Abstract
The dopamine D2 Receptor (D2R) is a member of the G-Protein-Coupled Receptor family and plays a critical role in neurotransmission activities in the human brain. Dysfunction in dopamine receptor signaling may lead to mental health illnesses such as schizophrenia and Parkinson’s disease. D2R is the target protein of the commonly used antipsychotic drugs such as risperidone, clozapine, aripiprazole, olanzapine, ziprasidone, and quetiapine. Due to their significant side effects and non-selective profiles, the discovery of novel drugs has become a challenge for researchers working in this field. Recently, our group has focused on the interactions of these drug molecules in the active site of the D2R using different in silico approaches. We here compare the performances of different approaches in estimating the drug binding affinities using quantum chemical approaches. Conformations of drug molecules (ligands) at the binding site of the D2R taken from the preliminary docking studies and molecular dynamics simulations were used to generate protein–ligand interaction models. In a first approach, the BSSE-corrected interaction energies of the ligands with the most critical amino acid Asp114 and with the other amino acids closest to ligands in the binding cavity were calculated separately by density functional theory method in implicit water environment at the M06-2X/6-31 g(d,p) level of the theory. In a second approach, ligand binding affinities were calculated by taking into consideration not only the interaction energies but also deformation and desolvation energies of ligands with surrounding amino acid residues, in a radius of 5 Å of the protein-bound ligand. The quantum mechanically obtained results were compared with the experimentally obtained binding affinity values. We concluded that although H-bond interactions of ligands with Asp114 are the most dominant interaction in the binding site, if van der Waals and steric interactions of ligands which have cumulative effect on the ligand binding are not included in the calculations, the interaction energies are overestimated.
Introduction
G-protein-coupled receptors (GPCRs), also called as seven trans-membrane (7TM) proteins, constitute a large membrane protein group (Rompler et al., Citation2007). All GPCRs share the similar structural topology of seven TM helices into the lipid bilayer, six extra- and intra-membrane loop domains connecting the TMs, and an unstable short helical domain (helix VIII) (Trzaskowski et al., Citation2012). These 7TM receptors correspond to the receptor protein signal transduction pathway across the cell membrane via different receptor activation states, including agonist accommodation into the binding cavity, a conformational transition (particularly in cytoplasmic side of TM5 and TM6), and guanine nucleotide-binding proteins (G-protein) coupling to the cytoplasmic side of protein (Rasmussen et al., Citation2011). GPCR activation can be regulated by the different concentrations of agonist and antagonist compounds in the human body. Approximately 30% of all approved drugs bind to GPCRs and act either as inhibitors or as agonists. Thus, elucidation of structural and dynamical behaviors of GPCRs in their ligand binding sites is crucial for the studies focusing on the discovery of novel and highly potent GPCRs antagonists or agonists (Lagerström & Schiöth, Citation2008). Because of their membrane incorporation, protein structure determination by experimental techniques such as X-ray diffraction, electron microscopy, and NMR is still challenging (Daulat, Maurice, & Jockers, Citation2009). There are five sub-types of dopamine receptors, D1, D2, D3, D4, and D5 (Seeman, Lee, Chau-Wong, & Wong, Citation1976). The D1 and D5 types constitute D1-like family, whereas D2, D3, and D4 belong to D2-like family (Creese, Burt, & Snyder, Citation1976). Also, there are some reports that suggest the existence of additional D6 and D7 receptors, but these evidences were not clearly determined yet (Contreras et al., Citation2002). Dopamine is the primary D2R agonist in human body. This small molecule is as a neurotransmitter which belongs to the catecholamine and phenethylamine families which have a main role in the human brain (Björklund & Dunnett, Citation2007). Dysfunction of the dopamine receptor causes schizophrenia (including adolescent schizophrenia) and Parkinson’s disease which is associated with the level of dopamine in brain (Seeman, Lee, Chau-Wong, & Wong, Citation1976). The second-generation atypical antipsychotic (AAP) drugs include, risperidone, aripiprazole, ziprasidone, clozapine, olanzapine, and quetiapine which are mainly prescribed to treat mental health illnesses such as schizophrenia, bipolar disorder, and autism, and act as antagonists to the D2R (Newcomer, Citation2005). The 2D chemical structures of these drug molecules are shown in Figure .
In the current study, we refine the small molecule binding poses derived from docking and molecular dynamics (MD) trajectory frames in order to obtain an accurate ligand binding energy to the D2R models obtained in our earlier studies (Durdagi, Salmas, Stein, Yurtsever, & Seeman, Citation2016; Salmas, Yurtsever, & Durdagi, Citation2016; Salmas, Yurtsever, Stein, & Durdagi, Citation2015; Salmas et al., Citation2017a, 2017b). The D2R state models were generated by the use of comparative modeling approaches based on the inactive beta-2 adrenergic receptor (β2 adrenoreceptor) as a template structure (Hanson et al., Citation2008). Since we are investigating the antagonizing binding of molecules to D2R, the inactive form of the D2R was used (Salmas et al., Citation2015). Here, the binding of six marketed antipsychotic drug molecules into the catalytic domain of the D2R was studied quantum mechanically (QM) using two different approaches. For calculating accurate small molecule–receptor binding energies, the inclusion of dispersion effects is essential because of the weak non-covalent interactions in the system. The Minnesota functional MO6-2X is a newly parameterized functional which accounts for weak van der Waals dispersion interactions (Grimme, Citation2011). This functional was shown to perform best when calculating the relative energies of zwitterionic small clusters and H-bonded systems (Walker, Harvey, Sen, & Dessent, Citation2013). We here use this functional to calculate interaction energies of known D2R antagonists (Figure ) with the amino acids in the binding site of the target protein to assess the performance of QM approaches based on the frozen docking and MD conformations of drugs and surrounding amino acids. In the first approach, the BSSE-corrected interaction energies of the ligands with the most critical amino acid Asp114 and also with the other amino acids with spatial proximity of ligands in the binding cavity were calculated separately by DFT method in implicit water environment at the M06-2X/6-31 g(d,p) level of the theory. In the second approach, ligand binding affinities were calculated by taking into consideration not only the interaction energies with surrounding amino acid residues in a radius of 5 Å but also the deformation and desolvation energies of ligands. The energies presented here are mostly ligand binding enthalpies and entropic contributions are not explicitly considered. Determination of protein–ligand free energies based on both MM and QM approaches has been discussed in detail in the literature (Ryde & Soderhjelm, Citation2016). The importance of large basis sets and inclusion of dispersion corrections, polar and non-polar solvation, and entropy in the calculations of protein–ligand interaction energies are highlighted (Ryde & Soderhjelm, Citation2016). Recently, a new and faster (than HF-3c) semi-empirical method, PBEh-3c developed by Grimme’s group, was employed to evaluate all the contributions to the energy such as interaction energy and solvation energy in the active site containing about 1000 atoms on activated serine protease factor X (FXa) and tyrosine-protein kinase 2 (TYK2) targets as a test case (Ehrlich, Göller, & Grimme, Citation2017). Muddana and Gilson, reported mining minima (M2) approach together with a semi-empirical quantum mechanical energy function PM6-DH + in implicit solvent for computation of binding affinities of small host–guest complexes (Muddana & Gilson, Citation2012).
Methods
Homology modeling
The X-ray structure of the beta-2 adrenergic receptor in the inactive form was retrieved from Protein Data Bank server (PDB ID: 3D4S) and employed as a template to predict the 3D of the unsolved D2R structure. The amino acid sequence of D2R (UniProt ID: P14416) was obtained from the UniProt database (Apweiler, Citation2004). The sequence was modified by removing the ICL3 domain residues and only residues in the vicinity of TM5 and TM6 were kept. ClustalW (Chenna et al., Citation2003) method was used to align the amino acid residues of template and target. A combination of MODELLER (Eswar et al., Citation2007) and the ROSETTA loop-modeling protocol (Das & Baker, Citation2008) was performed to build and refine the 3D structure of D2R. The details of the modeling process were explained in our previous work (Salmas et al., Citation2015).
Ligand preparation
Two-dimensional structures of all antipsychotic drug molecules were generated using Marvin Sketch. The structures of all ligands were optimized using molecular mechanics (MM) and the OPLS 2005 force field. Then, the protonation states were adjusted to the physiological pH of 7.4.
Induced fit docking (IFD)
Due to the absence of a D2R co-crystallized structure with any of the drug molecules used in this study, induced fit docking protocol (IFD) (Sherman, Day, Jacobson, Friesner, & Farid, Citation2006) was employed. The IFD procedure involves: (i) docking of all ligands into the generated receptor model, (ii) refining of amino acid residues within 4 Å of docked poses, and (iii) re-docking of docked ligands into the refined protein binding cavity.
MD simulations
All MD simulations were performed with NAMD 2.9 (Nelson et al., Citation1996) using the CHARMM36 (Huang & MacKerell, Citation2013) force field for the protein and lipid atoms. The ParamChem server (Ghosh et al., Citation2011) (version 0.9.7.1) was used to generate parameters for the ligands. The SHAKE algorithm was applied to fix the bonds involving hydrogen atoms. The systems were equilibrated at 310 K and 1 bar in isothermal–isobaric (NPT) ensemble. The long-range electrostatic interactions of the systems were calculated using the Particle Mesh Ewald (PME) method within a cut-off distance of 10 Å. 100 ns MD simulations were carried out as a production run for each system under consideration. The details of the MD simulations, minimization, and also the equilibration process were described in detail in our previous study (Salmas et al., Citation2016). A 3D schematic view of the initial constructed system for the MD simulation is represented in Figure , in which risperidone–D2R complex was embedded in a membrane bilayer.
QM calculations
DFT calculations were performed using Gaussian09 (Frisch et al., Citation2016). The M06-2X functional (Zhao & Truhlar, Citation2008) with a 6-31G(d,p) basis set (Ditchfield, Hehre, & Pople, Citation1971) and the ‘counterpoise’ keyword (Boys & Bernardi, Citation1970) were used to calculate the single point, basis set superposition error-corrected interaction energies between the drug molecules and the protein. As an initial structure, we have used the coordinates of the lowest energy docking pose. The 2D ligand–protein interaction maps obtained from the docking studies clearly showed the residues interacting with ligands in the active site and the types of interactions. Binary interaction energies between the ligands and amino acid residues taking part in the active cavity were calculated. To do this, the amide bonds between the amino acids were removed and the missing valence atoms of the terminal N or C atoms were saturated by hydrogen atoms. The initial coordinates of amino acids and ligands taken either from docking or MD poses were preserved. The interaction energies between ligands and amino acids were calculated in gas phase and also in water using IEFPCM solvation model with a permittivity of 78. In the second part of the QM calculations, a different route was followed to calculate the ligand binding affinities. All the single point QM energies of protein-embedded docked and MD-refined poses of the ligands were computed with Turbomole v.6.5 (Citation1989–2007) using the def2-TZVP basis set (Weigend, Furche, & Ahlrichs, Citation2003) in vacuo and in an implicit solvent by employing the COSMO solvent model (Klamt & Schüürmann, Citation1993) with ε = 78. In addition to interaction energies, the contribution of desolvation and deformation energies was also calculated and included in the final binding affinities. The ligand desolvation energy is the energy required to remove the aqueous solvent from the ligand before approaching the protein binding site. The deformation energy is the energy difference between the docked conformation and the fully relaxed optimized structure. To assess the impact of the initial structure on the energies, the lowest energy docking poses were subjected to MD simulation studies, and all the energy calculations were repeated with the coordinates obtained from the MD equilibrium structures of protein–ligand systems.
Results and discussion
The binding poses, electron densities of the ligands as well as the important amino acid residues interacting with them in the active site of D2R are illustrated in Figure , in which the color mapping presents the different types of atoms for the ligands. We observed that Asp114 is the conserved amino acid in all of the bioactive conformations; it forms H-bond with the protonated nitrogen atoms of the ligands. In addition to Asp114, other key amino acids around the ligands, contributing to the non-polar and π–π stacking interactions, are described together with their stable rotations toward the compounds. The data from the 3D schematic views suggested that all the ligands were well accommodated not only by the charged amino acids, but also by the non-polar residues, which are essential to the inhibition process of D2R. It is obvious that the shape of the active sites is different for each of the complexes, which may be linked to the type and conformation of each ligand, and causes them to be categorized into two extended and bulky types of D2R inhibitors. Hence, in the first part of the study, only the interaction energy between Asp114 and the ligands was calculated according to the Equation 1 and summarized in Table .(1)
Figure 3. 3D structures of the compounds and their surfaces at the binding site of D2R. The amino acids interacting with the drugs are shown.
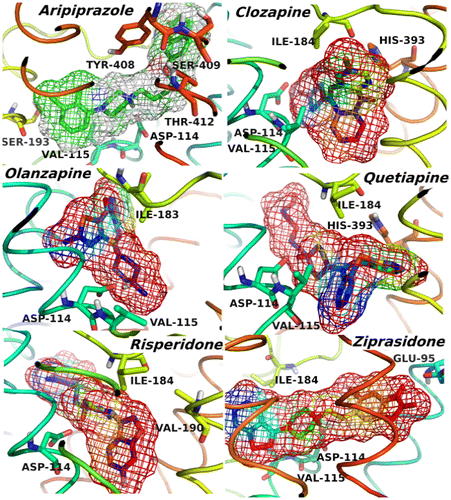
Table 1. Comparison of calculated ligand binding energies with the experimental results.
In Figure , the calculated interaction energies between the drugs and Asp114 using representative structures obtained from the docking and MD simulations were compared to the experimental binding energies. The chemical structures of ligands and hydrogen bonds they form with the Asp114 residue are shown in Figure . It should be noted that Class-I ligands have bulky structures, whereas Class-II ligands have more linear or extended structures. Calculated interaction energies and experimental binding energies showed similar trends and the H-bond is slightly stronger for class-II ligands. The energy differences calculated (using the initial positions of atoms taken from the preliminary MD simulations) for class-I and class-II ligands are more pronounced when compared to experimental energies. Small molecule–receptor binding is dominated by opposite effects of enthalpy and entropy. Here, only enthalpy contributions were calculated. The contributions of the other residues to the ligand binding were neglected. In order to understand total effects of all residues in the binding cavity on the interaction energy, the DFT calculations were repeated for larger protein–ligand complexes. The lowest energy docking poses were used as initial configuration of the ligands and the amino acid residues present in a radius of 5 Å around the ligand. The hydrogen atom positions were re-optimized at BP86/SVP level of the theory. Then, single point energies of the protein–ligand complex, protein, and ligand separately were calculated at M06-2X/TZVP level in the gas and finally in a COSMO implicit water with ε = 78.
Figure 4. Calculated interaction energies between the drugs and Asp114 using representative structures obtained from the docking and MD simulations. The experimental binding affinities between the drugs and D2R are also given to show more clearly the role of Asp114 in the active site.
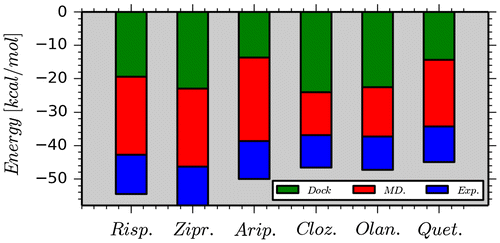
Table gives the QM interaction energies of docked and MD-refined poses. The desolvation energy is largest for aripiprazole (63.6 kcal/mol) due to its extended structure. The desolvation energies of the remaining other five compounds are very close and in a narrow range varied from 54 to 59 kcal/mol. This originates from the highly similar backbone structure of the medicinal compounds and a very close dipole moment. The ligand deformation energy is the largest for quetiapine (35.2 kcal/mol), followed by risperidone (28.3 kcal/mol). This energy has to be overcome for the ligand to adopt the conformation in the protein–ligand complex. The total protein ligand binding energy has to be corrected for the ligand desolvation and deformation energies.
Table 2. Desolvation, deformation, and binding energies and ligand affinities.
The overall ligand binding affinity of the docked poses is significantly overestimated when using single conformers after docking only without any structural refinements (−50 to −84 kcal/mol) compared to experiment (−12 to −10 kcal/mol). The protein–ligand complexes after MD refinement allow for structural adjustment of both the ligand structure and the protein binding pocket. The QM-calculated ligand binding affinities for MD-refined poses are significantly lower (−15 to −8 kcal/mol) and in a much better agreement with experimental binding energies. The deviation from experiment, however, is not systematic and of the order of 1–5 kcal/mol which does allow a clear discrimination between strong and weak binding ligands. In MD simulations, conformational sampling and generation of ensemble averages to obtain thermodynamic properties at equilibrium is the main goal. The correct and representative selection of conformational ligand states from MD trajectories needs to be further explored in order to improve the QM-calculated protein–ligand binding affinities.
Per-residue interaction analysis
Six antipsychotic drugs in the catalytic domain D2R were subjected to energetic assay in order to determine the enthalpic contribution of each amino acid to the non-bonded energies. The residues within 4 Å of the ligand were incorporated into the analysis. Figure illustrates the interaction enthalpies between the active site amino acids (except Asp114) and D2R inhibitors. The Asp114 located in the TM3 plays a very important role as a conserved amino acid in the ligand binding domain of the all complexes since it forms strong H-bond interaction with the protonated nitrogen atoms of the compounds. It is known that Asp114 plays a pivotal role in the inhibition mechanism of the D2R; all the D2R inhibitors should tightly bind to this critical amino acid via hydrogen bonds or salt bridges (Javitch, Li, Kaback, & Karlin, Citation1994; Shaikh et al., Citation1994). In the following section, the binding interactions of each ligand were analyzed individually.
Aripiprazole: This antagonist is a member of the class-II D2R inhibitors because of its extended conformation. Asp114 strongly interacts with this ligand in the 7TM domain, as expected. Relative enthalpy of ligand binding was predicted to be −13.63 kcal/mol. The other amino acids which critically participated in the active site formation were Val115 (TM3), Ser409 (TM7), and Tyr412 (TM7). The TM3 and TM7 domains were significantly involved in the accommodation and also stabilization of the inhibitor in the binding pocket as suggested by the previous experimental studies (Fu, Ballesteros, Weinstein, Chen, & Javitch, Citation1996; Javitch et al., Citation1994). Clozapine: This compound has a bulky structure and is known as a class-I antagonist. The Asp114 forms strong hydrogen bond with this ligand. The enthalpy of ligand binding was found to be −24.01 kcal/mol. Ile184 and His393 were the other main amino acids, interacting weakly with the ligand in the active site. The His393 residue through its aromatic ring forms π–π stacking interaction. Olanzapine: This is another bulky antagonist which has ability to inhibit the D2R via forming the strong hydrogen bond with the Asp114. The estimated enthalpy of ligand binding was found to be −22.46 kcal/mol which indicates the importance of Asp114 in stabilizing the ligand. Val115 and Ser193 amino acids have favorable interactions with the olanzapine and their energy contributions were −6.16 and −5.88 kcal/mol, respectively. Risperidone: It has an extended chemical structure and it is one of the class-II ligands. It forms strong H-bond with Asp114. The enthalpy of ligand binding was found to be −19.42 kcal/mol. The non-covalent interactions of the Asp114 and Val115 amino acids in the TM3 domain are noteworthy. Ziprasidone: This compound is also categorized as a class-II inhibitor. The enthalpy of binding to Asp114 was estimated to be −22.93 kcal/mol. The contributions of Val115, Ile184, and Tyr408 (TM7) residues were significant and enhanced the ziprasidone binding. Quetiapine: When compared to the other ligands in the class-I, the quetiapine interacted less strongly with the Asp114 with a binding enthalpy of −14.38 kcal/mol. This may be due to the polar tail attached to protonated N atom which affects the partial charge on the nitrogen atom and weakens H-bond formed between quetiapine and Asp114. The increased H-bond distance is consistent with the decreased binding energies.
All these energy analyses revealed a satisfactory agreement with the experimental results (Fu et al., Citation1996; Javitch, Ballesteros, Weinstein, & Chen, Citation1998; Wiens, Nelson, & Neve, Citation1998). However, it should be noted that such analyses can be very sensitive to the dynamics and motion of the compounds in the ligand binding pocket.
Conclusion
The experimentally reported binding affinities of six marketed antipsychotic drugs were used to compare the accuracies of two different approaches in calculating quantum mechanical binding affinities based on the preliminary docking and MD simulations studies. In the first approach, only the drug molecule and the amino acid having strongest interaction with the ligand (i.e. Asp114) were taken into consideration. Although we could show that the major interaction of Asp114 with the drugs is H-bonding interaction and dominates over all other amino acid residues in the binding cavity, the exclusion of weak interactions results in an overestimation of binding affinities because of the cumulative effect of neglected interactions. When all the interactions of drugs with amino acids in a radius of 5 Å around ligand at the binding site were considered by including vdW and steric energies, the calculated binding energies are in the same energy range. There is a better agreement between experimental and calculated binding energies using the latter approach, both showing that all the drugs bind to the catalytic domain of the D2R favorably, almost with the same efficiency.
Funding
This work was supported by the Max Planck Society for the Advancement of Science, the ‘Center for Dynamic Systems: Systems Engineering’ (an excellence initiative by Saxony-Anhalt and ERDF) and the Research Fund of Istanbul Technical University. Computing resources are provided by the National Center for High Performance Computing of Turkey (UHEM) under the Grant Number 5004452017.
Disclosure statement
No potential conflict of interest was reported by the authors.
Acknowledgments
We thank the Max Planck Society for the Advancement of Science and the Center for Dynamic Systems: Systems Engineering (an excellence initiative by Saxony-Anhalt and ERDF) for financial support.
References
- Apweiler, R. (2004). UniProt: The universal protein knowledgebase. Nucleic Acids Research, 32, D115–D119. doi:10.1093/nar/gkh131
- Björklund, A., & Dunnett, S. B. (2007). Dopamine neuron systems in the brain: An update. Trends in Neurosciences, 30, 194–202. doi:10.1016/j.tins.2007.03.006
- Boys, S. F., & Bernardi, F. (1970). The calculation of small molecular interactions by the differences of separate total energies. Some procedures with reduced errors. Molecular Physics, 19, 553–566. doi:10.1080/00268977000101561
- Chenna, R., Sugawara, H., Koike, T., Lopez, R., Gibson, T. J., Higgins, D. G., & Thompson, J. D. (2003). Multiple sequence alignment with the Clustal series of programs. Nucleic Acids Research, 31, 3497–3500. doi:10.1093/nar/gkg500
- Contreras, F., Fouillioux, C., Bolívar, A., Simonovis, N., Hernández-Hernández, R., Armas-Hernandez, M. J., & Velasco, M. (2002). Dopamine, hypertension and obesity. Journal of Human Hypertension, 16, S13–S17. doi:10.1016/S0531-5131(01)00579-9
- Creese, I., Burt, D. R., & Snyder, S. H. (1976). Dopamine receptor binding predicts clinical and pharmacological potencies of antischizophrenic drugs. Science, 192, 481–483. doi:10.1126/science.3854
- Das, R., & Baker, D. (2008). Macromolecular modeling with rosetta. Annual Review of Biochemistry, 77, 363–382. doi:10.1146/annurev.biochem.77.062906.171838
- Daulat, A. M., Maurice, P., & Jockers, R. (2009). Recent methodological advances in the discovery of GPCR-associated protein complexes. Trends in Pharmacological Sciences, 30, 72–78. doi:10.1016/j.tips.2008.10.009
- Ditchfield, R., Hehre, W. J., & Pople, J. A. (1971). Self-consistent molecular-orbital methods. IX. An extended Gaussian-type basis for molecular-orbital studies of organic molecules. The Journal of Chemical Physics, 54, 724–728. doi:10.1063/1.1674902
- Durdagi, S., Salmas, R. E., Stein, M., Yurtsever, M., & Seeman, P. (2016). Binding interactions of dopamine and apomorphine in D2 high and D2 low states of human dopamine D2 Receptor using computational and experimental techniques. ACS Chemical Neuroscience, 7, 185–195. doi:10.1021/acschemneuro.5b00271
- Ehrlich, S., Göller, A. H., & Grimme, S. (2017). Towards full quantum-mechanics-based protein-ligand binding affinities. ChemPhysChem, 18, 898–905.10.1002/cphc.201700082
- Eswar, N., Webb, B., Marti-Renom, M. A., Madhusudhan, M., Eramian, D., Shen, M., … Sali, A. (2007). Comparative protein structure modeling using MODELLER. Current Protocols in Protein Science, 50, 2.9.1–2.9.31. doi:10.1002/0471140864.ps0209s50
- Frisch, M. J., Trucks, G. W., Schlegel, H. B., Scuseria, G. E., Robb, M. A., Cheeseman, J. R., … Fox, D. J. (2016). Gaussian 09, Revision A.02. Wallingford, CT: Gaussian.
- Fu, D., Ballesteros, J. a., Weinstein, H., Chen, J., & Javitch, J. a. (1996). Residues in the seventh membrane-spanning segment of the dopamine D2 receptor accessible in the binding-site crevice. Biochemistry, 35, 11278–11285. doi:10.1021/bi960928x
- Ghosh, J., Marru, S., Singh, N., Vanomesslaeghe, K., Fan, Y., & Pamidighantam, S. (2011). Molecular parameter optimization gateway (ParamChem). Proceedings of the 2011 TeraGrid Conference: Extreme Digital Discovery, 35. doi:10.1145/2016741.2016779
- Grimme, S. (2011). Density functional theory with London dispersion corrections. Wiley Interdisciplinary Reviews: Computational Molecular Science, 1, 211–228. doi:10.1002/wcms.30
- Hanson, M. A., Cherezov, V., Griffith, M. T., Roth, C. B., Jaakola, V. P., Chien, E. Y. T., … Stevens, R. C. (2008). A specific cholesterol binding site is established by the 2.8 Å structure of the human β 2-adrenergic receptor. Structure, 16, 897–905. doi:10.1016/j.str.2008.05.001
- Huang, J., & MacKerell, A. (2013). CHARMM36 all atom additive protein force field: Validation based on comparison to NMR data. Journal of Computational Chemistry, 34, 2135–2145. Retrieved from http://onlinelibrary.wiley.com/doi/10.1002/jcc.23354/full10.1002/jcc.23354
- Javitch, J. A., Ballesteros, J. A., Weinstein, H., & Chen, J. (1998). A cluster of aromatic residues in the sixth membrane-spanning segment of the dopamine D2 receptor is accessible in the binding-site crevice. Biochemistry, 37, 998–1006. doi:10.1021/bi972241y
- Javitch, J. a., Li, X., Kaback, J., & Karlin, a. (1994). A cysteine residue in the third membrane-spanning segment of the human D2 dopamine receptor is exposed in the binding-site crevice. Proceedings of the National Academy of Sciences of the United States of America, 91, 10355–10359. doi:10.1073/pnas.91.22.10355
- Klamt, A., & Schüürmann, G. (1993). COSMO: A new approach to dielectric screening in solvents with explicit expressions for the screening energy and its gradient. Journal of the Chemical Society, Perkin Transactions, 2, 799–805. doi:10.1039/P29930000799
- Lagerström, M. C., & Schiöth, H. B. (2008). Structural diversity of G protein-coupled receptors and significance for drug discovery. Nature Reviews Drug Discovery, 7, 339–357. doi:10.1038/nrd2518
- Muddana, H. S., & Gilson, M. K. (2012). Calculation of host–guest binding affinities using a quantum-mechanical energy model. Journal of Chemical Theory and Computation, 8, 2023–2033. doi:10.1021/ct3002738
- Nelson, M. T., Humphrey, W., Gursoy, A., Dalke, A., Kale, L. V., Skeel, R. D., & Schulten, K. (1996). NAMD: A parallel, object-oriented molecular dynamics program. International Journal of High Performance Computing Applications, 10, 251–268. doi:10.1177/109434209601000401
- Newcomer, J. W. (2005). Second-generation (atypical) antipsychotics and metabolic effects: A comprehensive literature review. CNS Drugs, 19(1), 1–93. doi:10.2165/00023210-200519010-00001
- Rasmussen, S. G. F., DeVree, B. T., Zou, Y., Kruse, A. C., Chung, K. Y., Kobilka, T. S., … Kobilka, B. K. (2011). Crystal structure of the β2 adrenergic receptor-Gs protein complex. Nature, 477, 549–555. doi:10.1038/nature10361.Crystal
- Rompler, H., Staubert, C., Thor, D., Schulz, A., Hofreiter, M., & Schoneberg, T. (2007). G protein-coupled time travel: Evolutionary aspects of GPCR research. Molecular Interventions, 7, 17–25. doi:10.1124/mi.7.1.5
- Ryde, U., & Soderhjelm, P. (2016). Ligand-binding affinity estimates supported by quantum-mechanical methods. Chemical Reviews, 116, 5520–5566. doi:10.1021/acs.chemrev.5b00630
- Salmas, R. E., Seeman, P., Aksoydan, E. E., Kantarcioglu, K. B., Stein, M., Yurtsever, M., & Durdagi, S. (2017a). Analysis of the glutamate agonist LY404,039 binding to non-static dopamine receptor D2 dimer structures and consensus docking. ACS Chemical Neuroscience, 8, 1404–1415. doi:10.1021/acschemneuro.7b00070
- Salmas, R. E., Seeman, P., Aksoydan, B., Stein, M., Yurtsever, M., & Durdagi, S. (2017b). Biological insights of the dopaminergic stabilizer ACR16 at the binding pocket of dopamine D2 Receptor. ACS Chemical Neuroscience, 8, 826–836.10.1021/acschemneuro.6b00396
- Salmas, R. E., Yurtsever, M., & Durdagi, S. (2016). Atomistic molecular dynamics simulations of typical and atypical antipsychotic drugs at the dopamine D2 receptor (D2R) elucidates their inhibition mechanism. Journal of Biomolecular Structure & Dynamics, 35, 738–754. doi:10.1080/07391102.2016.1159986
- Salmas, R. E., Yurtsever, M., Stein, M., & Durdagi, S. (2015). Modeling and protein engineering studies of active and inactive states of human dopamine D2 receptor (D2R) and investigation of drug/receptor interactions. Molecular Diversity, 19, 321–332. doi:10.1007/s11030-015-9569-3
- Seeman, P., Lee, T., Chau-Wong, M., & Wong, K. (1976). Antipsychotic drug doses and neuroleptic/dopamine receptors. Nature, 261, 717–719. doi:10.1038/261717a0
- Shaikh, S., Hodgkinson, S., Pilowsky, L., Van Os, J., Vallada, H., Collier, D., & Gill, M. (1994). Analysis of the conserved Asp(114) residue of the dopamine D2 receptor in schizophrenic patients. Psychiatric Genetics, 4, 211–214. doi:10.1097/00041444-199400440-00004
- Sherman, W., Day, T., Jacobson, M. P., Friesner, R. A., & Farid, R. (2006). Novel procedure for modeling ligand/receptor induced fit effects. Journal of Medicinal Chemistry, 49, 534–553. doi:10.1021/jm050540c
- Trzaskowski, B., Latek, D., Yuan, S., Ghoshdastider, U., Debinski, a., & Filipek, S. (2012). Action of molecular switches in GPCRs – theoretical and experimental studies. Current Medicinal Chemistry, 19, 1090–1109. doi:10.2174/092986712799320556
- TURBOMOLE V6.5, a development of University of Karlsruhe and Forschungszentrum Karlsruhe GmbH. (1989–2007). TURBOMOLE GmbH, since 2007. Retrieved from https://www.turbomole.com
- Walker, M., Harvey, A. J. A., Sen, A., & Dessent, C. E. H. (2013). Performance of M06, M06-2X, and M06-HF density functionals for conformationally flexible anionic clusters: M06 functionals perform better than B3LYP for a model system with dispersion and ionic hydrogen-bonding interactions. The Journal of Physical Chemistry A, 117, 12590–12600.10.1021/jp408166 m
- Weigend, F., Furche, F., & Ahlrichs, R. (2003). Gaussian basis sets of quadruple zeta valence quality for atoms H-Kr. Journal of Chemical Physics, 119, 12753–12762. doi:10.1063/1.1627293
- Wiens, B. L., Nelson, C. S., & Neve, K. a. (1998). Contribution of serine residues to constitutive and agonist-induced signaling via the D2S dopamine receptor: Evidence for multiple, agonist-specific active conformations. Molecular Pharmacology, 54, 435–444. doi:10.1124/mol.54.2.435
- Zhao, Y., & Truhlar, D. G. (2008). The M06 suite of density functionals for main group thermochemistry, thermochemical kinetics, noncovalent interactions, excited states, and transition elements: Two new functionals and systematic testing of four M06-class functionals and 12 other function. Theoretical Chemistry Accounts, 120, 215–241. doi:10.1007/s00214-007-0310-x