1. Introduction
Video games have long been heralded as powerful learning tools. Yet half a century since the term “serious games” was coined (Abt, Citation1970), the evidence on whether and when games can afford learning remains mixed. We know that gameplay can produce learning (Qian & Clark, Citation2016; Tlili et al., Citation2020), but the evidence is mixed and contradictory, with some reviews (e.g., Acquah & Katz, Citation2020; Hussein et al., Citation2019) finding positive effects, while others (e.g., Milne-Ives et al., Citation2020) finding either no significant effects or insufficient evidence to draw conclusions.
This mixed data needs explaining. Setting questions of methodological rigor and study quality aside, one primary way of explaining contradictory findings is that current theories and studies fail to account for hidden moderating or mediating variables (Baron & Kenny, Citation1986). In this article, we propose that task-based attention is such a systemically relevant yet understudied moderator of learning in video game play. This claim is grounded in two empirical bases: (1) the rich psychological literature on attention, which robustly shows that people neither respond to nor recall unattended stimuli (e.g., Kuhn et al., Citation2016; Petersen & Posner, Citation2012; Rensink, Citation2000; Simons & Chabris, Citation1999); (2) an emerging series of empirical studies demonstrating that these effects hold in video game play (Cutting & Cairns, Citation2020; Cutting et al., Citation2020).
We emphasize that we theorize task-based attention as a moderator of game-based learning, not as the sole or primary mechanism that explains how gameplay supports learning. There are several complementary learning-supportive qualities to well-designed games mirrored in lines of research and theory. Following Whitton (Citation2014)’s useful framework, digital gameplay can offer active learning environments where players learn by working through meaningful and authentic challenges with rich social scaffolding and reflection, as theorized in experiential and problem-based learning and particularly socio-cultural learning theories. Games can be motivational tools that make learning inherently motivating and enjoyable by stoking curiosity, satisfying basic needs like competence or autonomy, keeping player attention absorbed with just-right challenges, and rewarding in-game activity, as theorized by Malone and Lepper (Citation1987), self-determination theory (Ryan & Rigby, Citation2019), or flow theory (Csikszentmihalyi, Citation1990). As suggested in constructionist learning theory (Kafai & Burke, Citation2015), games can offer safe playgrounds where players learn through exploratory and constructive play, be it by constructing things within games (as in Minecraft (Mojang, Citation2009)) or be it by constructing and modifying games themselves. Together, these aspects go a long way to explain why games can be potent learning environments as such. With task-based attention, we are modeling a moderator of learning that operates within gameplay, even if the game in question is otherwise a well-designed active learning environment, motivational tool, or playground. We also readily acknowledge that the features and demands that we identify as driving task-based attention are often multifunctional: for example, well-designed goals can focus attention on particular in-game information, but also motivate overall gameplay and scaffold learning; rewards can stoke emotional responses that may directly support the encoding of associated information in memory. “Everything is deeply intertwingled” (Nelson, Citation1974, p. 45). But instead of constructing a universal theory of learning, we analytically bracket such other aspects to squarely focus on one: attention.
Games have long been recognized as “a medium that demands our attention” (Bowman, Citation2018). Numerous models of game engagement, involvement, or immersion equate the former with attention being absorbed by gameplay (see, Calleja (Citation2011), for a review). Mood management theory, flow theory, and other accounts present this attentive absorption as one if not the major gratification people seek out in gameplay (Csikszentmihalyi, Citation1991; Goffman, Citation1961; Malaby, Citation2007; Reinecke, Citation2017; Schull, Citation2012). Yet these accounts notably concern themselves with how gameplay holds our attention as a whole (and why we enjoy this). They do not discuss how specific game design features focus attention on specific information within a given stretch of gameplay, nor how this attentional focus affects learning.
This idea – that attention affects experience and learning – is of course similarly not new. Many basic usability and interface design principles in human-computer interaction (like recognition over recall or using visual hierarchies) are based on fundamental findings on people’s visual attention and working memory (J. Johnson, Citation2020). HCI researchers and interaction designers regularly measure attention using eye tracking to investigate users’ experience of interface and information designs (e.g., Bergstrom & Schall, Citation2014; Bojko, Citation2013; Majaranta & Bulling, Citation2014), or develop computational models of attentional processes to predict users’ experience before designs are implemented (e.g., Halverson & Hornof, Citation2011; J. R. Anderson et al., Citation1997). Several general learning and media effects theories acknowledge attention implicitly or explicitly, including Social Cognitive Theory, where imitation learning requires attending to the behavior to be imitated (Bandura, Citation2001); Cognitive Load Theory (Sweller et al., Citation2011), a highly influential theory in educational research centering on limited working memory capacity; and the Limited Capacity Model of Motivated Mediated Message Processing (Lang, Citation2000), which places attentional selection at the heart of media effects.
Yet as we will show, none of these existing approaches or theories incorporate attentional selection and sampling mechanisms recognized in contemporary attention research.
Even more importantly, existing theories on attention and learning do not address the specific characteristics of video games as interactive media, since they were originally developed to model information processing in non-interactive media like television or textbooks. Gameplay differs from non-interactive media use in that it is participatory and task-directed: games demand players to perform certain tasks to attain goals, and display changed information in response to player performance. Following attention research (e.g., Chun et al., Citation2011), this task pursuit ties into a set of specific attentional mechanisms that direct players’ attention both onto the game and within the game onto task-relevant information. These mechanisms are not captured in the current theory yet essential for understanding, explaining, and predicting whether and when players learn from gameplay.
To model how task-based attention affects learning from gameplay, this paper introduces the Task-Attention Theory of Game Learning (shortened to task-attention theory). In short, task-attention theory proposes that (1) players’ learning is strongly moderated by what they attend to, and learning improves with increased attention; (2) what players attend to is determined by attentional selection and sampling mechanisms; (3) attentional demands like pressure or cognitive load moderate selection and sampling; (4) gameplay involves distinct task-based mechanisms and demands, which sets it apart from consuming non-interactive media; (5) game design can direct and sustain attention through identifiable features that tie into these task-based mechanisms and demands; and (6) attentional mechanisms and features found in non-interactive media also operate in video game play.
We will first briefly introduce key concepts in the psychology of attention, including task-based attentional selection, sampling, and learning (section 2). We will then review how attention has been treated in current theories of HCI, media processing, and learning (3). We find that task-based attention features are minimally at best in current theories. Section 4 will introduce task-attention theory, specifying (4.1.1 and 4.1.2) task-based attentional selection and sampling as core mechanisms (4.2), player dispositions, and game features that generate attentional direction through these mechanisms, and (4.3) task-based attentional demands. For each construct, we present current evidence, how it manifests in games, and differs from non-interactive media. We then discuss ramifications for game-based learning (5.1) and design (5.2), showing how task-attention theory offers a coherent explanation for intrinsic integration as a widely endorsed meta-design principle for learning games. We expand on ramifications for other fields (5.3) and conclude (6) with a summary and brief outlook.
2. A brief introduction to attention
2.1. Key terminology
Attention describes the fundamental phenomenon that organisms have a limited capacity to process sensory and internal information and therefore “select, modulate, and sustain focus on information most relevant for behavior” (Chun et al., Citation2011, p. 73). This is brought about by enactive, perceptual, and cognitive attentional processes.Footnote1 Notably, while these processes do steer what information reaches consciousness, attention is not the same as awareness: Much of the information we attend to, process, and are affected by does not give rise to conscious awareness; similarly, many attentional processes are themselves involuntary and unconscious (Dijksterhuis & Aarts, Citation2010). Attentional selection describes the processes that determine which information is picked up or ignored, as opposed to other attentional processes, such as suppression (reduction of processing resources; Chun et al., Citation2011).
Attention manifests across the whole perception-action loop (Rensink, Citation2015). For instance, attentional selection already happens in what we direct our sensory organs toward which is known as overt attention. Yet looking at an object does not necessarily mean that we will actually deeply process the information in the light being reflected from the object into our eye. Covert attention describes those internal processes, which direct processing resources to and away from stimuli within the field of perception (Mack & Rock, Citation1998). Attention can be directed spatially, that is, to or away from a particular location and onto or away from particular stimulus features such as color, orientation, or movement (Carrasco, Citation2011).
One further important distinction is that between bottom-up attentional mechanisms (also called stimulus-driven, exogenous, reflexive, or automatic), and top-down (also goal-directed, endogenous, or non-automatic) mechanisms (Chun et al., Citation2011). In bottom-up mechanisms, stimulus or task characteristics involuntarily focus attention on themselves – think of how a sudden loud noise has us involuntarily turn our heads and pay attention to its direction. In top-down mechanisms, organism-internal goals and sense-making direct attentional selection. Top-down mechanisms can but need not always be conscious and deliberate (e.g., Kuhn et al., Citation2016).
2.2. Task-based attention
In psychological parlance, when people pursue a concrete goal like “ironing this shirt” or “solving this equation”, they are said to perform a task. This requires readying, orienting, and maintaining a task set: the complete bodily and cognitive processes and resources needed to perform said task, as research on the difficulties of multitasking and task switching demonstrates (Koch et al., Citation2018). Part of such a task set is an aligned attentional set (Most & Astur, Citation2007), which determines what information receives attention and what is unattended. This is starkly demonstrated by the phenomenon of inattentional blindness (Mack & Rock, Citation1998). In inattentional blindness experiments, participants are given a task to perform. While they perform the task, a task-unrelated unexpected event happens, which participants typically fail to notice. For example, Simons and Chabris (Citation1999) had participants count the number of passes made by basketball players. While they were doing this, someone in a gorilla suit passed through the players, which the majority of participants did not notice, as their attention was focused on the basketball. Hence, participants did not consciously recall seeing the gorilla.
This in itself would not be sufficient evidence that task-based attention impacts all kinds of learning. Consider that not all learning requires conscious awareness or recall of what is learned: many learning processes and outcomes are tacit or implicit. Yet studies show that task-based attentional selection also affects learning of tacit skills (Eitam et al., Citation2013; Kinoshita, Citation1995), and attention in general has been found to strongly moderate implicit (= non-conscious) learning (Cleeremans et al., Citation1998; Vuilleumier et al., Citation2005).
Aligned with this general literature on task-based attention and learning, attentional selection has been found to moderate learning effects in interactive media and games. Both Xu and Sundar (Citation2016) and Sreejesh and Anusree (Citation2017) found that higher levels of interactivity increased attention on the elements, which were interacted with and increased recognition of those elements afterward. Wood and Simons (Citation2019) found task-dependent spatial inattentional blindness in an interactive environment similar to the video game Frogger (Konami Digital Entertainment, Citation1981). Most and Astur (Citation2007) found feature-based attentional selection in a driving simulator, similar to driving games. Cutting et al. (Citation2020) cloned the popular self-paced puzzle game Two Dots (PlayDots, Citation2014) and found that players only retained game features such as graphic images if they were relevant to the game task. Other features were ignored and not recalled. They hypothesized that players knew that the graphic elements were present but suppressed attention onto them which reduced retention.
2.3. Active sampling
As ecological analyses show, organisms do not passively receive all perceptual information available in their environment: they continually actively move their bodies and sensory organs and manipulate their environment to sample and elicit action-relevant information (Linderoth, Citation2013). In attention and perception research, this is referred to as active sensing (Schroeder et al., Citation2010) or active sampling (Gottlieb & Oudeyer, Citation2018). To give some simple examples, we need to actively move our fingertips to feel the texture of a surface or move our heads to see behind an occluding object. Counter to long-standing laboratory research traditions that experimentally dissociate attention and decision-making processes, active sensing and sampling research points out that active sampling forms a necessary part of attentional (selection) processes, involving conscious and subconscious decisions about what information to sample (Gottlieb, Citation2018). This will become crucial to our argument because games constitutively involve user actions that prompt the medium to display selected information. To engage in gameplay is to necessarily engage in active sampling, which is comparatively minimized in non-interactive media.
3. Attention in current HCI, media effects, and learning theories
Given the robust evidence that attention affects performance and learning, one would assume it to feature prominently in contemporary HCI, media effects, and learning theories. As we will see, current HCI approaches do commonly tackle overt attention and considerations of limited cognitive capacity are indeed well-represented in current media and learning theories, yet attentional selection, especially top-down and task-based selection and sampling, are mostly absent. To assess how current research has engaged with links between attention and learning on the micro-level of individual users and media offerings,Footnote2 this section presents a mini-review of relevant approaches and theories. We consider HCI approaches to attention (eye tracking, cognitive models, information foraging), general media effects models (LC4MP and the recent interactivity-as-demand perspective) and learning and educational game theories (covering Cognitive Load Theory, Multimedia Learning Theory, and the Cognitive Theory of Game-Based Learning).
3.1. Human-computer interaction
Attention research has found widespread use in HCI (see, Roda, Citation2011, for a review). The main application area has been deriving pragmatic principles and heuristics for interface and interaction design (see, J. Johnson, Citation2020, for a review). Methodologically, this has been paralleled by the use of eye tracking to record overt attention within graphical user interfaces (GUIs; e.g., Bergstrom & Schall, Citation2014; Bojko, Citation2013; Majaranta & Bulling, Citation2014). Beyond its practical use in usability testing, eye tracking is used to understand basic human-computer interaction processes like how interface elements are used (Byrne et al., Citation1999), or how users interpret structured information designs (e.g., Djamasbi et al., Citation2011; Hornof & Halverson, Citation2003). A second area of work is computational models of user’s visual search and attention (e.g., Halverson & Hornof, Citation2011; J. R. Anderson et al., Citation1997), sometimes as part of more general computational models of human cognition like ACT-R (Fu & Pirolli, Citation2007). Such computational models are then used in adaptive systems, which are aware of users’ attentional state (Roda & Thomas, Citation2006), and computational interfaces that automatically design and optimize user interface elements, such as keyboards and webpage layouts (Jokinen et al., Citation2017; Todi et al., Citation2019). A third area is designing against user attention being fragmented by continuous interruptions and multi-tasking (Case, Citation2015), e.g., by supporting peripheral interactions (Bakker et al., Citation2016). Arguably, the most interesting and relevant theoretical HCI contribution to attention research is information foraging (Pirolli, Citation2005; Pirolli & Card, Citation1999), which proposes a rational cost–benefit analysis model of how users gather information in an environment, highlighting “information scent” as cues (like a link text) that signal probable information utility gained when following them through. This more or less aligns with concepts of active sampling that task-attention theory draws on.
However, current considerations of attentional processes in HCI are of limited value for the present project, as they chiefly consider modeling and designing for attention in the service of direct instrumental goal pursuit – how attention moderates learning processes does by and large not figure. Furthermore, eye tracking studies and clickstreams as the major measurement methods have the disadvantage that they focus only on overt spatial attention (where people visibly look and click), rather than considering covert or feature-based selection. This leads to the flawed assumption that if a user’s gaze or pointer is focused on an area, then their full attention must be directed to processing all of the information in that area. This is contradicted by the eye tracking studies of “Banner Blindness” (Hervet et al., Citation2011) which indicate that although users’ overt attention may be fixated on advertising banners, their covert attention is focused on performing the task that prompted visiting that webpage, resulting in low rates of recall of the adverts. Similarly, HCI models of attentional processes tend to consider only very low-level moderators of attention such as visual saliency (e.g., Leiva et al., Citation2020).
3.2. General media effects models
The Limited Capacity Model of Motivated Mediated Message Processing (LC4MP; Lang, Citation2000, Citation2006) is arguably the most prominent media effects theory that acknowledges that media effects are moderated by the selective allocation of limited information-processing resources. In this model, media may present too much information at a time for all of it to be processed and remembered, so attentional processes determine which media information receives processing resources. Information that receives no or less cognitive resources is not or less well-encoded, stored, and retrieved. LC4MP sees resource allocation affected by top-down user goals (a mechanism that is recognized but not really specified), bottom-up orienting responses to so-called orienting-eliciting structural features (OESFs) like novel, motivationally relevant, or signal stimuli, and by motivated processing. LC4MP assumes two basic motivational systems – appetitive and aversive – that can be activated or not in parallel by message properties, and that are differentially active in different people, explaining, for example, why media preferences may vary in preferred levels of arousal due to the presence of threat or risk situations. Elicited arousal levels and appetitive/aversive system activations are seen to differently affect resource allocation, and thus, memory. Information presented close to OESFs or in moderately arousing media is therefore predicted to be better encoded, stored, and retrieved. Recent systematic reviews find overall good support for these and other predictions of the LC4MP (Fisher et al., Citation2018).
While the LC4MP positions itself as media-agnostic, it was primarily developed around ‘passive’ television-viewing. Hence, although there have been several LC4MP-based studies of interactive media and even games (see, Fisher et al., Citation2018, for a review) these studied standard OESFs already found in television, like emotional content or sudden on-screen movement. And while LC4MP acknowledges attentional selection by top-down user goals, these are far less well articulated and studied than OESFs. Furthermore, LC4MP conceptualizes user goals as global perceptual-cognitive goals like entertainment or finding a particular information. It does not acknowledge that in interactive media, users regularly perform specific tasks in pursuit of pragmatic goals, which produces task-based attentional selection.
The recent “Demand Perspective” can be read as a partial response to this oversight of interactivity: spearheaded by Bowman (Citation2018), this line of research proposes that the process of interactive media use (like gameplay) uniquely puts various cognitive, emotional, physical, and social demands on users. These demands are seen to mediate many effects and experiences characteristic for interactive media. Explicitly drawing on LC4MP, Bowman (Citation2018, p. 7) acknowledges that cognitive demands encompass attentional demands and that users have limited cognitive resources, leading them to selectively (dis)attend to information in information-dense game stimuli. However, in the actual operationalization of cognitive demands in Bowman et al. (Citation2018)’s Video Game Demand Scale, we find no attention-related items. Furthermore, demand perspective research has – to date – not articulated or tested specific antecedents, mechanisms, or consequences of attentional demand as a presumed subset of cognitive demands.
In summary, LC4MP has identified several attentional selection mechanisms and connected media features and demonstrated their impact on learning: there is evidence that these mechanisms also operate in interactive media like games. Yet, it does not incorporate task-based attentional selection characteristics for interactive media. Demand perspective research has zeroed in on the demand characteristics of interactive media, but to-date has not modeled attentional demand and its impact on learning.
3.3. Learning and educational games
In educational research on games, attention primarily figures as a learning outcome. Action games that demand ongoing focused attention, rapid and flexible visual search, and rapid task-switching improve players’ attentional and executive control abilities at both neurological and behavioral levels (Cardoso-Leite et al., Citation2020; Gan et al., Citation2020; Green & Bavelier, Citation2012). Thus, in playing action games, players learn to attend ‘better’ in general, which thus may improve their overall performance and learning ability. Educational researchers, by and large, have not inferred from this that the same demands that train attention may also moderate learning: “attention” is notably absent across the key contemporary theories of digital games and learning (see, Whitton (Citation2014), for a review). One exception is Kiili (Citation2005)’s experiential gaming model, which sees flow states as the main driver of learning in computer-mediated environments like games, and acknowledges focused attention as an antecedent of flow.
This is all the more surprising as Cognitive Load Theory (CLT), arguably one of the most influential cognitive theories of learning and instruction today, is constructed around limited information processing capacities (Sweller et al., Citation2011). CLT argues that limited working memory capacity mediates whether information is learnt, that is, stored as new or changed schemata in long-term memory. Different information requires different amounts of working memory capacity, which is referred to as cognitive load. Intrinsic load describes load due to the inherent complexity of to-be-learnt information. Extraneous load are other elements of the perceived learning material that occupy working memory but don’t need to be learnt. Germane load is the remaining working memory capacity that can be allocated to long-term storing information.
Importantly, CLT assumes that people indiscriminately absorb all information presented to them into working memory until capacity is reached. Thus, all observed effects and derived instructional design principles in CLT revolve around minimizing extraneous and modulating intrinsic load to optimally fit fixed working memory capacity (Sweller et al., Citation2011). CLT recognizes that “split attention” – having to forage information from two separate locations like a diagram and its spatially distant labels – reduces learning. But it models this as extraneous load (ibid., pp. 111–128). In fact, Sweller and others expressly stipulate that attention requires no separate treatment: “We assume that limitations in attentional resources can be explained by the limited capacity of working memory” (ibid., p. 96). Put differently, CLT ignores that organisms engage in any form of active attentional selection.
By contrast, the CLT-derived theory of multimedia learning (MMT) (Mayer, Citation2009) and connected Cognitive Theory of Game-Based Learning (CTGBL; Mayer, Citation2020) both acknowledge that “active processing” involves selecting what information encoded in “sensory memory” is actually processed in working memory (ibid., pp. 88–89). However, MMT and CTGBL do not work out how this selection occurs nor what media features affect selection, with the exception of signaling: pointers, highlighting, and the like improve learning by directing people’s attention toward essential information (Mayer, Citation2009, pp. 108–117). MMT and CTGBL also ignore that active sampling affects what information reaches sensory memory.
Furthermore, neither CLT nor MMT or CTGBL recognize attentional mechanisms linked to the interactive, task-based nature of games. Games for them are chiefly multimedia: bundles of visual-and-auditory/verbal media types, which make use of presumed-separate-yet-interacting verbal and visual processing channels (Mayer, Citation2009). Interactivity here chiefly entails that media systems can just-in-time personalize the information presented to an individual learner to fit their schemata and working memory capacity (Mayer, Citation2020).
In summary, while game-based learning research acknowledges that gameplay demands and trains attention, current theories in the field by-and-large ignore task-based attentional selection and sampling as a learning moderator, with the partial exception of signaling in MMT/CTGBL.
4. How task-based attention moderates game learning
The previous sections established that task-based attention affects learning. A range of influential media effects and learning theories acknowledge that human information processing capacity is limited. However, CLT as the major educational theory assumes that people indiscriminately absorb all information presented. Theories that acknowledge attentional selection – LC4MP, MMT, CTGBL – predominantly focus and flesh out bottom-up media features; top-down processes are not or minimally (LC4MP) modeled. Most importantly, currently theorized media features and mechanisms – like motivational relevance, emotional content, novelty, or signaling – are not distinguishing characteristics of interactive media, nor do they touch on task-based attentional selection or sampling. This is not to say that features like novelty or emotional appeal don’t matter: they do steer attention, in interactive and non-interactive media alike. But it highlights a crucial gap in current theories when it comes to how player attention is steered in games.
To fill this gap, we propose the Task-Attention Theory of Game Learning, which we will refer to as task-attention theory from now on. We chose this (arguably generic) form for two reasons: one, it’s short. Second, we believe that the mechanisms specified here could and should in principle generalize beyond game learning to the effects of interactive media (see, esp. section 5.3.4). The generic form allows for such future generalizing work.
integrates the key propositions of task-attention theory into a graphic nomological network:
Figure 1. Nomological network of task-attention theory, showing how learning is moderated by task-related attentional mechanisms. Task-based design features (A) direct attentional selection and sampling (C) onto task-relevant information, which (D) is learned, including task-related attentional sets that steer future sampling and selection. Game tasks also (B) create specific demands that affect attentional selection and sampling. The model recognizes that non-task-based design features (E), also found in non-interactive media, similarly affect learning.
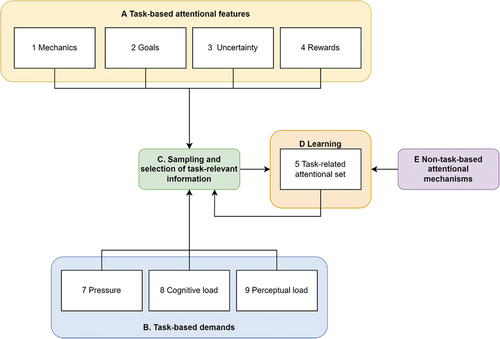
Put simply, task-attention theory posits that:
Learning is strongly moderated by attention – people are more likely to learn what they attend to.
What people attend to is determined by attentional selection and sampling – the information people actively elicit from the world and selectively attend to, which are steered by learned attentional sets.
Video game play characteristically invokes task-based attentional mechanisms – this sets video games and other interactive media apart from non-interactive media.
Game design can direct and sustain attentional sampling and selection through identifiable design features – features, such as mechanics, goals, uncertainty, or rewards.
Attentional sampling and selection are affected by attentional demands – pressures and perceptual and cognitive loads.
Non-task-based attentional mechanisms and demands found in non-interactive media also moderate learning in video game play.
(i) Learning is moderated by attention.
This proposition stakes the major practical import of task-attention theory: in line with prior theories and current research, task-attention theory acknowledges that human information processing capacity is limited, wherefore people differentially process information. Choice and depth of processing moderate whether and how well information is learned.
(ii) What people attend to is determined by attentional selection and sampling, which are steered by learned attentional sets.
Here, task-attention theory parts with and expands on prior CLT-based theories: People are not passively exposed to a stream of stimuli emanating from a media offering: they actively elicit and sample information from it. This sampled information is selectively attended to, guided not just by bottom-up media features, but also top-down user goals and learned dispositions: as part of learning to play a game, people acquire mental models and perceptual encodings of the game and its stimulus array, which form part of the attentional set directing sampling and selection to relevant information.
(iii) Video game play characteristically invokes task-based attentional mechanisms and demands.
This proposition articulates that attentional direction in games and interactive media differs from non-interactive media and marks where task-attention theory goes beyond the mechanisms articulated in theories such as LC4MP.
As we have seen, task pursuit involves particular and particularly strong attentional selection processes, organized by attentional sets. These task-based attentional processes are characteristically invoked by games. Games are “the medium of agency” (Nguyen, Citation2020): to play a game is to be agentic, to act, do, strive, pursue.Footnote3 This agency, in turn, is afforded by the interactivity of games. We follow (Murray, Citation2011) in specifying games as interactive media with four key affordances: they are procedural, participatory, spatial, and encyclopedic. Procedurality means that displayed information is not fixed but selected or generated by some algorithm. They are participatory in that algorithms depend on and process ongoing user input to determine output information – what Aarseth (Citation1997) calls ergodicity. Such input often consists of navigating (and with that, sampling) a (virtual) information space in a more or less embodied fashion. Finally, displayed information is a tiny selection from a vast or encyclopedic possibility space of stored or generatable information. Taken together, these affordances mean that play or game ‘usage’ is not just inherently performative – doing and pursuing tasks – what information is presented by a game is constitutively co-determined by the game user’s actions and choices.
(iv) Game design can direct and sustain attentional sampling and selection through identifiable design features.
Just like LC4MP identifies a range of orienting-eliciting structural features that direct attention, so task-attention theory articulates a range of structural features of games that steer attentional selection and sampling. Specifically, games direct attention through (a) game mechanics which specify possible tasks and (b) explicit goals for these tasks that require non-trivial effort to attain. Succeeding at goal-specified tasks is typically (c) uncertain and comes with (d) rewards, which further direct attention on likely rewarded and uncertainty-reducing task information.
(v) Attentional sampling and selection are affected by attentional demands.
Prior theories like CLT or LC4MP model demands as cognitive load that moderates the processing, storage, and retrieval of information. The more (extraneous) load, the less capacity is available for processing, storage, or retrieval. Task-attention theory instead unpacks how different kinds of task-based demands moderate attentional direction. Specifically, it identifies (a) time and performance pressure which can narrow attentional focus on task-relevant information but also generate cognitions that occupy executive control resources otherwise available for top-down attentional control; (b) task-related perceptual load which reduces susceptibility to distraction; and (c) task-related cognitive load which again reduces available resources for top-down attentional control.
(vi) Non-task-based attentional mechanisms and demands found in non-interactive media also moderate learning in video game play.
This proposition articulates the relationship of task-attention theory to prior theories: task-attention theory acknowledges the constructs and relations they propose for attention, but brackets them for the sake of parsimony. We emphasize here again that the theory also brackets any non-attentional mechanisms that affect game learning (e.g., motivation), recognizing that many features and demands our theory identifies also tie into these non-attentional mechanisms.
The following subsections will each unpack core constructs and relations of our nomological network, grouped into the core mechanism of attentional sets, selective attention, and active sampling (4.1.1 and 4.1.2) task-based game features driving sampling and selection (4.2), task-based demands (4.3), and mechanisms not specific to task pursuit (4.4). For each construct in our model, we specify its relations with other constructs as directional predictions. We identify these in the nomological network () by noting the specific nodes and directional edges after each prediction. For example, “1→C” denotes the predicted relation that “1 Task-related mechanics” lead to “C Sampling and selection of task-relevant information.” summarizes the formal predictions of task-attention theory:
Table 1. Formal predictions of task-attention theory
4.0.1. Attentional sets and attentional selection
How do players determine what information is task-relevant and thus belongs in an attentional set? Attentional sets can be seen to comprise and flow from the player’s mental model of the game, i.e., their internal representations of what inputs they can produce (the mechanics or verbs) and how the game as a system produces outputs in response to the player’s inputs (e.g., Martinez-Garza & Clark, Citation2017). Learning a game and learning from a game, are both understood to centrally involve building up such mental models (Wasserman & Koban, Citation2019).
As has been exhaustively studied with expert chess players, mental models encompass different hierarchical levels of task-relevant schemata (chunks, templates) that expert performers use to process, encode, and retrieve information about a task – and that direct their attention. Expert chess players rapidly and holistically perceive and encode the total board in terms of higher-level position patterns; they more rapidly direct their attention to those parts of the board that are relevant than novices; and they spend more time attending to task-relevant board areas than novices (Sheridan & Reingold, Citation2014). This is called perceptual encoding to indicate that learned schemata already operate at the stage of early (involuntary, non-conscious) perception, steering, e.g., overt attention in the form of eye fixations (Sheridan & Reingold, Citation2014).
At the most basic level, players learn to differentiate and attend to the perceptual features and units in the game stimulus array that are task-relevant and manipulable to begin with: in Pac-Man (Bandai Namco Entertainment, Citation1980), for instance, players learn to pay attention to Pac-Man and how he reliably responds to joystick input, or to ghosts, where they move, and whether they are purplish-blue (which signifies they can be eaten). Put differently, players learn to perceive and selectively attend to affordances – the action opportunities the game offers relative to their dispositions (Linderoth, Citation2013; Rambusch, Citation2011). As Linderoth and Bennerstedt (Citation2007) demonstrate in a video interaction analysis, acquiring general gaming skills and skill in a particular game entails developing the “professional vision” (Linderoth & Bennerstedt, Citation2007, p. 602) of picking up and attending to just those game stimulus features that have pragmatic-functional meaning in the game (like a red glow indicating an enemy’s health status), actively disattending all nonfunctional information regardless of how “lush,” “beautiful,” or “immersive” it may be – at least, while players are under task performance pressure. ”Admiring the scene” is a form of game engagement available when cognitive resources are neither occupied nor orientated by task pursuit.
This is supported by Cutting et al. (Citation2020), who found that players disregarded game information not functionally required to attain the game’s goals, and Eitam et al. (Citation2013), who manipulated participants’ mental models and found that this moderated covert attention, which in turn moderated learning (in this case, of an artificial grammar). Notably, both studies controlled for cognitive load and found that learning was moderated solely by attention, not cognitive load, contradicting CLT claims (Sweller et al., Citation2011) that working memory capacity can account for such attention effects.
In short, as players interact with a game, they build a mental model of how the game works and which features and higher-level patterns of the game stimulus array are important for performing game tasks. Bottom-up, they learn to perceptually encode game stimuli in terms of task-relevant features and units (such as affordances), which then selectively focuses attention on just these features and units. Top-down, players may engage in action planning, consciously directing attention to those parts and aspects of the game stimulus array relevant for deliberating alternative courses of action or performing a chosen task. Mental models and perceptual encoding form constituent parts of the player’s task-related attentional set: as we learn to play a game well and attain its goals, we learn to pick up and selectively attend to task-relevant game information. This is a positive feedback loop: task performance reveals what information is task-relevant, how the game is manipulable, etc., which builds up an attentional set that directs attentional selection on that likely relevant information during task performance. Formally expressed, task-attention theory predicts that (a) learning to play video games entails the buildup of task-related attentional sets, including mental models and perceptual encodings; (b) stronger task-related attentional sets will lead to more focused attentional selection onto task-relevant information; (c) stronger attentional selection onto task-relevant information will lead to greater learning of task-relevant information.
In non-interactive media such as movies or books, users similarly build up mental models (of the world, its characters, the plot) that direct attentional selection (Bower & Morrow, Citation1990). However, these are arguably guided by global usage goals like story comprehension, and not anchored on what the user can or cannot do. Non-interactive media don’t constitutively specify concrete tasks to perform; users may self-devise tasks (e.g., count bloopers), or tasks may be set by instrumental contexts (e.g., devised by a teacher), which then direct task-based selective attention. However, this neither arises from the structure of the medium and interaction, nor train up task-related mental models and perceptual encodings through learning-by-doing. This difference directly ties in with constructivist and constructionist theories of learning that argue games and interactive systems are particularly good at supporting learning because they give learners controllable microworlds to interact with or build, which allows them to test and revise internal models of the world via active, experiential learning (Kafai & Burke, Citation2015; Whitton, Citation2014, pp. 26–31). Books and movies do not afford looking for and then prodding the antagonist to see whether our mental model of their behavior is correct, which leads us directly to active sampling.
4.0.2. Active sampling
Video games as interactive media enable and indeed demand active sampling. To return to Murray’s (Citation2011) terminology, interactive or computing media are procedural, participatory, encyclopedic, and spatial: They are procedural in that displayed information is not fixed, but generated or selected by some algorithm. What information is displayed in what sequence crucially depends on active user input being processed by this algorithm, making the medium participatory. Finally, interactive media are encyclopedic and spatial in that they can store unparalleled amounts of information, which are only ever selectively accessed and revealed by navigating through them: while any normal viewer can watch all 121 minutes of Star Wars, no player will ever visit all 18 quintillion worlds of No Man’s Sky (Hello Games, Citation2016).
This procedural, participatory, encyclopedic, and spatial constitution of interactive media means that the information that a game presents to a player (out of the total possibility space of its stored assets or content-generating algorithms) is co-determined by the player’s ongoing inputs, which the player deliberately steers in their task pursuit. In most video games, most players never exhaustively see all content, let alone all its possible sequential permutations: player choice determines which parts they see in which sequence. Active sampling research suggests that such choice is guided by pragmatic and epistemic value: we actively sample just that information, which we expect will either most effectively guide our action in pursuance of desired rewards or most effectively increase our understanding of how we can effectively act in the world to produce rewards (Gottlieb & Oudeyer, Citation2018). In task-based attention terms, we will sample that information, which we believe – based on our attentional set – will best guide successful task pursuit (pragmatic value) or best inform and improve our attentional set (epistemic value).
Many video games turn this active sampling into a core game mechanic in itself. Take the card game Memory (also known as Pairs or Pelmanism): this game is about judiciously choosing, which cards to reveal to build up a mental model of the hidden board state. Part of the skilled gameplay in, e.g., multi-player shooters and MOBAs is the constant active manipulation of the game’s camera and viewport to make available to your senses just that game state information relevant to your current task (Reeves et al., Citation2017, pp. 324–326). In the popular indie game Papers, Please (Pope, Citation2013), part of the core gameplay is managing the information displayed by placing in-game objects and opening or closing in-game menus.
It is technically true that non-interactive media audiences need to act as well to reveal a media offering’s information (e.g., flip book pages). But non-interactive media offerings are designed for sequential (not procedural), fixed (not participatory), and exhaustive (not selective) consumption. Skipping, scrolling, browsing to, e.g., locate a particular information is not the default mode of engagement, whereas to play is to explore and prod the medium to see how to best attain the game’s goals.
To summarize, as players pursue game tasks, they not only overtly and covertly select attention onto task-relevant features and areas in their perceptual field, as identified in their attentional set but also actively and selectively manipulate the game to generate, select, and position information into their perceptual field, which they expect will best serve their task pursuit or improve their gameplay skills and attentional set. This makes active sampling an attentional precondition to any learning – we can only process and learn the information we sample from the environment. However, in another feedback loop, this also makes active sampling the result of learning. Prior game knowledge (in the form of attentional sets) and perceived knowledge gaps steer us to sample just that information, which we believe will best improve said knowledge. Formally, stronger task-related attentional sets will lead to more focused active sampling of task-relevant information; and stronger active sampling of task-relevant information will lead to greater learning of task-relevant information.
This aligns with prominent critiques of “minimally guided instruction” as found in constructivist, inquiry-, or problem-based learning (Kirschner et al., Citation2006) who argue that learners use prior learned schemata to parse relevant information from their environment. Handing novices without any such prior schemata a new learning problem without explicit guidance will therefore burden them with the extraneous cognitive load of having to indiscriminately process all information available. Task-attention theory agrees with this problem diagnosis, but it specifies attentional mechanisms and capacities (active sampling and selection driven by attentional sets) operating prior to working memory that explain why this poses a problem.
4.1. Task-based attentional direction
We have now set out the core mechanism of task-attention theory: task-based active sampling and attentional selection steer attention and learning toward task-relevant information, which builds up task-based attentional sets that in turn steer future sampling and selection. In this section, we will set out key structural game features that evidence suggests affect these attentional mechanisms and that designers can manipulate: mechanics, goals, uncertainty, and rewards.
4.1.1. Mechanics
Game designers and researchers commonly present the “mechanics” (“methods invoked by agents, designed for interaction with the game state” (Sicart, Citation2008)) or “verbs” (“any rule that lets the player do something” (Anthropy & Clark, Citation2014, p. 14)) that specify player actions as the central aspect of game design. In any given game scene or level, the specific in-game objects and environment, rules, and goals then structure an array of varied tasks for actuating those verbs or mechanics in specific, hopefully new and interesting ways (Anthropy & Clark, Citation2014, pp. 40–70). For example, a different arrangement of candies to swap every Candy Crush Saga (King, Citation2012) level or a different arrangement of platforms and obstacles to navigate in every Super Mario Bros. (Nintendo, Citation1985) scene.
Put differently, mechanics articulate the possible tasks of a game; playing video games revolves around doing – and learning how to do – these tasks (Nguyen, Citation2020). As players learn to differentiate the game interface’s affordances for actuating mechanics and doing tasks, they build up task-related attentional sets, which guide them to selectively attend to that information in the game stimulus relevant to performing the task: the distance of a platform relative to the character’s maximum jump distance, for instance, rather than the pixel texture of the platform or the current score counter. This attentional selection guided by attentional sets thus fundamentally moderates what information available actually gets processed at what depth, and thus can be learned.
4.1.2. Goals
By many accounts (Juul, Citation2005, pp. 29–40) one defining feature of games are goals that players have to exert effort to attain, such as beating your opponent in a multiplayer game, narrative quests (rescue the prince), achievements (heal X friends in under Y seconds), or player-defined goals (Björk & Holopainen, Citation2006) like trying to catapult a goat on-top of a building in Goat Simulator (Coffee Stain Studios, Citation2014). Similarly, gameplay is co-constituted by actively pursuing the goals of the present game (Deterding, Citation2013): a player doing something other than autotelically pursuing a game’s goals is not, by common understanding, playing that game.
Where game mechanics afford possible tasks, game goals specify concrete tasks for a player to complete, albeit the task of exploring the game interface and game world to discover how to attain the goal. Thus, goal pursuit will again lead players to over time acquire, and in the moment, activate attentional task sets that direct player attention onto the task itself and within the task, onto task-relevant information. This aligns with rich evidence that just having a goal can direct attention toward goal-relevant activities (Locke & Latham, Citation2002), and that goals increase the consistency of attention and reduce distraction (Robison et al., Citation2020). Put formally, task-attention theory predicts that task-related goals will lead to increased sampling of and attentional selection onto task-relevant information.
4.1.3. Uncertainty
As players pursue goal-specified game tasks, they are likely to experience uncertainty (Abuhamdeh et al., Citation2015; Kumari et al., Citation2019; Power et al., Citation2018).Footnote4 Uncertainty is recognized as a key design feature of games afforded by, e.g., stochastic uncertainty about random events, the unpredictability of other players, hidden information, or narrative uncertainty about how events will play out (Costikyan, Citation2013).
As the name indicates, narrative uncertainty is also found in non-interactive narrative media like films and novels, where it is linked to engaging and attention-directed suspense (Delatorre et al., Citation2018). However, following recent qualitative work by Kumari et al. (Citation2019), task pursuit in games entails certain unique forms of uncertainty not found in non-interactive media, namely decision uncertainty (what shall I do?), interaction uncertainty (will I do it successfully?), adaptation uncertainty (how good am I at doing it?), and result uncertainty (what will happen as a consequence of what I do?). Game designers intentionally manipulate the difficulty of a game to make task attainment uncertain, shooting for a goldilocks optimum of uncertainty that is believed to maximally absorb player attention (Abuhamdeh et al., Citation2015; Costikyan, Citation2013, p. 72).
This matters because uncertainty – and different kinds and degrees of uncertainty – have been found to direct bottom-up attentional selection onto those stimuli and stimulus features, which promise to resolve uncertainty, using both EEG measurements (Dieterich et al., Citation2016; Nelson & Hajcak, Citation2017; Tanovic et al., Citation2018) and eye-tracking (Walker et al., Citation2017). Active sampling work similarly shows that people forage their environment for uncertainty-reducing information (Gottlieb & Oudeyer, Citation2018). This is usually theoretically linked to interest or curiosity as a learning-optimizing intrinsic motive or policy (Kidd & Hayden, Citation2015): humans are intrinsically motivated to seek out information that improves their long-term ability to predict future world states and select and execute actions resulting in desired future world states. Information-theoretically, uncertainty reduction (over future states or actions) equals information gain. Thus, current formal theories of curiosity suggest that all else being equal, humans actively sample and focus attention on situations and actions where they expect the greatest uncertainty reduction (Friston et al., Citation2017; Gottlieb & Oudeyer, Citation2018). Uncertainty reduction or information gain is seen as a distinct intrinsic reward that can consciously manifest as the positively valenced experience of insight or relief from the negatively valenced experience of an information gap. Large volumes of empirical work support that “moderate” levels of uncertainty best hold attention (Kidd et al., Citation2012) where agents bring some but not highly precise prior expectations and therefore can expect the highest uncertainty reduction (Kiverstein et al., Citation2019).
Research on uncertainty and curiosity in games is still at an early stage (Kumari et al., Citation2019), but as we can see, psychology and neuroscience suggest that task-related uncertainty directs attention to uncertainty-reducing actions and information through multiple bottom-up and top-down processes so as to optimize the agent’s learning progress. Put formally, task-attention theory predicts that task-related uncertainty will lead to increased sampling of and attentional selection onto information that is expected to reduce task-related uncertainty.
4.1.4. Rewards
A robust and growing body of literature suggests that stimuli which are associated with some relevance (B. A. Anderson et al., Citation2011), such as threat stimuli, emotionally charged stimuli, or stimuli that are rewarding (Pessoa, Citation2015), draw attention via both bottom-up and top-down mechanisms. Basic associative learning makes stimuli that are stably connected to rewards more salient already at ‘low’ levels of perception and cognition (Jiang et al., Citation2015), while top-down reward expectations lead us to selectively attend to aspects of a situation linked to the reward – be it because this improves our chances of attaining the reward, or because we anticipatingly savor the expected reward (Gottlieb & Oudeyer, Citation2018).
Video games routinely reward players for attaining game goals or tasks or making partial progress toward them (Johnson et al., Citation2018; Schell, Citation2008, pp. 188–191). This spans enjoyable, “juicy” success feedback; praise from virtual others; new virtual currency, items, or buffs; or unlocked new content or story progression. These video game rewards should direct player attention to information relevant for likely rewarded tasks. Formally expressed, task-attention theory predicts that task-contingent rewards will lead to increased sampling of and attentional selection onto task-relevant information.
This differs from non-interactive media where audiences may build up general expectations that a media activity (watching TV) or offering (watching The Big Bang Theory) holds experiential rewards (enjoyment), which may lead them to actively sample and focus attention on these activities and offerings as a whole. Yet non-interactive media do not articulate specific tasks that focus attention on specific information within the media offering and make rewards task-contingent. (An exception to this rule are Easter eggs and in-jokes, such as spotting Stan Lee cameo appearances in Marvel Cinematic Universe movies.)
4.2. Task-based attentional demands
Mechanics, goals, uncertainty, and rewards are structural game features that more or less directly steer attentional selection and sampling. In addition to these, task-attention theory recognizes a number of task-based demands, which moderate attentional selection: perceptual load, cognitive load, and time and performance pressure.
Broadly speaking, pursuing game tasks puts various demands on users, including the cognitive demands of performing cognitive skills and making decisions (Bowman, Citation2018). This construct of “cognitive demands” mirrors the “cognitive load” construct in LC4MP, which refers to the cognitive resources required to process a media message (Lang, Citation2000). This, in turn, mirrors the eponymous “cognitive load” construct in CLT (Sweller et al., Citation2011). Even more confusingly, they both mirror the “cognitive load” construct in Load Theory, developed in psychological attention research (Lavie et al., Citation2004; Murphy et al., Citation2016). Yet to our knowledge, the LC4MP, CLT, and Load Theory literatures have not taken notice of each other.
Task-attention theory focuses on the attentional sampling and selection of information, which logically precedes how selected information splits into extraneous, intrinsic, and germane load (following CLT), or how much processing capacity selected information leaves for encoding, storage, and retrieval (following LC4MP). We do not disagree with the by-and-large well-evidenced effects modeled in CLT and LC4MP. For the sake of parsimony, our model acknowledges but brackets these relations (see 4.4 below). Instead, task-attention theory expressly models the relations between loads or demands and attentional selection, which have been well-studied in attentional Load Theory. In fact, Load Theory distinguishes two kinds of load – perceptual load and cognitive load – that impact attentional selection at different stages and with opposite effect. In addition to these two, there is evidence that time and performance pressure experienced in the pursuit of a task similarly affect attentional selection in contradictory ways. Notably, whereas mechanics, goals, rewards, and uncertainty all focus attention on task-relevant information, perceptual and cognitive load and pressure can all be described as modulating attentional selection of task-irrelevant information or distractors (extraneous load in CLT terms). In line with CLT and LC4MP, task-attention theory posits that the more task-irrelevant information gets selected for processing, the less processing capacity remains for task-relevant information, impeding its learning.
4.2.1. Perceptual load
Video games often require players to process and filter complex and fast-changing audio-visual displays, such as tracking enemies in shooter games or scanning a scene for particular items in hidden object games. Such tasks put so-called perceptual load on our perceptual system (Lavie et al., Citation2004). Perceptual load increases with the number of items to be identified and tracked, the number of perceptual operations (such as rotation) required to identify each item, and the effort required by each perceptual operation. Research shows that increasing the perceptual load of a task reduces distraction by non-task-related stimuli, as people have fewer resources available for them (Lavie et al., Citation2004; Murphy et al., Citation2016). Hence, high perceptual load has been found to increase inattentional blindness (Cartwright-Finch & Lavie, Citation2007; Murphy & Greene, Citation2016). Perceptual load can thus be seen as an early, bottom-up, more or less automatized form of attentional selection, where stimulus features of the task-relevant information ‘crowd out’ distractors. That is, contrary to the belief that the “busy” or “hectic” screens of real-time games like bullet hell shooters are highly distracting, once a player has tuned themselves into the task of tracking relevant items, it absorbs their attention and prevents distraction (M. Johnson, Citation2016). Formally, task-attention theory predicts that greater task-related perceptual load will lead to less attentional selection of task-irrelevant information.
4.2.2. Cognitive load
Where the perceptual load of a task is low, we have remaining perceptual capacity that can be attracted by irrelevant information: we are open to distraction. In video games, these may be games that involve less visual tracking, are slow, information-sparse, and/or perceptually well-encoded.
In such situations, we need to use top-down or executive attentional control to filter out distractors. This requires limited executive control resources, which include working memory. Thus, the more cognitive load is put on our executive control resources, the less resources remain for top-down attentional control, and the more distractable we become. In line with this theoretical prediction, Load Theory research has found that when perceptual load is low and people are put under high cognitive load (e.g., asking them to hold a string of numbers in mind), people are more prone to process and remember task-irrelevant distractors (Lavie et al., Citation2004; Murphy et al., Citation2016).
This leads to the puzzling conclusion that if games involve high cognitive load (e.g., complex strategic decision-making), they should make players more distractable. We say “puzzling” because flow theory argues in straight contradiction that tasks requiring our full cognitive capacities minimize cognitive processing of non-task-related information (Csikszentmihalyi, Citation1990). In fact, there is some evidence that under some circumstances, increased cognitive load reduces distraction (Lleras et al., Citation2017). One possible explanation is that flow theory fails to distinguish perceptual and cognitive load: standard Load Theory would argue that the distraction-blocking absorption flow theory observes holds, but only for perceptual load.
Another explanation would be that Load Theory only applies for uninteresting and unenjoyable tasks, which most visual attention experiments arguably classify as. Where a task affords ‘spontaneous’ interest because it is associated with extrinsic or intrinsic rewards, such as uncertainty reduction, these bottom-up mechanisms may suffice to keep attention “automatically” on task, no executive control required. Neutral or aversive tasks in contrast might require effortful executive control to direct attention away from more attractive stimuli (for recent evidence in support of this, see, (C. S. Deterding, Citation2019); Eden et al. (Citation2018)). Thus, high cognitive load in video game play would only impede attentional selection away from irrelevant information where (a) perceptual load is low and (b) the task at hand is boring, aversive, or associated with less reward than perceivable alternatives. Put formally, task-attention theory predicts that greater task-related cognitive load will lead to less top-down attentional suppression of task-irrelevant information under conditions of low task-related perceptual load, reward, and uncertainty.
How does this play out in non-interactive media? LC4MP research similarly discusses fast-cut movies in terms of load (Lang, Citation2000). However, it ignores active sampling, and treats perceptual load as information density, assuming that it decreases attention when information density exceeds processing capacity and people disengage because they cannot “keep up”. When people leisurely consume non-interactive entertainment media that unfold at a fixed pace, this may be globally accurate: a TV watcher may typically just indiscriminately process “the whole scene” of an anime instead of, e.g., focussing visual attention on scene transitions to count continuity errors. Interactive games, in contrast, suggest or impose such specific visual search tasks on users via their goal structures, and the information displayed directly depends on the choices players make in pursuing such tasks. If cuts are too fast (i.e., perceptual load is high), the anime watcher may stop trying to comprehend each sequence. Similar high perceptual load in video games is often a deliberate part of the core challenge of a visual search task, driving player to double down on attentional selection (cf., M. Johnson, Citation2016).
4.2.3. Pressure
In demanding skilled task performance with uncertain success, video game play often creates a feeling of being “under pressure.” This can heighten arousal (e.g., Bowman, Citation2010; McGloin et al., Citation2016), which is often an intended and desirable effect: following mood management theory, people aim for an optimum medium arousal level (Reinecke, Citation2017). If their arousal is low, they may play high-pressure action games to raise their arousal level. Importantly for our context, arousal has also been shown to narrow attentional focus (Bacon, Citation1974; Gardony et al., Citation2011).
While “feeling pressured” is an everyday phrase, there is no matching unitary “pressure” construct in psychology. Pressure is usually discussed under two headers (Caviola et al., Citation2017): time pressure, where people are given limited time to perform a task or decision (Caviola et al., Citation2017; Ordóñez et al., Citation2015), and performance pressure, also called social pressure: situations where people value optimal performance highly because it is tied to social evaluations of one’s self or high stakes (like prizes or admissions; Caviola et al., Citation2017; DeCaro et al., Citation2011). Time and performance pressure affect performance in complex ways that are not fully settled – different levels of pressure under different conditions are seen to variously enhance or impede performance. Be that as it may, games use a variety of mechanisms that tie into both, and there is evidence that each of these affects attention.
Time pressure leads individuals to adaptively select decision-making and action strategies (like ‘fast and frugal’ heuristics) that maximize accuracy for the effort available; part of these strategies is to selectively focus attention on the most likely task-relevant information (Ordóñez et al., Citation2015, p. 522). Video games often induce time pressure around tasks by giving players limited time to decide or act (e.g., quick-time events or timed decisions in dialogs). In the so-called “real-time” games, like real-time strategy games and many sports and shooter games, the time pressure is constant as players need to continually monitor and respond to their (virtual or real) opponents’ moves (Zagal & Mateas, Citation2010).
High-performance pressure is commonly found to impede effective attentional control and, with it, performance, leading to so-called “choking under pressure” (Caviola et al., Citation2017). This is explained by two mechanisms: one, performance pressure leads people to generate worrying thoughts, which leave less working memory for performing the task; two, people pay conscious attention to otherwise automatically performed tasks, which interferes with such tacit skill performance (Caviola et al., Citation2017; DeCaro et al., Citation2011). In contrast, Normand et al. (Citation2014) found that performance pressure also leads people to exert more top-down attentional control to focus on task-relevant features. Thus, performance pressure may paradoxically increase attentional selection onto both task-relevant and task-irrelevant (worries) information at once.
Especially competitive multi-player games raise the performance stakes and pressures for playersin particular, within contexts and communities (tournaments, leagues, Esports, ‘hardcore’ gamers, streaming) where skilled performance is highly prized, tied to a player’s identity and self-worth, and on public display (Deterding, Citation2013; Poels et al., Citation2012; Taylor, Citation2012). Many gamified experiences and single-player games feature design elements like leader boards that afford public performance evaluation, which have been shown to induce performance-impeding worries in some (Christy & Fox, Citation2014).
In leisurely non-interactive media consumption, there are few if any time and performance pressures. This changes if media consumption is made part of an instrumental context like high-stakes educational testing: much of the literature on pressure is fact in on such assessment. These pressures, however, derive from the structure of the assessment, not of the media offering.
In sum, many game genres and mechanics afford time and performance pressure, which can narrow selective attention on task-relevant features through heightened arousal and top-down attentional control. Formally, greater perceived pressure leads to greater attentional selection on task-relevant information. That said, pressure can also reduce overall available attentional resources by inducing worrying thoughts or directing conscious attentional focus on otherwise tacit, automatic performance. Data on this “choking under pressure” are contradictory, suggesting hidden moderators or non-monotonic relations. Task-attention theory therefore leaves this relation as an unspecified possibility: Greater perceived pressure can lead to performance worries and attending to automated skill performance, which manifest as selecting task-irrelevant information.
4.3. Non-task-based attentional mechanisms
Task-attention theory acknowledges that games and interactive media can and often do feature the attentional mechanisms and connected design features already studied in non-interactive media like movies, TV, novels, or textbooks. This is the systematic ‘place’ for findings connected to LC4MP, CLT, MMT, and CTGBL. To keep our already-complex model concise, we do not expand on these detail features and mechanisms. Our model intentionally abstracts these away into a single construct, “Non-Task-Based Attentional Mechanisms” (E). Thus, task-attention theory formally predicts that non-task-based attentional mechanisms will affect learning.
5. Discussion: implications and future work
The shared theoretical ground of CLT, MMT, CTGBL, and LC4MP is that media offerings carry different kinds and densities of information that meet a fixed human processing capacity. Too much (irrelevant) information, and people will learn the desired information less well or not at all. From there, LC4MP, MMT, and CTGBL go on to posit that attentional processes determine the depth of processing of different elements of information presented in a media offering. However, despite claims to the contrary, they still conceive the user as a mostly passive receiver: media features like signaling or OESFs determine what gets selected. The derived design principles therefore all revolve around minimizing extraneous or distracting information, portioning relevant information to fit processing capacity, and making relevant information salient.
Task-attention theory does not disagree with these points; rather, it extends them in three major ways. First, it argues that active sampling and attentional selection moderate what information becomes available for processing already at enactive and perceptual levels, preceding the cognitive processes described in prior theories. Prior theories do not acknowledge attentional processes at these ‘early’ phases in the perception-action loop. Second, sampling and selection are partially afforded by media features, but they are also driven by learned dispositions (attentional sets) and active, ‘top-down’ attentional control. This active user role is absent in current CLT-based theories and minimally developed in LC4MP research. Third, performing tasks involves distinct attention-directing processes. Games (and other interactive media) afford and require ongoing task performance and thus invoke these distinct mechanisms that are not similarly invoked in consuming non-interactive media like television or books. This implies that attentional direction in games and interactive media is likely different and stronger in moderating learning compared to non-interactive media.
These three extensions together hold major implications and point to important future research. The following sections will address these implications and possible future work for (5.1) game-based learning research, (5.2) game-based learning design, and (5.3) HCI, learning, and media effects research. This is followed by reflections on a major counterpoint we see for task-attention theory: the roles of disattention, reflection, and attentional breadth for learning (5.4).
5.1. Game-based learning research
The first and foremost area of future research is the severe testing of task-attention theory itself. Its main proposition––learning in games is moderated by attention––has some empirical validation (such as Cutting et al., Citation2020). Similarly, the proposed mechanisms underlying this main effect, specified in formal predictions (see, ), have all been developed from prior empirical work, and are plausible within existing, empirically supported models of active sampling and attentional selection. That said, these formal predictions have not been directly empirically tested. This points to an immediate series of empirical studies as low-hanging fruit which would both test the theory and pave the way for use in real-world applications, as unpacked in the following subsections.
5.1.1. Attention as a moderator could explain heterogeneous findings in game learning
We started this paper with the observation that existing research on game learning shows heterogeneous results. Recent work has found that game task design strongly shapes covert attention and memory: Players only attended to and recalled particular task-relevant features of a game element such as color or direction whilst completely ignoring other features such as the image used to represent the element (Cutting et al., Citation2020). This aligns with eye tracking studies that have found players direct overt attention (like their gaze) only on those areas needed for performing in-game tasks (Moreira & Okimoto, Citation2018; Sundstedt et al., Citation2008), even in slow moving “self-paced” games (Cutting & Cairns, Citation2020). Players’ overall attentional focus is lessened if players becomes less engaged by the game (Cutting & Cairns, Citation2020; Jennett, Citation2010).
Studies like these prompted us to develop task-attention theory and formalize their implication that task-based attentional direction is a key moderator of learning. Since most game-based learning research to date has not controlled for task-based attentional direction, this moderator could go a long way in explaining prior conflicting results, and why different learning games show different learning effects. Thus, studying the moderating effect of task-based attention on learning is a major area of future work.
5.1.2. Identifying game features that direct attention
A further area of future work is identifying which game design features direct attention. The features we have set out in this paper are mainly derived from existing research on attentional selection that uses abstract tasks unrelated to real games, and measures attention for very short periods of time, typically, a fraction of a second. It is possible that these findings may not generalize to games: future studies should determine whether the features we identified actually produce meaningful effects in games.
Furthermore, it is unlikely that prior literature has identified all major ways in which game design steers player attention. Game designers already acknowledge and design for attention in many areas. Take “mini-maps,” small dynamic maps shown on top of the main game graphics that inform players about what part of a larger territory they presently see and manipulate. Many designers report the unwelcome side-effect that players traversing a game world often focus their attention solely on the map instead of the lushly rendered main environment display, because the map provides the clearest display of the information needed for the task of movement (Honcharuk, Citation2017; Khan & Rahman, Citation2018). Moreover, the visual surface design of games is geared to support task performance: Graphic artists and interface designers make characters and manipulable game objects “readable” by giving them simple, distinct, and consistent shapes and colors, which signal their function within the game task (Gunardi Teguh, Citation2018; Solarski, Citation2013). This lets players perform game tasks more efficiently by focusing their attention just on these simple features, ignoring more complex design details.
As these examples show, mechanics, goals, rewards, and uncertainty are but some of the many different features game designers already regularly use to steer player attention. Such features may feed into similarly attention-directing global gameplay dynamics – emergent patterns of run-time behavior of the total player-game system, as conceptualized by (Hunicke et al., Citation2004). A fruitful area for future research would therefore be bottom-up, qualitative work identifying these other features and dynamics that games and their designers use to steer attention.
5.1.3. Validating moderating effects of attention on game learning
The third major area for future work are empirical studies on whether these different game features create differences in active sampling and attentional selection which then moderate learning effects. One approach would be to reproduce existing studies on game learning effects whilst considering the moderating effect of attention. Specifically, the attention-directing features and demands identified in task-attention theory could be modified in existing learning games to direct attention toward or away from particular information, or increase or decrease resources available for top-down attentional control, to see whether these manipulations affect learning outcomes. One important methodological caveat here is that many if not most features and demands identified in task-attention theory are likely multifunctional, affecting learning through multiple pathways. For instance, rewards likely focus attention and evoke emotional responses that facilitate encoding. Thus, studies testing the effects of attention-directing features should capture and control for other likely candidate moderators and mediators.
5.1.4. Advancing methods for attention measurement
Fourth, measuring attentional selection in games is still in its infancy. Overt attention has been measured using eye tracking (Johansen et al., Citation2008; Sundstedt et al., Citation2008). Covert attentional selection is more difficult to study; most methods are based on measuring players’ post-game retention of information from the game. Thus, Jennett (Citation2010) measured the strength of attentional selection onto the game using a post-game test of recall of irrelevant audio clips played during the game. Similarly, Chung and Sparks (Citation2016) used a “signal detection” approach and tested players’ recognition of adverts shown in the game. Cutting and Cairns (Citation2020) broadened this approach to create a general measure of attentional selection known as the Distractor Recognition Paradigm. They later used this technique to show that varying the goals of a simple puzzle game created sustained attentional selection away from task-irrelevant visual features (Cutting et al., Citation2020). Advancing methods for measuring different forms of attention and how they moderate learning would be highly valuable future work.
5.2. Game-based learning design
Going back to early work by Malone (Citation1981), one of the most enduring (and well-supported) design principles for game-based learning is that the information to be learned needs to be intrinsic or endogenous to the game (Deterding, Citation2015; Echeverría et al., Citation2012; Habgood & Ainsworth, Citation2011; Squire, Citation2006). Malone (Citation1981) originally understood this as “intrinsic fantasy”: learning is more effective and engaging when the fiction of the game provides an informative, fitting metaphor for the taught concept (e.g., firing a ballista on a castle is an intrinsic fantasy for calculating projectile motion). Later work has extended this intrinsic integration principle to mean integrating learning material into “the core mechanics of the gameplay” (Habgood & Ainsworth, Citation2011, p. 173).
There is some evidence that more intrinsically integrated learning games provide better learning outcomes (Echeverría et al., Citation2012; Habgood & Ainsworth, Citation2011). Nevertheless, why and how intrinsic integration exactly works better remains an open question. Summarizing prior speculations, Habgood and Ainsworth (Citation2011) suggest that intrinsic integration may work because players’ attention is attracted by those elements that are most fun and important to gameplay, hence players attend to (and thus, learn) whatever information is embedded in the core fun gameplay – a logic akin to the proposed functioning of OESFs in LC4MP. However, this has remained untested speculation with no clearly specified mechanism.
Task-attention theory provides a clearly specified and testable mechanism explaining intrinsic integration: “Core mechanics” (Habgood & Ainsworth, Citation2011, p. 173) articulate the particular tasks a player has to do over and over again in a game, which usually come with varied goals whose attainment is rewarded but uncertain. All these features direct attentional selection and sampling toward task-relevant information. As they learn to play, players build up core-task-related mental models and perceptual encodings which increasingly guide future sampling and selection toward information relevant for task performance or improvement of the attentional set. Learning to play a game to some extent is learning to identify and focus on task-relevant information. From a task-attention theory perspective, then, intrinsic integration is nothing other than task relevance. The more task-relevant information is in a game and the more frequently the given task is performed, the more likely players will (learn to) elicit and attend to it and build up lasting schemata representing that information. Experimentally testing this explanation is one immediate fruitful area for future work.
Next to intrinsic integration, another common high-level design principle for entertainment and learning games is game balance, especially balanced challenges whose difficulty matches and sequentially grows with growing player skill (Schreiber & Romero, Citation2021). Flow theory argues that such balanced challenges are engaging because they fully absorb player attention and don’t induce boredom or anxiety (Csikszentmihalyi, Citation1990). Socio-cultural learning theories following Vygotsky hold that they keep learners in a learning-optimal zone of proximal development, where learners can just about accomplish a task with social guidance and thereby internalize that guidance as new knowledge (Kiili, Citation2005, Citation2007). CLT proponents argue that well-balanced challenges sequence and portion information so that the to-be-learned schemata have existing learned schemata to build on and processing and storing them doesn’t exceed working memory capacity. Task-attention theory adds two congruent aspects worthy of future inquiry here: one, what is learned and needs to be balanced for includes attentional sets. Two, balance will affect task-based demands, where too-great perceived pressure and cognitive load will impede players’ ability to focus and select task-relevant information, and too little perceptual load leaves attentional capacity free to select task-irrelevant information.
A broader implication of task-attention theory for learning game design is that games direct attention not just with ‘surface’ features (emotional imagery, highlighting), but also with structural features and dynamics like mechanics, goals, rewards, and uncertainty. These are already key building blocks and analytic lenses (learning) game designers work with, but usually only with a view to motivation and engagement (Whitton, Citation2014, pp. 67–108). Task-attention theory adds that the very same structural features also function on an attentional level. However, these structural features are articulated at a level of abstraction that is likely too unspecific to usefully guide design decisions (Hekler et al., Citation2013). Future work should therefore identify and test derived “intermediate” forms of knowledge (Löwgren, Citation2013) like lenses or patterns that are more practically useful and support knowledge transfer between research and practice.
5.3. Implications for HCI, learning, and media effects research
5.3.1. Active sampling and attentional selection as learning moderators
While task-attention theory focuses on game-based learning, the major mechanisms it identifies – active sampling, attentional selection, attentional sets – are basic building blocks of human action, perception, and cognition. Therefore, they should moderate learning in any learning task, regardless of the learning medium. Mechanisms like active sampling may manifest less in highly controlled and pre-structured learning environments, as can be found in much formal schooling or controlled experiments in educational research. The more we move into informal learning in open-ended learning environments, the more impactful such attentional direction actively flowing from the learner will arguably be. One upshot of task-attention theory for learning research is thus to study when and how active sampling and attentional direction moderate learning more generally.
A second upshot concerns learning and interactive media. CLT-informed learning theories like MMT have chiefly considered interactive media as multi-media that can deliver information on parallel (auditory and visual) sensory channels. Task-attention theory suggests that an equally if not more important differentiator is that interactive media are task-based, activating distinct, strong forms of attentional selection and active sampling. Many of the attention-directing features found in games (mechanics, goals, rewards, and uncertainty) are not necessarily found across all interactive media. Future research should thus explore what other features may steer task-based attention in interactive media more widely.
5.3.2. Attentional sets as learning outcomes
One marked downside of attentionally controlled and pre-structured learning environments in experiments or formal schooling is that they are likely not very ecologically valid, which may limit transfer of learning, but more importantly, fail to actually develop key competencies. Arguably, everyday work environments rarely present task-relevant information as foregrounded, apportioned, and distraction-free as textbooks and other learning materials, especially if the latter are designed following CLT principles. ‘In the wild,’ people need to actively elicit, identify, and focus attention on relevant information in a blooming, ambiguous, uncertain, and distraction-rich work environment. Part of skilled task performance is to engage in judicious active sampling and attentional selection, for which people need to develop task-related professional vision or attentional sets. Pre-structured, non-interactive learning environments likely don’t require and therefore won’t train these competencies, while digital games and ‘authentic’ problem-based learning contexts arguably develop them as a matter of course. This nicely qualifies the debate between proponents of constructivist learning versus direct instruction (Kirschner et al., Citation2006). Working through a problem in a rich, not fully pre-structured environment, as experiential, problem-based game learning suggests (Kiili, Citation2005, Citation2007), is necessary to train up the attentional sets for performing similar tasks in similarly poorly structured real-world contexts. But, in line with direct instruction arguments, this training can arguably be facilitated and sped up with initial guidance (like highlighting) that directs attention to the right information, to then gradually remove such guidance. We already find this in the onboarding of especially casual games, which often offers a highly pre-structured first run-through of the core mechanics, with plenty of audio-visual highlighting of task-relevant objects and information. Either way, we see valuable future research in studying, assessing, and supporting attentional sets as an important learning outcome.
5.3.3. Distinguishing perceptual and cognitive load
One surprising discovery of our review was that learning theory (CLT), media effects research (LC4MP), and attention research (Load Theory) each feature “cognitive load” as a core construct, yet with different intensions, extensions, and connected observed effects. While these different renderings broadly concur, Load Theory in attention research makes a crucial (and well-evidenced) distinction between perceptual and cognitive load, with opposite attentional effects. We haven’t found similar differentiations in learning and media effects research, but there is nothing in the evidence to suggest that it should not generalize to these domains. Thus, one specific area of future research is exploring whether perceptual load similarly increases attentional focus in media consumption and learning, and whether this differentiation may help explain conflicting findings in both fields.
5.3.4. Media effects beyond learning
Task-attention theory intentionally focuses on learning. However, many other media effects – like persuasion, stereotype formation, or aggression – are de facto learning processes. Hence, it is plausible that active sampling and attentional selection will also moderate these other effects, in games and other media. Task-attention theory suggests that beyond the content of a game or a media offering (such as violent imagery or scripts, stereotyped representations, or persuasive messages), we need to consider the task that the user is performing. Depending on the nature and difficulty of the task, it is likely that users will just pay attention to a narrow set of features that the task directs their attention to and ignore everything else.
Consider the debate on violent content in video games and aggression. Most studies in the area only consider violence depictions in a game, rather than the tasks that players pursue, and whether these tasks select attention onto the violent content. While playing a first-person shooter, such as Doom (Id Software, Citation1993), players may completely ignore the graphic content of the game, instead selecting attention onto the abstract information needed to avoid bullets and clear the level of enemies, namely enemy, crosshair, and obstacle locations. Breuer et al. (Citation2014) recently tested this line of reasoning and found that participants who played a violent video game perceived more violence than those watching a gameplay video, suggesting an attentional selection effect, if in the opposite direction. They also found that players with greater play experience perceived less violence, which they explained as desensitization. Task-attention theory offers an alternative explanation that more experienced players are better at perceptually encoding and selecting task-relevant information and thus, filtering out task-irrelevant detailed depictions of violence.
While an extended review is beyond the scope of this paper, many persuasion theories informing persuasive games (like the elaboration likelihood model) present attentional focus as an important moderator of persuasion effects. Thus, Jacobs (Citation2017) recently posited that persuasive games work best if they focus full player attention on the game’s strong procedural argument, or leave little attentional resource for elaboration if the game features weak procedural arguments. While he did not find the predicted effects, his work points the way for future task-attention theory-inspired research on general media effects, especially for interactive media.
5.3.5. Broader HCI implications
Current HCI work on attention generally focuses on overt spatial attention as measured by eye tracking or logged inputs, with selection usually considered to be directed by visual saliency, task goals, or expected utility. Task-attention theory extends this to highlight the importance of covert and feature-based selection which cannot be measured using eye tracking. Task-attention theory also introduces a wider range of factors, which may direct attentions such as goals, rewards, mechanics, uncertainty, or task demands. Some of these could be re-expressed in existing HCI models as information foraging (Pirolli, Citation2005). Importantly, HCI often takes aspects like goals and rewards as givens that the user and their context bring to an interface. In contrast, approaches like gamification or gameful design (Deterding, Citation2015) suggest that these aspects can be deliberately designed – in our case, designed to guide attention. As we argued earlier, HCI research has focused on designing for and modeling attention as part of instrumental goal pursuit, not as a moderator of learning. As the features of games that task-attention theory articulates also focus attention in interactive media more broadly, there is no reason why the theory shouldn’t also extend to learning in interactive systems. Thus, task-attention theory might offer a fruitful starting point for general HCI work on designing tutorials, onboarding, and interactive learning environments more generally.
5.4. The value of attentional breadth, disattention, and reflection
Task-attention theory articulates mechanisms in gameplay that drive learning by directing and focussing attention on to-be-learned information during gameplay. However, a range of theories and observations suggest that more focused attention is not always better for learning and that gameplay is not all that matters for learning.
First, a long-standing saying in simulation and gaming is that “debriefing is where the learning happens” (Lederman, Citation1992). Particularly in authentic, rich, embodied, high-pressure role-play, and simulation as found in, e.g., military or medical training, participants’ attention is so absorbed in the doing that little cognitive and attentional resources remain for reflection and deliberation. The latter, however, are crucial processes for learning from failures (by analyzing how failures happened and what one might do instead) as well as developing and connecting more explicit forms of knowledge (words, models, etc.) to the just-lived experience (Fanning & Gaba, Citation2007). Thus, to afford actual learning from simulations and role-play requires debriefing where a facilitator structures reflection and deliberation on gameplay. This is explicitly recognized in (Kiili, Citation2005, Citation2007) experiential gaming model. Task-attention theory does not extend to post-play debriefing, but it explains and predicts what information players attend to while playing that then becomes material for it.
Second, several games are intentionally designed to break unconscious attentive absorption in gameplay and broaden attention by, e.g., breaking with game mechanical or interface conventions (Whitby et al., Citation2019). Such reflective game design (Boyd & Fales, Citation1983; Khaled, Citation2018) often follows modernist esthetics like Brechtian theater that try to shake people out of the unthinking acceptance of their social world as it is, instead provoking critical questioning and reflection (Mekler et al., Citation2018). Maximizing attentional focus on task-relevant information is anathema to this kind of critical learning approach and outcome. However, in articulating how games focus on player attention, task-attention theory provides pointers for breaking or reducing focused attention – another possible area for future work.
Third and finally, in the context or games for attitude and belief change, Kaufman et al. (Citation2015) have highlighted that games with a very overt agenda can trigger reactance – players reasserting threatened autonomy by rejecting the persuasion attempt and reinforcing their existing beliefs and attitudes. In those situations, Kaufman and colleagues suggest taking a ‘stealthy’ route and not directing player attention overtly on the game’s main message. They suggest a range of design strategies for this. Here, task-attention theory might be useful both for inspiring new design strategies for ‘stealth’ learning and for modeling and testing whether and how such stealth strategies work.
6. Conclusion
Task-attention theory synthesizes existing models of attention, media effects, learning, and game design to propose that task-based attentional selection and sampling is an important moderator of learning in video games. As such, it offers an explanation for the mixed effectiveness of current game-based learning. Games research has long recognized that games hold players’ attention onto the game. HCI, media effects, and learning research acknowledge the importance of attentional selection within a media offering. Task-attention theory builds on and goes beyond these approaches. All media activate attentional mechanisms, but games as interactive media afford and demand performing tasks, which activates a different set of attentional mechanisms, namely task-based active sampling and attentional selection that train up and are steered by a task-related attentional set, which precede many of the attentional mechanisms modeled in media effects and learning theories. Game designers have long considered game tasks in terms of structural features such as goals, mechanics, rewards and uncertainty. Task-attention theory suggests that these features also direct attentional sampling and selection in different ways. This contrasts with existing approaches to game-based learning, which chiefly consider surface features such as graphics and animations as steering attention. As one result, task-attention theory offers a compelling attentional explanation of the well-established game-based learning principle of intrinsic integration: for learning content to be intrinsically integrated into a game is for it to be relevant to the task the game asks players to perform. Game tasks also place different types of demands on players, such as perceptual load, cognitive load, or pressure. Where prior theories have modeled how these demands affect available resources for processing information, task-attention theory unpacks how demands affect the attentional sampling and selection processes that precede processing.
Moving to wider implications, other media effects, such as persuasion, stereotyping, or the promotion of violence can be seen as forms of learning and as such have been linked to attentional processes. Task-attention theory invites us to consider how these learning effects may be similarly moderated by attentional selection and active sampling, especially in interactive media like games. We have studied what players are looking at for a long time; task-attention theory may help us understand what they have been seeing.
Disclosure statement
No potential conflict of interest was reported by the author(s).
Additional information
Funding
Notes on contributors
Joe Cutting
Joe Cutting is a researcher based in the Digital Creativity Labs at the University of York, UK. His research is focused on how players experience digital games. He looks at topics such as engagement, attention, the effect of difficulty and the appeal of idle games. Before starting his academic career, he ran a successful digital agency creating innovative digital exhibits for major museums such the London Science Museum and the National Museum of Scotland.
Sebastian Deterding
Sebastian Deterding is Chair of Design Engineering at the Dyson School of Design Engineering at Imperial College London, academic liaison and former director of the EPSRC Centre for Doctoral Training in Intelligent Games and Game Intelligence, founding editor-in-chief of ACM Games: Research and Practice, and co-editor of The Gameful World (MIT Press, 2018). His work focuses on game-inspired and motivational design for human flourishing.
Notes
1 We bracket the active debate whether these processes are grounded in a unitary attention system or multiple different mechanisms – compare e.g., Petersen and Posner (Citation2012) with Hommel et al. (Citation2019)
2 Attention obviously also figures in meso- and macro-level media effects theories, but these levels of analysis are outside the scope of our theory.
3 As in any modern art form, there are exceptions that deliberately break a convention to explore its esthetic effects and invite reflection. “Zero-player games” ((Björk & Juul, Citation2012)and other games taking away player agency can be seen as such “avant-garde videogames” ((Schrank & Bolter, Citation2014) that prove the agency convention by challenging it.
4 There are many ways of conceptualizing uncertainty, e.g., information-theoretically as objective, actor-independent probability distributions over possible states or subjective feelings of not knowing or (lacking) confidence. We here take a probabilistic inference view (Gottlieb & Oudeyer, Citation2018) and conceptualize uncertainty as an actor’s internal distribution of probabilities over a set of alternative beliefs. E.g. if you hear a bump in the dark, uncertainty captures how likely you think the bump is caused by your cat versus a burglar – the fewer options you believe could be the case and the higher the probability you assign to any one option, the lower your uncertainty about what is the matter with the bump in the night.
References
- Aarseth, E. J. (1997). Cybertext: Perspectives on ergodic literature. JHU Press.
- Abt, C. C. (1970). Serious games. The Viking Press.
- Abuhamdeh, S., Csikszentmihalyi, M., & Jalal, B. (2015). Enjoying the possibility of defeat: Outcome uncertainty, suspense, and intrinsic motivation. Motivation and Emotion, 39(1), 1–10. https://doi.org/https://doi.org/10.1007/s11031-014-9425-2
- Acquah, E. O., & Katz, H. T. (2020). Digital game-based L2 learning outcomes for primary through high-school students: A systematic literature review. Computers & Education, 143, 103667. https://doi.org/https://doi.org/10.1016/j.compedu.2019.103667
- Anderson, B. A., Laurent, P. A., & Yantis, S. (2011). Value-driven attentional capture. Proceedings of the National Academy of Sciences, 108(25), 10367–10371. https://doi.org/https://doi.org/10.1073/pnas.1104047108
- Anderson, J. R., Matessa, M., & Lebiere, C. (1997). ACT-R: A theory of higher level cognition and its relation to visual attention. Human–Computer Interaction, 12(4), 439–462. https://doi.org/https://doi.org/10.1207/s15327051hci1204_5
- Anthropy, A., & Clark, N. (2014). A game design vocabulary: Exploring the foundational principles behind good game design. Pearson Education.
- Bacon, S. J. (1974). Arousal and the range of cue utilization. Journal of Experimental Psychology, 102(1), 81. https://doi.org/https://doi.org/10.1037/h0035690
- Bakker, S., Hausen, D., & Selker, T. (2016). Peripheral interaction: Challenges and opportunities for hci in the periphery of attention. Springer.
- Bandai Namco Entertainment. (1980). Pac-Man (Atari 2600) [Video Game]. Nintendo
- Bandura, A. (2001). Social cognitive theory of mass communication. Media Psychology, 3(3), 265–299. https://doi.org/https://doi.org/10.1207/S1532785XMEP0303_03
- Baron, R. M., & Kenny, D. A. (1986). The moderator–mediator variable distinction in social psychological research: Conceptual, strategic, and statistical considerations. Journal of Personality and Social Psychology, 51(6), 1173. https://doi.org/https://doi.org/10.1037/0022-3514.51.6.1173
- Bergstrom, J. R., & Schall, A. (2014). Eye tracking in user experience design. Elsevier.
- Björk, S., & Holopainen, J. (2006). Games and design patterns. In K. S., Tekinbas & E. Zimmerman (Eds.), The game design reader (pp. 410–437). MIT press.
- Björk, S., & Juul, J. (2012). Zero-player games or: What we talk about when we talk about players. Philosophy of computer games conference, Madrid. Game Philosophy Network (gamephilosophy.org).
- Bojko, A. (2013). Eye tracking the user experience: A practical guide to research. Rosenfeld Media.
- Bower, G. H., & Morrow, D. G. (1990). Mental models in narrative comprehension. Science, 247(4938), 44–48. https://doi.org/https://doi.org/10.1126/science.2403694
- Bowman, N. D. (2010). The effect of task demand on mood repair and selective exposure to video games. Michigan State University. Communication.
- Bowman, N. D. (2018). Video games: A medium that demands our attention. Routledge.
- Bowman, N. D., Wasserman, J., & Banks, J. (2018). Development of the video game demand scale. in video games: A medium that demands our attention. Routledge.
- Boyd, E. M., & Fales, A. W. (1983). Reflective learning: Key to learning from experience. Journal of Humanistic Psychology, 23(2), 99–117. https://doi.org/https://doi.org/10.1177/0022167883232011
- Breuer, J., Scharkow, M., & Quandt, T. (2014). Tunnel vision or desensitization? The effect of interactivity and frequency of use on the perception and evaluation of violence in digital games. Journal of Media Psychology: Theories, Methods, and Applications, 26(4), 176. https://doi.org/https://doi.org/10.1027/1864-1105/a000122
- Byrne, M. D., Anderson, J. R., Douglass, S., & Matessa, M. (1999). Eye tracking the visual search of click-down menus. Proceedings of CHI (Vol. 99, pp. 402–409).
- Calleja, G. (2011). In-game: From immersion to incorporation. MIT Press.
- Cardoso-Leite, P., Joessel, A., & Bavelier, D. (2020). In J. L., Plass, R. E., Mayer, B. D., Homer (Eds.), Handbook of Game-based Learning (pp. 437). MIT Press. https://mitpress.mit.edu/books/handbook-game-based-learning
- Carrasco, M. (2011). Visual attention: The past 25 years. Vision Research, 51(13), 1484–1525. https://doi.org/https://doi.org/10.1016/j.visres.2011.04.012
- Cartwright-Finch, U., & Lavie, N. (2007). The role of perceptual load in inattentional blindness. Cognition, 102(3), 321–340. https://doi.org/https://doi.org/10.1016/j.cognition.2006.01.002
- Case, A. (2015). Calm technology: Principles and patterns for non-intrusive design. O’Reilly Media, Inc.
- Caviola, S., Carey, E., Mammarella, I. C., & Szucs, D. (2017). Stress, time pressure, strategy selection and math anxiety in mathematics: A review of the literature. Frontiers in Psychology, 8, 1488. https://doi.org/https://doi.org/10.3389/fpsyg.2017.01488
- Christy, K. R., & Fox, J. (2014). Leaderboards in a virtual classroom: A test of stereotype threat and social comparison explanations for women’s math performance. Computers & Education, 78, 66–77. https://doi.org/https://doi.org/10.1016/j.compedu.2014.05.005
- Chun, M. M., Golomb, J. D., & Turk-Browne, N. B. (2011). A taxonomy of external and internal attention. Annual Review of Psychology, 62(1), 73–101. https://doi.org/https://doi.org/10.1146/annurev.psych.093008.100427
- Chung, S., & Sparks, J. V. (2016). Motivated processing of peripheral advertising information in video games. Communication Research, 43(4), 518–541. https://doi.org/https://doi.org/10.1177/0093650214566623
- Cleeremans, A., Destrebecqz, A., & Boyer, M. (1998). Implicit learning: News from the front. Trends in Cognitive Sciences, 2(10), 406–416. https://doi.org/https://doi.org/10.1016/S1364-6613(98)01232-7
- Coffee Stain Studios. (2014) Goat simulator (windows PC) [Video Game]. Coffee Stain Studios
- Costikyan, G. (2013). Uncertainty in games. MIT Press.
- Csikszentmihalyi, M. (1990). Flow: The psychology of optimal experience (Vol. 1990). Harper & Row.
- Csikszentmihalyi, M. (1991). Flow: The psychology of optimal experience (Vol. 41). HarperPerennial.
- Cutting, J., & Cairns, P. (2020). Investigating game attention using the distraction recognition paradigm. Behaviour & Information Technology, 1–21. https://doi.org/https://doi.org/10.1080/0144929X.2020.1849402
- Cutting, J., Cairns, P., & Kuhn, G. (2020). Nothing else matters: Video games create sustained attentional selection away from task-irrelevant features. Attention, Perception, & Psychophysics, 82(8), 3907–3919. https://doi.org/https://doi.org/10.3758/s13414-020-02122-y
- DeCaro, M. S., Thomas, R. D., Albert, N. B., & Beilock, S. L. (2011). Choking under pressure: Multiple routes to skill failure. Journal of Experimental Psychology: General, 140(3), 390. https://doi.org/https://doi.org/10.1037/a0023466
- Delatorre, P., León, C., Salguero, A., Palomo-Duarte, M., & Gervás, P. (2018). Confronting a paradox: A new perspective of the impact of uncertainty in suspense. Frontiers in Psychology, 9, 1392. https://doi.org/https://doi.org/10.3389/fpsyg.2018.01392
- Deterding, S. (2013). Modes of play: A frame analytic account of video game play. Staats-und Universitätsbibliothek Hamburg Carl von Ossietzky].
- Deterding, S. (2015). The lens of intrinsic skill atoms: A method for gameful design. Human–Computer Interaction, 30(3–4), 294–335. https://doi.org/https://doi.org/10.1080/07370024.2014.993471
- Deterding, C. S. (2019). Interaction tension: A sociological model of attention and emotion demands in video gaming. Media and Communication, 7(4), 226–236. https://doi.org/https://doi.org/10.17645/mac.v7i4.2366
- Dieterich, R., Endrass, T., & Kathmann, N. (2016). Uncertainty is associated with increased selective attention and sustained stimulus processing. Cognitive, Affective, & Behavioral Neuroscience, 16(3), 447–456. https://doi.org/https://doi.org/10.3758/s13415-016-0405-8
- Dijksterhuis, A., & Aarts, H. (2010). Goals, attention, and (un)consciousness. Annual Review of Psychology, 61(1), 467–490. https://doi.org/https://doi.org/10.1146/annurev.psych.093008.100445
- Djamasbi, S., Siegel, M., & Tullis, T. (2011). Visual hierarchy and viewing behavior: An eye tracking study. In International conference on human-computer interaction (pp. 331–340). Berlin, Heidelberg: Springer.
- Echeverría, A., Barrios, E., Nussbaum, M., Améstica, M., & Leclerc, S. (2012). The atomic intrinsic integration approach: A structured methodology for the design of games for the conceptual understanding of physics. Computers & Education, 59(2), 806–816. https://doi.org/https://doi.org/10.1016/j.compedu.2012.03.025
- Eden, A., Johnson, B. K., & Hartmann, T. (2018). Entertainment as a creature comfort: Self-control and selection of challenging media. Media Psychology, 21(3), 352–376. https://doi.org/https://doi.org/10.1080/15213269.2017.1345640
- Eitam, B., Glicksohn, A., Shoval, R., Cohen, A., Schul, Y., & Hassin, R. R. (2013). Relevance-based selectivity: The case of implicit learning. Journal of Experimental Psychology: Human Perception and Performance, 39(6), 1508. https://doi.org/https://doi.org/10.1037/a0033853
- Fanning, R. M., & Gaba, D. M. (2007). The role of debriefing in simulation-based learning. Simulation in Healthcare, 2(2), 115–125. https://doi.org/https://doi.org/10.1097/SIH.0b013e3180315539
- Fisher, J. T., Keene, J. R., Huskey, R., & Weber, R. (2018). The limited capacity model of motivated mediated message processing: Taking stock of the past. Annals of the International Communication Association, 42(4), 270–290. https://doi.org/https://doi.org/10.1080/23808985.2018.1534552
- Friston, K. J., Lin, M., Frith, C. D., Pezzulo, G., Hobson, J. A., & Ondobaka, S. (2017). Active inference, curiosity and insight. Neural Computation, 29(10), 2633–2683. https://doi.org/https://doi.org/10.1162/neco_a_00999
- Fu, W.-T., & Pirolli, P. (2007). SNIF-ACT: A cognitive model of user navigation on the world wide web. Human–Computer Interaction, 22(4),355–412. https://www.tandfonline.com/doi/full/https://doi.org/10.1080/07370020701638806
- Gan, X., Yao, Y., Liu, H., Zong, X., Cui, R., Qiu, N., Xie, J., Jiang, D., Ying, S., & Tang, X. (2020). Action real-time strategy gaming experience related to increased attentional resources: An attentional blink study. Frontiers in Human Neuroscience, 14, 101. https://doi.org/https://doi.org/10.3389/fnhum.2020.00101
- Gardony, A., Brunyé, T. T., Mahoney, C. R., & Taylor, H. A. (2011). Affective states influence spatial cue utilization during navigation. Presence: Teleoperators and Virtual Environments, 20(3), 223–240. https://doi.org/https://doi.org/10.1162/PRES_a_00046
- Goffman, E. (1961). Encounters: Two studies in the sociology of interaction. Ravenio Books.
- Gottlieb, J. (2018). Understanding active sampling strategies: Empirical approaches and implications for attention and decision research. Cortex, 102, 150–160. https://doi.org/https://doi.org/10.1016/j.cortex.2017.08.019
- Gottlieb, J., & Oudeyer, P.-Y. (2018). Towards a neuroscience of active sampling and curiosity. Nature Reviews Neuroscience, 19(12), 758–770. https://doi.org/https://doi.org/10.1038/s41583-018-0078-0
- Green, C. S., & Bavelier, D. (2012). Learning, attentional control, and action video games. Current Biology, 22(6), R197–R206. https://doi.org/https://doi.org/10.1016/j.cub.2012.02.012
- Gunardi Teguh, T. (2018). Character design: Shape language and readability. Medium, https://medium.com/@EightyLevel/character-design-shape-language-and-readability-6ee4bb6f98a6
- Habgood, M. J., & Ainsworth, S. E. (2011). Motivating children to learn effectively: Exploring the value of intrinsic integration in educational games. The Journal of the Learning Sciences, 20(2), 169–206. https://doi.org/https://doi.org/10.1080/10508406.2010.508029
- Halverson, T., & Hornof, A. J. (2011). A computational model of “active vision” for visual search in human–computer interaction. Human–Computer Interaction, 26(4), 285–314. https://doi.org/https://doi.org/10.1080/07370024.2011.625237
- Hekler, E. B., Klasnja, P., Froehlich, J. E., & Buman, M. P. (2013). Mind the theoretical gap: Interpreting, using, and developing behavioral theory in HCI research. In Proceedings of the SIGCHI Conference on Human Factors in Computing Systems (pp. 3307–3316).
- Hello Games. (2016) No man’s sky (windows PC) [Video Game]. Hello Games
- Hervet, G., Guérard, K., Tremblay, S., & Chtourou, M. S. (2011). Is banner blindness genuine? Eye tracking internet text advertising. Applied Cognitive Psychology, 25(5), 708–716. https://doi.org/https://doi.org/10.1002/acp.1742
- Hommel, B., Chapman, C. S., Cisek, P., Neyedli, H. F., Song, J.-H., & Welsh, T. N. (2019). No one knows what attention is. Attention, Perception, & Psychophysics, 81(7), 2288–2303. https://doi.org/https://doi.org/10.3758/s13414-019-01846-w
- Honcharuk, A. (2017). My personal crusade against mini-maps and other corner based HUD elements in immersive games. Game Developer. https://www.gamedeveloper.com/design/my-personal-crusade-against-mini-maps-and-other-corner-based-hud-elements-in-immersive-games-
- Hornof, A. J., & Halverson, T. (2003). Cognitive strategies and eye movements for searching hierarchical computer displays. Proceedings of the SIGCHI conference on human factors in computing systems (pp. 249–256).
- Hunicke, R., LeBlanc, M., & Zubek, R. (2004). MDA: A formal approach to game design and game research. Proceedings of the AAAI workshop on challenges in game AI (Vol. 4, No. 1. p.1722).
- Hussein, M. H., Ow, S. H., Cheong, L. S., Thong, M.-K., & Ebrahim, N. A. (2019). Effects of digital game-based learning on elementary science learning: A systematic review. IEEE Access, 7, 62465–62478. https://doi.org/https://doi.org/10.1109/ACCESS.2019.2916324
- Id Software. (1993) Doom (PC) [Video Game]. id Software
- Jacobs, R. (2017). Playing to win over: Validating persuasive games. Doctoral dissertation, Erasmus School of History, Culture and Communication (ESHCC).
- Jennett, C. (2010). Is game immersion just another form of selective attention? An empirical investigation of real world dissociation in computer game immersion. UCL (University College London)].
- Jiang, Y. V., Li, Z. S., & Remington, R. W. (2015). Modulation of spatial attention by goals, statistical learning, and monetary reward. Attention, Perception, & Psychophysics, 77(7), 2189–2206. https://doi.org/https://doi.org/10.3758/s13414-015-0952-z
- Johansen, S. A., Noergaard, M., & Rau, J. (2008). Can eye tracking boost usability evaluation of computer games. In Proceedings of CHI (Vol. 8).
- Johnson, M. (2016). Bullet hell: The globalized growth of danmaku games and the digital culture of high scores and world records. In A. Pulos & S.A., Lee (Eds.), Transnational contexts of culture, gender, class, and colonialism in play (pp. 17–42). Springer.
- Johnson, J. (2020). Designing with the mind in mind: Simple guide to understanding user interface design guidelines. Morgan Kaufmann.
- Johnson, D., Klarkowski, M., Vella, K., Phillips, C., McEwan, M., & Watling, C. N. (2018). Greater rewards in videogames lead to more presence, enjoyment and effort. Computers in Human Behavior, 87, 66–74. https://doi.org/https://doi.org/10.1016/j.chb.2018.05.025
- Jokinen, J. P., Sarcar, S., Oulasvirta, A., Silpasuwanchai, C., Wang, Z., & Ren, X. (2017). Modelling learning of new keyboard layouts. Proceedings of the 2017 CHI conference on human factors in computing systems. MIT press.
- Juul, J. (2005). Half-real. Video games between real rules and fictional worlds.
- Kafai, Y. B., & Burke, Q. (2015). Constructionist gaming: Understanding the benefits of making games for learning. Educational Psychologist, 50(4), 313–334. https://doi.org/https://doi.org/10.1080/00461520.2015.1124022
- Kaufman, G. F., Flanagan, M., & Seidman, M. (2015). Creating stealth game interventions for attitude and behavior change: An” embedded design” model. DiGRA Conference, 2( 3).
- Khaled, R. (2018). Questions over answers: Reflective game design. In D. Cermak-Sassenrath (Ed.), Playful disruption of digital media (pp. 3–27). Springer.
- Khan, N., & Rahman, A. U. (2018). Rethinking the mini-map: A navigational aid to support spatial learning in urban game environments. International Journal of Human–Computer Interaction, 34(12), 1135–1147. https://doi.org/https://doi.org/10.1080/10447318.2017.1418804
- Kidd, C., & Hayden, B. Y. (2015). The psychology and neuroscience of curiosity. Neuron, 88(3), 449–460. https://doi.org/https://doi.org/10.1016/j.neuron.2015.09.010
- Kidd, C., Piantadosi, S. T., & Aslin, R. N. (2012). The goldilocks effect: Human infants allocate attention to visual sequences that are neither too simple nor too complex. PloS one, 7(5), e36399. https://doi.org/https://doi.org/10.1371/journal.pone.0036399
- Kiili, K. (2005). Digital game-based learning: Towards an experiential gaming model. The Internet and Higher Education, 8(1), 13–24. https://doi.org/https://doi.org/10.1016/j.iheduc.2004.12.001
- Kiili, K. (2007). Foundation for problem‐based gaming. British Journal of Educational Technology, 38(3), 394–404. https://doi.org/https://doi.org/10.1111/j.1467-8535.2007.00704.x
- King. (2012) Candy crush saga (android) [Video Game]. King.com
- Kinoshita, S. (1995). The word frequency effect in recognition memory versus repetition priming. Memory & Cognition, 23(5), 569–580. https://doi.org/https://doi.org/10.3758/BF03197259
- Kirschner, P., Sweller, J., & Clark, R. E. (2006). Why unguided learning does not work: An analysis of the failure of discovery learning, problem-based learning, experiential learning and inquiry-based learning. Educational Psychologist, 41(2), 75–86. https://doi.org/https://doi.org/10.1207/s15326985ep4102_1
- Kiverstein, J., Miller, M., & Rietveld, E. (2019). The feeling of grip: Novelty, error dynamics, and the predictive brain. Synthese, 196(7), 2847–2869. https://doi.org/https://doi.org/10.1007/s11229-017-1583-9
- Koch, I., Poljac, E., Müller, H., & Kiesel, A. (2018). Cognitive structure, flexibility, and plasticity in human multitasking—An integrative review of dual-task and task-switching research. Psychological bulletin, 144(6), 557.
- Konami Digital Entertainment. (1981) Frogger (Atari 5200) [Video Game]. SEGA
- Kuhn, G., Teszka, R., Tenaw, N., & Kingstone, A. (2016). Don’t be fooled! Attentional responses to social cues in a face-to-face and video magic trick reveals greater top-down control for overt than covert attention. Cognition, 146, 136–142. https://doi.org/https://doi.org/10.1016/j.cognition.2015.08.005
- Kumari, S., Deterding, S., & Freeman, J. (2019). The role of uncertainty in moment-to-moment player motivation: A Grounded theory. Proceedings of the annual symposium on computer-human interaction in play. https://doi.org/https://doi.org/10.1145/3311350.3347148
- Lang, A. (2000). The limited capacity model of mediated message processing. Journal of Communication, 50(1), 46–70. https://doi.org/https://doi.org/10.1111/j.1460-2466.2000.tb02833.x
- Lang, A. (2006). Using the limited capacity model of motivated mediated message processing to design effective cancer communication messages. Journal of Communication, 56(suppl_1), S57–S80. https://doi.org/https://doi.org/10.1111/j.1460-2466.2006.00283.x
- Lavie, N., Hirst, A., De Fockert, J. W., & Viding, E. (2004). Load theory of selective attention and cognitive control. Journal of Experimental Psychology: General, 133(3), 339. https://doi.org/https://doi.org/10.1037/0096-3445.133.3.339
- Lederman, L. C. (1992). Debriefing: Toward a systematic assessment of theory and practice. Simulation & Gaming, 23(2), 145–160. https://doi.org/https://doi.org/10.1177/1046878192232003
- Leiva, L. A., Xue, Y., Bansal, A., Tavakoli, H. R., Köroðlu, T., Du, J., Dayama, N. R., & Oulasvirta, A. (2020). Understanding visual saliency in mobile user interfaces. 22nd International conference on human-computer interaction with mobile devices and services, (pp. 1–12). New York, NY, USA: Association for Computing Machiner.
- Linderoth, J. (2013). Beyond the digital divide: An ecological approach to gameplay. Transactions of the Digital Games Research Association, 1(1). https://doi.org/https://doi.org/10.26503/todigra.v1i1.9
- Linderoth, J., & Bennerstedt, U. (2007). This is not a door: An ecological approach to computer games. DiGRA Conference. http://www.digra.org/digital-library/publications/this-is-not-a-door-an-ecological-approach-to-computer-games/
- Lleras, A., Chu, H., & Buetti, S. (2017). Can we “apply” the findings of forster and lavie (2008)? On the generalizability of attentional capture effects under varying levels of perceptual load. Journal of Experimental Psychology: Applied, 23(2), 158. https://doi.org/https://doi.org/10.1037/xap0000116
- Locke, E. A., & Latham, G. P. (2002). Building a practically useful theory of goal setting and task motivation: A 35-year odyssey. American Psychologist, 57(9), 705. https://doi.org/https://doi.org/10.1037/0003-066X.57.9.705
- Löwgren, J. (2013). Annotated portfolios and other forms of intermediate-level knowledge. Interactions, 20(1), 30–34. https://doi.org/https://doi.org/10.1145/2405716.2405725
- Mack, A., & Rock, I. (1998). Inattentional blindness. MIT press.
- Majaranta, P., & Bulling, A. (2014). Eye tracking and eye-based human–computer interaction. In S. H., Fairclough & K. Gilleade(Eds.), Advances in physiological computing (pp. 39–65). Springer.
- Malaby, T. M. (2007). Beyond play: A new approach to games. Games and Culture, 2(2), 95–113. https://doi.org/https://doi.org/10.1177/1555412007299434
- Malone, T. W. (1981). Toward a theory of intrinsically motivating instruction. Cognitive Science, 5(4), 333–369. https://doi.org/https://doi.org/10.1207/s15516709cog0504_2
- Malone, T. W., & Lepper, M. R. (1987). Making learning fun: A taxonomy of intrinsic motivations for learning. In R. E. Snow, M. J. Farr (Eds.), Aptitude, learning, and instruction (pp. 223–254). Routledge.
- Martinez-Garza, M. M., & Clark, D. B. (2017). Two systems, two stances: A novel theoretical framework for model-based learning in digital games. In P. Wouters & H. Van Oostendorp (Eds.), Instructional techniques to facilitate learning and motivation of serious games (pp. 37–58). Springer.
- Mayer, R. E. (2009). Multimedia learning (2nd ed.). New York, NY: Cambridge University Press.
- Mayer, R. E. (2020). Cognitive foundations of game-based learning. In J. L. Plass, R. E. Mayer, & B. D. Homer (Eds.), Handbook of game-based learning (pp. 83–110). MIT Press.
- McGloin, R., Farrar, K. M., Krcmar, M., Park, S., & Fishlock, J. (2016). Modeling outcomes of violent video game play: Applying mental models and model matching to explain the relationship between user differences, game characteristics, enjoyment, and aggressive intentions. Computers in Human Behavior, 62, 442–451. https://doi.org/https://doi.org/10.1016/j.chb.2016.04.018
- Mekler, E. D., Iacovides, I., & Bopp, J. A. (2018). ” A game that makes you question … ” exploring the role of reflection for the player experience. Proceedings of the 2018 annual symposium on computer-human interaction in play (pp. 315–327). ACM.
- Milne-Ives, M., Lam, C., De Cock, C., Van Velthoven, M. H., & Meinert, E. (2020). Mobile apps for health behavior change in physical activity, diet, drug and alcohol use, and mental health: Systematic review. JMIR mHealth and uHealth, 8(3), e17046. https://doi.org/https://doi.org/10.2196/17046
- Mojang. (2009) Minecraft (windows PC) [Video Game]. Microsoft Studios
- Moreira, V., & Okimoto, M. L. (2018). The relation of attention between player profiles: A study on the eye-tracking and profile brainHex. International conference on applied human factors and ergonomics (pp.425–434). Springer, Cham.
- Most, S. B., & Astur, R. S. (2007). Feature-based attentional set as a cause of traffic accidents. Visual Cognition, 15(2), 125–132. https://doi.org/https://doi.org/10.1080/13506280600959316
- Murphy, G., & Greene, C. M. (2016). Perceptual load induces inattentional blindness in drivers. Applied Cognitive Psychology, 30(3), 479–483. https://doi.org/https://doi.org/10.1002/acp.3216
- Murphy, G., Groeger, J. A., & Greene, C. M. (2016). Twenty years of load theory—Where are we now, and where should we go next? Psychonomic Bulletin & Review, 23(5), 1316–1340. https://doi.org/https://doi.org/10.3758/s13423-015-0982-5
- Murray, J. H. (2011). Inventing the medium: Principles of interaction design as a cultural practice. Mit Press.
- Nelson, T. H. (1974). Computer lib: you can and must understand computers now /dream machines: New freedoms through computer screens—a minority report. The Distributors.
- Nelson, B. D., & Hajcak, G. (2017). Defensive motivation and attention in anticipation of different types of predictable and unpredictable threat: A startle and event‐related potential investigation. Psychophysiology, 54(8), 1180–1194. https://doi.org/https://doi.org/10.1111/psyp.12869
- Nguyen, C. T. (2020). Games: Agency as art. Oxford University Press.
- Nintendo. (1985) Super mario bros. (game boy advance) [Video Game]. Nintendo
- Normand, A., Bouquet, C. A., & Croizet, J.-C. (2014). Does evaluative pressure make you less or more distractible? Role of top-down attentional control over response selection. Journal of Experimental Psychology: General, 143(3), 1097. https://doi.org/https://doi.org/10.1037/a0034985
- Ordóñez, L. D., Benson, L., III, & Pittarello, A. (2015). Time-pressure perception and decision making. The Wiley Blackwell Handbook of Judgment and Decision Making, 2, 519–542. https://doi.org/https://doi.org/10.1002/9781118468333.ch18
- Pessoa, L. (2015). Multiple influences of reward on perception and attention. Visual Cognition, 23(1–2), 272–290. https://doi.org/https://doi.org/10.1080/13506285.2014.974729
- Petersen, S. E., & Posner, M. I. (2012). The attention system of the human brain: 20 years after. Annual Review of Neuroscience, 35(1), 73–89. https://doi.org/https://doi.org/10.1146/annurev-neuro-062111-150525
- Pirolli, P. (2005). Rational analyses of information foraging on the web. Cognitive Science, 29(3), 343–373. https://doi.org/https://doi.org/10.1207/s15516709cog0000_20
- Pirolli, P., & Card, S. (1999). Information foraging. Psychological Review, 106(4), 643. https://doi.org/https://doi.org/10.1037/0033-295X.106.4.643
- PlayDots. (2014) Two dots (android) [Video Game]. Playdots Inc
- Poels, Y., Annema, J. H., Verstraete, M., Zaman, B., & De Grooff, D. (2012). Are you a gamer? A qualititive study on the parameters for categorizing casual and hardcore gamers. Iadis International Journal on Www/internet, (1), 1–16. https://lirias.kuleuven.be/1859869?limo=0
- Pope, L. (2013) Papers, Please (Windows PC) [Video Game]. 3909 LLC.
- Power, C., Cairns, P., Denisova, A., Papaioannou, T., & Gultrom, R. (2018). Lost at the edge of uncertainty: Measuring player uncertainty in digital games. International Journal of Human–Computer Interaction, 35(12), 1033–1045. https://doi.org/https://doi.org/10.1080/10447318.2018.1507161
- Qian, M., & Clark, K. R. (2016). Game-based learning and 21st century skills: A review of recent research. Computers in Human Behavior, 63, 50–58. https://doi.org/https://doi.org/10.1016/j.chb.2016.05.023
- Rambusch, J. (2011). Mind games extended: Understanding gameplay as situated activity. Linköping University Electronic Press].
- Reeves, S., Greiffenhagen, C., & Laurier, E. (2017). Video gaming as practical accomplishment: Ethnomethodology, conversation analysis, and play. Topics in Cognitive Science, 9(2), 308–342. https://doi.org/https://doi.org/10.1111/tops.12234
- Reinecke, L. (2017). Mood management theory. The International Encyclopedia of Media Effects, 1, 1–13. https://doi.org/https://doi.org/10.1002/9781118783764.wbieme0085
- Rensink, R. A. (2000). When good observers go bad: Change blindness, inattentional blindness, and visual experience. Psyche, 6(9). http://cogprints.org/1050/
- Rensink, R. A. (2015). A function-centered taxonomy of visual attention. Phenomenal Qualities: Sense, Perception, and Consciousness, 31. https://www2.psych.ubc.ca/~rensink/publications/download/taxonomy-rr.pdf
- Robison, M., Unsworth, N., & Brewer, G. (2020). Examining the effects of goal-setting, feedback, and incentives on sustained attention,47(6), 869. https://doi.org/https://doi.org/10.1037/xhp0000926
- Roda, C. E. (2011). Human attention and its implications for human-computer interaction. Cambridge University Press.
- Roda, C., & Thomas, J. (2006). Attention aware systems: Theories, applications, and research agenda. Computers in Human Behavior, 22(4), 557–587. https://doi.org/https://doi.org/10.1016/j.chb.2005.12.005
- Ryan, R. M., & Rigby, C. S. (2019). Motivational foundations of game-based learning. Handbook of Game-based Learning, 153–176.
- Schell, J. (2008). The art of game design: A book of lenses. CRC Press.
- Schrank, B., & Bolter, J. D. (2014). Avant-garde videogames: Playing with technoculture. MIT Press.
- Schreiber, I., & Romero, B. (2021). Game balance. CRC Press.
- Schroeder, C. E., Wilson, D. A., Radman, T., Scharfman, H., & Lakatos, P. (2010). Dynamics of active sensing and perceptual selection. Current Opinion in Neurobiology, 20(2), 172–176. https://doi.org/https://doi.org/10.1016/j.conb.2010.02.010
- Schull, N. D. (2012). Addiction by design: Machine gambling in las vegas. Princeton University Press.
- Sheridan, H., & Reingold, E. M. (2014). Expert vs. novice differences in the detection of relevant information during a chess game: Evidence from eye movements. Frontiers in Psychology, 5, 941. https://doi.org/https://doi.org/10.3389/fpsyg.2014.00941
- Sicart, M. (2008). Defining game mechanics. Game Studies, 8(2). http://www.gamestudies.org/0802/articles/sicart
- Simons, D. J., & Chabris, C. F. (1999). Gorillas in our midst: Sustained inattentional blindness for dynamic events. Perception-London, 28(9), 1059–1074. https://doi.org/https://doi.org/10.1068/p281059
- Solarski, C. (2013). The aesthetics of game art and game design . Game Developer. https://www.gamedeveloper.com/design/the-aesthetics-of-game-art-and-game-design
- Squire, K. (2006). From content to context: Videogames as designed experience. Educational Researcher, 35(8), 19–29. https://doi.org/https://doi.org/10.3102/0013189X035008019
- Sreejesh, S., & Anusree, M. (2017). Effects of cognition demand, mode of interactivity and brand anthropomorphism on gamers’ brand attention and memory in advergames. Computers in Human Behavior, 70, 575–588. https://doi.org/https://doi.org/10.1016/j.chb.2017.01.033
- Sundstedt, V., Stavrakis, E., Wimmer, M., & Reinhard, E. (2008). A psychophysical study of fixation behavior in a computer game. Proceedings of the 5th symposium on applied perception in graphics and visualization (pp.43–50).
- Sweller, J., Ayres, P., & Kalyuga, S. (2011). Cognitive load theory in perspective. In Cognitive load theory (pp. 237–242). Springer.
- Tanovic, E., Pruessner, L., & Joormann, J. (2018). Attention and anticipation in response to varying levels of uncertain threat: An ERP study. Cognitive, Affective, & Behavioral Neuroscience, 18(6), 1207–1220. https://doi.org/https://doi.org/10.3758/s13415-018-0632-2
- Taylor, T. L. (2012). Raising the stakes: E-sports and the professionalization of computer gaming. Mit Press.
- Tlili, A., Essalmi, F., Jemni, M., Chen, N.-S., Huang, R., & Burgos, D. (2020). The evolution of educational game designs from computers to mobile devices: A comprehensive review. In D. Burgos (Ed.), Radical solutions and eLearning (pp. 81–99). Springer.
- Todi, K., Jokinen, J., Luyten, K., & Oulasvirta, A. (2019). Individualising graphical layouts with predictive visual search models. ACM Transactions on Interactive Intelligent Systems (Tiis), 10(1), 1–24. https://doi.org/https://doi.org/10.1145/3241381
- Vuilleumier, P., Schwartz, S., Duhoux, S., Dolan, R. J., & Driver, J. (2005). Selective attention modulates neural substrates of repetition priming and “implicit” visual memory: Suppressions and enhancements revealed by FMRI. Journal of Cognitive Neuroscience, 17(8), 1245–1260. https://doi.org/https://doi.org/10.1162/0898929055002409
- Walker, A., Le Pelley, M., & Beesley, T. (2017). Exploitative and exploratory attention in a four-armed bandit task. In CogSci. The Annual meeting of the Cognitive Science Society. https://cogsci.mindmodeling.org/2017/papers/0656/
- Wasserman, J. A., & Koban, K. (2019). Bugs on the brain: A mental model matching approach to cognitive skill acquisition in a strategy game. Journal of Expertise/June, 2(2). https://www.journalofexpertise.org/articles/volume2_issue2/JoE_2019_2_2_Wasserman_Koban.pdf
- Whitby, M. A., Deterding, S., & Iacovides, I. (2019). “One of the baddies all along” moments that challenge a player’s perspective. Proceedings of the annual symposium on computer-human interaction in play (pp.339–350). Association for Computing Machinery, Inc.
- Whitton, N. (2014). Digital games and learning: Research and theory. Routledge.
- Wood, K., & Simons, D. J. (2019). The spatial allocation of attention in an interactive environment. Cognitive Research: Principles and Implications, 4(1), 13. https://doi.org/https://doi.org/10.1186/s41235-019-0164-5
- Xu, Q., & Sundar, S. S. (2016). Interactivity and memory: Information processing of interactive versus non-interactive content. Computers in Human Behavior, 63, 620–629. https://doi.org/https://doi.org/10.1016/j.chb.2016.05.046
- Zagal, J. P., & Mateas, M. (2010). Time in video games: A survey and analysis. Simulation & Gaming, 41(6), 844–868. https://doi.org/https://doi.org/10.1177/1046878110375594