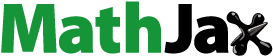
Abstract
Pancreatic cancer (PC) is associated with a high mortality rate. We explored the interindividual variation of cancer outcomes, attributable to DNA methylation, gene expression, and clinical factors among PC patients. We aim to determine whether we could differentiate subjects with greater nodal involvement, higher cancer staging, and subsequent survival. We modeled every response variable as a function of a linear predictor involving the effects of clinical variables, methylation, and gene expression in a Bayesian framework. Our results highlight the overall importance of wide-spread alterations in methylation and gene expression patterns associated with survival, nodal metastasis, and staging.
Introduction
Pancreatic cancer (PC) is a fatal disease originating in the ductal epithelium of the pancreas. Prognosis for patients diagnosed with PC is grave, with 5-year survival rates ranging between 1% and 9% (Citation1,Citation2). Omic signatures have shown to determine specific characteristics of the cancer (Citation3). Specific alterations in DNA methylation and gene expression have been suggested to influence the aggressiveness of PC (Citation4,Citation5). Hypermethylation of CpG islands and promoter regions in PC is linked to transcriptional silencing of tumor suppressor genes, such as CDKN2A/INK4A, TP53, and DPC4/SMAD4 (Citation4,Citation5). Hypermethylation is observed in PC stem cells. Conversely, hypomethylation tends to be associated with over-expression of oncogenes and genomic instability, as evidenced by the activation of the KRAS oncogene (Citation4–6).
Due to the complex nature of PC, it is crucial to understand the omics responsible for the interindividual variation observed in patient survival. Variation could be attributed to DNA methylation and gene expression patterns across the whole genome. While 0% variability would indicate that interindividual variation in omics is disconnected to a patient’s severity of PC and subsequent chance of survival, 100% variability would indicate the interindividual variation in omics is critically related to the patient’s outcome. Differentially methylated genes can create an assortment of phenotypes, which can account for susceptibility to specific diseases and pathogens, and responsiveness to pharmacologic agents or environmental stressors (Citation7). Similarly, variations in gene expression can provide knowledge regarding the molecular aspect of phenotypic diversity and expression motifs in disease (Citation7,Citation8). Cancer stage and nodal metastasis are considered the most important clinical factors to determine survival. Previous research in cancer indicates nodal metastasis can also be associated to specific omic signatures (Citation9,Citation10). Thus, this study aims to explore the interindividual variation of DNA methylation and gene expression samples from primary tumors of PC patients eligible for tumor resection. These omics will be used in conjunction with clinical covariates to determine whether their variation could explain and separate subjects based on whether they have greater nodal involvement, higher cancer staging, and expected survival. In this study, we quantified the interindividual variation of PC outcomes (nodal involvement, cancer staging, and survival) explained by the whole-genome omic profile from methylation and gene expression sets, instead of pre-selecting features from these sets.
Materials and methods
Data
Patient data consisted of 179 patients with 77 variables from the Cancer Genome Atlas (TCGA) Research Network, with clinical data and tissue from the primary tumor of PC. Seventy-eight percent of these patients had resectable tumors and received the Whipple procedure for treatment. The three outcomes studied were: 1) cancer staging; 2) nodal involvement; and 3) days to death. Days to death was treated as right-censored for living patients (censoring occurred at the day of last follow up recorded), while cancer staging and nodal involvement were treated as ordinal. Covariates with 50% or more missing data were excluded. Subjects who had between 0% and 50% missing data were imputed. Covariates included in all three outcomes were patient age at the moment of diagnosis, race, and gender, supporting literature associating them with PC prognosis (Citation2,Citation11). For the survival outcome, covariates were preselected based on their association with days to death using the likelihood ratio test in Cox-regression. Covariables included were: histologic diagnosis, initial pathologic diagnosis, and surgical procedure. Omic data from patients were level-three DNA methylation and gene expression profiles obtained by HumanMethylation450 and IlluminaHiSeq RNASeqV2 platforms. In these assays, invariable features and those with >20% missing were removed (Citation12). The remaining were imputed by the mean, centered, and standardized. Complete randomness of remaining missing values was tested with several FDR corrected Wald-Wolfowitz tests (Citation13).
Validation data
Interindividual variation between cancer staging and survival outcomes was estimated from the Canadian cohort of PC data (https://dcc.icgc.org/projects/PACA-CA), which was obtained from the International Cancer Genome Consortium (ICGC). Nodal involvement was not available in this dataset. Inclusion criteria for analysis were the presence of survival time, cancer staging, and having gene expression and methylation platforms genotyped. Though the original dataset contained 430 patients, only 85 individuals had complete information for survival time, cancer staging, and omic data. Following the same transformations as the ones described for TCGA patients, donor survival time was log-transformed and was treated as right-censored for living patients. For our survival model, covariates included in the validation were gender, age at diagnosis, histologic type, and cancer staging. For the cancer staging outcome, covariables included age at diagnosis and gender. For subjects missing between 1% and 50% of clinical data, we imputed values by the mean. The same series of models were executed for both datasets.
We modeled every response variable as a function of a linear predictor involving the effects of: (a) age, race, and gender (clinical covariates; COV); (b) methylation; (c) gene expression (). We extended our COV list in survival outcomes with nodal involvement and cancer stage. This was done using the Bayesian framework, assuming different likelihood functions and prior distributions. We used a truncated normal likelihood for the logarithm of survival time, and censored records were sampled from the truncated distribution; a deeper description of these models is available elsewhere (Citation14). For nodal involvement and cancer stage, a multi-threshold model was used. The effect of covariates were assumed as fixed (coming from an uninformative prior), and the omic effects were assumed as random with the following distributions: and
and
represent gene expression and DNA methylation, respectively, while the
represent a matrix of similarities among individuals for each omic (Citation15,Citation16). Model residuals were assumed ∼
Following the approach described by previous literature (Citation17–19), Reproducing Kernel Hilbert Spaces regressions (Citation20) were used to fit the data. The proportion of interindividual variation explained by gene expression and by methylation was calculated after obtaining the residual variance and individual variances due to methylation and gene expression. R 3.2.2 was used to evaluate and explore the datasets. R-package Bayesian Generalized Linear Regression (Citation14) was used to fit the linear mixed models for obtaining the proportions of variability explained. The 95% confidence intervals were computed for the methylation and gene expression variances, using R-package ‘Coda’ (Citation21). Inferences were based on MCMC samples.
Figure 1. The percentage of interindividual variability in nodal involvement, cancer stage, and survival time among PC patients from TCGA and ICGC cohorts. The contribution of each random term (UMETH = methylation effects, UGE = gene expression, ε = model residual) to the interindividual variance for each response is shown. ε was fixed to 1 since Tumor Staging and Nodal Involvement were treated as multi-threshold probit responses.
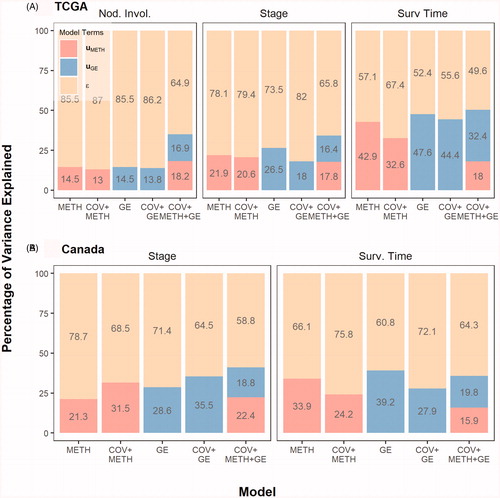
Results
DNA methylation and gene expression profiles were analyzed along with clinical covariates through a Bayesian generalized linear mixed model. The aim was to determine whether omic variation could explain and differentiate individuals based on their nodal involvement, varying degrees of cancer staging, and subsequent survival time. The results are summarized in .
DNA methylation and gene expression from the primary tumor captured the least amount of interindividual variation for nodal involvement. On the other hand, the omics explained a moderate amount of the variability observed in cancer staging and almost half of the variation between individuals in survival. Results from our validation study on cancer staging and survival outcome with the ICGC cohort were relatively consistent, where omics explained 36% of the interindividual variation for both cancer staging and survival. Deviance information criteria, a measure of model fitness, suggests that age, race, and gender do not improve models, but omics do. Thus, the use of omics could provide insight into the status of a cancer (nodal involvement and stage) or the survival prognosis of patients. While nodal involvement and cancer stage are important predictors of cancer outcome – e.g. survival (Citation22,Citation23) – omics explained a vital percentage of interindividual variability in survival. This suggests the impact of omics determining cancer aggressiveness. In agreement with this finding, a recent study in breast cancer also demonstrated that given cancer stage, gene expression, and methylation explained interindividual variation in breast cancer patients, highlighting that the models capture other mechanisms associated with survival beyond nodal metastasis and cancer stage (Citation18).
Discussion
Whole profiles of gene expression (Citation18) and gene expression in combination with methylation (Citation19) in breast cancer explain a substantial proportion of interindividual variation in survival, demonstrating the importance of mRNA and methylation changes in breast tumors associated with survival. Both gene expression and methylation profiles explain a substantial percentage of variability in survival. In glioblastoma multiforme, variation in mRNA appear to play a smaller role in the interindividual variation of patient survival, while methylation was the strongest predictor of this variation in survival (Citation24). Results from this study suggest that omics in tumors may be influential in PC development and proliferation. The use of omics and clinical covariates can help us understand interindividual variability for cancer patients and can offer insights on treatment. A potential explanation could be attributable to genes ADM, ASPM, DCBLD2, E2F7, KRT6A, which appear to be associated with vascular invasion and aggressiveness of the squamous tumor subtype (Citation25). New methods are in the intersection between the search of single biomarkers and whole genome variance estimation, such as Local Bayesian Regressions (Citation26). These methods could shed light on the amount of variation explained by the specific genomic signature as a predictive tool. However, larger datasets are necessary to implement these methods.
We acknowledge intrinsic limitations in this study, such as the small sample size and the fact that all patients were eligible for surgical resection, which is not representative of individuals who die of PC within a few months of diagnosis. Beyond limitations, we were able to replicate a previously observed role of mRNA and methylation levels at explaining the interindividual variation in survival of patients suffering from other cancers, elucidating the role of omics and their role in aggressive tumors.
Declaration of interest
The authors report no conflicts of interest. The authors alone are responsible for the content and writing of the article.
Additional information
Funding
References
- Imaoka H, Shimizu Y, Senda Y, Natsume S, Mizuno N, Hara K, et al. Post-adjuvant chemotherapy CA19-9 levels predict prognosis in patients with pancreatic ductal adenocarcinoma: a retrospective cohort study. Pancreatology. 2016;16(4):658–664. doi:10.1016/j.pan.2016.04.007.
- Lowenfels AB, Maisonneuve P. Epidemiology and prevention of pancreatic cancer. Jpn J Clin Oncol. 2004;34(5):238–244. doi:10.1093/jjco/hyh045.
- Gonzalez-Reymundez A, Vazquez AI. Multi-omic signatures identify pan-cancer classes of tumors beyond tissue of origin. bioRxiv. 2020;806323.
- Bardeesy N, DePinho RA. Pancreatic cancer biology and genetics. Nat Rev Cancer. 2002;2(12):897–909. doi:10.1038/nrc949.
- Hidalgo M. New insights into pancreatic cancer biology. Ann Oncol. 2012;23(Suppl_10):x135–x138. doi:10.1093/annonc/mds313.
- Nones K, Waddell N, Song S, Patch A-M, Miller D, Johns A, Wu J, et al. Genome-wide DNA methylation patterns in pancreatic ductal adenocarcinoma reveal epigenetic deregulation of SLIT-ROBO, ITGA2 and MET signaling. Int J Cancer. 2014;135(5):1110–1118. doi:10.1002/ijc.28765.
- Heyn H, Moran S, Hernando-Herraez I, Sayols S, Gomez A, Sandoval J, et al. DNA methylation contributes to natural human variation. Genome Res. 2013;23(9):1363–1372. doi:10.1101/gr.154187.112.
- Storey JD, Madeoy J, Strout JL, Wurfel M, Ronald J, Akey JM. Gene-expression variation within and among human populations. Am J Hum Genet. 2007;80(3):502–509. doi:10.1086/512017.
- Behring M, Shrestha S, Manne U, Cui X, Gonzalez-Reymundez A, Grueneberg A, et al. Integrated landscape of copy number variation and RNA expression associated with nodal metastasis in invasive ductal breast carcinoma. Oncotarget. 2018;9(96):36836–36848. doi:10.18632/oncotarget.26386.
- Shafi A, Nguyen T, Peyvandipour A, Nguyen H, Draghici S. A multi-cohort and multi-omics meta-analysis framework to identify network-based gene signatures. Front Genet. 2019;10:159. doi:10.3389/fgene.2019.00159.
- Yadav D, Lowenfels AB. The epidemiology of pancreatitis and pancreatic cancer. Gastroenterology. 2013;144(6):1252–1261. doi:10.1053/j.gastro.2013.01.068.
- Tom JA, Reeder J, Forrest WF, Graham RR, Hunkapiller J, Behrens TW, et al. Identifying and mitigating batch effects in whole genome sequencing data. BMC Bioinformatics. 2017;18(1):351 doi:10.1186/s12859-017-1756-z.
- Whaley FS. Optimizing the Wald-Wolfowitz runs statistic using a linkage tolerance: guidelines based on computer simulation. Commun Stat Theory Methods. 1987;16(7):2125–2138. doi:10.1080/03610928708829494.
- Pérez P, de los Campos G. Genome-wide regression and prediction with the BGLR statistical package. Genetics. 2014;198(2):483–495. doi:10.1534/genetics.114.164442.
- VanRaden PM. Efficient methods to compute genomic predictions. J Dairy Sci. 2008;91(11):4414–4423. doi:10.3168/jds.2007-0980.
- Vazquez A, Wiener H, Shrestha S, Tiwari H, de los Campos G. Integration of multi-layer omic data for prediction of disease risk in humans. Paper presented at: 10th World Congress of Genetics Applied to Livestock Production; 2014; Vancouver, BC, Canada.
- de los Campos G, Gianola D, Rosa G, Weigel KA, Crossa J. Semi-parametric genomic-enabled prediction of genetic values using reproducing kernel Hilbert spaces methods. Genet Res (Camb). 2010;92(4):295–308. doi:10.1017/S0016672310000285.
- Vazquez AI, Veturi Y, Behring M, Shrestha S, Kirst M, Resende MFR, et al. Increased proportion of variance explained and prediction accuracy of survival of breast cancer patients with use of whole-genome multiomic profiles. Genetics. 2016;203(3):1425–1438. doi:10.1534/genetics.115.185181.
- Gonzalez-Reymundez A, de los Campos G, Gutierrez L, Lunt SY, Vazquez AI. Prediction of years of life after diagnosis of breast cancer using omics and omic-by-treatment interactions. Eur J Hum Genet. 2017;25(5):538–544. doi:10.1038/ejhg.2017.12.
- Wahba G. Spline models for observational data. Philadelphia (PA): Society for Industrial and Applied Mathematics; 1990.
- Plummer M, Best N, Cowles K, Vines K. CODA: convergence diagnosis and output analysis for MCMC. Open Univ. 2006;6(1):7–11.
- Yang J, Long Q, Li H, Lv Q, Tan Q, Yang X. The value of positive lymph nodes ratio combined with negative lymph node count in prediction of breast cancer survival. J Thorac Dis. 2017;9(6):1531–1537. doi:10.21037/jtd.2017.05.30.
- Yu K-D, Jiang Y-Z, Chen S, Cao Z-G, Wu J, Shen Z-Z, et al. Effect of large tumor size on cancer-specific mortality in node-negative breast cancer. Mayo Clin Proc. 2012;87(12):1171–1180. doi:10.1016/j.mayocp.2012.07.023.
- Bernal Rubio YL, González-Reymúndez A, Wu K-HH, Griguer CE, Steibel JP, de los Campos G, et al. Whole-genome multi-omic study of survival in patients with glioblastoma multiforme. G3 (Bethesda). 2018;8(11):3627–3636. doi:10.1534/g3.118.200391.
- Raman P, Maddipati R, Lim KH, Tozeren A. Pancreatic cancer survival analysis defines a signature that predicts outcome. PLoS One. 2018;13(8):e0201751. doi:10.1371/journal.pone.0201751.
- Funkhouser SA, Vazquez AI, Steibel JP, Ernst CW, de los Campos G. Deciphering sex-specific genetic architectures using local Bayesian regressions. bioRxiv. 2019;653386.