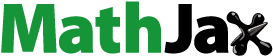
1. Introduction
Conversational agents (CAs) have been the subject of wide interest among researchers in different scientific domains. Equipped with one of the most prevalent indicators of humanness, speech interfaces can enhance machines with powerful social cues (Nass & Gong, Citation2000; Sah & Peng, Citation2015). A machine’s verbal cues can entice social responses in users and trigger them to apply social rules and expectations to computers (Nass & Moon, Citation2000). While it is still a matter of debate whether users actually anthropomorphize computers or just undertake mindless social responses, the user experience (UX) with CAs has gained a lot of attention in recent years. Researchers explore how users converse with CAs (Clark et al., Citation2019; Porcheron et al., Citation2018) and how they react to conversational breakdowns (Beneteau et al., Citation2019; Myers et al., Citation2018). Several studies have focused on understanding and measuring the unique user experience of interaction with CAs (Cowan et al., Citation2017; Lopatovska et al., Citation2018), often with a focus on anthropomorphized perceptions (Kuzminykh et al., Citation2020; Lopatovska & Williams, Citation2018). A number of studies found that gaps between user expectations and actual user experience diminish user satisfaction (Luger & Sellen, Citation2016; Nguyen & Sidorova, Citation2017; Zamora, Citation2017). In UX research, it therefore seems important to better understand emotional responses to this new technology. Yet only a few studies have investigated these (Cohen et al., Citation2016; Purington et al., Citation2017; Yang et al., Citation2019). When looking into the way emotional responses to CAs are studied (e.g., in Yang et al., Citation2019), we see that well-established scales from the UX design field are used, such as the PANAS scale developed by (Watson et al., Citation1988). However, in applying these scales, the ability to capture emotional experiences is limited. Emotions are rarely differentiated beyond a basic positive-negative distinction. In this article, we argue that this form of measuring the emotional user experience limits the depth in which CAs can be analyzed and understood. Our findings indicate that CAs evoke a much more nuanced spectrum of positive and negative emotions, which is also in line with previous work that identified finer distinctions of positive responses, such as satisfaction, affection or loyalty (Lopatovska & Williams, Citation2018; Nasirian et al., Citation2017; Portela & Granell-Canut, Citation2017). These emotional nuances can be better apprehended when coupling them to specific value implications.
The indication that “acts of value-feeling” allow people to understand the world around them (including CAs) has a long philosophical tradition in Material Value Ethics (Scheler, Citation1973/1921). Likewise, in the Value Sensitive Design field, emotions have been identified as a human response to the value implications of technology: “Emotions can and should play an important role in understanding values” (Desmet & Roeser, Citation2015, p. 207). Vice versa, we would argue that understanding the values that matter to an individual is a gateway to identifying the sources of their emotional reactions to technology. Most scholars agree by now that artifacts “embody” values (Kroes & Verbeek, Citation2014) and that values are implicated through engagement with technological artifacts (Davis & Nathan, Citation2015; Spiekermann, Citation2016). In studies that have aimed to reveal the fundamental values implicated by CA usage, values have often been conceptualized as user objectives (e.g., in Rzepka (Citation2019)), which are achievable through the system or in terms of system affordances that extend or constrain a user’s space of action (Moussawi, Citation2018). Moussawi identified a variety of sensory, functional, cognitive and physical affordances, which shape the interaction between users and CAs. However, conceptualizing values as objectives or affordances, which shape the immediate interaction falls short of capturing the variety of moral, social and personal values that can be fostered or harmed through a CA.
Against this background, we integrate in this article the two separately treated fields of values in philosophy and design on one side and emotional user experience research on the other. We develop a novel integrated representation we call “Emotion Value Assessment” (EVA). The proposed EVA allows researchers and practitioners in the Human-Computer Interaction (HCI) domain to explore the emotional reactions to a technology in relation to the underlying values fostered or harmed. It takes into consideration that the human-technology relationship plays out on different levels of interaction, i.e. when users perceive technology, use technology or consider the social implications of a technology. In a qualitative study of 30 Alexa users, we demonstrate how an EVA can capture values and emotions alongside these categories. The results of the EVA can provide HCI scholars and professionals with deep insights into the interaction dynamics at play and help them explore the emotional aspects of technology in a more nuanced way than has been possible with existing UX approaches. We argue that EVAs can enrich existing HCI research with a suitable framework to conceptualize experiential aspects that go beyond the immediate interaction with technological artifacts. In practice, EVAs could be used to inform product design and development cycles, as the assessment may lead to conclusive ideas about how specific product dispositions support or undermine human values and how that is emotionally perceived by users.
Our research article addresses two research questions. First, which value implications can be identified when users perceive, interact with, or consider the social implications of a CA? Secondly, how do these value implications relate to the emotional responses experienced by CA users?
2. Background
Several disciplines provided the theoretical framework for our study and guided our study design, specifically the fields of Value Sensitive Design (VSD), Material Value Ethics, Emotional Design, and HCI. To outline our methodological choices for constructing the EVA, we will first explain the concepts and assumptions that underlie our approach, and depict how we relate value conceptualizations of different disciplines to technology. In the second section, we tie the idea of value implications to classical UX models and introduce the concept of value-bearers as a bridge between these seemingly unrelated domains. We then evaluate different approaches to capturing emotional responses and turn to the Design field to categorize the sources of emotions in the interaction with technology. Section 2.4 discusses how our theoretical framework informed and guided our methodological choices. Finally, as we focus this study on CAs, section 2.5 takes a deep dive into prior CA research with a particular focus on the emotional perception of CAs and on studies that have considered the value implications of CAs.
2.1. Values and emotions
Value-sensitive design scholars have embraced a definition of values as “what is important to people in their lives, with a focus on ethics and morality” (Friedman & Hendry, Citation2019, p. 24). A definition that cultural anthropologists have developed goes into a similar direction, with an emphasis on values as implicit or explicit conceptions of the desirable, which “influence the selection from available modes, means and ends of actions” (Kluckhohn, Citation1962, p. 395). This definition underlines that the values humans consider to judge behavior and take decisions have affective, cognitive and conative elements. Their affective elements stem from the attraction (desirability) that people feel toward values. Their cognitive elements lie in their verbalizability as conceptions and their conative elements become clear in their nature of providing us with orientation when we take decisions and select from a set of actions.
To clarify how the concept of values relates to objects, a look into Material Value Ethics is helpful (Kelly, Citation2011; Scheler, Citation1973/1921). Philosophers in this field have long regarded values as phenomena that give sense and meaning to objects, essentially turning “objects” into “goods” (Hartmann, Citation1949). With a more specific focus on values in technology, VSD (Friedman & Hendry, Citation2019) and Value-based Engineering scholars (VbE, Spiekermann & Winkler, Citation2020) agree on the idea that designed artifacts have an ethical impact when they positively or negatively implicate human values. Values are seen as being “embodied” by technology (Van de Poel & Kroes, Citation2014) or “carried” by the system’s designed qualities (IEEE Standards Association, Citation2021). Either way, the underlying understanding is that technology has specific dispositions (deliberately designed or not) that constitute its potential to implicate values among those who use it. Whether these potential value implications actually do unfold eventually depends on the individual users, their background and moral views, their unique personal situation and specific use context (Van de Poel & Kroes, Citation2014). Based on these assumptions, we can argue that technological value implications are phenomena in which a technology––directly and indirectly, consciously or unconsciously––gains relevance for individual users because it affects something they consider valuable. Through this mechanism technology has implicit impacts “on our moral decisions and actions, and on the quality of our lives” (Verbeek, Citation2008, p. 92).
What needs to be clarified at this point is precisely which values should be taken into consideration when looking into technological value implications. We see the central gateway to values in their verbalizability as conceptions. Many disciplines have verbalized values, often in the form of value lists. Winkler and Spiekermann (Citation2019) integrated some of the most important of these lists in an aggregation of human values that they argue should be considered when designing sustainable technology. Their value collection includes principles from the design and engineering field (such as accountability, usability, maintainability), fundamental rights and goals drawn from the area of law (e.g., dignity, justice, freedom), ethical principles and virtues drawn from philosophy (such as benevolence, kindness, wisdom) and fundamental human needs drawn from psychology (such as relatedness, well-being, self-actualization). In consideration of the important overlap among some of these value collections, they pooled and categorized them to develop a system of 31 overarching values, each specified with various subordinate values. Given the collection’s direct relevance for information systems, we use this extensive value framework as the starting point for our qualitative analysis of technological value implications (Appendix A).
At this point, the question arises of how values relate to emotions. There are indeed good arguments to consider emotional responses when attempting to identify value implications in human interaction with technology. From a psychological perspective, emotions are essential in prioritizing and organizing ongoing behaviors in order to optimize an individual’s adjustment to its physical and social environment (Keltner & Gross, Citation1999). The conative function of emotions is a remarkable parallel to the previously stated definition of values. It therefore appears reasonable that Value Sensitive Design scholars consider emotions as spontaneous reactions to the values involved in technology. As “an expression of personal and moral values,” emotions can be a source of awareness of what matters to a user (Desmet & Roeser, Citation2015, p. 204) and they can serve as a gateway to users’ values and moral considerations regarding technologies (Steinert & Roeser, Citation2020). This argumentation, again, resonates with Material Value Ethics (Scheler, Citation1973/1921). Here, emotions are understood as responses to the value-ladenness of objects, persons, symbols, relationships, activities or situations. The “emotional a priori” is a first, intuitive sense for values, which can lead to conscious awareness of what “ought to be” (Hartmann, Citation1949, pp. 135–138). The often implicit nature of values hence becomes tangible and concrete in the emotional response they elicit. If we understand emotions as a natural human response to value implications, it is reasonable to approach the value implications of technology through the emotional responses they create.
2.2. Distinguishing value-bearers: person-based vs. artifact-based values
Where does current UX research position itself with regard to values and emotional responses in technology? Hassenzahl (Citation2005) distinguished between “hedonic” and “pragmatic” software attributes. This still widely applied distinction is made by examining the function a technology fulfills for a particular user: “Pragmatic attributes emphasize the fulfilment of individuals’ behavioral goals, hedonic attributes emphasize individuals’ psychological well-being” (Hassenzahl, Citation2005, p. 35). Yet in recent years, notable arguments have emerged in the UX field to develop a broader understanding of (user) experience as subjective, holistic, situated, dynamic, and worthwhile (Hassenzahl, Citation2013). Accordingly, it is argued, we need to understand why specific experiences matter and evoke emotions. We need to consider that there is an underlying meaning that specific experiences have for individual users in their situation. Hassenzahl contends that this meaning level can lead us to the source and reason of emotional responses and suggests approaching the meaning level by considering universal psychological needs. Accordingly, technology-mediated experiences are pleasant when they answer to psychological needs: A smartphone, for example, can help users to fulfill their need for relatedness (Hassenzahl, Citation2013).
While we generally agree with this idea, we argue that the value terminology could allow us to extend the perspective beyond psychological needs. At this point, we see two interesting parallels to the conceptualization of values––one in their conative function of providing meaning and orientation, and another in their affective component of being perceived emotionally. Arguably, when users form an attitude toward a technology, they are likely to consider more aspects than just their own psychological needs. For example, a technology could be perceived negatively if users reflect on bad working conditions and child labor involved in the production process of their smartphones. The value terminology is broad enough to capture these aspects, and values like fairness, human welfare and sustainability can grasp the meaning-level of such considerations precisely. Fairness is not a basic human need. Nevertheless, the instinctive perception of fair and unfair can significantly alter a person’s attitude and behavior. Moreover, many value lists explicitly include psychological needs and thus we would not lose them if we address values instead of psychological needs to tackle the inherent meaning of experiences. The value terminology can capture a technology’s dispositions and qualities as well as its impacts–beneficial or harmful, intended or unintended–on anything that users value in their lives.
Nevertheless, Hassenzahl’s distinction of pragmatic and hedonic software attributes is helpful. Combined with the value terminology, it can help us to locate where value impacts unfold. We therefore seek to maintain a similar distinction for values. Following Hassenzahl (Citation2005), pragmatic attributes include all qualities that support the fulfillment of users’ (functional) goals, such as utility, usability or usefulness. However, a focus on the artifact itself instead of users’ intentions may be preferable. Users can form an impression about an artifact without a pragmatic goal in mind. Prior assumptions, for example, can remarkably shape users’ attitudes toward an artifact before they even use it. A recent study that identified concerns over data protection as a major reason for non-adoption of CAs underlines this notion (Lau et al., Citation2018). Such perceptions appear to be detached from concrete interactions and explicit user objectives, and they remain largely out-of-scope in models that focus on the goals users try to achieve when using a technology (such as the often applied Means-End approach by Reynolds and Olson (Citation2001)). In order to include them, we will refer to artifact-based values instead of pragmatic attributes. This framing allows us to capture the qualities and flaws of an artifact without being constrained by explicit user goals.
Secondly, we refer to person-based values instead of hedonic attributes. In current UX models, hedonic attributes of a product are defined as means to help users to achieve hedonic goals that relate to human needs, such as relatedness, self-expression or achievement (Hassenzahl & Roto, Citation2007; Mahlke & Thüring, Citation2007). With our terminology, we capture values that unfold in the person. More precisely, we aim to capture any direct and indirect, beneficial and harmful impacts on users, their social environment and society. The term “hedonic” is particularly problematic, as it refers to the attribute’s potential to generate pleasure. This terminology anticipates an emotional response, whereas we seek a clear differentiation between emotional responses and value implications. Another important shortcoming is the term’s concentration on positive emotions (pleasure), which is bound to ignore impacts of the artifact that generate negative emotions.
A practical example can best illustrate what the distinction of person-based values and artifact-based values means when we look at concrete user experiences. Let us assume a CA’s voice interface is used by a visually impaired person. By giving this user the option to perform requests verbally, the artifact becomes accessible. Conceptually, the artifact-based value of accessibility is elicited. The same incident, however, can create a value impact in the person to whom the artifact is made accessible: The visually impaired user may feel more independent, a value borne by the person. Consequently, the artifact’s accessibility is instrumental in eliciting person-based values like independence. Along the same line of argumentation, harms of artifact-based values can come with a potential to harm person-based values. Inadequate data protection, for example, creates the potential to harm users’ privacy. Conceptually, this means that the artifact-based value of data protection is harmed, resulting in the potential harm of the person-based value privacy. The importance of this distinction becomes even clearer when we add emotional responses to the picture. The visually impaired user may experience the voice interface with pride and joy. To explain this emotional response, it is critical to disentangle whether it was elicited by the accessibility of the artifact or by the independence created in the user. To address the meaning-level of emotional experiences we have to identify whether the emotion was a response to an artifact-based value or a person-based value.
2.3. Capturing value-based emotions
As values are perceived emotionally, emotions can point us to relevant value implications in human interaction with technology. Given this important role of emotional responses, the question of how to adequately identify and capture them becomes immanent. Emotional responses to technology have been measured in manifold ways. Verbal measurement tools, in particular semantic differentials, are widely used in UX research because they require comparably little time and effort to collect and analyze the data (cf. PANAS scale (Watson et al., Citation1988)). Yet, they often come with a simplified differentiation between positive and negative affect, which is likely to not adequately reflect the complex reality of user experiences. In order to establish a more nuanced representation of emotions, we suggest applying prototype emotion theory (Plutchik, Citation1980; Rosch, Citation1975; Shaver et al., Citation1987). This theory accounts for nuanced emotional reactions to phenomena and seems promising in terms of capturing, in a very granular way, how people react to distinct values. The theory is rooted in the idea that humans develop a common understanding of the meaning of emotion words during language acquisition. This emotional knowledge is organized in perceptual and semantic categories, which can be represented in tree-like taxonomies ().
Figure 1. Basic Emotion Prototypes (based on Shaver et al., Citation1987).
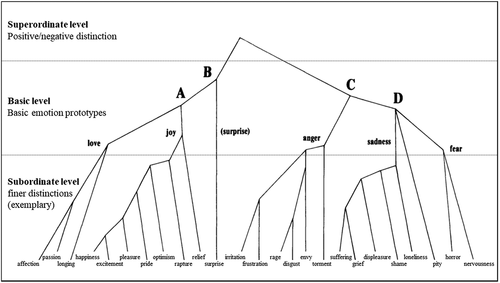
Prototype structures are typically represented on three levels. The top (superordinate) level distinguishes negative and positive emotions. The middle (basic) level usually distinguishes between 5–10 basic emotion prototypes. Basic emotions are the most salient: they are learned earliest and represent the categories that are most broadly understood and most frequently used (Shaver et al., Citation1987). The lowest (subordinate) level comprises finer distinctions of each basic emotion. Studies in UX research, which use prototype emotions as the theoretical basis for measuring emotional user experiences, are still rare (cf., Saariluoma & Jokinen, Citation2014).
In addition to this conceptual basis for emotions, we argue that it is possible to precisely locate the origin of emotional responses in human interaction with technology. Scholars in the Design field have developed a framework that categorizes the emotions elicited when we engage with technological artifacts (Desmet, Citation2012; Desmet & Roeser, Citation2015). Desmet and Roeser argue that emotions in human-technology interaction originate from different sources, and group product-related emotions according to what the emotion focuses on. According to this framework, emotions can originate from six different areas. First, the authors grouped emotions that arise when users perceive technology or connect a symbolized meaning to it. In a second category, emotions stem from interacting with a technology or from activities that the technology supports and enables users to perform. In the third group, emotions stem from the implications technology has on a user’s social relationships or their identity. A product’s unique qualities materialize in these six areas and elicit emotions. As argued above, we consider technological objects to have value dispositions that result in emotional responses. We therefore slightly adapt Desmet and Roeser’s categorization of product-emotions so that it refers to areas of qualities instead of foci of emotions (see, ).
Table 1. Framework to group technology qualities, based on Desmet and Roeser (Citation2015).
UX frameworks that combine the analysis of emotional responses and human values are rare but there have been noteworthy initiatives in prior research (cf., Fuchsberger et al., Citation2012; Nurkka, Citation2008). Compared with the EVA, the most central differences of the EVA lie in the underlying conceptualization of values and in the methods proposed for capturing emotional responses and values. Fuchsberger et al. (Citation2012) referred to a value classification based on the Theory of Consumption Values (Sheth et al., Citation1991) which treats “emotional value” as an end of its own. Without rejecting this framework in principle, we believe it contradicts our research goal of using emotional reactions as a gateway to capture underlying value implications. With regards to data collection methods, Nurkka (Citation2008) proposes to survey individual study participants about their values and beliefs and to use this evaluation as a predictor for the emotional perception of a technology. The idea is reasonable, and it has been argued in the past that people’s values and beliefs predict their emotional responses to technologies (Perlaviciute et al., Citation2018). Yet, a distinction needs to be drawn between the general moral beliefs and values a person holds and the nuanced and context-dependent value implications of a technology. Prior to using a technology, users may not be able to anticipate the spectrum of values that could be implicated by the technology, nor whether these value implications will eventually be of any importance to them in a specific context and situation. The actual value implications of a technology may be unexpected, delayed, or only indirectly related to immediate interactions. Therefore, the EVA identifies emotional responses in users’ reflections and uses them as indicators for the presence of a meaningful value implication. Value implications are accessed through the lens of emotional responses, essentially examining both phenomena interdependently. summarizes the framework of the different theoretical concepts that informed our study’s approach for constructing the EVA.
2.4. Transferring the theoretical basis into a suitable methodological framework
Our theoretical framework informed and guided our research design, and in particular our selection of data collection methods. We interpret value implications as phenomena in which a technology gains relevance for individual users because it affects something they consider valuable. The conceptual nature of values creates a strong argument for a qualitative research approach that allows for follow-up questions and remains open to discuss and explore the meaning that specific experiences had for users. Hence, we decided to focus our study design on the attitudinal dimension of experiences, as reflected by users in retrospect, instead of observing immediate actions and behaviors. Emotion prototypes are suitable for analyzing verbal data because they have their roots in the analysis of human language and expression.
Among specific qualitative study designs, we decided to combine two flexible data collection methods––semi-structured interviews and moderated focus group sessions. The combination of data collection methods allowed us to benefit from the advantages of each method: Interviews provide room for detailed discussions of individual experiences, as well as underlying motives and thoughts. The informal setting encourages participants to talk freely and uninhibitedly. In focus groups, on the other hand, participants can build upon the accounts of others and query each other (Carey, Citation1994). Value implications may often be unconscious, implicit or hard to formulate spontaneously. This is an argument for collecting data in group sessions where participants can inspire and complement each other, at times triggering a fruitful discussion without instruction from the moderator.
2.5. Values and emotional responses in CA research
In the HCI field, several streams of research have dealt with the user-CA relationship, and a number of studies investigated the values and emotions at work. Taking the abovementioned categories of “perceiving the object,” “using the object” and “social implications” as a general structure, we can summarize prior CA research as follows:
2.5.1. Perceiving CAs
Ever since the Computers Are Social Actors paradigm (Nass et al., Citation1994) deepened our understanding of users’ attribution of human-like characteristics to technology, researchers have investigated the effects of social cues in technology. Users’ personification of CAs has significant effects on their emotional responses and behavior. For example, it has been suggested that personification translates into higher loyalty and satisfaction and elicits affection in users (Lopatovska & Williams, Citation2018). Portela and Granell-Canut (Citation2017) found that CAs were perceived as empathetic when they remembered information from earlier conversations (such as the user’s name). This kind of agent behavior can trigger a rise in affection among users, which manifests in empathetic feelings toward the CA. Similarly, Cho (Citation2019) found that voice interactions significantly enhanced the perceived social presence of the agent and thus elicited positive attitudes toward the agent. This can be interpreted as a connection between a value implication in the area of object perception (social presence) and the user’s emotional response (positive attitude). Luger and Sellen (Citation2016) found that humorous episodes with a CA strengthened users’ impression that the CA possessed social intelligence. As humor increased the CA’s perceived human-likeness, it also raised expectations of system capabilities, which, if unmet, evoked an emotional response of dissatisfaction. Cowan et al. (Citation2017) studied the perceptions and attitudes of infrequent users and discovered that concerns about the values of privacy and transparency discourage these users from more regular usage.
2.5.2. Using CAs
In a number of studies that evaluated user needs and satisfaction, emotional user responses started to shift more to the center of investigation (Clark et al., Citation2019; Kiseleva et al., Citation2016; Nasirian et al., Citation2017; Zamora, Citation2017). Nasirian et al. (Citation2017) suggest that the interaction quality with CAs can foster users’ trust and adoption of CAs. Luger and Sellen (Citation2016) identified a common source of user dissatisfaction in the necessity to continuously work and invest time in order to make effective use of the CA. Interactional and conversational trouble were often discussed, and represent relevant value harms (such as usability, understandability or intuitiveness). A number of studies investigated typical errors that users encounter and the tactics they employ to overcome them (Beneteau et al., Citation2019; Jiang et al., Citation2013; Myers et al., Citation2018; Porcheron et al., Citation2018; Reeves et al., Citation2018). Many of these authors criticized CAs’ non-seamless, unnatural conversation patterns that are hardly compatible with “the real world’s complex […] multiactivity settings” (Reeves et al., Citation2018, p. 51). These studies shed light on a number of values harmed or insufficiently achieved by CAs, such as seamlessness, compatibility, or complexity. Two recent studies on usage behavior mark a first and significant step in CA research toward the identification of value implications in different areas of users’ lives. Rzepka (Citation2019) evaluated the objectives users try to fulfill when interacting with CAs. The five fundamental objectives identified in this study explain why users choose the CA over other interface modalities in particular situations (efficiency, convenience, ease of use, less cognitive effort, and enjoyment). Yet, the fulfillment of user objectives is likely to represent only a part of the value benefits CA can potentially offer, and value harms were not investigated. Moussawi (Citation2018) clustered the benefits of CA usage based on types of affordances: The author revealed sensory affordances (hands- and eyes-free usage, familiarity, emotional connection), functional affordances (such as speedy assistance, usefulness), cognitive affordances (such as personalization, learning from interactions) and physical affordances (potential improvement). The identified affordances focus primarily on the object and direct interaction with it. However, the study does not clearly disentangle value benefits from emotional responses (for example, in its categorization of emotional connection as sensory affordance). In addition, the author concentrates on value benefits and thus neglects potential value harms the system may create. Although the exploration of emotions in relation to CA usage has been highlighted as key in future CA design (Cohen et al., Citation2016), relatively little prior research focuses specifically on affective reactions to different CA qualities. The most relevant work was reported in Yang et al. (Citation2019). The authors categorized users’ affective responses into positive and negative affect, with three subgroups of positive affect. Affective responses are understood here as responses to hedonic and pragmatic qualities, a differentiation made based on the distinction of software product attributes (Hassenzahl, Citation2005). A central finding the authors report is that qualities that are not directly task-related, such as privacy or distraction from boredom, have a stronger influence on the overall positive affect reported by users. In contrast, task-related qualities such as CA responsiveness and fluidity of interactions had a slightly stronger impact on negative affect.
2.5.3. Social implications
A large stream of research has focused on specific user groups, for example, on the experiences of children (Druga et al., Citation2017; Lovato & Piper, Citation2015; Xu & Warschauer, Citation2020), visually impaired (Choi et al., Citation2020) or elderly users (Wulf et al., Citation2014). Many of these studies discussed accessibility as a central value quality for these user groups, clearly one that comes with social implications. The ability to control the device alone can enhance users’ quality of life, as voice interaction can “make their life easier and encourage their continued participation in the society” (Meliones & Maidonis, Citation2020, p. 272). Several studies investigated the integration of CAs in homes and their impacts on family routines. For example, Beirl et al. (Citation2019) found that CA usage in families uniquely changed family cohesion behavior and led to new family rituals. Their study revealed that families developed new interaction patterns and collaborated to manage Alexa.
With respect to prior research on conversational agents, it can be noted that nuanced emotional responses are still under-researched, particularly in relationship with value implications as the origin of these responses. While this seems to be a gap in previous HCI research in general, it is particularly interesting to bridge in the context of CA technology. CA’s endowment with social cues, their embeddedness in private homes and their rapidly growing adoption rates are unique. For a multifaceted technology like CAs, which can affect a wide range of values in diverse contextual settings, the deeper investigation supported by the EVA seems particularly worthwhile.
3. Method
A qualitative study based on interviews and focus groups was designed to collect data on value implications related to CAs and the emotional responses they elicit. The study was conducted in the fall of 2018. In the study design, we accounted for two main requirements. First, we aimed to avoid variations that stem from the differences in functions and attributes of different CA applications (e.g., Google Assistant, Cortana, Alexa). Our investigation therefore included only users of Amazon Alexa, the most common smart speaker-based voice assistant in Austria, where this study was undertaken (Statista, Citation2020). We did not exclude participants who also owned other smart speakers along with their Alexa device. Secondly, we aimed to improve the comparability of experiences by narrowing down the scope of use contexts to the household: We focused on participants who used Alexa within their home, usage on portable devices was not included (e.g., smartphone, smart watch or in-vehicle applications). Prior research has shown that there are reservations about using conversational agents in public spaces: users are more cautious regarding the type of information they transmit and generally prefer using CAs in private locations (Moorthy & Vu, Citation2015). The focus on in-home application therefore allows us to investigate a setting in which participants use the CA freely because they feel “at home.” Our department’s ethical committee formally approved our study design.
3.1. Data collection
We engaged 30 participants to share their experiences with Alexa in focus groups in the context of a research project investigating interactions with CAs. Participants were recruited via e-mail distribution to current and former university students and via a blog of a national newspaper, a strategy we adopted to improve our sample’s representativity. Still, with 9 female and 21 male participants, our sample was skewed toward male millennials, similar to Amazon Alexa’s market user base at the time the data were collected (eMarketer, Citation2018; Koksal, Citation2018). Participants were 18–67 years old (median 23, mean 27), with 63% of them students. An overview of our sample’s distribution into groups, demographics, use context, and most used functions is provided in Appendix C granted a compensation of 20€ to each participant. Before entering focus group sessions, we conducted one-to-one interviews via telephone with five participants (two females and three males, aged 22–67 with median 36 and mean 38.6). The interview participants were selected from the recruited sample as a high variation sample (Marshall, Citation1996), seeking diversity of age and CA use contexts (i.e. family with children, visual impairment, smart home, CA as multi-media center, rented-out apartment). We assumed that these participants have very different reasons for adopting a CA, and therefore attach importance to different applications and functions. Investigating these diverse use contexts helped us to establish a broad overview of possible experiences in diverse situations, which we deemed important in order to prepare focus group sessions. In the interviews, we followed a semi-structured interview protocol to openly explore participants’ experiences and the context behind them. The interviews lasted on average 62 minutes. We started by querying participants’ usage behavior (functions they used, how often and how regularly, how they interacted with the CA). Once we were convinced that interviewees felt comfortable and talked freely, we proceeded to ask for experiences that stood out, and why they did. Depending on their accounts, we followed up on specific situations or their emotional reception.
The interviews helped us develop a moderator guide that provided the structure and content for our focus group sessions (see Appendix D). We reviewed the interview material and thematically coded it on a high level to identify relevant themes that would be expedient in group discussions. We selected themes that were broad enough to include most of the experiences we had collected at that time, including functions and applications, communication, expectations, characterization, changes, surprises, and risks. The interviews also revealed to us that participants appeared to be more likely to discuss emotional responses when we asked them for specific situations they remembered and why they did. Memories of concrete situations often prompted interviewees to remember how they felt in that situation and how their general attitude toward the CA had evolved. We therefore selected focus group techniques that would lead to early discussions of specific situations and experiences along the lines of selected themes.
The remaining 25 participants were randomly assigned to six focus groups held on university campus. Sessions were initiated with an explanation of the tools and techniques to be used. An introductory round, during which participants discussed for how long they had owned a CA and in which situations they mostly used it, served as an icebreaker. Second, we performed a round-robin questionnaire (Langford & McDonagh, Citation2003). Participants were handed paper sheets on which the beginning of a sentence was written, such as: “I will never forget how Alexa … .” They were instructed to complete the sentences with what they thought was the most appropriate answer before handing the sheet clockwise to their neighbor. When all participants had completed all sentences, the sheets were pinned up and jointly discussed. This method proved to be particularly thought-provoking and suitable. It provided some time for reflection and granted participants a certain power to decide which personal experiences they considered worth sharing and discussing. In the third block, group members were engaged in a storytelling activity. Different keywords were displayed and participants were asked to recount an incident with their CA, or to share their thoughts related to one or more of the presented keywords (for example, “Expectations,” “Communication,” “Daily Routines”). These discussions were rounded off with follow-up questions, often regarding details of specific experiences or how they were emotionally perceived. On average, a focus group lasted 83 minutes.
3.2. Data analysis
Interviews and focus group sessions were audio recorded and led to verbatim transcriptions of 74,144 words. For pragmatic reasons, our dataset did not include narrow transcription details such as loudness, speed or intonation, nor was observation of non-verbal behavior part of the collected data. We developed a codebook including a list of human values (cf. Appendix A) informed by Winkler and Spiekermann (Citation2019) as a priori codes (Creswell, Citation2013, p. 152). ATLAS.ti, a software package designed for the analysis of qualitative data, was used to mark relevant segments and code for value implications and emotional responses. Three coders worked through the material independently and iteratively. The analysis process consisted of three main phases as outlined in .
Along with each coded value, coders identified whether the value was fostered or harmed and whether it was artifact-based or person-based. For example, data protection was coded as artifact-based because it materializes in the artifact, whereas privacy was coded as person-based because it materializes in the person. There were some values that we coded as person-based or artifact-based, depending on the context in which they were brought up by participants. The value “manners,” for example, was artifact-based when a participant recalled Alexa being polite (artifact-based value benefit), but person-based when participants said they used a rude commanding tone when talking to Alexa (person-based value harm).
Emotional reactions were coded using affective codes (Saldaña, Citation2009), which were integrated into the codebook in the form of six basic emotions (love, joy, surprise, sadness, fear and anger) and 25 subordinate emotion prototypes, based on (Shaver et al., Citation1987). Using magnitude coding (Saldaña, Citation2009), two levels of intensity were identified for emotions as well as for values, depending on the participants’ formulations. Utterances like “a little” or “somewhat” indicated low intensity, whereas strong adjectives, such as “a lot,” or “totally,” indicated high intensity. Ensuring a flexible coding process (Creswell, Citation2013), our codebook evolved gradually. The reliability and consistency of the coding process was assessed by measuring Inter-Coder Agreement. Agreement on the interpretation and application of codes lead to a Holsti Index of 82.1%, indicating a broad overlap of coded text segments (Holsti, Citation1969). Krippendorff’s Cu-α coefficient (Krippendorff et al., Citation2016) affirmed an acceptable agreement in assigning value codes, affective and magnitude codes to semantic domains (Cu-⍺ = 0,853). Values were grouped into the six categories of value qualities based on (Desmet & Roeser, Citation2015): object qualities, symbolic qualities, interactive qualities, enabling qualities, relational qualities and identity qualities. We ensured validity of this categorization with a card-sorting exercise (Nawaz, Citation2012). The predefined categories were explained to five subject experts from the fields of value-based engineering, ethics and user-centric design, who individually performed closed card sorts. For further analysis, we stepwise filtered out text segments that were irrelevant to our research questions. Text segments were eliminated that were not coded with at least one value and one emotion. In the next step, we retained only values that appeared together with an emotion at least 10 times, reducing the number of identified values from 48 to 37 (see Appendix B). In the remaining 235 text segments, we identified 768 instances of a value co-occurring with an emotion. For example, a text segment in which a user discussed her surprise about the variety of Alexa’s functions was coded with the emotion “surprise” and the artifact-based values functionality and variety. Although we had coded both basic and subordinate emotion prototypes, we decided to aggregate them during the analysis for better representability. A segment that was coded with a subordinate emotion prototype (e.g., “frustration” or “rage”) was assigned the respective overarching basic emotion type (“anger”). It should be noted, that segments could still be coded with two different basic emotions (for example, “anger” and “surprise”).
3.3. Development of the emotion value assessment
The Emotion Value Assessment is a bubble diagram visualization of users’ emotional perception of value implications. It depicts how often an identified value implication co-occurs with a specific emotional response in the underlying qualitative dataset. For example, the value transparency co-occurred 15 times with “anger” and two times with “fear.” These counts of co-occurrences were organized in four cross tables as outlined in . For our exemplary value transparency, the 15 co-occurrences with “anger” were located in artifact-based value harms. Our participants expressed anger when they found their CA to be non-transparent (for example: the data processing behind the CA is perceived as non-transparent.) “Fear,” on the other hand, co-occurred with transparency in the area of person-based value harms, for example, in a case where a participant feared that she herself would become more transparent as a customer. To account for the level of intensity, we weighed every co-occurrence (xi) that was tagged with an emotion intensity code (ei) or a value intensity code (vi). If a segment was assigned a code for “high intensity,” the corresponding co-occurrence was multiplied by a factor of 2.
If it was assigned a code for “low intensity” code, it was multiplied by a factor of 0.5. Finally, we calculated the sum of weighted co-occurrences (c) as described below.
In our example, two of the 15 co-occurrences of transparency and anger were coded with high emotion intensity, one with low emotion intensity and two with high value intensity. The remaining 10 co-occurrences were not tagged with any intensity code. This lead to a weighted sum of co-occurrences of c= 18.5 for transparency and anger in the artifact-based value harms cross table. The cross tables were then visualized as bubble diagrams and assembled to form the EVA. The bubbles’ sizes represent the weighted sum of co-occurrences (c). Axes are nominally scaled and the values are ordered to reflect the six categories of value qualities determined in the card-sorting exercise. Within these categories, values are ordered by decreasing size of c.
4. Results
The EVA () allows us to understand user-CA interactions from three perspectives.
Emotional response perspective––the EVA creates a nuanced understanding of emotional responses, covering six basic emotions (love, joy, surprise, sadness, fear and anger) and their co-occurrences with specific values.
Value quality perspective––the EVA illustrates differences between the categories of value qualities (object qualities, symbolic qualities, interactive qualities, enabling qualities, relational qualities and identity qualities).
Value-bearer perspective––the EVA contrasts the emotional responses to value harms and benefits for the two value-bearers person and artifact, allowing us to infer differences between the potentials and weaknesses of each value-bearer. This perspective can also reveal situations in which artifact-based values are instrumental in eliciting or harming person-based values.
The EVA creates an overarching and highly abstracted picture of the insights we were able to draw from the dataset. The underlying dataset consisted of quite dense and at times rather unstructured paragraphs, as participants freely discussed their experiences, explained themselves, hinted at contexts, and compared their own experiences to that of other participants. On the one hand, the process of coding and categorizing (i.e. abstracting) the underlying dataset is necessary for generating results that permit us to answer our research questions. On the other hand, it is important to retain a robust connection to participants’ rich verbal accounts so that the results can be interpreted and understood in depth. To achieve an acceptable balance we screened the material carefully to identify appropriate quotes that effectively represented specific codes and at the same time supported the interpretation of our findings.
4.1. Emotional response perspective
Overall, the most prominent emotional response we discovered involved anger-type emotions. Including the subordinate emotions of irritation, frustration and rage, the weighted sum of co-occurrences for anger-type emotions is 294. Of this sum, the overwhelming majority of co-occurrences (c= 284) come under the category of artifact-based value harms, in particular those related to functionality (70), usability (43), reliability (31), simplicity (26) and transparency (19). A typical example of artifact-based value harms eliciting anger was stated by P1: “Simple requests, pronounced clearly and explicitly, are just not understood. That does annoy me sometimes.”
The basic emotion of joy (c= 184, with the subordinates excitement, pleasure, satisfaction, relief and optimism) co-occurred predominantly with person-based value benefits (118). Here, participants particularly brought up joyfully perceived instances of comfort (17.5), quality of life (15), humor (15) playfulness (14) and sense of community (11). Sixty-three co-occurrences were identified in relation to artifact-based value benefits. These joyful episodes were clustered in manifestations of functionalities (18) and usability (11).
With the subordinates curiosity, astonishment and amazement, the basic emotion surprise (c = 155) is a response that can have a positive or negative connotation. It was clustered in artifact-based value harms (73) and in person-based value benefits (57). While, on the side of artifact-based value harms, surprise largely concentrated around episodes of functionality harms (13) and unreliability (13), surprise was related to more diverse values on the side of person-based value benefits. Here, participants were surprised when it came to manifestations of playfulness (9), variety (7), sense of community (6), humor (6) and friendship (3). A possible explanation of this phenomenon could be users’ expectations. Users may expect artifact-based values, and are negatively surprised when they are not present––but they may not expect person-based values, hence they react with positive surprise when these do materialize. This explanation is supported by manifestations of disappointment, the only sadness-type emotion we identified in the data (c= 125). Disappointment was overwhelmingly related to artifact-based value harms (c= 118) and often co-occurred with the same value harms that elicited surprise. P9: “You turn on a skill, then you get trapped in that skill and you can’t quickly, easily exit it. You ask her questions but they are wrongly formulated and at some point, your motivation is just gone.” This segment was coded with disappointment and harms of functionality, usability, speed and simplicity. The applied coding illustrates well how participants, in complaints about task-related errors, often indicated a broad spectrum of values, which were all coded.
The identified fear-type emotions (c= 107, including the subordinates concern, nervousness and doubt) showed up relatively equally distributed among artifact-based value harms (51) and person-based value harms (56). On the side of the CA, fear was a response to perceived harms of transparency (8), reliability (7), data protection (6) and trust (6). Trust was coded as an artifact-based value when participants discussed the trustworthiness of their CA, whereas it was coded as a person-based value when they elaborated on their trust in the CA. On the side of the person, fear was often connected to perceived harms of privacy (10.5) and autonomy (9). The specific value harms that co-occurred with fear show a remarkable coherence. Fear is a typical response when users consider different aspects of privacy. For example, our participants discussed a lack of transparency over where and how long their data might be stored, what it might be used for and whether Amazon as a company could be trusted. They brought up the possibility that the device might permanently record them or listen in on conversations, which were not addressed at the agent. P8: “That is also my greatest concern, […] that she actually always listens and wiretaps me. It might be just a loss of subjective feeling of security.” Participants often noted that this impression was triggered by instances of unprompted agent activations. Discussions about data protection and privacy often quickly evolved and shifted toward the consequences of these value harms, which again co-occurred with fear. A noteworthy example of such a consequence is the fear of being manipulated by the agent (say, into buying specific products), which we coded as a harm of autonomy.
The basic emotion love, or more precisely, its subordinate emotion “affection,” was only elicited by the artifact-based value human-likeness. For example in this instance: “When I imagine Alexa to be human, she is a nice, dark haired, middle-aged woman. She is certainly nice.” (P2). We interpreted this as an instance in which the perceived human-likeness of the CA elicited an affectionate reaction (“she is certainly nice”). The segment suggests that human-likeness can create an affectionate bond between user and agent. Interpreted as such, this finding supports previous studies on agent personification, which argued that perceived human-likeness has impacts on affection, loyalty and trust (e.g., Lopatovska & Williams, Citation2018).
4.2. Value quality perspective
The value quality perspective allows us to analyze and contrast the three groups of qualities (perceiving the object, using the object and social implications) and their subcategories. We see the strongest positive emotional responses in the areas of interactive, enabling, relational and identity qualities. The source of joy and surprise appears to lie in the effects of the technology on users. In the area of interactive qualities, positively perceived effects such as playfulness, diversion, and humor emerge directly when users interact with the CA. Yet, a look into the original data behind joyfully perceived interactive qualities indicated, the positive responses to these qualities were often ephemeral. They were often situated in the early phase of product adoption, and a number of our participants noted that the joyful early phase of playing around soon made room for more sober and goal-oriented interaction patterns. In contrast, we observed that value implications in the areas of enabling, relational and identity qualities appear to be detached from immediate product use and unfold in temporal distance to CA usage. These qualities point to the meaning-level of experiences: they reveal manifold instances in which CA interaction is not pleasant for its own sake; rather, it is pleasant because it affects meaningful areas of users’ lives.
A technology demonstrates enabling qualities when it enables users to perform an activity or to acquire a desirable state. We identified seven distinct values that relate to enabling qualities, i.e., on what the CA enabled participants to do or be (such as comfort, quality of life, knowledge or education). Among enabling qualities, there were very few accounts of value harms. The most discussed value in this group was comfort, which often elicited satisfaction (basic emotion joy). Describing the changes that the CA brought to his life, P13 indicated how the voice control of his TV elicited comfort: “It happens a lot to me that I find the TV advertising too loud but I can’t find the remote control and have to search for it. Now, I am pleased to note that I just do not have such moments anymore.” Instances in which participants detailed how their quality of life has improved mostly co-occurred with joy or a subordinate emotion of joy, such as satisfaction in this statement by participant P12: “Alexa can make it work that the lights slowly power up in the morning, and I am woken perfectly by the lights. The first time you’re not woken abruptly but naturally, without disruption, that is a really unique experience.”
Relational qualities focus on social relationships with other people. What stands out here is that we identified no value harm and no negative emotion in response to relational qualities. This supports the suggestion that relational qualities bear significant potential to create value and elicit positive, memorable experiences. The following quote by P1 underlines this notion: “On Chuck Norris’ birthday, she read a poem to me that contained Chuck Norris catchphrases. I was very surprised. I liked that a lot and even recorded it with my phone and sent it to friends.” Here, we identified a manifestation of the value humor, along with the emotions surprise and joy. Beyond that, we also identified that this participant was happy to share the delightful experience with his peers, fostering the value of friendship. In another case, P3 enthusiastically described how she places her smart speaker on the breakfast table every morning to join the conversation and read stories: “Before I sit down, I say ‘wait a minute, Alexa is still missing!’ Then I go get her from the living room and put her there. Somehow, this is part of the morning […]. Alexa is still missing! Like a little family member.” P3 compared the presence of her CA at the table to that of a family member, and we interpreted this as an elicitation of the value family, which sparked joy.
Identity qualities are values with an impact on users’ self-perception and identity, and they can shape how one is perceived by others. In this group, the value of autonomy in particular was harmed in several instances. For example, participants expressed concern that they would gradually lose basic cognitive skills (such as short-term memory or simple math) because Alexa helped them with calculations and to-do lists. Participant P2 described in detail how his reliance on Alexa for movie recommendations formed the image he had of himself. P2 took pride in the fact that he used to be very active in reading reviews and often discovered new genres and unorthodox productions. But he saw this behavior vanish with the comfort of getting recommendations from the CA. Consuming the agent’s suggested content increasingly passively, his viewing behavior shifted toward less diverse, more mainstream content. “As a user, sooner or later, you will be manipulated by the assistant. Then, it is not an assistant anymore, it’s a dictator. […] It is a gradual process […] at some point, you don’t search or read reviews anymore. You just trust Alexa blindly and watch what it suggests. Alexa dictates to you, simply by saying ‘you might like this.’” Identity qualities underline that technology has an enduring impact on how users behave and develop over time. The functions they use appear to gradually shape their sense of being an active, independent individual, for better or for worse. The quote underlines how an initially welcomed function was increasingly perceived as encroaching, limiting this participant’s sense of autonomy and independence. Yet, we also found a number of well-perceived elicitations of these values, for example, in a statement in which P22 noted that he bought a smart speaker for a relative with reduced mobility: “For her, it is super. She can do things that she never could before.”
4.3. Value-bearer perspective
What becomes visible in the upper sections of the EVA diagrams is that manifestations of person-based values appear to be more differentiated. Person-based value benefits are distributed across all categories of perceiving the object, using the object and considering its social implications. The strong positive emotional response to a variety of person-based value benefits suggests that value manifestations, which unfold in the users themselves, such as their comfort, their sense of humor, their sense of community, and many others, carry the greatest potential for delight and surprise. Hence, they point us to important knowledge about what technology can actually do for the user. When we focus on the two types of value-bearers (artifact or person), the EVA reveals how the CA can become instrumental in unfolding human values in the person. Our data suggests that the object is not perceived as pleasurable per se, yet it carries the potential to unfold person-based values, which can in turn elicit joy. For example, we identified that hands-free operation of the CA is instrumental in unfolding comfort, and it is the comfort that users appreciate and perceive with positive emotions. Similarly, P14 pointed out that the accessibility of the voice interface “clears a hurdle for non-tech savvy people.” This user, however, only expressed a positive emotional response when he discussed how satisfying it was to see the result of this: his parents’ inclusion in the digital world opened up new means of communicating as a family. What elicited emotions was the unfolding of person-based values (family and inclusion). The accessibility was the designed disposition of the CA that supported the materialization of person-based values.
A comparison of the value harms at the bottom of the diagrams suggests that harms are perceived more emotionally when they unfold in the artifact. The large cluster of anger-related emotional responses to artifact-based value harms is based on numerous accounts of participants who routinely interacted with the CA and encountered problems they were not able to solve. Hence, it appears as if the potential to avoid negative emotions and reduce consumer deterrence lies in artifact values. While participants did bring up instances of joyfully perceived artifact-based value benefits, these joyful episodes mostly referred to the early phase of playfully discovering the CA, as noted earlier. Several participants pointed out that this early phase of surprise and joy over different functionalities and features soon made room for episodes of frustration with repeated system failures and communication breakdowns. P2:“In the beginning, it was always funny and interesting to just talk to Alexa […] it was fun to try it out. But […] it was only funny in the beginning. Then, it became a little annoying, because it was less helpful, for example, when Alexa just didn’t recognize the speech. And then, that factor of playing around just declined and I lost interest in it.”
We also found instances in which artifact-based value harms led to harms of person-based values. P7 noted, that she “would like to be able to rely on Alexa” as a reminder and alarm for important appointments. Her CA, however, had once “let [her] down,” when an important alarm never went off and caused her to be late. In this specific experience, the distinction between value-bearers can point us to the meaning of her emotional response of frustration. The unreliability of the agent was instrumental in harming her trust. It also discouraged her from using the CA for important alarms in general, hence preventing a certain quality of life from materializing.
5. Discussion
The EVA meets several challenges, to which common approaches to user experience have provided only limited answers. Nonetheless, the EVA has a unique focus, and there may be many situations where the use of simpler tools is advisable. This discussion therefore puts a focus on the question in which contexts and at what point in the development lifecycle the application of an EVA makes sense. It also provides guidance for the interpretation of an EVA.
5.1. Contributions
Prior UX frameworks have rarely investigated emotions beyond a positive-negative distinction. We responded to this research gap by creating a more granular picture of emotional nuances by applying prototype theory in the construction of the EVA. This made it possible to identify a large spectrum of basic and subordinate emotions that users experience in response to CA usage. The EVA illustrates the complex nature of human emotions and illustrates a number of details that common UX frameworks have had only limited access to (Watson et al., Citation1988). This more elaborate model of human emotions promises to enhance our understanding of human perception of technology.
UX scholars have long called for deeper investigations into the meaning-level of user experiences and suggested that meaning lies in a technology’s ability to support the fulfillment of psychological needs (Hassenzahl, Citation2013). We are addressing the meaning-level of experiences by examining the impact of technology on human values. As human values explicitly include psychological needs, our approach answers to Hassenzahl’s call for the consideration of needs, yet it extends the perspective beyond needs by considering a much broader spectrum of values that guide human attitudes, behavioral choices and preferences. The consideration of human values in HCI can provide deep insights into ethically meaningful potentials and flaws of technology. The EVA illustrates why a product is valued by users, beyond the creation of ephemeral pleasure. We believe that the EVA promotes an understanding of the technological artifact as a set of dispositions that can be instrumental in creating or preventing meaningful experiences.
A majority of UX designers and researchers will be familiar with the fact that the way technology is experienced involves many indirect (or “person-based”) aspects, such as the undermining of users’ privacy. However, most of them may not have a suitable framework like the EVA at hand to conceptualize experiential aspects that go beyond the immediate interaction. In this regard, the EVA appears to close an important conceptual and methodological gap. UX frameworks that focus on user goals and objectives (e.g., Hassenzahl & Roto, Citation2007) provided only limited access to harmful, indirect and temporally delayed aspects of the user experience, such as long-term impacts on a user’s identity. The EVA addresses this limitation by considering a variety of moral, social and personal values that can be positively or negatively implicated. The broader focus on human values that underlies our assessment allows researchers to capture technological impacts––beneficial or harmful, intended and unintended––on what users consider important (“of value”) in life.
Finally, our findings also carry a philosophical contribution: They underline the argument that technology forms the environment in which it is situated. Our study illustrates active negative and positive forming processes driven by technology. Hence, we argue in line with Ihde and Malafouris (Citation2019) that value harms and benefits created by technology actively and significantly shape us as humans, communities and societies.
5.2. How to construct the EVA
The workflow outlined in represents the three relevant phases in the process of data collection and analysis, which is the required methodological foundation of an EVA. To support future researchers and practitioners in actually applying the EVA, it remains worthwhile discussing how exactly the EVA visualization was generated. We worked entirely in MS Excel where we kept the original selection of relevant text segments and the codes applied to each segment. Using dynamic links, we created four cross tables in different worksheets and visualized them as bubble diagrams () using Excel’s standard visualization tools. Here, close attention needs to be paid to the automatic re-adjustment of bubble sizes that Excel applies by default for better readability. To equalize the scaling across the four diagrams, we added a uniform reference value to each cross table, which was located outside of the visible diagram area. In general, we believe there is much potential for trying out other software programs when generating the EVA, for example, the programming language R.
5.3. How to interpret the EVA
To interpret the bubble visualization, we recommend taking into account the three perspectives that an EVA provides (emotional response perspective, value quality perspective and value-bearer perspective). When drawing conclusions based upon the EVA, we suggest returning to the original dataset regularly. The underlying participant quotes are highly important for retaining the contextuality of value manifestations and a reminder of the exact role a product played in harming or fostering selected values. We believe this is one of the most important requirements for interpreting the EVA. While the EVA reveals abstract patterns and relationships, the underlying actual experiences (situated and contextualized) reveal the concretely relevant product dispositions. This bridge is essential in order to use the EVA as a reliable analytical tool for need and requirement elicitation, and to translate its findings into precisely formulated system requirements.
5.3.1. Emotional response perspective
As a first step, we suggest to focus on values with high emotional impact, as those are likely to represent the most important implications for the majority of participants. To interpret these emotional responses precisely, it makes sense to identify typical, re-occurring experiences behind specific emotional responses to both value harms and benefits.
When interpreting the EVA, we also recommend to scrutinize emotional responses related to values with significant ethical importance, for example, values that have been recognized as basic human rights or are explicitly referred to in relevant legal frameworks, such as autonomy, freedom, independence or privacy. Their implications may not always represent the majority of user experiences, yet they carry significant ethical weight, as they affect some of the most profound principles in (western) societies. Again, a return to the original data is recommended to identify typical examples of these impacts on higher values and translate these findings into concrete design requirements. In our CA study, we found that CAs can both harm and strengthen identity qualities, depending on the individual context. Arguably, it should be possible to design CAs in a way that allows users to grow, learn and develop their abilities, without undermining their autonomous decision-making. If CA technology achieves this, it could reach beyond ephemeral hedonic pleasures and create lasting positive impacts on users’ self-perception and confidence.
Finally, there may be emotional responses that are rare, such as the manifestations of fear that we found in our investigation of CAs. A look back into the data can reveal what the experiences that elicit rare emotional responses have in common. For example, we identified that fear occurred especially in relation to perceived harms of privacy, trust and controllability.
5.3.2. Value quality perspective
This perspective allows a comparison of the six value quality groups (i.e. object, symbolic, interactive, enabling, relational and identity qualities). The EVA could reveal clusters of positive or negative emotions in one or more of these groups. Values of higher ethical importance are usually situated in enabling, relational and identity qualities. Whenever positive responses such as joy or affection are particularly dominant in one specific group, this is a conclusive sign that it makes sense to prioritize the development of the product into a direction that supports these specific value quality groups. The underlying experiences can provide hints as to how exactly a product could be adapted to strengthen and multiply such experiences, or to make them available to an even broader group of users. An example from our investigation of CAs can clarify this notion: We found an interesting cluster of joyful responses in the area of relational qualities. Translating this finding into a specific design goal, one might aim to accentuate relational qualities. Future design approaches could equip CAs with more possibilities to foster users’ social relationships with friends, family or other peer groups. Concrete possibilities may range from group games to the (better) integration of messengers, voicemail and call functions, to collaborative functions and easy options for sharing and exchanging content (such as past interactions with Alexa).
The same line of thought can be followed when negative emotional clusters (anger or fear) show up in a specific value quality group. A closer examination of the particular contexts in which the technology has undermined values in this group will shed light on the exact product dispositions, which may need to be reconsidered.
5.3.3. Value-bearer perspective
The comparison of person-based and artifact-based value implications can be helpful to investigate how artifact-based values in a product are at times instrumental for creating meaningful user experiences that create lasting (person-based) value beyond brief pleasure. A look at the value-bearer can reveal how the designed dispositions of a product can help users strive, enjoy their communities and develop individually. Specifically manifestations of person-based values point to the indirect, non-immediate value that a technology elicits and that common UX models still only tap to a limited extent. When looking at the artifact, we found that it makes sense to improve artifact-based values (most importantly functionality, usability, reliability, simplicity and transparency), as they frequently elicit frustration and anger when they are harmed. Yet, when focussing on the person, our results indicate that a strong focus should be put on the functions and applications that foster person-based values, because they appear to be more potent in generating positive emotional responses. Here, technical improvements promise to reduce negative emotional responses while also strengthening and multiplying instances of positive emotional response. Plausible examples of such priority functions are those that feed into the person-based values of playfulness, diversion, humor and sense of community. Designers can translate these indications into concrete design requirements. One might aim to improve usability and reliability specifically for functions that cater to playfulness and humor (such as jokes, group games, quizzes, party functions, functions for children) or that foster comfort and users’ quality of life (for example the seamless compatibility with common third-party applications, devices and services).
At the same time, great attention should be paid to the undermining of important person-based values, particularly in the areas of enabling, identity and relational qualities. Such impacts may not only be ethically questionable, they can develop into serious threats to business models if they materialize repeatedly and become subject of the public discourse (Görnemann & Spiekermann, Citation2022). Again, the question one would need to answer is how the technological artifact needs to be designed in a way that protects ethically meaningful person-based values. An example from our findings can illustrate this consideration: We identified that arbitrary, unsolicited activations of the CA evoked fear of not being able to protect one’s privacy or effectively control the CA. The value-bearer perspective pointed us to the instrumental relationship between artifact-based values (controllability, transparency) and person-based values (privacy, autonomy). This relationship is still relatively abstract, and a look back into the data reveals the precise product disposition responsible for the identified artifact-based value harm (i.e. the unsolicited activation). The interpretation of the value-bearer perspective can thus point to important abstract challenges and opportunities whereas the precise situational context of the underlying user experiences can clarify which product dispositions are the drivers of this experience.
5.4. EVAs in system design and development
An EVA can help fostering a deeper understanding of users’ expectations and experiences. Agile software development is rooted in the idea that a focus on individuals and interactions as well as continuous, close collaboration with customers is crucial to develop technology that is actually human-centered (Highsmith, Citation2010). Development teams that work with agile methods are used to iteratively adapt existing products to external changes. For such teams, the regular use of an EVA can provide insights into potentially important issues, for example, when designers envision new product features, observe the immediate reaction to a new product release or monitor the long-term effects of their product. Applied in such a manner, the EVA could become part of a regular system validation and monitoring, in which value implications and their emotional reception are systematically and regularly assessed. This may help to identify relevant value implications faster and address them early on. Such systematic assessments could also focus on specific user groups or on certain product features to acquire deep insights into the user experience of specific components or certain user groups. The EVA is flexible enough to address such detailed questions: Topics discussed in focus groups can be defined with regard to what specific component the developer team wishes to explore, and specific user groups can be addressed with targeted sampling strategies.
In a more specific context, we believe that EVAs can meaningfully support ethically aligned system development. The IEEE 7000TM “Standard for Addressing Ethical Concerns during System Design” proposes to ethically align system engineering by anticipating potential value harms and value benefits early in the product development cycle (IEEE Standards Association, Citation2021; Spiekermann & Winkler, Citation2020). Relevant potential value manifestations are then translated into concrete technical or organizational system requirements. An EVA not only provides meaningful information on the actual ethical implications of a technology, it also explicitly addresses human values. We therefore believe that, specifically in the context of ethically aligned system development, EVAs could be used to assess the actual value implications on the side of the users. By conducting an EVA, companies could be provided with valuable information on whether their envisioned value propositions are actually materializing, and they could identify potential side effects that were not considered up front. In this context, EVAs could effectively inform the definition and conceptualization of software upgrades or product redesigns. Yet, even in earlier phases of the product development lifecycle, EVAs might play an important role, say, as part of the ideation phase in a typical Design Thinking approach. Design Thinkers approach innovation “by a thorough understanding, through direct observation, of what people want and need in their lives and what they like or dislike about the way particular products are made” (Brown, Citation2008, pp. 84–85). An EVA can provide designers with deep insights into exactly this kind of knowledge. As a fundamentally user-centered approach, EVAs can increase designers’ sensibility for the exact reasons why a specific technology is appreciated or disapproved of. Access to what specific experiences mean for users could help designers generate new ideas and holistically anticipate potential customer value. With their focus on emotional experiences, EVAs could meaningfully complement the set of tools commonly used in Design Thinking, especially in the early ideation phase.
5.5. When is it worthwhile to conduct an EVA?
The EVA is an extensive analysis method that requires time and effort in all phases from data collection to coding and analysis. It is reasonable to address the question in which situations it makes sense to conduct an EVA and when simpler analysis methods might be sufficient. Two criteria can guide this decision: The desired outcome and the technology under investigation.
5.5.1. Decision based on the desired outcome
Common UX frameworks already provide deep insights into directly interaction-related experiences, usability in particular. The outcome of an EVA includes these typical usability issues, as can be derived from harms of values like functionality, usability, reliability or seamlessness. Yet, much simpler heuristic evaluation methods would be sufficient to clearly identify them. If researchers’ aspiration is to capture such immediate, direct interaction patterns, they are well advised to choose simpler methods than the EVA. When designers aim to fix typical system failures and improve usability, it may not be relevant to know which values they relate to or whether these values are artifact-based or person-based. Even users’ emotional responses would not considerably advance designers in addressing system failures and usability.
What the EVA does provide that other UX frameworks typically have limited access to are value qualities outside of the direct interaction. Certainly, most researchers and HCI practitioners will already be familiar with the idea that the way technology is experienced involves many indirect (or person-based) aspects. The EVA provides a suitable framework to identify and conceptualize these dimensions. The assessment provides deep insights into users’ perceptions of a technology, as well as the indirect effects it may have in enabling users and affecting their social lives and their identity. It fosters an understanding of the artifact as an instrument with dispositions that support or inhibit the unfolding of person-based values. In this regard, the EVA can provide practical hints for the design of technology that specifically enhances individual capabilities. The assessment can increase the sensitivity for human values worth protecting and promotes a deeper understanding of the multi-faceted implications of a technology, supporting a highly granular, stakeholder-driven process for eliciting system requirements.
Also, when researchers seek a deeper understanding of emotional user experiences, the EVA allows them to capture a more nuanced range of emotional responses. While joy and pleasure have been part of existing UX frameworks, other distinct emotional responses have received less attention. For example, prior research has called for more focus on fear in particular, which has been argued to be a “powerful factor of user experience” (Kaptelinin, Citation2010, p. 66). Common UX research methods have provided limited access to fear-type responses. The EVA is an adequate analytical tool, which supports need and requirement elicitation based on understanding users’ fears. The assessment can help identify the precise situations that elicit fear and thus merit immediate action.
5.5.2. Decision based on the technology under investigation
We believe that the application of the EVA can be particularly promising for specific technologies and application areas. Primarily, we recommend conducting an EVA for technologies, which may bear a multitude of values. The EVA is especially suitable to address technology that is widely adopted and deeply integrated into users’ personal lives. Versatile technological devices that users invite into their homes or attach to their bodies, and that perform a variety of functions, can bear and influence a greater variety of values. The artifact is exposed to a wider spectrum of situational contexts in which it can implicate many different aspects of users’ lives. When technologies or interface modalities are being assessed which are not as multi-faceted, the application of a simpler framework (for example, a focus on pragmatic and hedonic aspects) may be sufficient and possibly more efficient.
Another interesting application scenario for the EVA is human-like technology (e.g., CAs, avatars or humanoid agents) which comes with a whole new spectrum of potential value implications in the area of technology perception. Embodied or unembodied, conversational agents are equipped with uniquely interactive interface modalities, especially when they can be conversed with in natural spoken language. Here, specifically the perception dimension (which includes values like human-likeness) is interesting. For instance, an EVA can uncover the diverse effects on users that arise when human-like cues are convincingly or inadequately implemented.
Finally, an EVA is a promising tool for the investigation of technology in sensible areas. The assessment can point to indirect impacts on values of higher ethical weight, such as dignity, freedom or autonomy. With this focus, an EVA can be particularly important in contexts where recent technological advances have entered sensible areas of individual lives and societies. Contexts like care homes, educational systems, medical facilities and workplaces merit a detailed account of the indirect impacts of technology on users in need of protection.
6. Challenges and limitations
An EVA is, in many terms, a challenging project and as most UX tools and frameworks it comes with strengths and weaknesses, and has its limitations with regard to what it can explain and capture.
6.1. Generalizability
One of the most important points to make here is that the EVA represents the experiences of a comparably small sample of individuals. This circumstance has several implications concerning the generalizability of results.
Because the user sample is unavoidably smaller in qualitative data collection methods than in quantitative research, great attention should be paid to sample selection. The generalizability may increase with a larger, more representative sample. Yet, basing the EVA on a larger sample would also considerably complicate matters, as more data needs to be collected, coded and analyzed. In addition to this, one might risk equalizing out experiences that are rare but yet important, for example, the experiences of minority user groups, such as users with visual impairments or disabilities. To come to a decision regarding this problem, researchers and practitioners who wish to apply the EVA need to decide upfront, whether their goal is to create technology that is “better for the masses.” If that is the case, equalizing out rare experiences appears to be unproblematic. On the other hand, researchers could try to investigate only the experiences of a specific group of users.
A well-designed sampling strategy is one way to address the question of generalizability. Another solution lies in the interpretation of the EVA itself. An investigation of the most frequent experiences should always be the starting point of the EVA. Yet, we recommend looking beyond frequent experiences and always investigate values with higher ethical significance, even if their implication is rare. Impacts on users’ dignity, freedom, independence, autonomy or privacy should be considered significant per se, and not be at risk of being equalized out due to their rarity. To create technology that is good in an ethical sense, higher values should consequently be addressed with the goal of eliminating situations in which they are harmed and prioritizing their elicitation through the technological artifact. It is one of the key strengths of the EVA to elucidate a technology’s indirect impact on higher human values. In this regard, the assessment can support ethical decision-making and well-considered alignment of design decisions.
There is another aspect of generalizability that is worth discussing at this point, which is that of the set of values that finds its way into the final EVA. The values that play a role in individual user experiences is uniquely dependent on the respective user, the specific use context and the technology that is being assessed. Due to the contextualized nature of human values, every unique use context is potentially associated with a different spectrum of values. Future researchers are therefore well advised to bottom-up elicit relevant values from the collected data, rather than constraining their findings by only applying predefined value lists. The initial set of values that is being considered when investigating the data material should be as broad as possible because limited, predefined values lists will not be conclusive for all contexts.
6.2. Challenges in conducting an EVA
We faced a number of important challenges when we conducted the EVA that future researchers who wish to apply the EVA are likely to be confronted with as well.
We decided to follow a two-pronged data collection strategy by conducting interviews and focus group sessions. This decision was based on reasonable arguments, namely being able to prepare a moderator guide that addresses the right topics in the right order, and with suitable methods. Nevertheless, we believe that the two-step collection method can be reduced to one step, especially when the EVA of a specific technology is conducted repeatedly.
When coding the material, the distinction between artifact-based or person-based is not always straightforward, especially in cases where a value could be assigned to both value-bearers, such as, in our case, the value trust. In addition, the attribution of specific values or emotions to a statement may not always be clear-cut. There may be ambiguity in the underlying values, or in how much their harm or benefit affects the emotional response. To come to a solution, the work with several coders in an iterative, reflexive process seems indispensable. As regularly recommended in qualitative methodological guides, the coding team needs sufficient time and opportunity to revisit the data and discuss its interpretation, so that they progressively acquire a refined focus and a common understanding (cf., (Srivastava & Hopwood, Citation2009)). In essence, the EVA’s capabilities of linking emotional responses to their source and meaning depends on a reasonable, well-considered interpretation of the data.
6.3. Limitations and future research
The EVA has some general limitations and also our specific application case of CA users had shortcomings that need to be addressed to inform future research. The EVA was primarily designed to illustrate how values co-occur with emotional responses, and not how values co-occur with other values. Theoretically, the proposed analysis may allow researchers to identify co-occurrences of different values, but these connections cannot be directly inferred from the EVA illustration. We decided that an illustrative representation that includes this information would become too complex and eventually impractical. Instead, we suggest taking the value-bearer perspective to identify such co-occurrences. This approach did help us find a number of instances where an instrumental relationship between values appears to be present (for example, in the identified link between insufficient controllability of the CA and perceived autonomy harms). Nevertheless, having easier access to this information would be very valuable and it could provide deeper insights into instrumental relationships between artifact-based and person-based values. Future research could certainly go into that direction and aim to illustrate instrumental relationships between different values.
Another aspect the EVA misses in its current form is an illustration of subordinate emotions. Although our coding included subordinate emotions, we decided to narrow down the scope of emotional response types to six basic emotions as our analysis progressed. We took this decision to render the EVA more comprehensible. However, future research could significantly extend the level of detail depicted in the EVA by exploring ways to illustrate a wider spectrum of subordinate emotions. A finer distinction of subordinate emotion prototypes promises to produce valuable insights and further differentiate the dynamic interplay between value qualities and emotional responses.
Regarding our specific assessment of CAs, we feel that a few shortcomings need to be articulated. CAs have certainly evolved since we collected our data in 2018 and the results of the EVA regarding this technology need to be read having this in mind. Most CAs integrate artificial intelligence components, specifically in the domains of Automatic Speech Recognition and Natural Language Understanding. Technical progress of these components has possibly lead to a reduction of value implications that relate to misunderstandings (for example, reliability, simplicity, seamlessness). Also the compatibility with certain third-party applications and smart home appliances is likely to have improved by now, as both the system of interest as well as related third parties have had some time to adjust, update and integrate their services.
Since 2018, the user base of CAs has broadened significantly, and the technology has become much more common in older age groups (Loose et al., Citation2020). Our sample was skewed toward male millennials and the rather large proportion of students in our sample may have had an additional impact on the findings our study was able to produce. A sample that is more representative of the current, actual user base of CAs could create a more accurate picture of the most commonly experienced value-related emotions. Aiming for better representation is one way to adapt the sample structure in a meaningful way. Future research might also aim for a higher diversity sample in terms of demographics and use contexts, for example, by including a larger share of the elderly, families with children, people with disabilities, users with visual impairments, or people of diverse nationalities. This approach promises to create interesting insights because it adds rare perspectives from different life-worlds to the picture. We might expect a more nuanced EVA that depicts rare experiences and a broader spectrum of value implications.
7. Conclusion
Well-established tools for measuring aspects of the user experience mostly fall short of capturing the richness and complexity of value implications and their emotional experience. The EVA allows us to extend the spectrum of system qualities and emotional responses typically considered in UX research in order to account for their complex nature. It is a reusable methodology that invites future researchers to apply this approach to other technologies besides CAs. The meaning that specific interactions with technology have for individual users can deviate significantly from developers’ expectations. Therefore, it makes sense to assess that meaning by applying a method that provides room and flexibility to tackle areas of the user experience that common approaches typically do not capture. Most importantly for future research, the EVA points out that a system’s designed qualities are instrumental for value implications in the person and life of users––effects that, in turn, elicit emotional responses. By disentangling these effects and patterns, the EVA can inform a long-term, strategic setup for the technology development process. It allows developers to take a step back and investigate which qualities of their product translate into meaningful experiences. The EVA can thus foster a more precise judgment of the aspects that merit further strengthening in the future design process. Overall, the goal of technology development should be to precisely identify the direct and indirect impacts in different areas of users’ lives in order to build technology that is––in the ethical sense of the word––good for humans. In times of rapid digitalization and post-phenomenon consideration of ethical consequences, it has become increasingly relevant to deepen our understanding of the user experience and explore the multifaceted effects of technology usage in human lives. Our article illustrates how qualitative research can significantly contribute to the field and extend the understanding of interactional patterns that have often remained overlooked when applying common quantitative models.
Disclosure statement
No potential conflict of interest was reported by the author(s).
References
- Beirl, D., Yuill, N., & Rogers, Y. (2019). Using voice assistant skills in family life. Proceedings of the 2019 CSCL International Conference on Computer Supported Collaborative Learning, 13, 96–103. ACM. https://discovery.ucl.ac.uk/id/eprint/10084820/
- Beneteau, E., Richards, O. K., Zhang, M., Kientz, J. A., Yip, J., & Hiniker, A. (2019). Communication breakdowns between families and Alexa. Proceedings of the 2019 CHI Conference on Human Factors in Computing Systems. ACM. https://doi.org/10.1145/3290605.3300473
- Brown, T. (2008). Design Thinking. Harvard Business Review, 86(6), 84–92. https://doi.org/10.1145/3290605.3300473
- Carey, M. A. (1994). The group effect in focus groups: Planning, implementing, and interpreting focus group research. In J. Morse (Ed.), Critical issues in qualitative research methods (Vol. 225, pp. 41). SAGE.
- Cho, E. (2019). Hey Google, can I ask you something in private? Proceedings of the 2019 CHI conference on human factors in computing systems. ACM. https://doi.org/10.1145/3290605.3300488
- Choi, D., Kwak, D., Cho, M., & Lee, S. (2020). “Nobody speaks that fast!” An empirical study of speech rate in conversational agents for people with vision impairments. Proceedings of the 2020 CHI Conference on Human Factors in Computing Systems. ACM. https://doi.org/10.1145/3313831.3376569
- Clark, L., Munteanu, C., Wade, V., Cowan, B. R., Pantidi, N., Cooney, O., Doyle, P., Garaialde, D., Edwards, J., Spillane, B., Gilmartin, E., & Murad, C. (2019). What makes a good conversation? Proceedings of the 2019 CHI Conference on Human Factors in Computing Systems. ACM. https://doi.org/10.1145/3290605.3300705
- Cohen, P., Cheyer, A., Horvitz, E., El Kaliouby, R., & Whittaker, S. (2016). On the future of personal assistants. Extended abstracts of the 2016 CHI conference on human factors in computing systems. 1032–1037. ACM. https://doi.org/10.1145/2851581.2886425
- Cowan, B. R., Pantidi, N., Coyle, D., Morrissey, K., Clarke, P., Al-Shehri, S., Earley, D., & Bandeira, N. (2017). “What can I help you with?”: Infrequent users’ experiences of intelligent personal assistants. Proceedings of the MobileHCI 2017 International Conference on Human-Computer Interaction with Mobile Devices and Services. ACM. https://doi.org/10.1145/3098279.3098539
- Creswell, J. W. (2013). Qualitative inquiry and research design: Choosing among five approaches (3rd ed.). SAGE.
- Davis, J., & Nathan, L. P. (2015). Value sensitive design: Applications, adaptions, and critiques. In J. van den Hoven, P. E. Vermaas, & I. van de Poel (Eds.), Handbook of ethics, values, and technological design: Sources, theory, values and application domains (pp. 11–40). Springer.
- Desmet, P. M. A. (2012). Faces of product pleasure: 25 positive emotions in human-product interactions. International Journal of Design. 6(2) https://ijdesign.org/index.php/IJDesign/article/view/1190.
- Desmet, P. M. A., & Roeser, S. (2015). Emotions in Design for Values. In J. van den Hoven, P. E. Vermaas, & I. van de Poel (Eds.), Handbook of ethics, values, and technological design: Sources, theory, values and application Domains (pp. 203–218). Springer.
- Druga, S., Williams, R., Breazeal, C., & Resnick, M. (2017). “Hey Google is it ok if I eat you?” Initial Explorations in Child-Agent Interaction. Proceedings of the IDC 2017 conference on interaction design and children. 595–600. ACM. https://doi.org/10.1145/3078072.3084330
- eMarketer. (2018). US Smart Speaker User Penetration, by Demographic, 2018. https://www.emarketer.com/chart/218758/us-smart-speaker-user-penetration-by-demographic-2018-of-population-each-group
- Friedman, B., & Hendry, D. (2019). Value sensitive design: Shaping technology with moral imagination. The MIT Press.
- Fuchsberger, V., Moser, C., & Tscheligi, M. (2012). Values in action (ViA): Combining usability, user experience and user acceptance. In J. A. Konstan (Ed.), Extended abstracts of the 2012 CHI conference on human factors in computing systems. 1793–1798. ACM. https://doi.org/10.1145/2212776.2223711
- Görnemann, E., & Spiekermann, S. (2022). Moments of truth: How rupturing events uncover the value impacts of technology. In S. Roth & H. Corsten (Eds.), Handbuch der Digitalisierung (pp. 73–90). Franz Vahlen.
- Hartmann, N. (1949). Ethik. De Gruyter.
- Hassenzahl, M. (2005). The thing and I: Understanding the relationship between user and product. In M. A. Blythe, K. Overbeeke, A. F. Monk, & P. C. Wright (Eds.), Funology: from usability to enjoyment (pp. 31–42). Springer Science + Business Media Inc.
- Hassenzahl, M. (2013). User experience and experience design. The Encyclopedia of Human-Computer Interaction. Interaction Design Foundation. https://www.interaction-design.org/literature/book/the-encyclopedia-of-human-computer-interaction-2nd-ed/user-experience-and-experience-design
- Hassenzahl, M., & Roto, V. (2007). Being and doing - a perspective on user experience and its measurement. Interfaces, 72, 10–12. https://citeseerx.ist.psu.edu/viewdoc/summary?doi=10.1.1.146.3362
- Highsmith, J. A. (2010). Agile project management: Creating innovative products. Addison-Wesley; Safari Books Online.
- Holsti, O. R. (1969). Content analysis for the social sciences and humanities. Addison-Wesley Pub. Co.
- IEEE Standards Association. (2021). IEEE 7000 Standard model process for addressing ethical concerns during system design. ( IEEE 7000-2021). IEEE Computer Society. https://standards.ieee.org/ieee/7000/6781/
- Ihde, D., & Malafouris, L. (2019). Homo faber revisited: Postphenomenology and material engagement theory. Philosophy & Technology, 32(2), 195–214. https://doi.org/10.1007/s13347-018-0321-7
- Jiang, J., Jeng, W., & He, D. (2013). How do users respond to voice input errors? Lexical and phonetic query reformulation in voice search. Proceedings of the 2013 SIGIR conference on Research and development in information Retrieval. 143–152. ACM. https://doi.org/10.1145/2484028.2484092
- Kaptelinin, V. (2010). Facing the dark side of life: The challenge of addressing users’ fears in interaction design. Proceedings of the 2010 CREATE international conference on The Interaction Design. 66–67. BCS Learning & Development Ltd.
- Kelly, E. (2011). Material ethics of value: Max Scheler and Nicolai Hartmann. Springer.
- Keltner, D., & Gross, J. J. (1999). Functional accounts of emotions. Cognition & Emotion, 13(5), 467–480. https://doi.org/10.1080/026999399379140
- Kiseleva, J., Williams, K., Jiang, J., Hassan Awadallah, A., Crook, A. C., Zitouni, I., & Anastasakos, T. (2016). Understanding user satisfaction with intelligent assistants. Proceedings of the 2016 CHIIR Conference on Human Information Interaction and Retrieval. 121–130. ACM. https://doi.org/10.1145/2854946.2854961
- Kluckhohn, C. (1962). Values and value orientations in the theory of action: An exploration in definition and classification. In T. Parsons, E. A. Shils, & N. J. Smelser (Eds.), Toward a General Theory of Action (pp. 388–433). Transaction Publishers.
- Koksal, I. (2018). Who’s the Amazon Alexa target market, anyway? Forbes Magazine. https://www.forbes.com/sites/ilkerkoksal/2018/10/10/whos-the-amazon-alexa-target-market-anyway
- Krippendorff, K., Mathet, Y., Bouvry, S., & Widlöcher, A. (2016). On the reliability of unitizing textual continua: Further developments. Quality & Quantity, 50(6), 2347–2364. https://doi.org/10.1007/s11135-015-0289-7
- Kroes, P., & Verbeek, -P.-P. (2014). The moral status of technical artefacts. Springer.
- Kuzminykh, A., Sun, J., Govindaraju, N., Avery, J., & Lank, E. (2020). Genie in the bottle: Anthropomorphized perceptions of conversational agents. Proceedings of the 2020 CHI conference on human factors in computing systems. ACM. https://doi.org/10.1145/3313831.3376665
- Langford, J., & McDonagh, D. (2003). Focus group tools. In J. Langford & D. McDonagh (Eds.), Focus groups: Supporting effective product development (pp. 166–218). Taylor & Francis.
- Lau, J., Zimmerman, B., & Schaub, F. (2018). Alexa, are you listening? Privacy perceptions, concerns and privacy-seeking behaviors with smart speakers. Proceedings of the ACM on human-computer interaction. ACM. https://doi.org/10.1145/3274371
- Loose, N., Spearman, J., & Gewiese, J. (2020). Statista global consumer survey: Smart speaker: Amazon Echo (Alexa) brand report (Österreich) 2020. https://de.statista.com/statistik/studie/id/80314/dokument/smart-speaker-amazon-echo-alexa-brand-report-oesterreich/
- Lopatovska, I., Rink, K., Knight, I., Raines, K., Cosenza, K., Williams, H., Sorsche, P., Hirsch, D., Li, Q., & Martinez, A. (2018). Talk to me: Exploring user interactions with the Amazon Alexa. Journal of Librarianship and Information Science, 51(4), 984–997. https://doi.org/10.1177/0961000618759414
- Lopatovska, I., & Williams, H. (2018). Personification of the Amazon Alexa. Proceedings of the 2018 CHIIR Conference on Human Information Interaction & Retrieval. 265–268. ACM. https://doi.org/10.1145/3176349.3176868
- Lovato, S., & Piper, A. M. (2015). “Siri, is this you?”: Understanding young children’s interactions with voice input systems. Proceedings of the 2015 IDC International Conference on Interaction Design and Children. 335–338. ACM. https://doi.org/10.1145/2771839.2771910
- Luger, E., & Sellen, A. (2016). “Like having a really bad PA”: The gulf between user expectation and experience of conversational agents. Proceedings of the 2016 CHI Conference on Human Factors in Computing Systems. 5286–5297. ACM. https://doi.org/10.1145/2858036.2858288
- Mahlke, S., & Thüring, M. (2007). The diversity of non-instrumental qualities in human-technology interaction. Mmi Interaktiv, 13, 55–64. http://mmi-interaktiv.de/uploads/media/05-mahlke_et_al.pdf
- Marshall, M. N. (1996). Sampling for qualitative research. Family Practice, 13(6), 522–526. https://doi.org/10.1093/fampra/13.6.522
- Meliones, A., & Maidonis, S. (2020). DALÍ: A digital assistant for the elderly and visually impaired using Alexa speech interaction and TV display. Proceedings of the 2020 PETRA International Conference on Pervasive Technologies Related to Assistive Environments. ACM. https://doi.org/10.1145/3389189.3397972
- Moorthy, A., & Vu, K. (2015). Privacy concerns for use of voice activated personal assistant in the public space. International Journal of Human-Computer Interaction, 31(4), 307–335. https://doi.org/10.1080/10447318.2014.986642
- Moussawi, S. (2018). User experiences with personal intelligent agents: A sensory, physical, functional and cognitive affordances view. Proceedings of the 2018 CPR Conference on Computers and People Research. 86–92. ACM. https://doi.org/10.1145/3209626.3209709
- Myers, C., Furqan, A., Nebolsky, J., Caro, K., & Zhu, J. (2018). Patterns for how users overcome obstacles in voice user interfaces. Proceedings of the 2018 CHI conference on human factors in computing systems. ACM.
- Nasirian, F., Ahmadian, M., & Lee, O.-K. (2017). AI-based voice assistant systems: Evaluating from the interaction and trust perspectives. Proceedings of the 2017 AMCIS Americas Conference on Information Systems. Association for Information Systems. AIS. https://aisel.aisnet.org/amcis2017/AdoptionIT/Presentations/27/
- Nass, C., & Gong, L. (2000). Speech interfaces from an evolutionary perspective. Communications of the ACM, 43(9), 36–43. https://doi.org/10.1145/348941.348976
- Nass, C., & Moon, Y. (2000). Machines and mindlessness: Social responses to computers. Journal of Social Issues, 56(1), 81–103. https://doi.org/10.1111/0022-4537.00153
- Nass, C., Steuer, J., & Tauber, E. R. (1994). Computers are social actors. Proceedings of the 1994 CHI Conference on Human Factors in Computing Systems. 72–78. ACM. https://doi.org/10.1145/191666.191703
- Nawaz, A. (2012). A comparison of card-sorting analysis methods. Proceedings of the 2012 APCHI Asia pacific conference on Computer human interaction. 583–592. ACM.
- Nguyen, Q. N., & Sidorova, A. (2017). AI capabilities and user experiences: A comparative study of user reviews for assistant and non-assistant mobile apps. Proceedings of the 2017 AMCIS Americas Conference on Information Systems. AIS.
- Nurkka, P. (2008). User experience evaluation based on values and emotions Pre-print article. Tampere University of Technology]. Citeseerx. http://citeseerx.ist.psu.edu/viewdoc/download?doi=10.1.1.177.7230&rep=rep1&type=pdf
- Perlaviciute, G., Steg, L., Contzen, N., Roeser, S., & Huijts, N. (2018). Emotional responses to energy projects: Insights for responsible decision making in a sustainable energy transition. Sustainability, 10(7), 2526. https://doi.org/10.3390/su10072526
- Plutchik, R. (1980). Emotion, a psychoevolutionary synthesis. Harper & Row.
- Porcheron, M., Fischer, J. E., Reeves, S., & Sharples, S. (2018). Voice interfaces in everyday life. Proceedings of the 2018 CHI conference on human factors in computing systems. ACM. https://doi.org/10.1145/3173574.3174214
- Portela, M., & Granell-Canut, C. (2017). A new friend in our smartphone? Observing interactions with chatbots in the search of emotional engagement. Proceedings of the 2017 interacción international conference on human computer interaction. ACM. https://doi.org/10.1145/3123818.3123826
- Purington, A., Taft, J. G., Sannon, S., Bazarova, N. N., & Taylor, S. H. (2017). “Alexa is my new BFF”. Extended Abstracts of the 2017 CHI conference on human factors in computing systems. ACM. https://doi.org/10.1145/3027063.3053246
- Reeves, S., Porcheron, M., & Fischer, J. (2018). ‘This is not what we wanted’: Designing for conversation with voice interfaces. Interactions, 26(1), 46–51. https://doi.org/10.1145/3296699
- Reynolds, T. J., & Olson, J. C. (2001). Understanding consumer decision making: The means-end approach to marketing and advertising strategy. Erlbaum.
- Rosch, E. (1975). Cognitive representations of semantic categories. Journal of Experimental Psychology. General, 104(3), 192. https://doi.org/10.1037/0096-3445.104.3.192
- Rzepka, C. (2019). Examining the use of voice assistants: A value-focused thinking approach. Proceedings of the 2019 AMCIS Americas Conference on Information Systems. AIS. https://aisel.aisnet.org/amcis2019/human_computer_interact/human_computer_interact/20
- Saariluoma, P., & Jokinen, J. P. P. (2014). Emotional dimensions of user experience: A user psychological analysis. International Journal of Human-Computer Interaction, 30(4), 303–320. https://doi.org/10.1080/10447318.2013.858460
- Sah, Y. J., & Peng, W. (2015). Effects of visual and linguistic anthropomorphic cues on social perception, self-awareness, and information disclosure in a health website. Computers in Human Behavior, 45, 392–401. https://doi.org/10.1016/j.chb.2014.12.055
- Saldaña, J. (2009). The coding manual for qualitative researchers. SAGE.
- Scheler, M. (1973). Formalism in ethics and non-formal ethics of values: A new attempt toward the foundation of an ethical personalism. Original work published in 1921. Northwestern University Press.
- Shaver, P., Schwartz, J., Kirson, D., & O’Connor, C. (1987). Emotion knowledge: Further exploration of a prototype approach. Journal of Personality and Social Psychology, 52(6), 1061–1086. https://doi.org/10.1037//0022-3514.52.6.1061
- Sheth, J. N., Newman, B. I., & Gross, B. L. (1991). Why we buy what we buy: A theory of consumption values. Journal of Business Research, 22(2), 159–170. https://doi.org/10.1016/0148-2963(91)90050-8
- Spiekermann, S. (2016). Ethical IT innovation: A value-based system design approach. Routledge, Taylor & Francis Group.
- Spiekermann, S., & Winkler, T. (2020). Value-based engineering for ethically aligned design. Arxiv: https://arxiv.org/pdf/2004.13676.
- Srivastava, P., & Hopwood, N. (2009). A practical iterative framework for qualitative data analysis. International Journal of Qualitative Methods, 8(1), 76–84. https://doi.org/10.1177/160940690900800107
- Statista. (2020). Statista global consumer survey: Welchen smart-speaker mit integriertem Assistenten besitzen Sie? [Statista global consumer survey: Which smart speaker with integrated assistant do you own?]. Statista. https://de.statista.com/prognosen/1000260/oesterreich-beliebteste-smart-speaker-marken
- Steinert, S., & Roeser, S. (2020). Emotions, values and technology: Illuminating the blind spots. Journal of Responsible Innovation, 7(3), 298–319. https://doi.org/10.1080/23299460.2020.1738024
- van de Poel, I., & Kroes, P. (2014). Can Technology Embody Values? In P. Kroes & -P.-P. Verbeek (Eds.), Philosophy of engineering and technology. the moral status of technical artefacts (pp. 103–124). Springer.
- Verbeek, -P.-P. (2008). Morality in design: Design ethics and the morality of technological artifacts. In P. Kroes, P. E. Vermaas, A. Light, & S. A. Moore (Eds.), Philosophy and design: From engineering to architecture (pp. 91–103). Springer.
- Watson, D., Clark, L. A., & Tellegen, A. (1988). Development and validation of brief measures of positive and negative affect: The PANAS scales. Journal of Personality and Social Psychology, 54(6), 1063–1070. https://doi.org/10.1037//0022-3514.54.6.1063
- Winkler, T., & Spiekermann, S. (2019). Human values as the basis for sustainable information system design. IEEE Technology and Society Magazine, 38(3), 34–43. https://doi.org/10.1109/MTS.2019.2930268
- Wulf, L., Garschall, M., Himmelsbach, J., & Tscheligi, M. (2014). Hands free - care free: Elderly people taking advantage of speech-only interaction. Proceedings of the 2014 NordiCHI Nordic Conference on Human-Computer Interaction. 203–206. ACM. https://doi.org/10.1145/2639189.2639251
- Xu, Y., & Warschauer, M. (2020). What are you talking to? Understanding children’s perceptions of conversational agents. Proceedings of the 2020 CHI conference on human factors in computing systems. ACM. https://doi.org/10.1145/3313831.3376416
- Yang, X., Aurisicchio, M., & Baxter, W. (2019). Understanding affective experiences with conversational agents. Proceedings of the 2019 CHI conference on human factors in computing systems. ACM. https://doi.org/10.1145/3290605.3300772
- Zamora, J. (2017). I’m sorry, Dave, I’m afraid I can’t do that: Chatbot perception and expectations. Proceedings of the 2017 HAI International Conference on Human Agent Interaction. 253–260. ACM. https://doi.org/10.1145/3125739.3125766
Appendix A
A-priori list of values in codebook
Appendix B
Final list of identified values and assignment to categories
Appendix C
Recruited sample detailS
Appendix D
Moderator guide