Abstract
Many independent studies in social robotics and human–robot interaction have gained knowledge on various factors that affect people’s perceptions of and behaviors toward robots. However, only a few of those studies aimed to develop models of social robot acceptance integrating a wider range of such factors. With the rise of robotic technologies for everyday environments, such comprehensive research on relevant acceptance factors is increasingly necessary. This article presents a conceptual model of social robot acceptance with a strong theoretical base, which has been tested among the general Dutch population (n = 1,168) using structural equation modeling. The results show a strong role of normative believes that both directly and indirectly affect the anticipated acceptance of social robots for domestic purposes. Moreover, the data show that, at least at this stage of diffusion within society, people seem somewhat reluctant to accept social behaviors from robots. The current findings of our study and their implications serve to push the field of acceptable social robotics forward. For the societal acceptance of social robots, it is vital to include the opinions of future users at an early stage of development. This way future designs can be better adapted to the preferences of potential users.
CONTENTS
INTRODUCTION
EVALUATING RELEVANT ACCEPTANCE MODELS
2.1. Reviewing Traditional Models of Technology Acceptance
2.2. Reviewing Existing Models for Social Robot Acceptance
2.3. Reviewing the Theory of Planned Behavior
2.4. Toward a Model of Social Robot Acceptance
INFLUENTIAL FACTORS FOR SOCIAL ROBOT ACCEPTANCE
3.1. Attitudinal Beliefs Structure
3.2. Normative Beliefs Structure
3.3. Control Beliefs Structure
3.4. The Conceptual Model
METHOD
4.1. Sampling of Participants
4.2. Design of the Questionnaire
4.3. The Measurement Model
Establishing the First-Order Factor Model
Establishing the Second-Order Factor Model
RESULTS
5.1. Interpreting the Effects of the Attitudinal Beliefs
5.2. Interpreting the Effects of the Normative Beliefs
5.3. Interpreting the Effects of the Control Beliefs
GENERAL DISCUSSION
6.1. Implications
Influential Factors for Social Robot Acceptance
The Unwanted Sociability of Robots
Practical Implications for the Development of Social Robots
6.2. Limitations
6.3. Conclusion
1. INTRODUCTION
The economic prospects of the robotics market are rapidly expanding. In 2013, approximately 4 million service robots for personal and domestic use were sold worldwide, and this number is expected to increase to 31 million by the end of 2017 (International Federation of Robotics, Citation2014). However, the increasing presence of robots in our everyday lives will not simply be accepted unreservedly by human users. Research in robotics suggests that the mere presence of robots in everyday life automatically increases neither their chances of being accepted nor the willingness of users to interact with them (Bartneck et al., Citation2005), which is a major challenge for the success of social robots. Although there have been many studies in the field of social robotics regarding the various factors affecting people’s perceptions of and behavior toward robots, only a few aimed to develop models of social robot acceptance. As researchers focus more on developing robotic technologies for everyday environments, more comprehensive studies on the factors relating to their acceptance are increasingly necessary. Furthermore, the inclusion of future users during the early stages of design is important for developing socially robust, rather than merely acceptable, robotic technologies (Sabanovic, 2010). Therefore, the goal of this article is to present a conceptual model of social robot acceptance for domestic purposes and to test it using structural equation modelling (SEM). We begin by evaluating the current acceptance model and then present the theoretical framework of our conceptual model within the theory of planned behavior (TPB). Thereafter we describe several influential factors for social robot acceptance in domestic environments, resulting in our proposed conceptual model. We then outline our research methods, including the establishment of the measurement model. Following this, we present the test results of our conceptual model, along with its hypotheses. This article concludes with the implications for social robot acceptance in domestic environments and how our model could serve to advance the field of social robotics.
2. EVALUATING RELEVANT ACCEPTANCE MODELS
Applying existing acceptance models from human–computer interaction to the field of social robotics without modification is problematic, because robot technology is far more complex than other technological devices (Flandorfer, Citation2012). With robots recognizing our faces, making eye contact, and responding socially, they are pushing our Darwinian buttons by displaying behavior associated with sentience, intentions, and emotions (Turkle, Citation2011). Therefore, some researchers have argued that robots should be regarded as a new technological genre (de Graaf, Citation2016; Kahn, Gary, & Shen, Citation2013; Young, Hawkins, Sharlin, & Igarashi, Citation2007). In this section, we review the most prominent models applied to technology acceptance in general, then critically reflect on the few existing models developed specifically for social robot acceptance. We later conclude that we need to deviate from these models in the development of our conceptual model of social robot acceptance. As we argue in what follows, we suggest building on the framework of TPB. Because we acknowledge that TPB has its shortcomings, which we elaborate next, the final part of this section provides suggestions for improvement on our conceptual model of social robot acceptance.
2.1. Reviewing Traditional Models of Technology Acceptance
The technology acceptance model (TAM) developed by Davis (Citation1989) is considered the most influential and commonly applied theory for describing an individual’s acceptance of information systems (Y. Lee, Kozar, & Larsen, Citation2003). The widespread popularity of TAM is broadly attributable to three factors. First, it is a parsimonious and IT-specific model, designed to adequately explain and predict the acceptance of a wide range of systems and technologies among a diverse population of users across varying organizational and cultural contexts and expertise levels. Second, the TAM model has a strong theoretical base and a well-researched, validated inventory of psychometric measurement scales, which makes its use operationally appealing. Third, the model has accumulated strong empirical support for its overall explanatory power and has emerged as a preeminent model of users’ acceptance of technology (Yousafzai, Foxall, & Pallister, Citation2007a). TAM views user acceptance as being dependent upon the perceived usefulness of the technology and its perceived ease of use. The model was first developed by Davis (Citation1989) to provide validated measurement scales for predicting the user acceptance of computers, as these subjective measures were not yet validated and their relationships to systems use unknown. The model adopts a causal chain of beliefs, attitudes, intention, and behavior, introduced previously by social psychologists (Ajzen, Citation1991; Fishbein & Ajzen, Citation1975). Based on certain beliefs, people form attitudes about a specific object, the basis upon which they form an intention to behave regarding that object. Here, the effects of the outcome variables end at intention to use, or even at attitude toward use. In TAM, the only predictor of actual system use is behavioral intention. Although TAM has been found to be a useful predictor of acceptance behavior in numerous contexts, it does not provide a mechanism for the inclusion of other salient beliefs (Benbasat & Barki, Citation2007). The literature suggests that other factors may play a role in explaining use behavior, including expected outcomes and habits (LaRose & Eastin, 1994), motives to use a technology (Katz, Blumler, & Gurevitch, Citation1973), or environmental factors (Bandura, Citation1977). As a result, many recent studies focused on the elaboration of the model, including those undertaken by Davis and his colleagues (e.g., Davis, Citation1989; Venkatesh & Davis, Citation2000). A review of TAM-related research shows that many determinants of perceived usefulness and perceived ease of use have been discovered (Y. Lee et al., Citation2003). Therefore, the creators of TAM expanded their original model, resulting in the introduction of a second edition of TAM (Venkatesh & Davis, Citation2000) and later a third edition (Venkatesh & Bala, Citation2008). However, even this third edition of their model is still somewhat limited.
TAM is a very economical model that does not specifically include other external factors, besides usefulness and ease of use. Moreover, the model presumes that all external factors are moderated by the evaluation of usefulness and ease of use. However, many studies adopting the principles of TAM have demonstrated that several other factors directly influence behavioral intentions and actual behavior (see Y. Lee et al., Citation2003, for a summary). Indeed, the relation between perceived usefulness, perceived ease of use, and use behavior may be more complex and less linear than reflected by TAM. As depicted in TPB (Ajzen, Citation1991), social influence, facilitating conditions (Venkatesh, Morris, Davis, & Davis, Citation2003), and habitual use (Ouellette & Wood, Citation1998; Triandis, Citation1979) have also been found to explain actual use directly, and not, as the original TAM assumes, to only mediate it through usefulness and ease of use. In addition, TAM assumes that technology use is directly accepted or not accepted independently of other factors preventing individuals from using a technology. However, many situational factors, such as lack of time, money, or experience, can prevent individuals from using a technology (Mathieson, Peacock, & Chin, Citation2001). Other researchers argue that the overly simple conceptualization and operationalization of the constructs of usefulness and ease of use have prevented researchers from understanding the internal workings of these central constructs within TAM (Benbasat & Barki, Citation2007). These examples indicate that the acceptance of domestic social robots is more complex and less linear than the limited TAM model suggests, raising objections against its applicability for the investigation of social robot acceptance in domestic environments.
One of the most prominently applied models of technology acceptance is the unified theory of acceptance and use of technology (UTAUT), developed by the same researchers who worked on the TAM modifications (Venkatesh et al., Citation2003). In developing UTAUT, the researchers reviewed and consolidated the constructs of eight theoretical models, employed in previous research, to explain information systems use behavior (i.e., theory of reasoned action [TRA], TAM, motivational model, TPB, a combined TPB/TAM, model of personal computer use, diffusion of innovations theory, and social cognitive theory). In building this eclectic model, the researchers chose an empirical, rather than theoretical, approach. Of all these theoretical constructs, only those shown to have the highest significant effect in an empirical study investigating the user acceptance of an information system were picked for their model. UTAUT holds that performance expectancy, effort expectancy, social influence, and facilitating conditions are direct determinants of use intention and actual use. Gender, age, experience, and voluntariness of use are posited to moderate the impact of these four key constructs on use intention and actual use. The inclusion of moderators in the model is reminiscent of a social psychological approach.
The effects of the independent variables thus do not spread beyond the user’s intention to use, and the single predictor of actual system use is behavioral intention. Obviously, there are both advantages and limitations to UTAUT’s utilization in acceptance research. An advantage is its holistic approach to explaining many psychological and social factors that impact technology acceptance, together with the consistent validity and reliability of data collection through the instrument (Yoo, Han, & Huang, Citation2012). However, despite being an eclectic model that combines highly correlated variables to create an extremely high explained variance (Yoo et al., Citation2012), UTAUT is criticized for not being parsimonious enough, because it requires several variables to achieve a substantial level of explained variance (Straub & Burton-Jones, Citation2007). Parsimony, the goal of which is to identify factors accounting for the most variation, is to be greatly valued (Burgoon & Buller, Citation1996), but not at the expense of explanatory power. UTAUT does not explain the different underlying mechanisms, although such an explanation would make the unified model more suitable for explaining the user’s general opinions about expected use, rather than explaining the user’s motivations relating to the continued and increased adoption of a particular technology (Peters, Citation2011). Another disadvantage is that, even though the founders of the model are working toward extending the original model to a second edition (Venkatesh, Thong, & Xu, Citation2012), both measurements of social influence and facilitating conditions are not robustly constructed. These concepts are quite complex but are measured with only two items. In addition, by adding social influence and facilitating conditions to the original technology acceptance model, we are essentially faced with a model that is not very different from the model of planned behavior theory. The two constructs of social influence and facilitating conditions from UTAUT overlap considerably with the constructs of subjective norm and perceived behavioral control from TPB. Moreover, the original TAM and UTAUT constructs were merely developed for utilitarian systems and were validated in a working environment. The applicability of these models on hedonic systems or more pleasure-oriented systems is limited (van der Heijden, Citation2004). Yet the use of social robots in domestic environments could result in an experience that goes beyond its utility. These robotic systems have been observed to evoke a social reaction from its users (Kahn, Friedman, Perez-Granados, & Freier, Citation2006; K. Lee, Park, & Song, Citation2005; Reeves & Nass, Citation1996). In addition, the context in which these models have been validated (i.e., in the working environment) is not congruent with our study’s objective, which is social robot acceptance in domestic environments. This suggests that other models may be more appropriate for the development of a model of acceptance for domestic social robots.
2.2. Reviewing Existing Models for Social Robot Acceptance
To our knowledge, only two user acceptance models for social robots have been proposed to date using SEM. The current most cited model of social robot acceptance is the Almere model of Heerink, Kröse, Evers, and Wielinga (Citation2010). Shin and Choo (Citation2011) presented an alternative acceptance model for social robots. Although these models offer useful insights into the factors influencing social robot acceptance, they show some weakness regarding its general application in the domestic context. First, both the Almere model (Heerink et al., Citation2010) and the acceptance model for socially interactive robots (Shin & Choo, Citation2011) have their roots in UTAUT. As previously indicated, UTAUT is not considered to be parsimonious (Straub & Burton-Jones, Citation2007), and it is an eclectic model that combines highly correlated variables to create an unnaturally high explained variance (Yoo et al., Citation2012). In what follows, we argue that TPB offers a more suitable theoretical base for a model of social robot acceptance that focuses on individual adoption behavior in a domestic environment. Second, both models have been tested only on specific user groups. The Almere model (Heerink et al., Citation2010) has been developed for the acceptance of socially interactive agents in the eldercare facilities context, and the acceptance model for socially interactive robots (Shin & Choo, Citation2011) has been tested on a sample of students. This limits the generalizability of these models to other user groups and contexts. Our study focuses on the general population and social robot use within the domestic context, for which the two existing models have not yet been validated. Third, both models are based on grouped findings from previous research in human–robot interaction (HRI) and human–computer interaction. They lack both a theoretical foundation and strong arguments for the inclusion of the chosen factors in the model and the exclusion of other factors. Fourth, the SEM, used to test the Almere model, was performed on a data set that consisted of a combined dataset from four separate studies. Similarly, the acceptance model for socially assistive robots (Shin & Choo, Citation2011) is based on different groups of participants, who used different types of robots with varying functionalities. Neither of the two studies statistically confirmed any similarities between the data sets to justify merging their samples into one data set to test their models. A final shortcoming of the Almere model can be found in the application of the model modification indices, which were accepted without any theoretical support. Based on the deficiencies of both models, we decided to deviate from these existing models by proposing a new model for social robot acceptance, conceptualized within a strong theoretical foundation.
2.3. Reviewing the Theory of Planned Behavior
Because our focus is mainly on psychological aspects of individual users, we have chosen to build on an existing theory from a psychological perspective. We use the TPB (Ajzen, Citation1991) as a starting point in the development of our proposed model. We chose TPB as a guiding framework because (a) it is particularly suitable for explaining and predicting volitional behaviors, including technology acceptance (Mathiesson, 1991; S. Taylor & Todd, Citation1995; Venkatesh & Brown, Citation2001); (b) it has been successfully applied to explain a wide range of behaviors (Ajzen, Citation1991); and (c) its origin invites researchers to extend the model to adapt to a specific behavior (Ajzen, Citation1991). Moreover, when considering use intention as the main outcome variable to explain future use of a new technology—in this case, social robots—the explanatory power of TPB is greater than that of TAM and its successors, especially when it is decomposed to a specific technology (S. Taylor & Todd, Citation1995). Therefore, TPB provides a solid basis for the development of a conceptual model to investigate social robot acceptance from an individual perspective.
TPB, which is an extension of TRA (Ajzen & Fishbein, Citation1980), has been one of the most influential, well-researched theories in explaining and predicting behavior across a variety of settings (Manstead & Parker, Citation1995). As a general model, it is intended to provide a parsimonious explanation of informational and motivational influences on most human behavior and can therefore be used to predict and understand human behavior (Ajzen, Citation1991). The TPB approach is embedded in expectancy-value models of attitudes and decision making, with an underlying logic that the expected personal and social outcomes of a particular action influence the intention to behave in a certain way (Manstead & Parker, Citation1995). According to TPB, the main determinant of a behavior is a behavioral intention, which in turn is determined by attitude, subjective norms, and perceived behavioral control. Attitude captures an individual’s overall evaluation of performing the behavior, whereas subjective norms refer to an individual’s perception of the expectations of important others about the specific behavior. Because the achievement of behavioral goals is not always completely under volitional control, Ajzen (Citation1991) added a third concept to the prediction of behavior, namely, perceived behavior control. Perceived behavioral control is an individual’s perceived ease or difficulty in performing the behavior and is conceptually related to Bandura’s (Citation1977) self-efficacy. The concept of perceived behavioral control may include both internal (e.g., skills, knowledge, adequate planning) and external (e.g., facilitating conditions, availability of resources) factors.
Despite its success in behavior research (Manstead & Parker, Citation1995), a flaw of TPB’s original model and the hypothesized relations between its constructs is that only moderate correlations exist between the global and belief measures of its constructs (Benbasat & Barki, Citation2007). This means that these concepts are not strongly related and other factors may influence the formation of people’s beliefs about a certain behavior. Moreover, the model suggests correlations between attitudes, subjective norms, and perceived behavioral control (Ajzen, Citation1991), which result in a lack of knowledge regarding the precise nature of the relations between these concepts. Meta-analytic reviews on TPB (e.g., Armitage & Conner, Citation2001; Sheppard, Hartwick, & Warshaw, Citation1988) indicate that a substantial proportion of the variance of behavior intention remains unexplained by the core variables of attitudinal beliefs, subjective norms, and perceived behavior control. This has led some researchers to postulate that other factors play a role in explaining and predicting human behavior (Bentler & Speckart, Citation1981). TPB has also been challenged for its claim that attitude, subjective norms, and perceived behavioral control are the sole antecedents of intentions.
The critics can be divided into four groups: (a) those who challenge the lack of emotional components in the model, (b) those who criticize the sole focus on social pressure in the social components in the model, (c) those who criticize the assumption that all behaviors are consciously performed, and (d) those who argue that a lot of behavior is a result of habitual routines. Next we focus on these criticisms and explain how we address these shortcomings in our conceptual model of domestic social robot acceptance.
First, TPB is challenged for the lack of emotional components in the model, as it mainly focuses on cognitive or instrumental components and neglects affective evaluations or emotional aspects of human behavior (Bagozzi et al., Citation2001). However, although both concepts are highly correlated, they can be empirically discriminated and have different functions in explaining or predicting human behavior (Breckler & Wiggins, Citation1989; Greenwald, Citation1989). Human behavior is not purely rational. In fact, emotions are intertwined in the determination of human behavioral reactions to environmental and internal events that are very important to the needs and goals of an individual (Izard, Citation1977). Many researchers believe that it is impossible for humans to act or think without the involvement of, at least subconsciously, our emotions (Mehrabian & Russell, Citation1974). Indeed, rational evaluations and forming expectations, as well as nonrational attitudes, feelings, and other affective or emotional-related concepts have been acknowledged by researchers to influence human behavior (Limayem & Hirt, Citation2003; Manstead & Parker, Citation1995; Richard, Plicht, & Vries, Citation1995; Sun & Zhang, Citation2006). If emotions affect human behavior in general, they might be relevant for HRI research as well. Several studies have indicated that people react emotionally when confronted with robots. People are more aroused after watching a robot being tortured than when watching a robot being petted (Rosenthal–von der Pütten et al., 2013). Moreover, people’s negative attitudes toward robots decreased significantly after interacting with robots, which in turn explained the significant variance in the overall rating of the robot (Stafford et al., Citation2010). As negative emotions are naturally unpleasant, people tend to perform corrective behaviors or avoid bad behaviors to mitigate them (Izard, Citation1977). This reasoning reflects the importance of including emotions as a factor influencing human behavior. Therefore, in addition to utilitarian attitudes that entail the more rational evaluation of the behavior, we include hedonic attitudes that compose the emotional components of the behavior as determinants of social robot acceptance in our model.
Second, TPB has a narrow conceptualization and focuses solely on social pressure experienced when making decisions about human behavior (Rivis & Sheeran, Citation2003; Sheeran & Orbell, Citation1999). Previous studies have largely used subjective norms to capture the essence of social influence, but their inconsistent findings have led some researchers to question whether these reflect the full extent of social influence (Y. Lee, Lee, & Lee, Citation2006). Therefore, the link between social influence and technology acceptance requires further investigation (Karahanna & Limayem, Citation2000). Only a few empirical studies have investigated the underlying components of normative beliefs (Fisher & Price, Citation1992), and some researchers have suggested the introduction of further dimensions to TPB to tap the complete function of normative beliefs in explaining human behavior (Fisher & Price, 1992; Sheeran & Orbell, Citation1999). Therefore, further exploration regarding additional factors that better explain the normative component is needed. Our model of social robot acceptance, which splits the normative component into a personal and a social element, attempts to achieve this.
Third, although these additions and alterations to the theory provide greater insights into the rational-based and deliberate nature of behavior, its assumption that people consciously act in a certain way could be problematic. In general, psychological research originates from goal-directed human behavior and relies on expectancy-value models of attitudes and decision making, which are rooted in theories of rational choice. TPB may be considered one of the most influential models in this perspective (Aarts, Verplanken, & van Knippenberg, Citation1998). However, humans are the only animal species with the ability for metacognition or to reflect on their actions and their thoughts (Cartwright-Hatton & Wells, Citation1997). For example, when a ball is thrown at someone, their reflex will most likely be to catch the ball without thinking about the action. Similarly, our environment is capable of activating goal-directed behavior automatically, without an individual’s awareness (Bargh & Gollwitzer, Citation1994). Thus, not all human behavior is part of a conscious decision-making process, which is an assumption of TPB. Therefore, we include emotional aspects, as well as automated behavior, in our model of social robot acceptance to overcome this single rational focus in explaining or predicting human behavior.
Fourth, other researchers similarly speculate that TPB overlooks the fact that human behavior is executed on a repetitive, daily basis and therefore may become routinized or habitual (Aarts, Verplanken, & van Knippenberg, Citation1998). People are likely to draw on experiences from similar previous behavior in deciding to perform their current behavior. Although Ajzen (Citation1991) incorporated previous behavior into his theory, he presumed that the impact of past behavior produces feedback through subsequent attitudes and perceptions of social norms and behavioral control. However, as most of our behavioral repertoire is frequently performed in the same physical and social environment, behavior usually becomes habitual in nature (Ouellette & Wood, Citation1998; Triandis, Citation1979). Habits allow us to behave in a rather “mindless” state and therefore may be perceived as automatic behavior. Automatic processes lack conscious attention (i.e., are cognitively efficient), intentionality, awareness, and/or controllability (Bargh & Chartrand, Citation1999). Most habitual behavior arises and proceeds efficiently, effortlessly and unconsciously (Aarts, Verplanken, & van Knippenberg, Citation1998), and technology use is often associated with habitual use (Ortiz de Guinea, & Markus, Citation2009; Peters & Ben Allouch, Citation2005). Thus, by omitting nonrational, routinized, and automatic behavior, TPB may not be suitable to predict human behavior in its original state. Moreover, robots for domestic use should also be socially accepted within our society. This is a process that involves emotional evaluations of the technology in addition to rational decisions to adopt a robot system (Scopelliti, Giuliani, & Fornara, Citation2005; Weiss, Igelsböck, Wurhofer, & Tscheligi, Citation2011). In addition, robots for domestic use must be accepted by households. Thus, although social robot acceptance might be an individual decision, this decision is influenced by the social structure of the household, which argues for the inclusion of a more social perspective if multiple persons are living in one household.
2.4. Toward a Model of Social Robot Acceptance
As just argued, we use the framework of TPB as a starting point in an attempt to explain the (long-term) acceptance of social robots in domestic environments. Some studies revise existing theoretical models by adding an independent variable as a parallel predictor of the dependent variables, together with established predictors. The aim of this approach is to account for more variation by specifying processes formally contained in error terms in the testing of the theory. Such an approach could be characterized as theory broadening. A second approach to the revision of any theory is introducing a variable explaining how existing predictors influence intentions, as many studies have done to expand TPB (Liao, Chen, & Yen, Citation2007; Pavlou & Fygenson, Citation2006; Perugini & Bagozzi, Citation2001; Wand, Citation2011). Here, the idea is to better understand theoretical mechanisms and their effects by introducing a new variable that mediates or moderates the effects of existing variables. Such an approach could be characterized as theory deepening. The goal of this article is to present a conceptual model of social robot acceptance that both expands and deepens TPB. This will be achieved by decomposing the TPB model to a specific technology—in this case, social robots for domestic purposes—as suggested by S. Taylor and Todd (Citation1995). This decomposition allows for the inclusion of factors from other theories (Benbasat & Barki, Citation2007), based on a comprehensive overview of predictors for technology acceptance and behavioral intention from psychology, information systems, communication science, human–computer interaction, and HRI, which have been shown to influence the acceptance and use of technology in general, and robots or virtual agents specifically. As previously indicated, TPB only includes a rational perspective on human behavior. Therefore, factors for affective evaluations and the social context of behavior are included in the proposed model of social robot acceptance, which is presented in the next section.
3. INFLUENTIAL FACTORS FOR SOCIAL ROBOT ACCEPTANCE
Following others (S. Taylor & Todd, Citation1995; Venkatesh & Brown, Citation2001), the three constructs of attitudinal beliefs, social normative beliefs, and control beliefs from TPB will be decomposed to reflect the specific underlying factors, based on a detailed literature review on social robot acceptance. Here, a variety of salient beliefs may be generated, depending on the context of use of a specific technology—in this case, social robots. This course of action exposes the left side of the model (i.e., the influencing factors), which provides an adequate theoretical grounding to incorporate various factors from other theories (Benbasat & Barki, Citation2007). For our study, we included those factors relevant for social robot acceptance. Specifically, the model includes the missing factors influencing the affective and interactive use of social robots (e.g., hedonic attitudes), as well as the social and societal influences (e.g., normative beliefs such as privacy and trust) on robot technology use.
Because intentions are found to be good predictors of specific behavior, they have become a critical part of many contemporary theories of human behavior (Ajzen & Fishbein, Citation2005). Although these theories differ in detail, they all show convergence on a small number of factors that account for much of the variation in behavioral intentions. These factors can be regarded as the three major types of considerations influencing the decision to engage in a given behavior. First, attitudinal beliefs are the anticipated positive or negative consequences of the behavior, which, in the case of social robot acceptance, can be accepted as the user’s evaluation of the beliefs when using a robot in the future. Second, normative beliefs are the anticipated approval or disapproval of the behavior by prevailing norms in the individual’s social environment, which in the scope of this study can be perceived as the user’s evaluation of the prevailing norms regarding the use of a robot. Third, control beliefs are the factors that may facilitate or impede the performance of the behavior, which can be observed here as the contextual factors influencing the use of a robot. Next we present the different factors included in our conceptual model of social robot acceptance. Refer to our previous work for a more detailed discussion on the inclusion of these factors (de Graaf & Ben Allouch, Citation2013a).
3.1. Attitudinal Beliefs Structure
The attitudinal belief structure involves the user’s favorable or unfavorable evaluation of a specific (future) behavior (Ajzen & Fishbein, Citation2005), or in this case the evaluation of behavioral beliefs resulting from the (anticipated) use of a social robot. According to some researchers in human–computer interaction (Hassenzahl, Citation2004; Van der Heijden, Citation2003), there are both utilitarian and hedonic product aspects to the attitudinal belief structure. Utilitarian aspects are attributes involved in the practicality and usability of a product. In contrast, hedonic aspects are attributes relating to the user’s experience when using a product. The dichotomy of both utilitarian and hedonic attitudes as determinants of technology acceptance also arises from motivation theory, suggesting a main classification between extrinsic and intrinsic motivators of human behavior, which are based on the different reasons or goals that encourage a person’s actions (Ryan & Deci, Citation2000; Vallerand, Citation1997). Extrinsic motivation refers to doing something because it leads to a separate valued outcome (e.g., utilitarian attitudes). Intrinsic motivation relates to the performance of an activity for no apparent reinforcement other than for the process of performing that behavior itself (e.g., hedonic attitudes). Intrinsic motivations are expected to be a powerful incentive of human behavior, as a person can autonomously decide on a course of action (Deci & Ryan, Citation1985). Because this article examines social robot acceptance in the context of voluntary use, intrinsic motivations or hedonic attitudes should therefore be among the influential factors under study.
Several utilitarian attitudes can be deduced from general acceptance literature as being important factors in the context of HRI, namely, usefulness (Chin & Shoo, 2011; Fink, Bauwens, Kaplan, & Dillenbourg, Citation2013; Heerink et al., Citation2010), ease of use (Chin & Shoo, 2011; Heerink et al., Citation2010), and adaptability (Broadbent, Stafford, & MacDonald, Citation2009; Fong, Nourbakhsh, & Dautenhahn, Citation2003; Goetz, Kiesler, & Powers, Citation2003; Heerink et al., Citation2010; Shin & Choo, Citation2011). For social robot acceptance, several studies (Bartneck, Kulić, Croft, & Zoghbi, Citation2009; Cuijpers, Bruna, Ham, & Torta, Citation2011) point to the utilitarian attitude of perceived intelligence as an influential factor in user evaluations. Regarding the hedonic attitudes, well-known factors in technology acceptance research are enjoyment and attractiveness, which have also been shown to be crucial factors in HRI (Heerink et al., Citation2010; Shin & Choo, Citation2011). For social robots specifically, the factors of anthropomorphism (Heerink et al., Citation2010; Kahn Ishiguru, Friedman, & Kanda, Citation2006; K. Lee et al., Citation2005; K. M. Lee, Jung, Kim, & Kim, Citation2006; Salem, Eyssel, Rohlfing, Kopp, & Joublin, Citation2013), realism (Bartneck, Kanda, Mubin, & Al Mahmud, Citation2009; Goetz et al., Citation2003; Groom et al., Citation2009), sociability (Breazeal, Citation2003; de Ruyter, Saini, Markopoulos, & van Breemen, Citation2005; Fong et al., Citation2003; Heerink et al., Citation2010; Joosse, Sardar, Lohse, & Evers, Citation2013; Mutlu, Citation2011; Shin & Choo, 2012), and companionship (Dautenhahn et al., Citation2005; de Graaf, Ben Allouch, & Klamer, Citation2015; K. M. Lee et al., Citation2006) also influence the user experience and acceptance of these types of robots.
The attitudinal beliefs of social robot acceptance compose both utilitarian and hedonic attitudes of HRI. Including both types of attitudinal beliefs allows for the broadening of the view that robots are social actors in an interaction scenario and enables the evaluation of interactive and pleasure-oriented, as well as usability, aspects. There is thus an acknowledgment of the unique factors that distinguish social robots as a new technological genre (de Graaf, Ben Allouch, & van Dijk, Citation2015; Young et al., Citation2011), which demonstrates the need to include these unique factors, as well as the traditional antecedents, in human–computer interaction. Several sources in the information systems literature (e.g., Agarwal & Karahanna, Citation2000; Y. Lee et al., Citation2003) and the HRI literature (e.g., Heerink et al., Citation2010; K. M. Lee et al., Citation2006; Shin & Choo, Citation2011) indicate that hedonic attitudes directly influence the utilitarian attitudes of system use or social robot use. In addition, renowned human technology use behavior theories (Ajzen, Citation1991; Rogers, Citation2003) indicate that attitudinal beliefs influence people’s intentions to perform a particular behavior. These interrelationships result in the following hypotheses:
H1:
The users’ utilitarian attitudes of a robot directly influence their intention to use that robot.
H2:
The users’ hedonic attitudes of a robot directly influence their intention to use that robot.
H3:
The users’ hedonic attitudes of a robot directly influence their utilitarian attitudes of that robot.
3.2. Normative Beliefs Structure
Social context plays an important role in technology acceptance, especially in early adoption behavior (Rogers, Citation2003). Yet only a few empirical studies have investigated the underlying components of normative beliefs (Fisher & Price, Citation1992). Miniard (Citation1981) argued that the normative beliefs structure comprises both social normative and personal normative components. The social component encompasses an individual’s belief regarding the likelihood and importance of the social consequences of performing a particular behavior. The personal component refers to an individual’s belief that engaging in a behavior leads to salient personal beliefs, which are related to what is perceived as the norm within one’s social environment.
The technology acceptance literature focuses largely on the normative concepts of social influence and status (Y. Lee et al., Citation2003). To our knowledge, only the effects of social influence have been studied to date in the context of social robot acceptance (Heerink et al., Citation2010; Shin & Choo, Citation2011). However, if other important role-players support the use of an innovation, it is believed that using that innovation will elevate one’s status within that group (Fisher & Price, Citation1992; Rogers, Citation2003; Venkatesh & Davis, Citation2000). Social robots, being a relatively new technology in the consumer market, might also be subject to this status process. In terms of personal norms, privacy, trust (Cramer et al., Citation2008; DeSteno et al., Citation2012; Hancock et al., Citation2011; Li, Rau, & Li, 2010), and societal impact (Nomura, Kanda, Suzuki, & Kato, Citation2006; Nomura, Kanda, Suzuki, Yamada, & Kato, Citation2009; Nomura et al., Citation2008) factors have been shown to influence the user evaluation and acceptance of these autonomous robot systems.
This study conceptualizes a distinction between social and personal norms, which to our knowledge are not yet included in theories of technology acceptance or human behavior. Therefore, for now, the theoretically grounded relations between normative beliefs and other factors in the model are assumed for both social and personal norms, because personal norms arise from beliefs considered to be the norm in one’s social environment. Social system factors influence the knowledge a person possesses and upon which opinions about using a technology are based (Rogers, Citation2003). Thus, a person’s normative beliefs directly affect that individual’s attitudinal beliefs. This theoretical interrelation between normative beliefs and attitudinal beliefs has been acknowledged in both the information systems literature (e.g., Ben Allouch, van Dijk, & Peters, Citation2009; Y. Lee et al., Citation2003; Yu, Ha, Choi, & Rho, Citation2005) and the HRI literature (e.g., Heerink et al., Citation2010; Shin & Choo, Citation2011). In addition, renowned theories of human technology use behavior (Ajzen, Citation1991; Venkatesh et al., Citation2003) indicate that normative beliefs influence people’s intentions to perform a particular behavior. These interrelationships result in the following hypotheses:
H4:
The users’ personal norms, involving the use of a robot, directly influence their intention to use that robot.
H5:
The users’ social norms, involving the use of a robot, directly influence their intention to use that robot.
H6:
The users’ personal norms, involving the use of a robot, directly influence their utilitarian attitudes of that robot.
H7:
The users’ personal norms, involving the use of a robot, directly influence their hedonic attitudes of that robot.
H8:
The users’ social norms, involving the use of a robot, directly influence their utilitarian attitudes of that robot.
H9:
The users’ social norms, involving the use of a robot, directly influence their hedonic attitudes of that robot.
H10:
The users’ social norms, involving the use of a robot, directly influence their personal norms involving the use of that robot.
3.3. Control Beliefs Structure
Psychology research, and research on TPB in particular, has established inhibiting effects or constraints for the intention to perform a behavior, as well as for the behavior itself (Ajzen, Citation1991). Control beliefs consist of the user’s beliefs about salient control factors, meaning their beliefs about the presence or absence of resources, opportunities and obstacles that may facilitate or impede the performance of the behavior.
For social robot acceptance, the control belief of previous experiences (Broadbent et al., Citation2009; Fong et al., Citation2003), either with robots or technology in general, has shown to affect acceptance. This is particularly true of people who have not yet had a chance to fully interact with robots (de Graaf, Ben Allouch, & van Dijk, Citation2016). Previous interactions with robots enhance the user’s self-efficacy in using that robot (Ahlgren & Verner, Citation2009; Liu, Lin, & Chang, Citation2010), which in turn increases robot acceptance (Bartneck, Suzuki, Kanda, & Nomura, Citation2007). Other relevant control beliefs for social robot acceptance are safety (Bartneck et al., Citation2009; Young et al., Citation2007) and anxiety toward robots (Nomura et al., Citation2008), which have been shown to influence the user’s evaluation and acceptance of such systems. In addition to these HRI contextual factors, we argue for the inclusion of the factors personal innovativeness and cost in a conceptual model of social robot acceptance. The core aspect of the control beliefs is self-efficacy and is related theoretically to the concept of perceived behavioral control in Ajzen’s TPB (Citation1991). Self-efficacy is mainly relevant for novice users, who have not yet acquired the requisite skills to successfully perform the behavior (LaRose & Eastin, 1994). As social robots are not widespread in society, most people are unfamiliar with these systems. Some people are more willing to experiment with or try out innovative technologies, conceptualized by Serenko (Citation2008) as personal innovativeness. In the consumer context, people are responsible for the expenses associated with technology use. The perceived cost is found to be an additional barrier to the adoption of home technologies (S. A. Brown & Venkatesh, Citation2005). Thus, perceiving a robot as an expensive item might be another determining factor when evaluating social robot acceptance.
A renowned theory of technology use behavior, social cognitive theory (LaRose & Eastin, 2004), indicates that people’s self-efficacy, perceived as the core of a person’s control beliefs as defined in TPB (Ajzen, Citation1991), influences their attitudinal beliefs. This theoretical interrelation between control beliefs and attitudinal beliefs has been found in several studies in both information systems (Hackbarth, Grover, & Yi, Citation2003; Karahanna & Limayem, Citation2000) and HRI literature (Bartneck et al., Citation2007b). Consequently, our model of social robot acceptance defines a direct influence of control beliefs on both attitudinal beliefs structures. Moreover, prominent theories on human behavior (Ajzen, Citation1991; Bandura, Citation1977) indicate that control beliefs are affected by social network opinions. Thus, our model of social robot acceptance will incorporate the effect of social norms on control beliefs. In addition, several theories including TPB (Ajzen, Citation1991) and UTAUT (Venkatesh et al., Citation2003) indicate that control beliefs influence a user’s intention to use a technology. These interrelationships result in the following hypotheses:
H11:
The users’ control beliefs, involving the use of a robot, directly influence their intention to use that robot.
H12:
The users’ control beliefs, involving the use of a robot, directly influence their utilitarian attitudes of that robot.
H13:
The users’ control beliefs, involving the use of a robot directly, influence their hedonic attitudes of that robot.
H14:
The users’ social norms, involving the use of a robot, directly influence their control beliefs, involving the use of that robot.
3.4. The Conceptual Model
Relevant theories of technology acceptance, together with findings from HRI research, have identified the importance of considering different factors regarding the robot and the user, as well as the context of use. The proposed conceptual model of social robot acceptance, as visualized in , advances existing technology acceptance and robotics research by introducing new factors into TPB and adapts it for the new social robot acceptance context. This literature review has revealed three key acceptance categories that are important when evaluating social robot acceptance in domestic environments. The first category comprises the attitudinal beliefs, including both utilitarian and hedonic attitudes, which reflect the user’s evaluation of the beliefs when using a robot. The second category consists of the normative beliefs, including both personal and social norms that entail the user’s evaluation of the prevailing norms involving using a robot. The third category encompasses the control beliefs composing the contextual factors that play a role when using a robot. By adding components for affective evaluations (i.e., hedonic attitudes) and the normative beliefs regarding behavior (i.e., social and personal norms), our conceptual model of social robot acceptance endeavors to overcome the shortcomings of Ajzen’s (Citation1991) TPB model, which approaches human behavior from a rational and purely psychological perspective. This article thus contributes to the literature on the HRI by modeling the behavioral processes that attempt to explain the intention to use social robots.
4. METHOD
4.1. Sampling of Participants
In December 2013, 4,750 people, representative of the Dutch population, were invited via e-mail to voluntarily participate in our study. In total, 1,649 people started the questionnaire, of whom 1,248 completed it. This yielded a response rate of 26.3%. A reasonable explanation for the dropout during the 80-item questionnaire is related to its relatively long length. It took the participants on average 15 min to complete the questionnaire. Among the completed questionnaires, 86 were removed from the data because of respondents straight-lining the answers. This resulted in the final number of completed questionnaires included in further data analysis of 1,162. The demographic characteristics of the participants included in the final sample are displayed in , together with the demographics of the general Dutch population (Central Bureau of Statistics, 2013). It shows that the sample used in our study serves as a satisfactory representation of the Dutch population.
4.2. Design of the Questionnaire
An online survey was designed to investigate the anticipated acceptance of a social robot in people’s own homes. The questionnaire contained two parts. The first part of the questionnaire collected the demographic data (i.e., gender, age, educational level, income, and household type) from the participants, together with the more static traitlike and general constructs. These were personal innovativeness measured with the scale presented in Agarwal and Karahanna (Citation2000), and anxiety toward discourse with robots measured with the similarly named subscale from Nomura et al. (Citation2008). Both constructs belong to the control beliefs and were assumed to be stable, traitlike concepts. Therefore, it was our goal to measure the items of these concepts without any interference from the other items or descriptions used in the questionnaire. The items were presented on a 7-point Likert scale.
The goal of the second part of the questionnaire was to empirically test the conceptual model of social robot acceptance and started with an open question asking what first comes to mind when thinking of the word robot. The qualitative analyses of the associations have been presented elsewhere (de Graaf & Ben Allouch, Citation2016) and show that people conceptualize robots as autonomous machines, endowed with artificial intelligence but lacking consciousness and emotions, that are able to switch between several tasks when helping human users. Afterward, a definition of social robots was given:
Social robots are created in such a way that they can operate independently in our everyday environments, such as our home. Social robots can understand everyday social situations and react according human social norms. Regarding social situations includes conversations between people as well as how we ought to behave in the presence of other people. Social robots work with us and are able to communicate with us in a humanlike way through speech interactions with supportive gestures and facial expressions.
In addition, because our focus is on domestic use of robots, we provided a short description of potential use purposes:
There are different applications for social robots at home. For example, a robot could do several chores in and around the home according to one’s personal preferences, is connected to an online database enabling it to answers all your questions, or build upon online shared stories by other humans to provide social support to its user.
Afterward, the participants were confronted with different statements about the participants’ expectations of social robots and their related behavioral expectations regarding the use of such robots. The statements represent all the acceptance factors as presented in the conceptual model. The outcome variable was use intention (e.g., “Assuming I have a robot, I will frequently use it in the future”) measured with the similarly named scale from Moon and Kim (Citation2000). For the utilitarian attitudinal beliefs, these were usefulness (e.g., “I think a social robot would be useful to me”), ease of use (e.g., “I think I would know quickly how to use a social robot”), and adaptability (e.g., “I think a social robot would be adaptive to what I need”) measured with the similarly named scales as in Heerink et al. (Citation2010). For the hedonic attitudinal beliefs these were enjoyment (e.g., “I would enjoy a social robot talking to me”) measured with the scale from Heerink et al. (Citation2010), attractiveness (e.g., “I think a social robot would look quite pretty”) measured with the Physical Attraction scale from McCroskey & McCain (Citation1974), animacy (e.g., “A social robot would be: dead … alive”) with the scale from Bartneck et al. (Citation2009), social presence (e.g., “Interacting with a social robot would feel like interacting with an intelligent being”) with the scale from Biocca et al. (Citation2003), sociability (e.g., “A social robot would feel comfortable in social situations”) with the Social Competence scale from R. B. Rubin and Martin (Citation1994), and companionship (e.g., “I would be able to establish a personal relationship with a social robot”) measured with the Social Attraction scale from McCroskey et al. (Citation1974). For the social normative beliefs, these were social influence (e.g., “People would find it interesting to use a social robot”) measured with the scale from Karahanna and Limayem (Citation2000), and status (e.g., “People who would own a social robot would have more prestige than those who do not”) measured with the scale from Moore & Benbasat (1991). For the personal normative beliefs privacy concern measured with the subscale of Privacy Concern of Data Collection (e.g., “It would bother me if I had to give personal information to a social robot”) from Malhotra, Kim, and Agarwal (Citation2004), trust (e.g., “A social robot should be: dishonest … honest”) measured with the subscale Trustworthiness from McCroskey and Teven (Citation1999), and societal impact of robots (e.g., “I feel that society will be dominated by robots in the future”) measured with the subscale Social Influence of Robots from Nomura et al. (Citation2008). Finally, for the control beliefs these were self-efficacy (e.g., “I would be able to use a social robot if someone showed me how to do it first”) measured with the scale from Bandura (Citation1977), safety (e.g., “Being near a social robot would make me feel: anxious … relaxed”) measured with the scale from Bartneck et al. (Citation2009), and cost (e.g., “I think social robots would be quite pricy”) measured with the scale from S. A. Brown and Venkatesh (Citation2005). The statements in the questionnaire were randomized. Both Likert scales and semantic differentials were included in the questionnaire to prevent monotony. All answers contained 7-point scales. To obtain a more compact measurement model, some scales were reduced to three items based on the factor loadings in a pretest sample from the same participant’s database (n = 100). Incorporating fewer items from validated constructs in a questionnaire leads to a more parsimonious model and lowers the burden on the participants (Kline, Citation2011).
4.3. The Measurement Model
When doing SEM, the latent variable measurement specification uses the Jöreskog (Citation1969) confirmatory factor analysis (CFA) model. Although this encourages researchers to formalize their measurement hypotheses and makes the definition of the latent variables better grounded in subject matter theory leading to parsimonious models, CFA also assumes a strong basis in theory with thorough prior analysis under diverse conditions (Asparouhov & Muthén, Citation2009). It would be too ambitious and practically not feasible in the current study to test a complete and assumed fixed theory in the relatively unexplored field of real-world HRI research with new challenges where exploration precedes causal theory building. Another disadvantage is that a CFA approach requires strong measure conditions that are often not available in practice. Measurement instruments often have many small cross-loadings that are well motivated by either substantive theory or the formulation of the measurements (i.e., the items in the questionnaire). Fixing the cross-loadings to be zero may therefore force researchers to specify a more parsimonious model than is actually suitable for the data (Asparouhov & Muthén, Citation2009; Morin, Marsh, & Nagengast, Citation2013). Together, this contributes to poor applications of SEM where the believability and replicability of the final model is in doubt. Moreover, fixing factor loadings at zero tends to give distorted factors, as the correlation between items representing different variables is forced to go through their main factors only (Asparouhov & Muthén, Citation2009). This process usually leads to overestimated factor correlations and subsequent distorted structural relations. It is thus important to extend SEM to allow less restrictive measurement models to be used together with the traditional CFA models.
Establishing the First-Order Factor Model
Before developing a structural model of social robot acceptance, it is essential to have a measurement model fitting to the data. The first step is to explore how the items fit into clusters with factor analysis. An exploratory factor analysis (EFA) was executed to check for construct validity to obtain evidence that the items from the questionnaire load onto separate factors in the expected manner (Brown, Chorpita, & Barlow, Citation1998). EFA is an exploratory and descriptive technique to determine the appropriate number of common factors and to uncover which measured items are reasonable indicators of the constructs (T. A. Brown, Citation2006). EFA was performed in Mplus version 7.11 developed by Muthén and Muthén (Citation1998–2012) to analyze the intended measurement model, which included all items. Consecutively, several measurement models were run with a varying number of factors but included all the items.
All analyses used an oblique (Geomin) rotation as factors were expected to be interrelated (Sass & Schmitt, Citation2010). In addition, oblique rotation is preferred when aiming at CFA that fits the data well (T. A. Brown, Citation2006). For the extraction, the maximum likelihood method was used to estimate the common factors. This was done because this is most frequently used with continuous indicators when the data are normally distributed (T. A. Brown, Citation2006) and because it has the desirable asymptotic properties of being unbiased, consistent, and efficient (Kmenta, Citation1971).
As the total questionnaire contains 20 scales, it was expected to find 20 separate factors in the EFA. Therefore, several models were run with factor variations from 17 to 23 factors. In the end, a 19-factor set was considered to be most suitable based on the Akaike information criterion (AIC) and Bayesian information criterion (BIC) indices. Moreover, when a model was run with more than 19 factors, the additional factors contained no factor loadings above the value of .3 and thus did not represent a new concept or construct within the data. Each factor comprises a unique set of items belonging to separate constructs. The model fit indices of the first EFA solution are presented in . The chi-square values were not reported, as they are always nonsignificant with large sample sizes, and even small differences between the observed model and the perfect-fit model may lead to nonsignificant results (Jöreskog, Citation1969). Moreover, there seems to be an overreliance toward overall goodness of fit indices as in actuality models with good fit indices could still be considered poor based on other measures (Chin, Citation1998).
The first EFA, where all items were included, provided a root mean square error of approximation (RSMEA) and standardized root mean square residual (SRMR) that both indicate a good model fit (Morin et al., Citation2013). Moreover, also the comparative fit index (CFI) and Tucker–Lewis index (TLI) both indicate a good model fit (Hox & Bechger, Citation1998; Hu & Bentler, Citation1999). Altogether, these fit indices indicate an acceptance measurement model after the first run. However, despite the acceptable model fit, it is chosen to exclude those items from the analysis that poorly loaded onto its unique factor. In total, three items (RAS01, RAS02, and SP02) were removed before a second EFA was run. Results of this second solution are also presented in . The fit indices of the CFI and TLI are increased—.981 and .960, respectively—and the AIC and BIC are decreased to 212,976 and 218,902, respectively. This points to an improved model fit. However, in this second solution, two items with cross-loadings on other factors occurred. In the third EFA analysis, these two items (PU03 and PR03) were removed from the analysis, and the model fit indices are also reported in . The fit indices of the CFI and TLI increased—.986 and .970, respectively—and the AIC and BIC decreased again to 206,466 and 212,180, respectively. However, once more, an item that poorly loaded onto its unique factors occurred. Thus, a fourth EFA analysis ran without this item (PAD01). The fit indices of the CFI and TLI did not change much—.986 and .971, respectively—but the AIC and BIC decreased again, to 203,311 and 209,819, respectively. Although a few cross-loadings still existed, it was chosen to continue with this fourth and final solution as eliminating any more items from the model did not improve the model fit indices (see ). The final factor solution is shown in .
The items of the final factor solution of the explorative factor analysis were examined for internal consistency using coefficients of Cronbach’s alpha. All constructs had a coefficient above .70 and were considered to be reliable measures (Nunnally & Bernstein, Citation1994). Once the final exploratory factor model had been established, the robustness of the data was tested to ensure the continuance with CFA. The large number of parameters and latent variables within the data set causes the measurement model to be very complex. Continuing with CFA is preferred, because it allows data analysis with a simpler model (Browne, Citation2001). Some researchers (Morin et al., Citation2013) argue that it is a commonly used approach “to use exploratory EFA to ‘discover’ an appropriate factor structure and then incorporate this post hoc model into a CFA framework” (p. 400). Although some purist may be offended by this approach as it blurs the distinction between EFA and CFA, Morin et al. (Citation2013) do not instantly discard this approach as long as researchers are careful with their interpretations and apply them with appropriate caution.
Testing for robustness means that a small part of the measurement model—in this case, the weakest part according to the final exploratory factor solution—is run in both an EFA and CFA setting. In the EFA all items are related to the defined number of factors, and in the CFA the relation between the items and its latent variable are predefined. In addition, an intermediate model is tested, which includes only the observed significant relations in the EFA. All this is done for a small part of the model—in this case, the items of adaptability, enjoyment, companionship, sociability, cost, and privacy concern. Reasons for the inclusion of these items in the robustness test is the cross-loading of the items of adaptability (PAD02 and PAD03) on the factor of enjoyment, the cross-loading of an item of sociability (SB03) on both the factors of companionship and adaptability, and the cross-loading of an item of privacy concern (PR04) on the factor of cost. The three models (e.g., EFA model, intermediate model, and CFA model) are depicted in .
FIGURE 5. From left to right: The exploratory factor analysis model, the intermediate model, and the comparative factor analysis model.
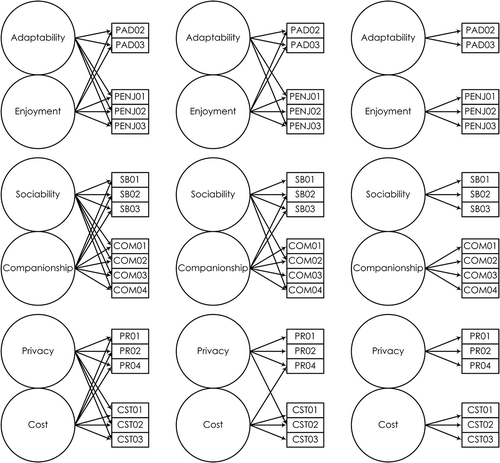
When the measurement model is considered to be robust based on the model fit indices, it is acceptable to continue with a confirmatory SEM approach. As continuing with the CFA approach is preferred for reasons of simplicity, the CFA model is chosen when the change in AIC and BIC values, compared to the EFA and intermediary model, is relatively small and the model fit indices show an acceptable to a good fit. presents the results of the robustness tests. The robustness analysis shows that the model fit indices overall decrease from the EFA to the CFA setting. Nevertheless, they still indicate a good to acceptable model fit, and the changes in AIC and BIC are relatively small. The baseline of this research is the theoretical background as hypothesized in the conceptual model. Statistical analysis is used as a means to confirm (or reject) the theoretical-based hypotheses. Thus, it is decided to continue with a CFA approach.
The final measurement model from the EFA setting was rerun in a CFA setting. With CFA, the indicated relations of the observed items are specified to the latent variables in advance allowing for correlations between the various latent variables (Anderson & Gerbing, Citation1988). The prespecified factors solution is evaluated in terms of how well it reproduces the sample correlation or covariance matrix of the measured items, and thus requires a strong empirical or conceptual foundation to guide the specification and evaluation of the factor model (T. A. Brown, Citation2006). As the constructs are both defined by theory (conceptual foundation) and emerged from the EFA (empirical foundation), carrying on with CFA was deemed appropriate. shows that the model fit indices of the CFA model are acceptable.
Establishing the Second-Order Factor Model
Once the first-order CFA had been completed, the next step was to examine the magnitude and pattern of correlations among the factors in the first-order solution before trying to fit the second-order factor analysis (Kline, Citation2011). In the first-order factor analysis, correlations among the factors were assumed based on the theory as hypothesized in the conceptual model, hence the use of an oblique rotation in the first-order factor analysis. One goal of second-order factor analysis is to provide a more parsimonious theory-based account for the correlations among the first-order factors (T. A. Brown, Citation2006). These specifications assert that the second-order factors have direct effects on the first-order factors. These direct effects and correlations within the second-order factors are responsible for the covariation among the first-order factors. According to Chen, Sousa, and West (Citation2005), a second-order factor model has several potential advantages over a first-order factor model. First, the second-order model can test whether the hypothesized higher order factor actually accounts for the pattern of relations between the first-order factors. Second, a second-order factor model puts a structure on the pattern of covariance between the first-order factors, explaining the covariance in a more parsimonious way with fewer parameters, which has also been denoted by Gustafsson and Balke (Citation1993) and Rindskopf and Rose (Citation1988). Third, a second-order factor model separates variance due to specific factors from measurement error, leading to a theoretically error-free estimate of the specific factors. Finally, second-order factor models can provide useful simplification of the interpretation of complex measurement structures.
Tests of validity for a second-order factor model follow the same rules of identification as for a first-order factor model. Thus, similar thresholds for model fit will be applied here. The first step of validating the second-order factor structure is to determine if the implicit constrains are realistic (Chin, Citation1998). This can be done by examining the correlations among the different first-order factors included in the model. presents these correlations along with its expected second-order factor structure. According to R. Taylor (Citation1990), correlation coefficients below or equal to .35 are considered as weak correlations, between .36 and .67 as moderate correlations, and greater than .67 as strong correlations. The second-order factor structure can be validated by the observation of (a) high correlations between the first-order factors that are expected to form a second-order factor together and (b) weak correlation between those first-order factors that are not expected to be part of the same second-order factor. As shown in , no clear pattern for a second factor structure can be derived from the correlations. Although some moderate to strong correlations exist for the hedonic attitude beliefs structure, other correlations in these groups are weak. Moreover, the correlations among the personal normative and control beliefs structure are mostly weak to nonsignificant. Based on these results it is probably impossible to obtain acceptable fit indices for the second-order factor model as hypothesized in the conceptual model.
The next step to validate the second-order factor structure is to demonstrate the convergent validity of the first-order factors by examining the strength of the paths connecting the second-order factors to the first-order factors (Chin, Citation1998). Because the measurement model should theoretically contain more than two second-order factors (e.g., utilitarian attitudes, hedonic attitudes, personal norms, social norms, and control beliefs) and correlations among the second-order factors are assumed, at least two first-order factors per second-order factor are necessary to be able to identify the model (Rindskopf & Rose, Citation1988). As a first step, the three second-order factor structures were run independently. The final results are presented in . As shown in , most model fit indices indicate a good fit; however, the TLI for both the attitudinal and control beliefs structure is slightly lower than aimed for (Kenny & McCoach, Citation2003). Nevertheless, because there were no other suggested modification indices with sufficient impact on the model fit, it was decided to continue with testing the model fit of the complete second-order factor model.
As the final step to validate the second-order factor structure, the paths and model fit should still hold when applied in a nomological network of other factors (Chin, Citation1998). shows the model fit indices of the second-order factor model. Although the RMSEA shows a good fit, all other model fit indices are not at an acceptable level. The poor model fit could be a result of low data quality or simply the lack of fit between the data and the conceptual model based on theory. Especially based on the high value of the SRMR, one reason could be that, because the three beliefs structures indicated (nearly) acceptable model fits individually, the interrelations between the separate beliefs structures result in misspecification of the model when put together. This argument is supported by the presence of correlations among the second-order factors greater than one (see ). Empirical underidentification can occur in a case of near zero or near unity correlations among the second-order factors (Rindskopf & Rose, Citation1988). One option could be to constrain the correlations between utilitarian attitudes and control beliefs and between social norms and control beliefs to 1. However, this is allowed only when the confidence interval of the correlation contains 1 (Muthén & Muthén, Citation1998–2012), and this is not the case for the correlation between utilitarian attitudes and control beliefs which is 1.086 [1.031, 1.141].
Based on the preceding results, it can be concluded that the second-order factor structure as hypothesized in the conceptual model does not fit the collected data. The theoretical implications of this result are addressed in the Discussion section. To pursue with data analysis without completely abandoning the conceptual model of social robot acceptance, it was decided to continue with testing the same interrelations as hypothesized between the second-order factors but then directly between the underlying first-order factors. This enables the identification of the specific components that account for the users’ acceptance of social robots in domestic environments
5. RESULTS
Based on the measurement model, this section tests the structural model of social robot acceptance and reports on the proposed hypotheses in the conceptual model using Mplus version 7.11 developed by Muthén and Muthén (Citation1998–2012). The original model showed that the model fit was acceptable for the RMSEA and SRMR but not quite acceptable for the CFI and the TLI (see ). Post hoc modification indices suggested that specifying six correlations between first-order factors would increase the model fit. These were three correlations of factors from the hedonic attitudes (e.g., enjoyment with sociability, enjoyment with companionship, and attractiveness with animacy) and three correlations of factors from the control beliefs (e.g., personal innovativeness with anxiety toward robots, personal innovativeness with safety, and anxiety toward robots with safety). Our theoretical framework suggested that each correlation would be part of a second-order factor structure as hypothesized in the conceptual model. This would justify the existence of these correlations, and it was decided to add these six suggested correlations in a second run of the model and the model fit increased to an almost acceptable level (see ). To further increase the model fit, a last correlation pair as suggested in the post hoc modification indices was added (i.e., societal impact with anxiety toward robot). Although these two concepts were not hypothesized as belonging to the same second-order factor, its inclusion in the model can be supported by the high correlation between the two concepts that has been reported in multiple studies (e.g., Dautenhahn & Saunders, Citation2011; de Graaf & Ben Allouch, Citation2013b; Nomura et al., Citation2008). With the inclusion of this correlation, the model fit increased to an acceptable level in the final model (see .
5.1. Interpreting the Effects of the Attitudinal Beliefs
Once a good model fit was established, the hypothesized regression paths were interpreted. Examining the attitudinal beliefs structure, it was hypothesized that utilitarian attitudes influence use intention (H1). The results indicate that use intention cannot be explained by either one of the utilitarian attitudes (see ). These results lead to the rejection of Hypothesis 1.
On the other hand, examining the results in , two of the six hedonic attitudes, could significantly explain use intention (H2). When the participants expected to enjoy having a social robot in their home (β = .531, p < .001), and expected that robot to be less sociable (β = –.099, p = .029), they had higher intentions to use it. These results partially support Hypothesis 2.
Furthermore, it was hypothesized that hedonic attitudes affected utilitarian attitudes (H3). Examining the results in , it is shown that almost half of the regression paths were significant. When participants expected they would enjoy having a social robot in their home, they believed that the robot would be easier to use (β = .307, p = .018) and more adaptive to their personal needs (β = .532, p < .001). In addition, when the participants expected a social robot to be more sociable (β = .211, p = .002) but offers less companionship (β = –.239, p < .001), they thought such a robot would be more capable to adapt to their personal needs. These results partially support Hypothesis 3.
5.2. Interpreting the Effects of the Normative Beliefs
Examining the normative beliefs structure, it was hypothesized that personal norms influence use intention (H4). The results show that use intention could be significantly explained only by one personal norm (see ). Participants who expected less privacy concerns when having a social robot in their home had higher intentions to use such a robot (β = –.059, p = .022). These results weakly support Hypothesis 4.
A similar pattern was found for the influence of social norms on use intention (H5), where also only one regression path was significant (see ). Participants who expected that having a social robot increased their status had higher intentions to use such a robot (β = .100, p = .001). This leads to partially support for Hypothesis 5.
Furthermore, it was hypothesized that personal norms influenced utilitarian attitudes (H6). Examining the results in , it is shown that none of the regression paths are significant, which leads to a rejection of Hypothesis 6.
In addition, it was hypothesized that personal norms would have an effect on hedonic attitudes (H7). The results show that almost half of the regression paths are significant (see ). When the participants expected fewer privacy concerns when having a robot in their home, they believed they would enjoy that robot more (β = –.151, p < .001); would find the robot more attractive (β = –.057, p = .025), more animate (β = –.151, p < .001), and more socially present (β = –.104, p = .003); and the robot would provide more companionship (β = –.193, p < .001). In addition, when participants expected they could trust a social robot in their home, they would find that robot more attractive (β = .885, p < .001), more animate (β = .552, p < .001), and more sociable (β = .178, p < .001). These results partially support Hypothesis 7.
It was also hypothesized that social norms affect utilitarian attitudes (H8). Examining the results in , it is shown that none of the social norms could significantly explain any of the utilitarian attitudes, which leads to the rejection of Hypothesis 8.
In addition, it was hypothesized that social norms would have an effect on hedonic attitudes (H9). The results show that three of the regression paths are significant (see ). When the participants expected that having a social robot in their home would increase their status, they thought the robot would be more enjoyable (β = .135, p < .001) and more socially present (β = .230, p < .001) and would offer more companionship (β = .273, p < .001). These results somewhat weakly support Hypothesis 9.
In addition, it was hypothesized that social norms would affect personal norms (H10). Examining the results in , it is shown that all regression paths are significant. When the participants expected to experience more social influence, they thought that having a social robot in their home would involve fewer privacy concerns (β = –.168, p = .002) and that they could trust that such a robot more (β = .511, p < .001), and they expect smaller societal impact from such robots (β = –.318, p < .001). Moreover, when the participants expected that having a social robot in their home would increase their status, they thought that such a robot would involve less privacy concerns (β = –.098, p = .028) but could be trusted less (β = –.264, p < .001) and would elicit a greater societal impact (β = .108, p = .031). These results fully support Hypothesis 10
5.3. Interpreting the Effects of the Control Beliefs
Examining the control beliefs, the conceptual model hypothesized that control beliefs directly influence use intention (H11). The results show that only one control belief could significantly explain use intention (see ). When participants expected to have the necessary skills to use a social robot in their home, they had higher intentions to use such a robot (β = .267, p = .034). These results weakly support Hypothesis 11.
In addition, it was hypothesized that control beliefs influence utilitarian attitudes (H12). Examining the results in , it is shown that almost half of the regression paths are significant. Participants who evaluated themselves as more innovative (β = .214, p < .001) expected that using such a robot would be more safe (β = .318, p < .001), that such a robot would be more expensive (β = .067, p = .033), and that a social robot would be easier to use. Moreover, participants who thought that such a robot would be more expensive (β = .079, p = .002) expected that a social robot would be able to better adapt to their personal needs. These results demonstrate that Hypothesis 12 is partially supported.
It was also hypothesized that control beliefs would affect hedonic attitudes (H13). Examining the results in , it is shown that almost half of the regression path is significant. When the participants felt more capable of using a social robot, they expected to enjoy using such a robot more (β = .465, p < .001); to find it more animate (β = .383, p = .007), more socially present (β = .370, p < .001), and more sociable (β = .645, p < .001), and that such a robot could provide more companionship (β = .601, p < .001). Moreover, when participants evaluated themselves as more innovative, they expected that using a social robot in their home would be more enjoyable (β = .123, p < .001). Also, when participants indicated that they would feel less anxiety toward talking to a social robot, they expected to experience more companionship from such a robot (β = .165, p = .014). In addition, when participants stated they would feel safe being around a social robot, they thought such a robot would be more enjoyable (β = .173, p < .001) and could provide more companionship (β = .165, p = .014). Finally, when the participants evaluated a social robot as more expensive, they thought such a robot would be more sociable (β = .127, p < .001) but should provide less companionship (β = –.102, p = .002). These results partially support Hypothesis 13.
Finally, the conceptual model hypothesized that social norms influence the control beliefs (H14). Except for one, the results show that all regression paths are significant (see ). When the participants expected to experience more social influence, they thought they would be more capable to use a social robot (β = .733, p < .001), they evaluated themselves as more innovative (β = .376, p < .001), they were less anxious to talk to a robot (β = –.418, p < .001), they would feel more safe around a robot (β = .617, p < .001), and they would perceive such a robot as more expensive (β = .234, p < .001). Moreover, when the participants expected that having a social robot in their home would increase their status, they thought they would be more capable of using such a robot (β = .163, p < .001), they would feel more anxious to talk to such a robot (β = .309, p < .001), and they would feel less safe when being around such a robot (β = –.108, p = .008); they expected that such a robot would be less expensive (β = –.284, p < .001). These results almost fully support Hypothesis 14.
6. GENERAL DISCUSSION
This article presents a conceptual model that both expands and deepens TPB by providing a comprehensive overview of predictors for social robot acceptance and behavioral intention from a wide variety of disciplines relevant to social robot acceptance behavior. The proposed conceptual model of social robot acceptance overcomes the disadvantages posed by Heerink et al. (Citation2010) and Shin and Choo’s (Citation2011) existing models by (a) using a strong theoretical base for the model, (b) hypothesizing the interrelationships between concepts in the model based on theory, (c) testing the model on a general population, (d) including a single drawn sample of participants for the data set, and (e) only incorporating those modifications to the model that can be supported by theory. We therefore believe that the proposed conceptual model of social robot acceptance in this article provides a strong basis for the further development of a model that may expand our understanding of the factors affecting the acceptance of social robots in domestic environments. Using SEM, we tested this model using a sample of the general Dutch population and investigated the influence of several factors on the anticipated acceptance of social robots for domestic use. To build a general model for social robot acceptance, a second-order structure was proposed to create a more parsimonious theory-based account of the correlations among the included acceptance variables (T. A. Brown, Citation2006). These specifications assert that the second-order factors directly affect the first-order factors. These direct effects and correlations among the second-order factors are responsible for the covariation among the first-order factors. However, our data did not support the proposed second-order factor model. This finding indicates that several concepts relating to the second-order structure should be reassessed in future research. The findings in this article may guide this reassessment by providing insight into the important acceptance variables that influence anticipated social robot acceptance.
One way to build on our current findings is to include only those factors that had the greatest direct and indirect impact on social robot acceptance. The results of the direct regression paths indicate that the acceptance of a social robot for domestic use increases when future users believe that they possess the necessary skills to use a social robot, when they perceive that having such a robot enhances their status, and when they anticipate that such a robot will provide more enjoyable interactions, behave less sociably, and cause fewer privacy concerns. A study of all direct and indirect effects of our social robot acceptance model suggests that the acceptance variables of enjoyment, privacy, status, and self-efficacy play a key role. Enjoyment has, by far, the largest direct effect on use intention. Although privacy does not have a large direct negative effect on use intention, it influences use intention indirectly via enjoyment. Similarly, self-efficacy both directly and indirectly affects use intention via enjoyment and sociability. Finally, status has a direct effect on use intention. However, status has an indirect effect on use intention, not only via privacy and self-efficacy but also via privacy through enjoyment. This, in turn, has an indirect effect on use intention of social robots. Future research on a model of social robot acceptance could focus further on the interrelationships between these factors and their influence on the complex process of social robot acceptance. However, given that this is essentially one of the first attempts to build a theoretical model of social robot acceptance, further research on what acceptance variables should be included in such a model is necessary.
An extensive literature review on acceptance variables from a wide variety of research fields resulted in the inclusion of many variables in our model (de Graaf & Ben Allouch, Citation2013a), which were then tested in this study on people who anticipated accepting a social robot in their own homes. Because the data did not fit the second-order factor structure, we decided to continue with the analyses of direct regression paths between the first-order factors, similar to the hypothesis between the second-order factors in the original conceptual model. The conceptual model of social robot acceptance with the direct regression paths was confirmed by our data. Two hypotheses were fully supported, nine hypotheses were partially supported, and three hypotheses were rejected in terms of the data. provides an overview of the hypotheses in the conceptual model and whether they are partially supported, according to the findings.
6.1. Implications
Influential Factors for Social Robot Acceptance
Utilitarian attitudes are tied to usability, emphasize the extrinsic motivations to accept or use a technology, and include the factors of ease of use and adaptability. The utilitarian attitudes of potential social robot users seem to be influenced by both hedonic attitudes and control beliefs but are not directly affected by personal or social norms. The direct effect of hedonic attitudes on utilitarian attitudes supports earlier findings in both the information systems literature (e.g., Agarwal & Karahanna, Citation2000; Y. Lee et al., Citation2003) and the HRI literature (e.g., Heerink et al., Citation2010; K. M. Lee et al., Citation2006; Shin & Choo, Citation2011). The direct effect of control beliefs has been previously reported in studies on information systems (e.g., Hackbarth et al., Citation2003; Karahanna & Limayem, Citation2000) and HRI (e.g., Bartneck et al., Citation2007). Hedonic attitudes are related to users’ experiences during the interaction; emphasize the intrinsic motivations in technology acceptance; and include the factors of enjoyment, attractiveness, animacy, social presence, sociability, and companionship. Potential future social robot users’ hedonic attitudes seem to be influenced by control beliefs, as well as by both personal and social norms. The direct effects of normative beliefs on attitudinal beliefs have been reported in both the information systems literature (e.g., Ben Allouch et al., Citation2009; Y. Lee et al., Citation2003; Yu et al., Citation2005) and the HRI literature (e.g., Heerink et al., Citation2010; Shin & Choo, Citation2011). One specific finding in the current study regarding the utilitarian attitudes is the prominent role of usefulness for social robot acceptance. Similar findings regarding the importance of utility or purpose for social robots have been presented by others (Ezer, Fisk, & Rogers, 2009a,b; Fink et al., Citation2013). The results show that usefulness is strongly related to use intention and that both usefulness and use intention influence the same factor in the EFA. Furthermore, with usefulness measured by items focusing on utility moving from utilitarian attitudes to the concept of use intention, all other regression paths from utilitarian attitudes were insignificant. Thus, the other utilitarian attitudes (i.e., ease of use and adaptability) seem to have lost their relevance in users’ anticipated acceptance of a social robot in their homes. One explanation of the empirical overlap between the two theoretical concepts may be that the psychological consideration of use intention and usefulness is made simultaneously and therefore cannot be empirically distinguished. In other words, for the participants, the decision to use a social robot is the same as evaluating whether a social robot is useful. In this manner, usefulness functions as a requirement for social robots before users even consider using them in the first place. The results of this research are based on prediction of future use. Because people remain unfamiliar with social robotic technologies, some variables, such as status and societal impact, remain unknown for potential future users. This is reflected by their minor role in the model of social robot acceptance at this stage of the diffusion of these technologies in our society. Real experiences with a technology are better predictors of future use of that technology. Therefore, the concept of usefulness needs further attention in HRI research as the technology develops and the diffusion of social robots within society increases.
Another explanation for the empirical overlap between the two theoretical concepts lies in the conceptualization of the measurement of usefulness. To measure usefulness, we adopted the construct of usefulness from Heerink et al. (Citation2010), a measurement based on frequently used items from the UTAUT model (Venkatesh et al., Citation2003). However, it may be argued that this construct only measures the objective and utility of usefulness and does not include the subjective and user-friendliness elements. Therefore, we have only objectively envisioned the possibilities of social robots. The definition of a technology, at its most basic level, is for it to help people do things (Orlikowski, Citation1992), which links the meaning of a technology with the evaluation of its meaning, significance, and utility, which is crucial for the potential user (Silverstone, Citation1996). As a result, the overlap between the measures of usefulness and use intention in our current study, and the strong correlations (Szajna, Citation1996; Yousafzai, Foxall, & Pallister, Citation2007b) between usefulness and use intention, as well as high cross-loadings between the two factors (Agarwal & Karahanna, Citation2000) in the technology acceptance literature, are not surprising. Future technology acceptance research may need to further investigate the underlying concepts that motivate users to evaluate a technology as useful. Some researchers indicate that usefulness is not a one-dimensional concept (Jaschinski & Ben Allouch, Citation2015). A suggestion for future research is to conceptualize usefulness by defining a multidimensional concept and to distinguish several benefits that, together, account for usefulness. For this process, a method similar to that proposed by the model of media attendance (LaRose & Eastin, 2004), which defines several expected outcomes of technology use, could be used.
In our study, personal norms encompass an individual’s belief that engaging in a particular behavior leads to salient personal beliefs, and we have included the factors of privacy, trust, and societal impact in our proposed model. To the best of our knowledge, this is the first time that the distinction between personal and social norms has been made in social robotics research. Given that personal norms arise from beliefs considered to be the norm in one’s social environment, we assumed that social norms would affect one’s personal norms. The results suggest that personal norms indeed appear to be influenced by social norms (e.g., social influence and image), which encompass an individual’s beliefs about the likelihood and importance of the social consequences of performing a particular behavior. Our conceptual model of social robot acceptance did not hypothesize any predictors for social norms. Social norms function as the core of the conceptual model because they not only influence use intention theoretically and all other factors in the model directly but also indirectly influence use intention theoretically through all these other factors. The results show that the direct effects of social norms on personal norms and control beliefs were fully supported, and only the direct effect of social norms on utilitarian attitudes was not supported by the data. However, social norms affect utilitarian norms indirectly, via both hedonic attitudes and control beliefs. The direct effects of social norms on all other factors in the model were partially supported. This means that social norms still have a core function in our empirical model of social robot acceptance. Indeed, as we explain in the next section on the unwanted sociability of social robots, normative beliefs play a significant role in the acceptance of social interactions with robots, especially when people anticipate their acceptance without any real-world experiences or interactions with robots.
Control beliefs involve the user’s beliefs regarding the presence or absence of resources, opportunities, and obstacles that may facilitate or impede performance of the behavior, and they include self-efficacy, personal innovativeness, anxiety toward robots, safety, and cost. The control beliefs of potential future users of social robots seem to be influenced by social norms. Both TPB (Ajzen, Citation1991) and social cognitive theory (Bandura, Citation1977) indicate that control beliefs are affected by opinions from one’s social network.
However, when people actually start using a robot in their own homes, other acceptance variables emerge that may also influence the long-term acceptance process (de Graaf et al., Citation2016). It is possible that some variables have a strong effect when people anticipate accepting a social robot, but the same variables may have less impact when the same people use that same robot for a longer time. When examining the long-term use of social robots in home environments, it appears that the importance of the acceptance variables in explaining social robot acceptance changes over time, shifting from control beliefs to attitudinal beliefs (de Graaf et al., Citation2016). The importance of the acceptance variables is believed to depend on the development stage in which the technology is located (Peters, Citation2011). As people gain experience using a social robot, different acceptance variables from those originally explaining their initial adoption, explain their intention to continue using it. Larger scale longitudinal social robot research is necessary to identify the variables that possess the most explanatory power during different phases of acceptance and how their effects on acceptance change over time.
The Unwanted Sociability of Robots
A remarkable finding of our study was that, overall, the robots’ social behaviors are seemingly not appreciated by the general Dutch population. The participants negatively evaluated the sociability and the companionship possibilities of future domestic robots. Thus, the data suggest that people do not want robots to behave socially, at least at this stage of social robot diffusion within society. Similar findings have been found before in the HRI literature indicating that the people disapprove of robots performing social tasks (Arras & Cerqui, Citation2005; de Graaf & Ben Allouch, Citation2016; European Commission, Citation2012) and that robots should not substitute humans but rather serve as collaborators or servants for people (Ray, Mondada, & Siegwart, Citation2008; Takayama, Ju, & Nass, Citation2008). One explanation for these results is that robots could be labelled as a “disruptive technology,” because they are more than just updated replacements of existing technologies (Ezer et al., 2009a), and people are not easily prompted to embrace disruptive technologies (Dewar & Dutton, Citation1986; Green, Gavin, & Aiman-Smith, Citation1995). In the case of social robots, it could be that people do not want to use robots that behave socially, and the development of such robots should not be pursued. The current findings reveal that potential future users appear to prefer robots that are less sociable. In addition, participants indicated that they believed that a social robot could better adapt to their needs when it provided less companionship. This is similar to conclusions drawn from another study focusing on anticipated acceptance of robots, which show that people largely expect utilitarian functionalities from robots and are less likely to perceive robots as socially interactive devices (Eze et al., 2009a,b). From this perspective, these results suggest that people do not want robots to behave socially or to provide companionship and that the development of these types of robots appears undesirable.
However, there are alternative explanations for our current findings. The participants in our study provided inconsistent assessments of social robots by indicating that a more sociable robot could better adapt to their needs. Thus, a second explanation for the more negative evaluation of the robot’s social behavior could be that people fear, or are not yet familiar with, social interactions and companionship with social robots. The average scores of the acceptance factors in our study show that the participants had serious concerns about their privacy when using a social robot in their own homes. In addition, the results show that when the participants believed that they were more competent to interact with a social robot and could better trust a social robot, they perceived the robot’s behavior as more sociable. Furthermore, the results indicate that when participants believed that they were more competent in their own skills to properly interact with social robots, they anticipated that they would feel less fear in doing so. When they expected to feel safer in the presence of a social robot, they believed that a social robot could provide more companionship. Privacy concerns may thus play a significant role in instigating fear, as people fear the sociability of future social robots that are capable of providing companionship. This fear is caused by people’s privacy concerns, their lack of competence in properly interacting with social robots, their anticipated fear of talking to robots, or the expected lack of safety when in the presence of a social robot. Above all, the participants indicated that the more expensive a social robot is and the more it increases the user’s social status, the greater the companionship they expect it to provide.
A third explanation for the relatively negative evaluations of sociability and companionship is that admitting to treating social robots as companions is perceived as socially undesirable by the participants. Just as depending on television for companionship has been characterized as an inappropriate motivation for use (A. M. Rubin, Citation1983), it is possible that using a robot for companionship is not acceptable in terms of prevailing social norms. Social desirability is the tendency to answer questions in a manner that will be viewed positively by others (Paulhus, Citation1991), which causes overreporting of “good” behavior and underreporting of “bad” or “undesirable” behavior. Social sciences report that a social desirability bias may occur in self-reported data, including questionnaire-collected data (Huang, Liao, & Chang, Citation1998), especially regarding sensitive topics (King & Bruner, Citation2000). In an online study measuring both people’s implicit and explicit associations with domestic robots (de Graaf, Ben Allouch, & Lutfi, Citation2016), it was found that the two measures had conflicting outcomes, which may be attributable to social desirability. Although people explicitly reported that they have positive associations with robots, the implicit measures revealed that they actually had negative associations with robots. Furthermore, people’s implicit associations negatively correlated with their attitudes toward robots and positively correlated with their anxiety toward robots. Yet participants’ explicit associations did not significantly correlate with their attitudes toward robots, and instead negatively correlated with anxiety toward robots. Based on these combined results, de Graaf et al. (Citation2016) concluded that people implicitly have opinions about robots that are different from what they wish to explicitly reveal.
These explanations are, however, based on findings from online-based research, without any real-world interactions between humans and robots. To further explore why the participants in our current study indicated that they did not want robots to behave socially or provide companionship, we must turn to other methods, such as observations and interviews, to determine how people interact with social robots. In contrast to the current results presented in this article, the results from our earlier long-term user studies, employing a social robot in domestic environments (de Graaf, Ben Allouch, & Klamer, Citation2015; de Graaf et al., Citation2016), indicate that people actually behave socially with robots in their own homes, despite their scepticism concerning perceiving robots as social actors and companions. The participants engaged in social interaction with the robot, talked to it, gave it a name, and interpreted the robot’s behavior in a social way. Furthermore, some participants indicated that they would appreciate a time when future robots can interact more socially with their users. Some participants attempted to increase social interactions with the robot used in our previous long-term home studies. However, not all participants appreciated the robot’s social behavior. Some participants experienced feelings of unease when the robot initiated unsolicited conversations, and those participants reduced the social features of the robot to a minimum. Combining findings from both the current study and our long-term home studies indicates that the social behavior of robots still has a long way to go in terms of their proper development, as well as their full societal acceptance by potential future users.
Practical Implications for the Development of Social Robots
Based on the findings of our study, practical implications can be drawn to guide the future development of social robots and their acceptance within society. The most important variables for social robot acceptance is its utility, its usefulness (Davis, Citation1989) or its relative advantage (Rogers, Citation2003). The purpose of the robot must be clear for successful acceptance, leading to continued use. The importance of usefulness has also been stressed in earlier long-term home studies, focusing on the acceptance of domestic robots (de Graaf et al., Citation2016; Fink et al., Citation2013). The majority of participants in these studies failed to perceive the robot as useful and discontinued its use or replaced it with another device. These other technological devices not only fulfilled similar purposes but also were reported to do so in a more satisfying manner. Together, these results indicate that social robot developers should aim for clear applicability in use of their robots or create an easier or more enjoyable way in which robots perform certain functions.
A second practical implication is linked to the sociability of robots. The sociability of robots is still relatively underdeveloped, both in terms of the technological development of the essential social abilities of robots (de Graaf et al., Citation2015) and the societal acceptance of social interactions with interactive technologies. Nevertheless, we believe that robot designers should aim for increased social abilities for robots. The results of our study indicate that a more sociable robot would enhance users’ perceptions of the robot’s adaptability to their needs, a finding supported by our earlier long-term home study (de Graaf et al., Citation2016). Given the simple dialog of the robot used in our longitudinal study, it is not surprising that participants found the interactions with the robot to be somewhat simple and repetitive. The need to first press a button before they could speak to the robot felt particularly unnatural. Most participants would have preferred simply calling the robot by name to get its attention, followed by a command or short conversation. Some participants even preferred engaging in additional conversations beyond the robot’s practical usability. In addition, a socially behaving robot should be able to express and interpret emotions. Another desired adjustment, according to our participants, was an awareness of their presence so that the robot knew when someone was in the room and could attract attention when necessary. Together, these results indicate that actual users would prefer more sociable behaviors and natural conversations for social robots and that the societal acceptance of social interactions with robots might be a matter of familiarization or time.
A final practical recommendation for an increased societal acceptance of social robots is to acknowledge that acceptance is a long-term process and that in each phase users focus on certain acceptance variables that influence social robot acceptance. For the initial adoption of social robots, users seem to focus on (a) control beliefs, such as previous experiences with similar technologies and self-efficacy, as found in this study as well as our previous work (de Graaf et al., Citation2016), and (b) normative beliefs, such as status and privacy concerns in our current study. After the initial adoption, the focus of the decision to continue the use of social robots shifts to the evaluation of the utilitarian and hedonic attitudes associated with the use of the robot. By far the most important utilitarian attitude was the robot’s usefulness as argued earlier in this article. For the hedonic attitudes, the enjoyable interactions a robot offers and the social presence experienced by the users were important variables during initial acceptance. However, the main hedonic reasons for continued use were the robot’s sociability. Thus, for a successful diffusion and acceptance of social robots within society, developers should provide potential consumers with the necessary information to make them feel more familiar with the robot’s technologies and enhance their self-assessment of their ability to use social robots. After people purchase the robot, developers should ensure that the users continue to perceive the robots as useful, enjoyable and sociable.
6.2. Limitations
Despite the observed acceptable values for internal consistency and construct validity, the data showed a few limitations to the proposed social robot acceptance model. The first is related to the inclusion of numerous variables in the structural model. This is a consequence of the main goal of our study, which is to determine the most important determinants for social robot acceptance in domestic environments. The research field for social robot acceptance is relatively new, and it remains unclear which factors have the greatest impact on social robot acceptance. Furthermore, a suitable theory or model for social robot acceptance has not yet been developed. Therefore, we began building the measurement model using EFA. However, the inclusion of numerous variables and their interrelationships impeded proper and straightforward model building, which led us to begin the development of a CFA and conventional SEM. The main reason for this decision was our aim to build a theory-based model of social robot acceptance. Although continuing data analysis with a CFA is not an uncommon approach (Morin et al., Citation2013), and the fit of the first-order factor structure in the model remained acceptable, the second-order factor structure did not. As a result, it became impossible to investigate the interrelationships between the higher order factors of our proposed conceptual model. In addition, because the second-order factor structure did not fit our data, two options remained in order to continue. One option was to conclude that there was no empirical evidence for the hypothesized conceptual model and stop further analysis. The second option was to continue data analysis with another method that would allow some insights into the influential factors of social robot acceptance. Although one should be aware that the data were used twice, we continued testing the same interrelationships in a full model between the second-order factors directly and between the underlying first-order factors. It must be acknowledged that the current version of the model of social robot acceptance remains flexible and open for refinement. Therefore, additional replication studies are necessary to further develop a more valid and reliable model of social robot acceptance, preferably implementing the second-order factor model.
A second limitation of our methodology was that we relied on constructs with three items only. Although three items are enough for building a reliable scale (DeVellis, Citation2003), beginning with initially three items only for each construct is sometimes inadequate. Despite the large sample (n = 1,148) used in our study, it was necessary to remove a few items from further data analysis, which left only two items for the constructs of adaptability and anxiety toward robots. Therefore, our advice for researchers performing quantitative data analysis is to begin with at least five items per construct to allow for the possible and legitimate exclusion of items with poor loadings or cross-loadings.
A third limitation is that some cross-loadings were observed between the constructs of adaptability and enjoyment, sociability and adaptability, sociability and companionship, and privacy and cost. Most of these cross-loadings can be explained by findings from previous studies. For example, Shin and Choo (Citation2011) confirmed the effect of adaptability on enjoyment for social robots. Furthermore, the concepts of adaptability and sociability are closely related, given that sociability entails the capability to successfully adapt to social situations (R. B. Rubin & Martin, Citation1994). The cross-loadings between sociability and companionship can be explained by the finding that an increased evaluation of a robot’s sociability results in higher social presence (Heerink et al., Citation2010), which in turn causes the perception of robots as social companions (K. M. Lee et al., Citation2006; Melson, Kahn, Jr., Beck, & Friedman, Citation2009). However, despite the explanation of cross-loadings by earlier findings, future research is required to better empirically and theoretically distinguish these concepts.
Fourth, as is true for any given SEM, there are alternative models that are equivalent in terms of overall model fit with the same data set and that may produce substantially different explanations of the same data (Chin, Citation1998). However, the model currently presented has a sound, validated theoretical basis. Although it is acknowledged that other possible, and perhaps better, empirical solutions are achievable, it may be difficult to support the parallel findings from these alternative models with the existing theoretical findings.
A fifth limitation is that this study tested social robot acceptance by using a text-based scenario for domestic social robots. The robot representations of our participants (de Graaf & Ben Allouch, Citation2016) were similar to those presented by other researchers (European Commission, Citation2012; Ray et al., Citation2008; Weiss et al., Citation2011), which means that members from developed societies generally have similar ideas about what robots represent. Therefore, we believe that using text-based scenarios was an appropriate way to administer our study without prompting the participants with specific images of robots. Nevertheless, a low knowledge base among the general population regarding robot applications might have affected the results of our study. For example, the lack of results on the impact of utilitarian attitudes on social robot acceptance could have been a result of our text-only approach. Because real interactions with robots are still scarce, some scientists (Arras & Cerqui, Citation2005; Enz, Diruf, Spielhagen, Zoll, & Vargas, Citation2011; Ray et al., Citation2008) suggest that current conceptualizations of robots may largely be shaped by mass mediated messages, including films. These media representations do not necessarily reflect the research field of social robotics, which raises the question of whether participants had any concept about the potential utility of these technologies. In addition, due to the reliance on a text-based scenario, the current results do not allow for an empirical evaluation of the actual use of robots and thus bypassed potential long-term effects. Once social robots are adopted by a larger number of people within society, future research should conduct a longitudinal study among actual users of social robots in home environments and repeatedly test the proposed model of social robot acceptance on actual users of social robots.
The final limitation is that we administered our questionnaire to a Dutch sample. Although we have developed our model using a large sample size, specific cultural assumptions, relating to technology in general or social robots specifically, might be relevant for its acceptance by individuals or society as a whole. Because nationality (European Commission, Citation2012) and cultural differences (H. R. Lee & Sabanovic, Citation2014) have been found to affect the evaluation of robot systems, a replication of our study among other nationalities and cultures is recommended to explore alternative opinions of the general public on future robot applications.
6.3. Conclusion
To develop acceptable social robots, it is necessary to consider future users and their input at an early stage of development. This article presents a conceptual model of social robot acceptance for domestic purposes and tests this model using SEM. To our knowledge, we are the first to present a model of social robot acceptance with a strong theoretical base that has been tested among a general population. The findings of our study indicate that usefulness is a requisite for social robot acceptance and that certain additional important acceptance variables may further explain why people anticipate the acceptance of a social robot in their own homes. These additional acceptance variables show that the anticipated acceptance of a social robot for domestic use increases when users believe that they possess the necessary skills to use a social robot; when they perceive that having such a robot enhances their social status; and when they anticipate that such a robot will provide more enjoyable interactions, behave less sociably, and cause fewer privacy concerns. However, when examining the long-term use of social robots in home environments (de Graaf et al., Citation2016), it appears that the importance of the acceptance variables in explaining social robot acceptance changes over time, shifting from control beliefs before adoption to attitudinal beliefs after initial adoption. It is believed that the importance of the acceptance variables depends on the development stage in which the technology is located (Peters, Citation2011). As users gain experience with a social robot, other acceptance variables explain their intention to continue using it, compared with the acceptance variables that explained their initial adoption. Moreover, given the complex effects of the robot’s sociability in the anticipated acceptance of these types of interactive technologies, the current implications of our results emphasize that robots may indeed represent a new technological genre (de Graaf et al., Citation2015; Young et al., Citation2011). Together, the current findings and implications of our study serve to advance the field of social robotics.
HCI Editorial Record
First received 9 July 2016. Revisions received 24 March 2017, and Accepted by xxx. Final manuscript received 25 March 2017. — Editor
Additional information
Notes on contributors
Maartje M. A. de Graaf
Maartje M.A. de Graaf ([email protected], https://robonarratives.wordpress.com) is a behavioral scientist with an interest in people’s social, emotional, and cognitive responses to robots along with the societal and ethical consequences of such responses. Currently she is a postdoctoral research associate at the Department of Cognitive Linguistic and Psychological Sciences of Brown University.
Somaya Ben Allouch
Somaya Ben Allouch ([email protected], https://www.saxion.nl/gezondheidwelzijnentechnologie/site/onderzoek/technologie/lector/lector/) is an Associate Professor with an interest in adoption and acceptance of new technologies in everyday life. She is the chair of the Technology, Health & Care research group at the Saxion University of Applied Science.
Jan A. G. M. van Dijk
Jan A. G. M. van Dijk ([email protected], https://www.utwente.nl/bms/mco/en/emp/dijk/) is a social scientist with an interest in the social aspects of new media, the network society, and the digital divide. He is Professor of Communication Science and the Sociology of the Information Society, and director of the Center for eGovernment Studies at the University of Twente.
REFERENCES
- Aarts, H., Verplanken, B., & van Knippenberg, A. (1998). Predicting behavior from actions in the past: Repeated decision making or a matter of habit? Journal of Applied Social Psychology, 28(15), 1355–1374.
- Agarwal, R., & Karahanna, E. (2000). Time flies when you’re having fun: Cognitive absorption and beliefs about IT usage. MIS Quarterly, 24(4), 665–694. doi:10.2307/3250951
- Ahlgren, D., & Verner, I. (2009, August). Fostering development of students’ collective and self-efficacy in robotics projects. In Kim, J.H., Sam Ge, S., Vadakkepat, P., Jesse, N., Al Mamun, A., Puthusserypady, S., Rückert, U., Sitte, J., Witkowski, U., Nakatsu, R., Braunl, T., Baltes, J., Anderson, J., Wong, C.C., & Ahlgren, D. (Eds), Proceedings of the FIRA RoboWorld Congress 2009 (Vol. 44, pp. 240–247). Berlin, Heidelberg: Springer.
- Ajzen, I. (1991). The theory of planned behavior. Organizational Behavior and Human Decision Processes, 50(2), 179–221. doi:10.1016/0749-5978(91)90020-T
- Ajzen, I., & Fishbein, M. (1980). Understanding attitudes and predicting social behaviour. London, UK: Pearson.
- Ajzen, I., & Fishbein, M. (2005). The influence of attitudes on behavior. In D. Albarracín, B. T. Johnson, & M. P. Zanna (Eds.), The handbook of attitudes (pp. 173–221). Mahwah, NJ: Erlbaum.
- Anderson, J. C., & Gerbing, D. W. (1988). Structural equation modeling in practice: A review and recommended two-step approach. Psychological Bulletin, 103(3), 411–423. doi:10.1037/0033-2909.103.3.411
- Armitage, C. J., Conner, M. (2001). Efficacy of the theory of planned behavior: A meta-analytic review. British Journal of Social Psychology, 40, 471–499.
- Arras, K. O., & Cerqui, D. (2005). Do we want to share our lives and bodies with robots. Lausanne, Switzerland: Swiss Federal Institute of Technology Lausanne, EPFL.
- Asparouhov, T., & Muthén, B. (2009). Exploratory structural equation modeling. Structural Equation Modeling: A Multidisciplinary Journal, 16(3), 397–438. doi:10.1080/10705510903008204
- Bagozzi, R. P., Lee, H. M., & Van Loo, M. F. (2001). Decisions to donate bone marrow: The role of attitudes and subjective norms across cultures. Psychology and Health, 16, 29–56.
- Bandura, A. (1977). Self-efficacy: Toward a unified theory of behavioral change. Psychological Review, 84(2), 191–215. doi:10.1037/0033-295X.84.2.191
- Bargh, J. A., & Chartrand, T. L. (1999). The unbearable automaticity of being. American Psychologist, 54(7), 462–479.
- Bargh, J.A., & Gollwitzer, P.M. (1994). Environmental control of goal directed action: Automatic and strategic contingencies between situation and behavior. Paper presented at the Nebraska Symposium on Motivation, Lincoln, NE, USA.
- Bartneck, C., Kanda, T., Mubin, O. and Mahmud, A.A. (2009). Does the design of a robot influence its animacy and perceived intelligence. International Journal of Social Robotics, 1(1), 195–204.
- Bartneck, C., Kulić, D., Croft, E., & Zoghbi, S. (2009). Measurement instruments for the anthropomorphism, animacy, likeability, perceived intelligence, and perceived safety of robots. International Journal of Social Robotics, 1(1), 71–81. doi:10.1007/s12369-008-0001-3
- Bartneck, C., Kanda, T., Mubin, O. and Mahmud, A.A. (2009). Does the design of a robot influence its animacy and perceived intelligence. International Journal of Social Robotics, 1(1), 195–204.
- Bartneck, C., Nomura, T., Suzuki, T., Kanda, T., & Kennsuke, K. (2005). A cross-cultural study on attitudes towards robots. Paper presented at the International Conference on Human-Computer Interaction, Las Vegas.
- Bartneck, C., Suzuki, T., Kanda, T., & Nomura, T. (2007). The influence of people’s culture and prior experiences with AIBO on their attitude towards robots. AI & Society, 21(1–2), 217–230. doi:10.1007/s00146-006-0052-7
- Ben Allouch, S., van Dijk, J. A. G. M., & Peters, O. (2009). The acceptance of domestic ambient intelligence appliances by prospective users. Proceedings of the Pervasive 2009 International Conference on Pervasive Computing. New York, NY: Springer.
- Benbasat, I., & Barki, H. (2007). Quo vadis, TAM? Journal of the Association for Information Systems, 8, 211–218.
- Bentler, P. M., & Speckart, G. (1981). Attitudes “cause” behaviors: A structural equation analysis. Journal of Personality and Social Psychology, 40(2), 226–238.
- Biocca, F., Harms, C., & Burgoon, J. K. (2003). Toward a more robust theory and measure of social presence: Review and suggested criteria. Presence: Teleoperators and virtual environments, 12(5), 456–480.
- Breazeal, C. L. (2003). Towards sociable robots. Robotics & Automation Systems, 42(3–4), 167–175. doi:10.1016/S0921-8890(02)00373-1
- Breckler, S.J., & Wiggins, E.C. (1989). On defining attitude and attitude theory: Once more with feeling. In A. R. Pratkanis, S. J. Breckler, & A. G. Greenwald. (Eds.), Attitude structure and function (pp. 407–427). Hillsdale, NJ: Erlbaum.
- Broadbent, E., Stafford, R., & MacDonald, B. (2009). Acceptance of healthcare robots for the older population: Review and future directions. International Journal of Social Robotics, 1(4), 319–330. doi:10.1007/s12369-009-0030-6
- Brown, S. A., & Venkatesh, V. (2005). Model of adoption of technology in households: A baseline model test and extension incorporating household life cycle. MIS Quarterly, 29(3), 399–426.
- Brown, T. A. (2006). Confirmatory factor analysis for applied research. New York, NY: Guilford Press.
- Brown, T. A., Chopita, B. F., & Barlow, D. H. (1998). Structural relationships among dimensions of the DSM-IV anxiety and mood disorders and dimensions of negative affect, positive affect, and autonomic arousal. Journal of Abnormal Psychology, 107, 179–192.
- Browne, M. W. (2001). An overview of analytic rotation in exploratory factor analysis. Multivariate Behavioral Research, 36(1), 111–150. doi:10.1207/S15327906MBR3601_05
- Burgoon, J. K., & Buller, D. B. (1996). Reflections on the nature of theory building and the theoretical status of interpersonal deception theory. Communication Theory, 6, 311–328. doi:10.1111/j.1468-2885.1996.tb00132.x
- Cartwright-Hatton, S., & Wells, A. (1997). Beliefs about worry and intrusions: The meta-cognitions questionnaire and its correlates. Journal of anxiety disorders, 11(3), 279–296.
- Central Bureau of Statistics (CBS), StatLine: Bevolking; kerncijfers. Den Haag/Heerlen, The Netherlands: CBS.
- Chen, F., Sousa, K. H., & West, S. G. (2005). Teacher’s corner: Testing measurement invariance of second-order factor models. Structural Equation Modeling: A Multidisciplinary Journal, 12(3), 471–492. doi:10.1207/s15328007sem1203_7
- Chin, W. W. (1998). Commentary: Issues and opinion on structural equation modeling. MIS Quarterly, 22(1), vii–xvi.
- Cramer, H., Evers, V., Ramlal, S., van Someren, M., Rutledge, L., Stash, N., … Wielinga, B. (2008). The effects of transparency on trust in and acceptance of a content-based art recommender. User Model User-Adapt Interaction, 18(5), 455–496. doi:10.1007/s11257-008-9051-3
- Cuijpers, R. H., Bruna, M. T., Ham, J. R. C., & Torta, E. (2011). Attitude towards robots depends on interaction but not on anticipatory behavior. In B. Mutlu, C. Bartneck, J. Ham, V. Evers, T. Kanda (Eds.), Proceedings of the ICSR 2011 International Conference on Social Robotics. Berlin, Germany: Springer-Verlag.
- Dautenhahn, K., & Saunders, J. (2011). New frontiers in human–robot interaction (Vol. 2). Amsterdam, the Netherlands: John Benjamins.
- Dautenhahn, K., Woods, S., Kaouri, C., Walters, M. L., Koay, K. L., & Werry, I. (2005). What is a robot companion: Friend, assistant or butler? Proceedings of the IROS 2005 International Conference on Intelligent Robots and Systems. New York, NY: IEEE.
- Davis, F. D. (1989). Perceived usefulness, perceived ease of use, and user acceptance of information technology. MIS Quarterly, 13(3), 319–340. doi:10.2307/249008
- Deci, E. L., & Ryan, R. M. (1985). Intrinsic motivation and self-determination in human behavior. New York, NY: Plenum.
- de Graaf, M. M. A. (2016). An ethical evaluation of human-robot relationships. International Journal of Social Robotics, 8(4), 589–598. doi:10.1007/s12369-016-0368-5
- de Graaf, M. M. A., & Ben Allouch, S. (2013a). Exploring influencing variables for the acceptance of social robots. Robotics and Autonomous Systems, 61, 1476–1486. doi:10.1016/j.robot.2013.07.007
- de Graaf, M. M. A., & Ben Allouch, S. (2013b). The relation between people’s attitude and anxiety towards robots in human-robot interaction. Proceedings of the RO-MAN 2013 International Symposium on Robot and Human Interactive Communication. New York, NY: IEEE.
- de Graaf, M. M. A., & Ben Allouch, S. (2016). Anticipating our future robot society: The evaluation of future robot applications from a user’s perspective. Proceedings of the RO-MAN 2016 International Symposium on Robot and Human Interactive Communication. New York, NY: IEEE.
- de Graaf, M. M. A., Ben Allouch, S., & Klamer, T. (2015). Sharing a life with Harvey: Exploring the acceptance of and relationship building with a social robot. Computers in Human Behavior, 43(1), 1–14. doi:10.1016/j.chb.2014.10.030
- de Graaf, M. M. A., Ben Allouch, S., & Lutfi, S. (2016). What are people’s associations of robots? Comparing implicit and explicit measures. Proceedings of the RO-MAN 2016 International Symposium on Robot and Human Interactive Communication.
- de Graaf, M. M. A., Ben Allouch, S., & van Dijk, J. A. G. M. (2015). What makes a robot social? A user’s perspective on characteristics for social human–robot interaction. In Agah, A., Cabibihan, J.-J., Howard, A., Salichs, M.A., He, H. (Eds.), Proceedings of the ICSR 2015 International Conference on Social Robotics. Berlin, Germany: Springer-Verlag.
- de Graaf, M. M. A., Ben Allouch, S., & van Dijk, J. A. G. M. (2016). Long-term evaluation of a social robot in real homes. Interaction Studies, 17(3), 1–25.
- de Ruyter, B., Saini, P., Markopoulos, P., & van Breemen, A. (2005). Assessing the effects of building social intelligence in a robotic interface for the home. Interacting with Computers, 17(5), 522–541. doi:10.1016/j.intcom.2005.03.003
- DeSteno, D., Breazeal, C., Frank, R. H., Pizarro, D., Baumann, J., Dickens, L., & Lee, J. J. (2012). Detecting the trustworthiness of novel partners in economic exchange. Psychological Science, 23, 1549–1556, 0956797612448793. doi:10.1177/0956797612448793
- DeVellis, R. F. (2003). Scale development: Theories and applications. Newbury Park, CA: Sage.
- Dewar, R. D., & Dutton, J. E. (1986). The adoption of radical and incremental innovations: An empirical analysis. Management Science, 32, 1422–1433. doi:10.1287/mnsc.32.11.1422
- Enz, S., Diruf, M., Spielhagen, C., Zoll, C., & Vargas, P. A. (2011). The social role of robots in the future: Explorative measurement of hopes and fears. International Journal of Social Robotics, 3(3), 263–271. doi:10.1007/s12369-011-0094-y
- European Commission. (2012). Public attitudes towards robots (Special Eurobarometer 382). Brussel, Belgium: Kantar Public.
- Ezer, N., Fisk, A. D., & Rogers, W. A. (2009a). Attitudinal and intentional acceptance of domestic robots by younger and older adults. Proceedings of the 2009 International Conference on Universal Access in Human–Computer Interaction.
- Ezer, N., Fisk, A. D., & Rogers, W. A. (2009b). More than a servant: Self-reported willingness of younger and older adults to having a robot perform interactive and critical tasks in the home. Proceedings of the HFES 2009 Human Factors and Ergonomics Society.
- Fink, J., Bauwens, V., Kaplan, F., & Dillenbourg, P. (2013). Living with a vacuum cleaning robot: A 6-month ethnographic study. International Journal of Social Robotics, 5(3), 389–408. doi:10.1007/s12369-013-0190-2
- Fishbein, M., & Ajzen, I. (1975). Belief, attitude and behavior: An introduction to theory and research. Reading, UK: Addison-Wesley.
- Fisher, R. J., & Price, L. L. (1992). An investigation into the social context of early adoption behavior. Journal of Consumer Research, 19(3), 477–486. doi:10.1086/jcr.1992.19.issue-3
- Flandorfer, P. (2012). Population ageing and socially assistive robots for elderly persons: The importance of sociodemographic factors for user acceptance. International Journal of Population Research, 2012, 1–13. doi:10.1155/2012/829835
- Fong, T., Nourbakhsh, I., & Dautenhahn, K. (2003). A survey of socially interactive robots. Robotics and Autonomous Systems, 42, 143–166. doi:10.1016/S0921-8890(02)00372-X
- Goetz, J., Kiesler, S., & Powers, A. (2003). Matching robot appearance and behavior to tasks to improve human-robot cooperation. Proceedings of the RO-MAN 2003 International Symposium on Robot and Human Interactive Communication. New York, NY: IEEE.
- Green, S. G., Gavin, M. B., & Aiman-Smith, L. (1995). Assessing a multidimensional measure of radical technological innovation. IEEE Transaction on Engineering Management, 42, 203–214. doi:10.1109/17.403738
- Greenwald, A.G. (1989). Why are attitudes important? In A. R. Pratkanis, S. J. Breckler, & A. G. Greenwald (Ed.), Attitude structure and function. Hillsdale, NJ, USA: Erlbaum.
- Groom, V., Nass, C., Chen, T., Nielsen, A., Scarborough, J. K., & Robles, E. (2009). Evaluating the effects of behavioral realism in embodied agents. International Journal of Human-Computer Studies, 67(10), 842–849. doi:10.1016/j.ijhcs.2009.07.001
- Gustafsson, J., & Balke, G. (1993). General and specific abilities as predictors of school achievement. Multivariate Behavioral Research, 28(4), 407–434. doi:10.1207/s15327906mbr2804_2
- Hackbarth, G., Grover, V., & Yi, M. Y. (2003). Computer playfulness and anxiety: Positive and negative mediators of the system experience effect on perceived ease of use. Information & Management, 40(3), 221–232. doi:10.1016/S0378-7206(02)00006-X
- Hancock, P. A., Billings, D. R., Schaefer, K. E., Chen, J. Y. C., de Visser, E. J., & Parasuraman, R. (2011). A meta-analysis of factors affecting trust in human-robot interaction. The Journal of the Human Factors and Ergonomics, 53(5), 517–527. doi:10.1177/0018720811417254
- Hassenzahl, M. (2004). The interplay of beauty, goodness, and usability in interactive products. Human–Computer Interaction, 19(4), 319–349. doi:10.1207/s15327051hci1904_2
- Heerink, M., Kröse, B., Evers, V., & Wielinga, B. (2010). Assessing acceptance of assistive social agent technology by older adults: The Almere model. International Journal of Social Robotics, 2(4), 361–375. doi:10.1007/s12369-010-0068-5
- van der Heijden, H. (2003). Factors influencing the use of websites: The case of a generic portal in The Netherlands. Information & Management, 40(6), 541–549.
- Hox, J. J., & Bechger, T. M. (1998). An introduction to structural equation modeling. Family Science Review, 11, 354–373.
- Hu, L. T., & Bentler, P. M. (1999). Cutoff criteria for fit indices in covariance structure analysis: Conventional criteria versus new alternatives. Structural Equation Modeling: A Multidisciplinary Journal, 6, 1–55. doi:10.1080/10705519909540118
- Huang, C., Liao, H., & Chang, S. (1998). Social desirability and the Clinical Self-Report Inventory: Methodological reconsideration. Journal of Clinical Psychology, 54, 517–528. doi:10.1002/(ISSN)1097-4679
- International Federation of Robotics. (2014). World robotics report 2014. Retrieved from http://www.worldrobotics.org/uploads/media/Executive_Summary_WR_2014_02.pdf (accessed February 7, 2015).
- Izard, C.E. (1977). Human emotions. New York, NY: Plenum.
- Jaschinski, C., & Ben Allouch, S. (2015). Why should I use this?: Identifying incentives for using AAL technologies. Proceedings of the Ami 2015 European Conference on Ambient Intelligence.
- Joosse, M., Sardar, A., Lohse, M., & Evers, V. (2013). BEHAVE-II: The revised set of measures to assess users’ attitudinal and behavioral responses to a social robot. International Journal of Social Robotics, 5(3), 379–388. doi:10.1007/s12369-013-0191-1
- Jöreskog, K. G. (1969). Efficient estimation in image factor analysis. Psychometrika, 34(1), 51–75. doi:10.1007/BF02290173
- Kahn, P. H., Friedman, B., Perez-Granados, D. R., & Freier, N. G. (2006). Robotic pets in the lives of preschool children. Interaction Studies, 7(3), 405–436. doi:10.1075/is.7.3.13kah
- Kahn, P. H., Gary, H. E., & Shen, S. (2013). Children’s social relationships with current and near-future robots. Child Development Perspectives, 7(1), 32–37. doi:10.1111/cdep.12011
- Kahn, P. H., Ishiguro, H., Friedman, B., & Kanda, T. (2006, September). What is a human?-Toward psychological benchmarks in the field of human-robot interaction. In Proceedings of the 15th IEEE International Symposium on Robot and Human Interactive Communication. Hatfield, UK: IEEE.
- Karahanna, E., & Limayem, M. (2000). E-mail and V-mail usage: Generalizing across technologies. Journal of Organizational Computing and Electronic Commerce, 10(1), 49–66. doi:10.1207/S15327744JOCE100103
- Katz, E., Blumler, J. G., & Gurevitch, M. (1973). Uses and gratifications research. Social Psychology, 37(4), 509–523. doi:10.1086/268109
- Kenny, D. A., & McCoach, D. B. (2003). Effect of number of variables on measures of fit in structural equation modeling. Structural Equation Modeling: A Multidisciplinary Journal, 10(3), 333–351. doi:10.1207/S15328007SEM1003_1
- King, M., & Bruner, G. (2000). Social desirability bias: A neglected aspect of validity testing. Psychology and Marketing, 17(2), 79–103. doi:10.1002/(ISSN)1520-6793
- Kline, R. B. (2011). Principles and practices of structural equation modeling (3rd ed.). New York, NY: Guilford Press.
- Kmenta, J. (1971). Elements of econometrics. New York, NY: Macmillan.
- LaRose, R., & Eastin, M. S. (1994). A social cognitive theory of internet uses and gratifications: Toward a new model of media attendance. Journal of Broadcasting & Electronic Media, 48(3), 358–377. doi:10.1207/s15506878jobem4803_2
- Lee, H. R., & Sabanovic, S. (2014). Culturally variable preferences for robot design and use in South Korea, Turkey and the United States. Proceedings of the HRI 2014 International Conference on Human–Robot Interaction. New York, NY: ACM.
- Lee, K., Park, N., & Song, H. (2005). Can a robot be perceived as a developing creature?: Effects of a robot’s long-term cognitive developments on its social presence and people’s social responses toward it. Human Communication Research, 31, 538–563. doi:10.1111/j.1468-2958.2005.tb00882.x
- Lee, K. M., Jung, Y., Kim, J., & Kim, S. R. (2006). Are physically embodied social agents better than disembodied social agents?: The effects of physical embodiment, tactile interaction, and people’s loneliness in human-robot interaction. International Journal of Human-Computer Studies, 64, 962–973. doi:10.1016/j.ijhcs.2006.05.002
- Lee, Y., Kozar, K. A., & Larsen, K. R. T. (2003). The technology acceptance model: Past, present and future. Communications of the Association for Information Systems, 12(1), 752–780.
- Lee, Y., Lee, J., & Lee, Z. (2006). Social influence on technology behaviour: Self-identity theory perspective. ACM SIGMIS Database, 37(2–3), 60–75. doi:10.1145/1161345.1161355
- Li, D., Rau, P. L. P., & Li, Y. (2010). A cross-cultural study: Effect of robot appearance and task. International Journal of Social Robotics, 2(2), 175–186. doi:10.1007/s12369-010-0056-9
- Liao, C., Chen, J. L., & Yen, D. C. (2007). Theory of planned behavior (TPB) and customer satisfaction in the continued use of e-service: An integrated model. Computers in Human Behavior, 23, 2804–2822. doi:10.1016/j.chb.2006.05.006
- Limayem, M., & Hirt, S. G. (2003). Force of habit and information system usage: Theory and initial validation. Journal of the Association for Information Systems, 4, 65–97.
- Liu, E. Z. F., Lin, C. H., & Chang, C. S. (2010). Student satisfaction and self-efficacy in a cooperative robotics course. Social Behavior and Personality, 38, 1135–1146. doi:10.2224/sbp.2010.38.8.1135
- Malhotra, N. K., Kim, S. S., & Agarwal, J. (2004). Internet users’ information privacy concerns (IUIPC): The construct, the scale, and a causal model. Information Systems Research, 15, 336–355. doi:10.1287/isre.1040.0032
- Manstead, A. S. R., & Parker, D. (1995). Evaluating and extending the theory of planned behavior. European Review of Social Psychology, 6(1), 69–95.
- Mathieson, K. (1991). Predicting user intentions: Comparing the technology acceptance model with the theory of planned behavior. Information Systems Research, 2(3), 173–191.
- Mathieson, K., Peacock, E., & Chin, W. W. (2001). Extending the technology acceptance model: The influence of perceived user resources. The DATA BASE for Advances in Information Systems, 32, 86–112. doi:10.1145/506724.506730
- McCroskey, J. C., & McCain, T. A. (1974). The measurement of interpersonal attraction. Speech Monographs, 41(3), 261–266.
- McCroskey, J. C., Richmond, V. P., & Daly, J. A. (1975). The development of a measure of perceived homophily in interpersonal communication. Human Communication Research, 1, 323–332. doi:10.1111/j.1468-2958.1975.tb00281.x
- McCroskey, J. C., & Teven, J. J. (1999). Goodwill: A reexamination of the construct and its measurement. Communication Monographs, 66(1), 90–103. doi:10.1080/03637759909376464
- Mehrabian, A., & Russell, J. A. (1974). An approach to environmental psychology. Cambridge, MA: MIT Press.
- Melson, G. F., Kahn, P. H., Beck, A., & Friedman, B. (2009). Robotic pets in human lives: Implications for the human-animal bond and for human relationships with personified technologies. Journal of Social Issues, 65, 545–567. doi:10.1111/j.1540-4560.2009.01613.x
- Miniard, P. W. (1981). Examining the diagnostic utility of the Fishbein behavioral intention model. Advances in Consumer Research, 8, 42–47.
- Moon, J. W., & Kim, J. G. (2000). Extending the TAM for a world-wide-web context. Information & Management, 38, 217–230.
- Moore, G. C., & Benbasat, I. (1991). Development of an instrument to measure the perceptions of adopting an information technology innovation. Information Systems Research, 2(3), 192–222. doi:10.1287/isre.2.3.192
- Morin, A. J. S., Marsh, H. W., & Nagengast, B. (2013). Exploratory structural equation modeling. In G. R. Hancock & R. O. Mueller (Eds.), Structural equation modeling: A second course (pp. 395–436). New York, NY: Information Age.
- Muthén, L. K., & Muthén, B. O. (1998–2012). Mplus user’s guide (7th ed.). Los Angelos, CA: Muthén & Muthén.
- Mutlu, B. (2011). Designing embodied cues for dialog with robots. AI Magazine, 32(4), 17–30.
- Nomura, T., Kanda, T., Suzuki, T., & Kato, K. (2006). Exploratory investigation onto influence of negative attitudes towards robots in human-robot interaction. AI & Society, 20, 138–150. doi:10.1007/s00146-005-0012-7
- Nomura, T., Kanda, T., Suzuki, T., Yamada, S., & Kato, K. (2009). Influences of concerns toward emotional interaction into social acceptability of robots. Proceedings of the HRI 2009 International Conference on Human-Robot Interaction. New York, NY: ACM.
- Nomura, T., Suzuki, T., Kanda, T., Han, J., Shin, N., Burke, J., & Kato, K. (2008). What people assume about humanoid and animal-type robots: Cross-cultural analysis between Japan, Korea, and the United States. International Journal of Humanoid Robotics, 5(1), 25–46. doi:10.1142/S0219843608001297
- Nunnally, J. C., & Bernstein, I. H. (1994). Psychometric theory. Sydney, Australia: McGraw-Hill.
- Orlikowski, W. J. (1992). The duality of technology: Rethinking the concept of technology in organizations. Organization Science, 3(3), 398–427. doi:10.1287/orsc.3.3.398
- Ortiz De Guinea, A., & Markus, M. L. (2009). Why break the habit of a lifetime? Rethinking the roles of intention, habit, and emotion in continuing information technology use. MIS Quarterly, 33(3), 433–444.
- Ouellette, J. A., & Wood, W. (1998). Habit and intention in everyday life: The multiple processes by which past behavior predicts future behavior. Psychological Bulletin, 124(1), 54–74. doi:10.1037/0033-2909.124.1.54
- Paulhus, D. L. (1991). Measurement and control of response bias. In J. P. Robinson, P. R. Shaver, & L. S. Wrightsman (Eds.), Measures of personality and socialpsychological attitudes (pp. 17–59). New York, NY: Academic Press.
- Pavlou, P. A., & Fygenson, M. (2006). Understanding and predicting electronic commerce adoption: An extension of the theory of planned behavior. MIS Quarterly, 30(1), 115–143.
- Perugini, M., & Bagozzi, R. P. (2001). The role of desires and anticipated emotions in goal-directed behaviours: Broadening and deepening the theory of planned behaviour. British Journal of Social Psychology, 40(1), 79–98. doi:10.1348/014466601164704
- Peters, O. (2011). Three theoretical perspectives on communication technology adoption. In A. Vishwanath, & G.A. Barnett (Eds.), The diffusions of innovations: A communication science perspective. New York, NY: Peter Lang.
- Peters, O., & Ben Allouch, S. (2005). Always connected: A longitudinal field study of mobile communication. Telematics and Informatics, 22, 239–256. doi:10.1016/j.tele.2004.11.002
- Ray, C., Mondada, F., & Siegwart, R. (2008). What do people expect from robots? Proceedings of the IROS 2008 International Conference on Intelligent Robots and Systems. New York, NY: IEEE.
- Reeves, B., & Nass, C. (1996). The media equation: How people treat computers, television, and new media like real people and places. New York, NY: CSLI Publications.
- Richard, R., Pligt, J., & Vries, N. (1995). Anticipated affective reactions and prevention of AIDS. British Journal of Social Psychology, 34(1), 9–21.
- Rindskopf, D., & Rose, T. (1988). Some theory and applications of confirmatory second-order factor analysis. Multivariate Behavioral Research, 23, 51–67. doi:10.1207/s15327906mbr2301_3
- Rivis, A., & Sheeran, P. (2003). Social influences and the theory of planned behavior: Evidence of a direct relationship between prototypes and young people's exercises behavior. Psychology and Health, 18, 567–586.
- Rogers, E. M. (2003). Diffusion of innovations (5th ed.). New York, NY: The Free Press.
- Rosenthal-von der Pütten, A. M., Krämer, N. C., Hoffmann, L., Sobieraj, S., & Eimler, S. C. (2013). An experimental study on emotional reactions towards a robot. International Journal of Social Robotics, 5(1), 17–34.
- Rubin, A. M. (1983). Television uses and gratifications: The interactions of viewing patterns and motivations. Journal of Broadcasting, 27(1), 37–51. doi:10.1080/08838158309386471
- Rubin, R. B., & Martin, M. M. (1994). Development of a measure of interpersonal communication competence. Communication Research Reports, 11(1), 33–44. doi:10.1080/08824099409359938
- Ryan, R. M., & Deci, E. L. (2000). Intrinsic and extrinsic motivations: Classic definitions and new directions. Contemporary Educational Psychology, 25(1), 54–67. doi:10.1006/ceps.1999.1020
- Šabanović, S. (2010). Robots in society, society in robots. International Journal of Social Robotics, 2, 439–450.
- Salem, M., Eyssel, F., Rohlfing, K., Kopp, S., & Joublin, F. (2013). To err is human (-like): Effects of robot gesture on perceived anthropomorphism and likability. International Journal of Social Robotics, 5(3), 313–323. doi:10.1007/s12369-013-0196-9
- Sass, D. A., & Schmitt, T. A. (2010). A comparative investigation of rotation criteria within exploratory factor analysis. Multivariate Behavioral Research, 45(1), 73–103. doi:10.1080/00273170903504810
- Scopelliti, M., Giuliani, M.V., & Fornara, F. (2005). Robots in a domestic setting: A psychological approach. Universal Access in the Information Society, 4(2), 146–155.
- Serenko, A. (2008). A model of user adoption of interface agents for email notification. Interacting with Computers, 20(4–5), 461–472.
- Sheeran, P., & Orbell, S. (1999). Augmenting the theory of planned behavior: Roles for anticipated regret and descriptive norms. Journal of Applied Social Psychology, 29, 2107–2142.
- Sheppard, B. H., Hartwick, J., & Warshaw, P. R. (1988). The theory of reasoned action: A meta-analysis of past research with recommendation and future research. Journal of Consumer Research, 15, 325–343.
- Shin, D. H., & Choo, H. (2011). Modeling the acceptance of socially interactive robotics: Social presence in human-robot interaction. Interaction Studies, 12(3), 430–460. doi:10.1075/is.12.3.04shi
- Silverstone, R., & Haddon, L. (1996). Design and the domestication of ICTs: Technical change and everyday life. In R. Silverstone, & R. Mansell (Eds.), Communication by design. The politics of information and communication technologies (pp. 44–74). Oxford: Oxford Press.
- Stafford, R. Q., Broadbent, E., Jayawardena, C., Unger, U., Kuo, I. H., Igic, A., Wong, R., Kerse, N., Watson, C., & MacDonald, B. A. (2010). Improved robot attitudes and emotions at a retirement home after meeting a robot. Paper presented at the International Symposium on Robot and Human Interactive Communication (RO-MAN 2010), Viareggio, Italy.
- Straub, D. W., & Burton-Jones, A. (2007). Veni, vidi, vici: Breaking the TAM logjam. Journal of the Association for Information Systems, 8(4), 223.
- Sun, H. S., & Zhang, P. (2006). The role of moderating factors in user technology acceptance. International Journal of Human-Computer Studies, 64(2), 53–78.
- Szajna, B. (1996). Emperical evaluation of the revised technology acceptance model. Management Science, 42(1), 85–92. doi:10.1287/mnsc.42.1.85
- Takayama, L., Ju, W., & Nass, C. (2008). Beyond dirty, dangerous, and dull: What everyday people think robots should do. Proceedings of the HRI 2008 International Conference on Human–Robot Interaction. New York, NY: ACM.
- Taylor, R. (1990). Interpretation of the correlation coefficient: A basic review. Journal of Diagnostic Medical Sonography, 6(1), 35–39. doi:10.1177/875647939000600106
- Taylor, S., & Todd, P. A. (1995). Understanding information technology usage: A test of competing models. Information Systems Research, 6(2), 144–176. doi:10.1287/isre.6.2.144
- Triandis, H. C. (1979). Values, attitudes, and interpersonal behavior. Paper presented at the Nebraska Symposium on Motivation, Lincoln, Nebraska.
- Turkle, S. (2011). Alone together: Why we expect more from technology and less from each other. New York, NY: Basic Books.
- Vallerand, R. J. (1997). Toward a hierarchical model of intrinsic and extrinsic motivation. Advances in experimental social psychology, 29, 271–360.
- Vallerand, R. J. (1997). Toward a hierarchical model of intrinsic and extrinsic motivation. Advances in Experimental Social Psychology, 29, 271–360. doi:10.1016/S0065-2601(08)60019-2
- van der Heijden, H. (2004). User acceptance of hedonic information systems. MIS Quarterly, 28(4), 695–704.
- Venkatesh, V., & Bala, H. (2008). Technology acceptance model 3 and a research agenda on interventions. Decision Sciences, 39(2), 273–315. doi:10.1111/j.1540-5915.2008.00192.x
- Venkatesh, V., & Brown, S. A. (2001). A longitudinal investigation of personal computers in homes: Adoption determinants and emerging challenges. MIS Quarterly, 25(1), 71–102. doi:10.2307/3250959
- Venkatesh, V., & Davis, F. D. (2000). A theoretical extension of the technology acceptance model: Four longitudinal field studies. Management Science, 46(2), 186–204. doi:10.1287/mnsc.46.2.186.11926
- Venkatesh, V., Morris, M. G., Davis, G. B., & Davis, F. D. (2003). User acceptance of information technology: Toward a unified view. MIS Quarterly, 27(3), 425–478.
- Venkatesh, V., Thong, J. Y. L., & Xu, X. (2012). Consumer acceptance and use of information technology: Extending the unified theory of acceptance and use of technology. MIS Quarterly, 36(1), 157–178.
- Wand, X. (2011). The role of anticipated negative emotions and past behavior in individuals’ physical activity intentions and behaviors. Psychology of Sport and Exercise, 12(3), 300–305. doi:10.1016/j.psychsport.2010.09.007
- Weiss, A., Igelsböck, J., Wurhofer, D., & Tscheligi, M. (2011). Looking forward to a ‘robot society’?: Notions of future human–robot relationships. International Journal of Social Robotics, 3(2), 111–123. doi:10.1007/s12369-010-0076-5
- Yoo, S. J., Han, S. H., & Huang, W. (2012). The roles of intrinsic motivators and extrinsic motivators in promoting e-learning in the workplace: A case from South Korea. Computers in Human Behavior, 28, 942–950. doi:10.1016/j.chb.2011.12.015
- Young, J. E., Hawkins, R., Sharlin, E., & Igarashi, T. (2007). Towards acceptable domestic robots: Applying insights from social psychology. International Journal of Social Robotics, 1(1), 95–108. doi:10.1007/s12369-008-0006-y
- Young, J. E., Sung, J. Y., Voida, A., Sharlin, E., Igarashi, T., Christensen, H. I., & Grinter, R. E. (2011). Evaluating human–robot interaction. International Journal of Social Robotics, 3(1), 53–67. doi:10.1007/s12369-010-0081-8
- Yousafzai, S. Y., Foxall, G. R., & Pallister, J. G. (2007a). Technology acceptance: A meta-analysis of the TAM: Part 1. Journal of Modeling in Management, 2(3), 251–280. doi:10.1108/17465660710834453
- Yousafzai, S. Y., Foxall, G. R., & Pallister, J. G. (2007b). Technology acceptance: A meta-analysis of the TAM: Part 2. Journal of Modeling in Management, 2(3), 281–304. doi:10.1108/17465660710834462
- Yu, J., Ha, I., Choi, M., & Rho, J. (2005). Extending the TAM for a t-commerce. Information & Management, 42, 965–976. doi:10.1016/j.im.2004.11.001./