J.C., Heritage, B. and Chan, D.K. 2021. “Trait Self-Control and Self-Discipline: Structure, Validity, and Invariance Across National Groups.” Current Psychology 40 (3): 1015–1030. doi:10.1007/s12144-018-0021-6.
- Hewson, T., A. Shepherd, J. Hard, and J. Shaw. 2020. “Effects of the COVID-19 Pandemic on the Mental Health of Prisoners.” The Lancet Psychiatry 7 (7): 568–570. doi:10.1016/S2215-0366(20)30241-8.
- Hobbs, M., K. Krazlan, S. Ridout, Q. Mai, M. Knuiman, and R. Chapman. 2006. Mortality and Morbidity in Prisoners After Release from Prison in Western Australia 1995–2003. Retrieved from Canberra: Australian Institute of Criminology.
- Johnson, L., K. Gutridge, J. Parkes, A. Roy, and E. Plugge. 2021. “Scoping Review of Mental Health in Prisons Through the COVID-19 Pandemic.” BMJ Open 11 (5): e046547. doi:10.1136/bmjopen-2020-046547.
- Jolliffe, D., and J. Murray. 2012. “Lack of Empathy and Offending: Implications for Tomorrow’s Research and Practice.” In The Future of Criminology, edited by R. Loeber and B. Welsh, 62–69. New York: Oxford University Press.
- Jones, S. E., J. D. Miller, and D. R. Lynam. 2011. “Personality, Antisocial Behavior, and Aggression: A Meta-Analytic Review.” Journal of Criminal Justice 39 (4): 329–337. doi:10.1016/j.jcrimjus.2011.03.004.
- Kariminia, A., M. G. Law, T. Butler, M. Levy, S. Corben, J. Kaldor, and L. Grant. 2007. “Suicide Risk Among Recently Released Prisoners in New South Wales, Australia.” The Medical Journal of Australia 187 (7): 387–390. doi:10.5694/j.1326-5377.2007.tb01307.x.
- Kessler, R. C., G. Andrews, K. J. Colpe, E. Hiripi, D. K. Mroczek, N.S. -L. T …, and A. M. Zaslavsky, A. Zaslavsky. 2002. “Short Screening Scales to Monitor Population Prevalences and Trends in Non-Specific Psychological Distress.” Psychological Medicine 32 (6): 959–976. doi:10.1017/S0033291702006074.
- Kim, Y., J. S. Richards, and A.J.S.C. Oldehinkel. 2022. “Mental Health Problems, and Family Functioning in Adolescence and Young Adulthood: Between-Person Differences and Within-Person Effects.” Journal of Youth and Adolescence 51 (6): 1181–1195. doi:https://doi.org/10.1007/s10964-021-01564-3.
- Lever, A. 2009. “Ethical Issues in Racial Profiling.” Jury Expert 21 (1): 1–9.
- Lindqvist, P., and P. Allebeck. 1990. “Schizophrenia and Assaultive Behaviour: The Role of Alcohol and Drug Abuse.” Acta psychiatrica Scandinavica 82 (3): 191–195. doi:10.1111/j.1600-0447.1990.tb03051.x.
- Loken, E., and A. Gelman. 2017. “Measurement Error and the Replication Crisis.” Science 355 (6325): 584–585. doi:10.1126/science.aal3618.
- Makkai, T., and K. McGregor. 2001. Drug Use Monitoring in Australia (DUMA): 2000 Annual Report on Drug Use Among Police Detainees. Retrieved from Canberra: Australian Institute of Criminology.
- Maydeu-Olivares, A., and H. Joe. 2006. “Limited Information Goodness-Of-Fit Testing in Multidimensional Contingency Tables.” Psychometrika 71 (4): 713–732. doi:10.1007/s11336-005-1295-9.
- McIntyre, C., M. G. Harris, A. J. Baxter, S. Leske, S. Diminic, J. P. Gone, and E. Hunter, H. Whiteford. 2017. “Assessing Service Use for Mental Health by Indigenous Populations in Australia, Canada, New Zealand and the United States of America: A Rapid Review of Population Surveys.” Health Research Policy and Systems 15 (1): 67. doi:10.1186/s12961-017-0233-5.
- McNemar, Q. 1946. “Opinion-Attitude Methodology.” Psychological Bulletin 43 (4): 289. doi:10.1037/h0060985.
- Moffitt, T. E., L. Arseneault, D. Belsky, N. Dickson, R. J. Hancox, H. Harrington, and R. Houts, R. Poulton, B. W. Roberts, S. Ross, M. R. Sears. 2011. “A Gradient of Childhood Self-Control Predicts Health, Wealth, and Public Safety.” Proceedings of the National Academy of Sciences 108 (7): 2693–2698. doi:10.1073/pnas.1010076108.
- Monahan, J., H. J. Steadman, E. Silver, P. S. Appelbaum, P. C. Robbins, E. P. Mulvey, and S. Banks. 2001. Rethinking Risk Assessment: The Macarthur Study of Mental Disorder and Violence. New York: Oxford University Press.
- Ogloff, J., M. Davis, G. Rivers, and S. Ross. 2007. “The Identification of Mental Disorders in the Criminal Justice System.” Trends and Issues in Crime and Criminal Justice(334)(334) 334: 1–6.
- Ogloff, J. R., M. R. Davis, G. Rivers, and S. Ross 2007. The Identification of Menal Disorders in the Criminal Justice System. Retrieved from Canberra: http://citeseerx.ist.psu.edu/viewdoc/download?doi=10.1.1.194.2393&rep=rep1&type=pdf
- Payne, J., and G. Wong. 2018. “Measuring Drug Dependence in Police Custody: An Item Response Theory and Differential Item Function Analysis of UNCOPE in Australia.” Drug and Alcohol Review 37 (7): 856–864. doi:10.1111/dar.12854.
- Rasch, G. 1980. Probabilistic Models for Some Intelligence and Attainment Tests. Originally published Copenhagen: Danmarks Paedagogiske Institut, 1960. expanded ed. Chicago: University of Chicago Press.
- Schneider, L. H., E. J. Pawluk, I. Milosevic, P. Shnaider, K. Rowa, M. M. Antony, and N. Musielak, R. E. McCabe. 2022. “The Diagnostic Assessment Research Tool in Action: A Preliminary Evaluation of a Semistructured Diagnostic Interview for DSM-5 Disorders.” Psychological Assessment 34 (1): 21–29. doi:10.1037/pas0001059.
- Schulte, C., J. Mouzos, and T. Makkai. 2005. Drug Use Monitoring in Australia: 2004 Annual Report on Drug Use Among Police Detainees. Retrieved from Canberra: Australian Institute of Criminology.
- Smith, N., and L. Trimboli. 2010. Comorbid Substance and Non-Substance Mental Health Disorders and Re-Offending Among NSW Prisoners. Retrieved from Sydney: Bureau of Crime Statistics and Research.
- Steadman, H. J., and M. Naples. 2005. “Assessing the Effectiveness of Jail Diversion Programs for Persons with Serious Mental Illness and Co-Occurring Substance Use Disorders.” Behavioral Sciences & the Law 23 (2): 163–170. doi:10.1002/bsl.640.
- Streiner, D. L. 2010. “Measure for Measure: New Developments in Measurement and Item Response Theory.” The Canadian Journal of Psychiatry 55 (3): 180–186. doi:10.1177/070674371005500310.
- StataCorp. 2017. Stata Statistical Software: Release 15. College Station, TX: StataCorp LLC.
- Tozzo, P., G. D’angiolella, and L. Caenazzo. 2020. “Prisoners in a Pandemic: We Should Think About Detainees During COVID-19 Outbreak.” Forensic Science International: Synergy 2: 162–163. doi:10.1016/j.fsisyn.2020.05.004.
- U.S. Department of Justice. (2002). I-ADAM in Eight Countries: Approaches and Challenges. Washington, D.C: U.S. Department of Justice Retrieved from https://www.ncjrs.gov/pdffiles1/nij/189768.pdf.
- White, P., and H. Whiteford. 2006. “Prisons: Mental Health Institutions of the 21st Century?” The Medical Journal of Australia 185 (6): 302–303. doi:10.5694/j.1326-5377.2006.tb00581.x.
- Whiting, D., P. Lichtenstein, and S. Fazel. 2021. “Violence and Mental Disorders: A Structured Review of Associations by Individual Diagnoses, Risk Factors, and Risk Assessment.” The Lancet Psychiatry 8 (2): 150–161. doi:10.1016/S2215-0366(20)30262-5.
- Wildeman, C., and L. H. Andersen. 2020. “Long-Term Consequences of Being Placed in Disciplinary segregation†.” Criminology 58 (3): 423–453. doi:10.1111/1745-9125.12241.