Abstract
Objective
The composition of microbiota which correlates with infiltrating immune cells and clinical signatures is not clarified in CRC.
Methods
We applied 4 kinds of bioinformatic tools GSVA (version: 1.42.0), ESTIMATE (version: 1.0.13), CIBERSORT (version: 2.0), and immune-related genes.
Results
We found that a total of 8 types of microbiotas appeared in the three immune correlation analyses. Among these microbiotas, significant enrichments in relative abundances associated with immune cell infiltration can be found for the dominant phyla Proteobacteria, Firmicutes, and Actinobacteria. Moreover, there existed correlations between some of the 8 microbiotas and clinical-related indicators.
Conclusion
We identified some novel microbiotas involved in immune regulation in CRC.
Introduction
Colorectal cancer (CRC) is one of the major reasons of cancer death in the world (Citation1). The common occurrence of CRC is associated with western food (Citation2). Therefore, in this context, the environment of intestinal microbiota, especially its microbiome composition modified by food behaviors, plays an important role in CRC initiation and progression (Citation3). Inequality of microbial community composition triggers dysbiosis, which involves the increase of pathobionts and the decrease of probiotics in the development of CRC. For instance, pathobionts contain Escherichia coli, Bacteroides fragilis, etc., whereas probiotics comprise butyrate-generating bacteria such as Faecalibacterium (Citation4).
In the development of CRC, dysbiosis often leads to inflammation and tumor immune response. Detailed examples are as follows (Citation5). Helicobacter hepaticus can induce activation of macrophages and neutrophils, thus releasing cytokines such as TNF-α, stimulating the NF-κB signal transduction, and promoting the occurrence of CRC. Bacteroides fragilis actuates Th17 cells secreting IL-17 and promotes CRC tumorigenesis. Fusobacterium nucleatum enhances MDSC and inhibits T cells, thereby linking to CRC progression. On the other hand, Lactobacillus plantarum activates NK cells to produce IL-22, so as to boost the anti-tumor immune response in CRC. With the imbalance of the overall proportion of intestinal microbiota in tumor microenvironment (TME), the particular microbiota in intestinal microecology affects CRC through immunization. For instance, Clostridium butyricum and Bacillus subtilis both restrained the expansion of CRC through a molecular mechanism of modified immune homeostasis (Citation6).
Therefore, we made an assumption that different kinds of microbiota were related to different types of immune cells infiltrating into CRC tissues. In addition, diverse microbiota, in this TME, are also associated with different clinical signatures. To confirm this hypothesis, we used 4 kinds of analysis tools: GSVA (version: 1.42.0), ESTIMATE (version: 1.0.13), CIBERSORT (version: 2.0) as well as immune-related genes to identify the relationships between microbiota and infiltrated immune cells, as well as relationships between microbiota and clinically related indexes.
Methods
Dataset
On June 6, 2022, we conducted a search in the Gene Expression Omnibus (GEO) database using the keywords ‘colorectal cancer, microbiome, transcriptomic, and Homo sapiens’. However, we found only one dataset, GSE165255, available in the GEO database. GSE165255 data set in GEO database includes 83 samples of expression data and 89 samples of 16S sequencing microbiota data. The data sequencing platforms are Illumina hiseq 2500 and Illumina miseq. Salmon (version 0.10.2) was used to process RNA-seq data. Human genome assembly GRCh37 was used as reference. After alignment, DEseq2 (version: 1.31.2) was used to concatenate all individual samples and run comparative analysis. For 16S rRNA data, llumina Miseq paired-end reads were aligned to human reference genome hg19 with Bowtie2 (version: 2.4.3). SINA/SILVA (version: 1.7.2) classifier was used for classification. Operational taxonomic units (OTUs) were produced by binning sequences with identical taxonomic assignments. Of these 83 samples, 34 were tumor samples without antibiotics. We based our analysis on the RNA expression data and microbial OTU data after normalization in the GSE165255 dataset. Corresponding clinical data for the 34 samples are shown in Supplementary Table 1.
Data processing and analysis methods
ESTIMATE algorithm: the immune score in the groups with high or low expression of CD58 was calculated by using ESTIMATE package (version 1.0.13 under R 4.1.3); ssGSEA algorithm: gsva software package(version 1.42.0 under R 4.1.3) was used to obtain the socres of 28 kinds of immune cells; CIBERSORT algorithm: by using CIBERSORT (https://github.com/jason-weirather/CIBERSORT), the proportion of 22 immune cell types in the lm22 matrix was evaluated. The software of R language (Windows version No. 4.1.3) was used for statistical analysis, and the difference (P < 0.05) was statistically significant. Non paired t-test was used for normal distribution data, and Mann Whitney U-test was used for non-normal distribution data.
Results
Analysis of GSVA
Intestinal microbiota plays a role in recruiting immune cells in CRC. To explain this issue, we first analyzed immune cells infiltration in different samples of CRC by GSVA (version: 1.42.0). 34 samples were divided into high microbiota group (high) and low microbiota group (low) by taking the median value of each OTU (Operational taxonomic units) of each sample as the dividing point. Corresponding to two groups, there are differences in different immune cells infiltration. The relationship between microbiota and immune cells was drawn as ‘Figure S1, GSVA_16s_plot and , Top 50 abundances of microbiotas for GSVA’. In this heatmap, the abscissa represents microbiota and the ordinate represents difference of immune cells infiltration between high and low microbiota group. If the difference is positive, it will be red, otherwise it will be blue. If no difference exists, it is yellow. As for a microbiota with consistently different median value of each OTU, difference of recruited immune cells infiltration is largely consistent. But difference of one immune cells infiltration recruited by different microbiota with consistently different median value of each OTU is largely inconsistent.
Figure 1. Top 50 abundances of microbiotas for GSVA. The GSVA analysis (Gene Set Variant Analysis) for the relationship between microbiota and immune cells. The abscissa represents top 50 abundances of microbiotas and the ordinate represents difference of immune cells infiltration between high and low microbiota group. If the difference is positive, it will be red, otherwise it will be blue. If no difference exists, it is yellow.
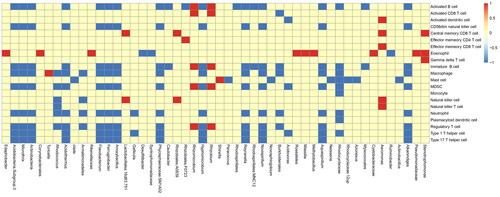
Estimate analysis
Score from the estimation algorithm reflect the infiltration degree of stromal cells and immune cells in CRC and the purity of tumor. All these factors are also associated with regulation of intestinal microbiota in CRC. According to the median value of each OTU (Operational taxonomic units), CRC samples could be divided into two groups. The differences of three indexes (StromalScore, ImmuneScore, and ESTIMATEScore) between the 2 groups were analyzed. A total of 16 kinds of microbiota were screened out by difference analysis.
CIBERSORT analysis
CIBERSORT (version: 2.0) tool can also reflect the infiltration degree of immune cells and its relationship with regulation of intestinal microbiota in CRC. According to the median value of microbiota content, CRC samples could be divided into two groups. The differences of immune cells infiltration between the high microbiota and low microbiota obtained by CIBERSORT algorithm were analyzed. In total, 180 microbiota groups were differentially expressed. The relationship between microbiota and immune cells is plotted as ‘Figure S2, CIB_ 16s. pdf and , Top 50 abundances of microbiotas for CIB’.
Figure 2. Top 50 abundances of microbiotas for CIB. The CIBERSORT analysis for the relationship between microbiota and immune cells. The abscissa represents top 50 abundances of microbiotas and the ordinate represents immune cells. If the difference between microbiota and immune cells is positive, it will be red, otherwise it will be blue. If no difference exists, it is yellow.
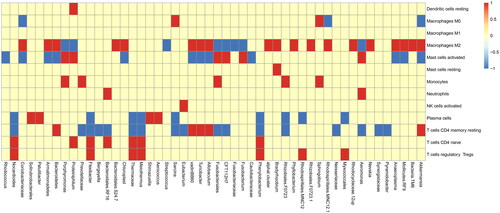
Analysis of immune related gene
Next, we evaluated the relationship between immune-related genes and intestinal microbiota of CRC. Immune-related genes were derived from literature ‘the immune landscape of cancer’, as shown in the . Only 61 reference genes were obtained from GSE165255 dataset. According to the median value of each OTU (Operational taxonomic units), CRC samples could be divided into two groups. 60 of the 61 genes are different between these two groups. ‘The relationship between microbiota and immune genes was drawn as “Figure S3, immune_16s.pdf and , Top 50 abundances of microbiotas for immune”’.
Figure 3. Top 50 abundances of microbiotas for Immune genes. Analysis for the relationship between microbiota and immune genes. The abscissa represents top 50 abundances of microbiotas and the ordinate represents immune genes. If the difference between microbiota and immune genes is positive, it will be red, otherwise it will be blue. If no difference exists, it is yellow.
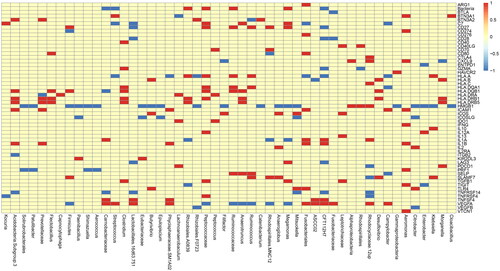
Table 1. Immune-related genes.
Intersection of four immune correlation analyses
Microbiota groups that appeared simultaneously in the four analyses did not exist, and a total of 8 microbiota groups appeared in the three analyses. Venn analysis figure and list was drawn as ‘, jVenn_chart.png’.
Analysis of other clinically related indexes
For identified 8 kinds of microbiota, there were no differences in the indicators of sex, race, ethnicity and colonic_ NEOPLASIA_ AFFECTION_ STATUS, LATERALITY, DIABETES_ STATUS, SMOKING_ status, age, but there were significant differences among the four indicators–-CRC_ Stage, FAMILY_ HISTORY_ CRC,2 types of BMI_ Group. ‘Bacteria/Proteobacteria/Alphaproteobacteria/Rhodospirillales/Rhodospirillaceae/Magnetospirillum’ mainly appears in stage II with family inheritance. ‘Bacteria/Actinobacteria/Coriobacteriia/Coriobacteriales/Coriobacteriaceae’ is more common in samples without family genetic history. Bacteria/Actinomycetota/Coriobacteriia/Eggerthellales/Eggerthellaceae/Eggerthella’ is more abundant in people with higher BMI, whereas ‘Bacteria/actinobacteria/Actinomycetota/Actinomycetes/Actinomycetales/Actinomycetaceae/Varibaculum’ is higher in people with lower BMI. ‘Results’ figure were drawn as ‘, CRC_STAGE.pdf’ (only stage II has microbiota content), ‘, FAMILY_HISTORY_CRC_Magnetospirillum.pdf’, ‘, BMI_Varibaculum.pdf’, and ‘, BMI_Eggerthella.pdf’.
Figure 5. Expression analysis of microbial populations according to clinical parameter (CRC_STAGE) were performed by using ANOVA.
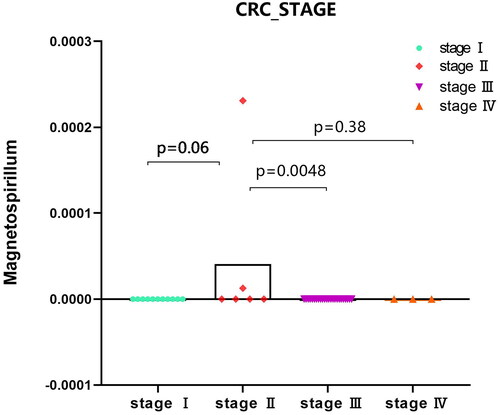
Figure 6. Expression analysis of microbial populations according to clinical parameter (FAMILY_HISTORY_CRC_Magnetospirillum) were performed by using Mann-Whitney U test.
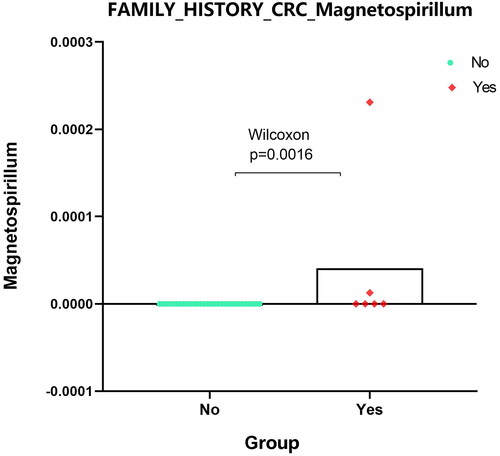
Discussion
The microbiota is a neglected part of the body, which reflects the closely interconnected ecosystem between the body and the environment. The accurate balance of microbiota plays a crucial role in health status and the prevention of many chronic diseases (Citation7). If this balance is thrown off (a dysbiosis), it can lead to CRCs. Dysbiosis is mainly manifested in two aspects: a lack of probiotics and an abundance of pathobionts. Microbiota-mediated carcinogenesis has been extensively studied. However, the mechanisms of microbiota-induced cancer development, especially relationships between microbiota and infiltrated immune cells, remain unclear. Therefore, it is very important to identify the unique microbiota and understand the immune cell infiltration mediated by microbiome for defeating dysbiosis-induced CRC.
In this research, GSVA, ESTIMATE, CIBERSORT and immune-related genes were used to study the correlation between microbiota and immune cells infiltration. We did not find the genes that appeared simultaneously in the four analyses. However, we found that a total of 8 microbiota appeared in the three analyses.
Among these 8 microbiotas, the relative abundances associated with immune cell infiltration is significantly enriched in Proteobacteria, Firmicutes, and Actinobacteria, which are dominant phyla. As an intestinal symbiote, Proteobacteria has pathogenic properties. A dysbiosis associated with metabolic disorders, such as obesity caused by diet, obesity caused by genetic factors, diabetes caused by genetic factors, etc., may all cause the growth and multiply of Proteobacteria (Citation8,Citation9). According to a recent study, there is a great difference in the abundance of Proteobacteria in mucosa-related microbiome samples from the ileum and the rectal between patients with Crohn’s disease and control subjects (Citation10). It has been reported that modification of intestinal Proteobacterial community appear in CRC associated with human colitis (Citation11). Stefano M. et al. (Citation12) reported that Magnetospirillum spp., acting as living iron chelators, could potentially affect tumor growth and progression by competing for nutrients. Repeated stress-related diarrhea could lead to significant changes in the relative abundance of 28 bacterial species, including Pelomonas between intestinal contents and mucosa (Citation13). Rhodoplanes and Rhodospirillales were correlated with colorectal cancer (CRC) due to their role in enhancing CD8+ T-cell infiltration (Citation14), and Rhodospirillales could also be associated with switch B cells and memory/effector CD4+ and CD8+ cells (Citation15). Some Proteobacteria have been associated with chronic inflammation in the colon, which is a known risk factor for colorectal cancer (Citation16). Chronic inflammation can create a pro-tumorigenic microenvironment by releasing cytokines and growth factors that promote cell proliferation and survival.
Firmicutes and actinobacteria, as the major microbiota community of intestinal tract, have several potentials of antitumour activity. Many food components have a direct impact on the kinds of intestinal microflora: in the Western food mode (high-fat, red meat), the ratio of conditional pathogens in the gut microbiota enhances, the ratio of commensal bacteria declines, and the happening of colorectal cancer is boosted. On the contrary, food rich in fiber can enhance the amount of Firmicutes and decrease the amount of Bacteroides, thus increasing the levels of short-chain fatty acids (SCFAs) in the intestine, and inhibiting the colorectal carcinogenesis (Citation17). Eggerthella is linked to drug metabolism, the processing of dietary phytochemicals, and bile acid metabolism with various intestinal disease (Citation18). Varibaculum and Granulicatella show closest connectivity with rectal neuroendocrine tumors, which indicates a tight interaction of gut microbial species with metabolites (Citation19). Many Firmicutes bacteria are involved in the fermentation of dietary fiber and produce short-chain fatty acids (SCFAs) such as butyrate. Butyrate is known to have anti-inflammatory and anti-tumorigenic properties. It can promote apoptosis (cell death) in cancer cells and enhance the barrier function of the intestinal epithelium, which can help prevent the infiltration of harmful substances into the mucosa. Some Actinobacteria can have immunomodulatory effects. They can influence the activity of immune cells in the gut, potentially impacting the immune response to colorectal cancer. Modulating the immune response within the TME can be both pro-tumorigenic or anti-tumorigenic, depending on the specific interactions and context. It’s important to note that the microbiome is highly diverse and can vary from person to person. The composition of these bacterial phyla within the gut microbiome can be influenced by various factors, including diet, genetics, and lifestyle. Moreover, the specific strains of bacteria within these phyla can have different effects on the TME.
Another critical feature of this study is that we found that the signature of CRC regulated by microbiota are related to different clinically relevant indexes. Our results demonstrated that the phyla Proteobacteria may be associated with stage II and family inheritance, whereas the phyla Actinobacteria is more common in samples without family genetic history, which may be associated with different levels of BMI. These results indicated that Proteobacteria may be triggers of genetic factors in CRC, and Actinobacteria may be related to the metabolic mechanisms in the pathogenesis of CRC.
To summary, based on the analysis of GEO datasets, this research established relationships networks including microbiota of probiotics and pathobionts and infiltrating immune cells in colorectal carcinogenesis and development. It supplies a thorough analysis of the microbiota regulatory mechanism in CRC. Furthermore, these novel microbiotas could be participants of diagnosis, therapy as well as prognosis in CRC. This is meaningful for future studies in the diagnosis, therapy as well as prognosis in CRC. Limitations of this study are based on an analysis of public datasets and short of validation at experimental and clinical level. Limited datasets hinder our ability to explore colorectal cancer development and gut microecology more extensively and comprehensively. Furthermore, as the study of CRC continues to increase and our knowledge expends regarding the intricate interplay between the intestinal microbiome, transcriptome, tumor microenvironment, and immune infiltration, we are poised to unlock deeper insights into CRC tumorigenesis and prognosis.
Authors’ contributions
Liu Dan and Heng Zhang conceived and designed the study. Jiang Zhou, Yuanzhu Zhao and Panpan Wang collected and assembled the data. Yang Zhang and Meihong Cui analyzed the data and prepared the figures. All authors wrote the manuscript. All authors have read and approved the final manuscript.
Supplemental Material
Download PDF (5.8 MB)Disclosure statement
No potential conflict of interest was reported by the author(s).
Data availability statement
All data sets for this study are included in the GEO (https://www.ncbi.nlm.nih.gov/geo/) GSE165255 dataset for the database.
Additional information
Funding
References
- Torre LA, Siegel RL, Ward EM, Jemal A. Global cancer incidence and mortality rates and trends—an update. Cancer Epidemiol Biomark Prev. 2016; 25(1):16–27. doi:10.1158/1055-9965.EPI-15-0578.
- Wu GD, Chen J, Hoffmann C, Bittinger K, Chen Y-Y, Keilbaugh SA, et al. Linking long-term dietary patterns with gut microbial enterotypes. Science. 2011; 334(6052):105–108. doi:10.1126/science.1208344.
- Costello EK, Lauber CL, Hamady M, Fierer N, Gordon JI, Knight R. Bacterial community variation in human body habitats across space and time. Science. 2009; 326(5960):1694–1697. doi:10.1126/science.1177486.
- Tilg H, Adolph TE, Gerner RR, Moschen AR. The intestinal microbiota in colorectal cancer. Cancer Cell. 2018; 33(6):954–964. doi:10.1016/j.ccell.2018.03.004.
- Yang X, Guo Y, Chen C, Shao B, Zhao L, Zhou Q, et al. Interaction between intestinal microbiota and tumour immunity in the tumour microenvironment. Immunology. 2021; 164(3):476–493. doi:10.1111/imm.13397.
- Chen Z-F, Ai L-Y, Wang J-L, Ren L-L, Yu Y-N, Xu J, et al. Probiotics Clostridium butyricum and Bacillus subtilis ameliorate intestinal tumorigenesis. Future Microbiol. 2015; 10(9):1433–1445. doi:10.2217/fmb.15.66.
- Ceapa C, Wopereis H, Rezaïki L, Kleerebezem M, Knol J, Oozeer R. Influence of fermented milk products, prebiotics and probiotics on microbiota composition and health. Best Pract Res Clin Gastroenterol. 2013; 27(1):139–155. doi:10.1016/j.bpg.2013.04.004.
- Zhang C, Zhang M, Pang X, Zhao Y, Wang L, Zhao L. Structural resilience of the gut microbiota in adult mice under high-fat dietary perturbations. ISME J. 2012; 6(10):1848–1857. doi:10.1038/ismej.2012.27.
- Everard A, Lazarevic V, Derrien M, Girard M, Muccioli GG, Neyrinck AM, et al. Responses of gut microbiota and glucose and lipid metabolism to prebiotics in genetic obese and diet-induced leptin-resistant mice. Diabetes. 2011; 60(11):2775–2786. doi:10.2337/db11-0227.
- Gevers D, Kugathasan S, Denson LA, Vázquez-Baeza Y, Van Treuren W, Ren B, et al. The treatment-naive microbiome in new-onset Crohn’s disease. Cell Host Microbe. 2014; 15(3):382–392. doi:10.1016/j.chom.2014.02.005.
- Bonnet M, Buc E, Sauvanet P, Darcha C, Dubois D, Pereira B, et al. Colonization of the human gut by E. coli and colorectal cancer risk. Clin Cancer Res. 2014; 20(4):859–867. doi:10.1158/1078-0432.CCR-13-1343.
- Menghini S, Ho PS, Gwisai T, Schuerle S. Magnetospirillum magneticum as a living iron chelator induces TfR1 upregulation and decreases cell viability in cancer cells. Int J Mol Sci. 2021; 22(2):498. doi:10.3390/ijms22020498.
- Zhang C-Y, Peng X-X, Shao H-Q, Li X-Y, Wu Y, Tan Z-J. Gut microbiota comparison between intestinal contents and mucosa in mice with repeated stress-related diarrhea provides novel insight. Front Microbiol. 2021; 12:626691. doi:10.3389/fmicb.2021.626691.
- Zhang J, Tao J, Gao R-N, Wei Z-Y, He Y-S, Ren C-Y, et al. Cytotoxic T-cell trafficking chemokine profiles correlate with defined mucosal microbial communities in colorectal cancer. Front Immunol. 2021; 12:715559. doi:10.3389/fimmu.2021.715559.
- Sbierski-Kind J, Grenkowitz S, Schlickeiser S, Sandforth A, Friedrich M, Kunkel D, et al. Effects of caloric restriction on the gut microbiome are linked with immune senescence. Microbiome. 2022; 10(1):57. doi:10.1186/s40168-022-01249-4.
- Cao Y, Zheng X, Hu Y, Li J, Huang B, Zhao N, et al. Levels of systemic inflammation response index are correlated with tumor-associated bacteria in colorectal cancer. Cell Death Dis. 2023; 14(1):69. doi:10.1038/s41419-023-05602-9.
- Huang P, Liu Y. A reasonable diet promotes balance of intestinal microbiota: prevention of precolorectal cancer. Biomed Res Int. 2019;2019:3405278. doi:10.1155/2019/3405278.
- Dong X, Guthrie BG, Alexander M, Noecker C, Ramirez L, Glasser NR, et al. Genetic manipulation of the human gut bacterium Eggerthella lenta reveals a widespread family of transcriptional regulators. Nat Commun. 2022; 13(1):7624. doi:10.1038/s41467-022-33576-3.
- Hu W, Chen ZM, Li XX, Lu L, Yang GH, Lei ZX, et al. Faecal microbiome and metabolic signatures in rectal neuroendocrine tumors. Theranostics. 2022;12(5):2015–2027. doi:10.7150/thno.66464.