Abstract
We report molecular dynamics simulations of three possible adducts of Fe(II) to the N-terminal 1–16 fragments of the amyloid-β peptide, along with analogous simulations of Cu(II) and Zn(II) adducts. We find that multiple simulations from different starting points reach pseudo-equilibration within 100–300 ns, leading to over 900 ns of equilibrated trajectory data for each system. The specifics of the coordination modes for Fe(II) have only a weak effect on peptide secondary and tertiary structures, and we therefore compare one of these with analogous models of Cu(II) and Zn(II) complexes. All share broadly similar structural features, with mixture of coil, turn and bend in the N-terminal region and helical structure for residues 11–16. Within this overall pattern, subtle effects due to changes in metal are evident: Fe(II) complexes are more compact and are more likely to occupy bridge and ribbon regions of Ramachandran maps, while Cu(II) coordination leads to greater occupancy of the poly-proline region. Analysis of representative clusters in terms of molecular mechanics energy and atoms-in-molecules properties indicates similarity of four-coordinate Cu and Zn complexes, compared to five-coordinate Fe complex that exhibits lower stability and weaker metal–ligand bonding.
Communicated by Ramaswamy H. Sarma
Introduction
Neurodegenerative diseases present one of the greatest health-care challenges to modern society. Alzheimer’s disease (AD) affects millions and is projected to increase markedly in the coming decades (Kepp, Citation2017; WHO, Citation2017; World Alzheimer Report 2015, Citation2015). AD is characterised by degeneration and death of nerve cells and their connections in addition to the presence of insoluble plaques and fibrils in diseased brains (Hardy & Higgins, Citation1992; Karantzoulis & Galvin, Citation2011; Kepp, Citation2012; Kosik, Joachim, & Selkoe, Citation1986). The amyloid (Cleary et al., Citation2005; Haass & De Strooper, Citation1999; Selkoe, Citation1991; Walsh et al., Citation2002) and metal ion (Bush & Tanzi, Citation2008; Kepp, Citation2017) hypotheses are leading theories to explain the onset of AD: in the former, accumulation of the β-amyloid (Aβ) peptide into toxic soluble oligomers as well as observed plaques is argued to be the cause of disease. In the latter, dysregulation of naturally occurring metals, notably copper, zinc and iron through genetic, environmental or age-related factors, is believed to promote aggregation and hence disease onset (Bush, Citation2013; Faller & Hureau, Citation2012; Kepp, Citation2017; Kozlowski, Luczkowski, Remelli, & Valensin, Citation2012; Lovell, Robertson, Teesdale, Campbell, & Markesbery, Citation1998; Pithadia & Lim, Citation2012); the exact role and behaviour of these metals in relation to AD is reviewed in detail elsewhere (Duce & Bush, Citation2010; Greenough, Camakaris, & Bush, Citation2013; Kepp, Citation2012, Citation2017; Kozlowski et al., Citation2012).
Metal ion coordination has distinct effects on the structure and chemistry of Aβ, including its propensity to aggregate. The anchoring effect of metal coordination provides structural restraint and potentially a seed for aggregation. Indeed, many studies report that metal ions act as strong inducers of Aβ aggregation (Bush et al., Citation1994; Esler et al., Citation1996; Huang et al., Citation2004) though the results are diverse (Miller, Ma, & Nussinov, Citation2012), including a range of fibrillar and non-fibrillar, amorphous aggregates. Furthermore, these results are strongly dependent on reaction conditions. The redox activity of Cu and Fe may also lead to the formation of reactive oxygen species that place diseased nerve cells and synapses under oxidative stress (Greenough et al., Citation2013).
Aβ contains a high-affinity metal-binding region at residues 1–16, which includes three histidine residues (His6, His13 and His14) as well as other groups (e.g. Asp and Glu) that can coordinate metal ions depending on conditions (Curtain et al., Citation2001; Faller & Hureau, Citation2009; Faller, Hureau, & La Penna, Citation2014; Kepp, Citation2012, Citation2017; Kozin, Zirah, Rebuffat, Hui Bon Hoa, & Debey, Citation2001). These metal–Aβ complexes usually form at 1:1 ratio, though secondary, lower-affinity binding has been reported (Atwood et al., Citation2000; Kepp, Citation2017; Kozin et al., Citation2001; Tõugu, Karafin, & Palumaa, Citation2008; Tõugu & Palumaa, Citation2012). To date, most studies have focussed on Cu(II) and Zn(II) binding to Aβ. In contrast, few studies have identified Fe(II)–Aβ interactions – suggested to occur via Asp1, Glu3, His6, His13 and His14 (Bousejra-ElGarah, Bijani, Coppel, Faller, & Hureau, Citation2011). However, given the seemingly major role of iron dysfunction in AD (Bush, Citation2013; Honda, Casadesus, Petersen, Perry, & Smith, Citation2004; Kepp, Citation2012, Citation2017), further investigation of iron–Aβ complexes is required.
Molecular simulation is a well-established tool to examine metal interactions with Aβ: many studies using a range of methods, from ab initio and DFT through QM/MM to atomistic molecular dynamics (MD), have been reported for both Cu(II) and Zn(II) (Alí-Torres, Maréchal, Rodríguez-Santiago, & Sodupe, Citation2011; Alí-Torres, Mirats, Maréchal, Rodríguez-Santiago, & Sodupe, Citation2015; Furlan, Hureau, Faller, & La Penna, Citation2010; Giannozzi et al., Citation2012; Huy, Vuong, La Penna, Faller, & Li, Citation2016; La Penna, Hureau, Andreussi, & Faller, Citation2013; Pan & Patterson, Citation2013; Raffa & Rauk, Citation2007; Wise-Scira, Xu, Perry, & Coskuner, Citation2012). However, comparisons of the effects of different metals on Aβ under comparable simulation conditions are uncommon; to the best of our knowledge, only one report of MD simulations on Fe(II)–Aβ systems has been reported in the literature (Boopathi & Kolandaivel, Citation2016), so our initial focus is on Fe(II) bound in three different proposed coordination modes. These are shown in : Fe_1, where Fe(II) is bound via Asp1 (NH2 and CO), His6 (Nδ and CO) and His14 (Nδ); Fe_2, where Fe(II) is bound via Asp1 (NH2 and CO), His6 (Nδ and CO) and His13 (Nδ); and Fe_3, where Fe(II) is bound via Asp1 (NH2 and CO), Glu3 (Oε), His6 (Nδ and CO) and His14 (Nδ). Then, to compare the binding of different biologically relevant transition metals to Aβ, we have carried out microsecond timescale MD of the N-terminal 1–16 fragments of Aβ to Cu(II), Fe(II) and Zn(II), taking binding modes from those proposed by Nasica-Labouze et al. (Citation2015) – illustrated in – specifically, Cu(II) bound through Asp1 (NH2 and CO), His6(Nε) and His14(Nε); Fe(II) bound through Asp1 (NH2 and CO), His6 (Nε and CO) and His14 (Nε); and Zn(II) bound through Asp1 (Oδ), His6 (Nε), Glu11 (Oε) and His14 (Nε). The purpose of the current study is twofold: firstly, to examine whether changes in the identity of the particular amino acid residues bound to the metal strongly affect the peptide structure obtained from MD, using three different binding modes of Fe(II) as a test case. We then compare the effect of different metals on peptide structure and dynamics using comparable binding modes. Our primary focus in this work is on establishing a suitable protocol for MD simulation of these flexible systems: we hope to report simulations of other important systems, such as trivalent Fe(III) or Al(III) ions or non-natural metals such as Pt(II) or Co(III), in future publications.
Computational methods
Aβ1–16 was constructed in an extended conformation in MOE (Molecular Operating Environment (MOE) (2013.08), Citation2013) with the appropriate residue protonation states for physiological pH. Metals were coordinated to the peptide as shown in and subjected to brief LowMode conformational searches (Labute, Citation2010) to obtain reasonable starting structures. MD simulations were performed using the AMBER16 package (Case et al., Citation2016). The AMBER ff14SB forcefield parameter set (Maier et al., Citation2015) was used to model all standard amino acid residues, while metal parameters were obtained using the MCPB.py program (Li & Merz, Citation2016). Here, parameters are obtained from B3LYP/6-31G(d), and RESP charges for the metal-coordinating regions were obtained at the same level of theory using Gaussian09 (Frisch et al., Citation2009). The geometry of each system was optimised using 1000 steps of steepest descent and 1000 steps of conjugate gradient methods. MD simulations were carried out in the NVT ensemble, using a Langevin thermostat to control the temperature at 310 K. The generalised Born solvation model (Clark Still, Tempczyk, Hawley, & Hendrickson, Citation1990; Constancien & Contreras, Citation1984; Schaefer & Karplus, Citation1996) was used to solvate all systems, with electrostatic interactions neglected beyond a cut-off of 12 Å: this approach has been shown to enhance conformational sampling of flexible systems (Anandakrishnan, Drozdetski, Walker, & Onufriev, Citation2015). During all simulations, the SHAKE algorithm (Ryckaert, Ciccotti, & Berendsen, Citation1977) was used to constrain bonds to hydrogen. Simulations were performed using a 2-fs integration time step. Analysis of the trajectories was performed using CPPTRAJ v16.16 (Roe & Cheatham, Citation2013) and VMD 1.9.3 (Humphrey, Dalke, & Schulten, Citation1996). Secondary structure assignment was made using the DSSP algorithm (Kabsch & Sander, Citation1983) via CPPTRAJ. Salt bridges were defined as any contact distance of less than 3.2 Å between oxygen and nitrogen atoms in charged residues. Ramachandran maps and hydrogen-bonding plots were made using MDplot (Margreitter & Oostenbrink, Citation2017). Atoms-in-molecules (AIM) analysis used the AIMAll package (Keith, Citation2017).
3. Results and discussion
3.1. Effect of Fe(II) coordination mode
Three separate 500 ns MD simulations of each Fe–Aβ complex (denoted Fe_1, Fe_2 and Fe_3) were carried out, starting from the same minimised structure but with different initial velocities, randomly sampled from the Maxwell–Boltzmann distribution at 310 K. Root-mean-square displacement (RMSD) relative to starting structure was used as our primary measure of equilibration (Huy et al., Citation2016; Mutter, Turner, Deeth, & Platts, Citation2018; Turner, Mutter, Deeth, & Platts, Citation2018). Plots of backbone RMSD for each run are shown in , which show that simulations reach stable values after 100–300 ns; equilibration points for each simulation are included in Table S1, supplementary material. All analyses reported are taken from data extracted from frames after each equilibration point, averaged over three separate runs, leading to 900, 950 and 950 ns of simulation data for Fe_1, Fe_2 and Fe_3, respectively. ‘Spikes’ in RMSD are seen in some plots, for instance around 350 ns in Fe_1C: closer inspection indicates that these correspond to relatively minor changes in backbone conformation for a few residues (, supplementary material), without affecting metal coordination or the overall structure of the peptide.
Table 1. Total percentage of secondary structure, by type, for Fe(II)–Aβ complexes.
Averages and standard deviations of RMSD, relative to a common starting point, over these trajectories (Table S2, supplementary material) further confirm equilibration: averages are in the range 2–4 Å with small standard deviations 0.2–0.45 Å. Radius of gyration (Rg) values are similar, with small standard deviations of 0.15–0.2 Å (Table S2, supplementary material). Averages for the three coordination modes converge to similar values of between 7.2 and 7.6 Å, with the largest value observed for the six-coordinate Fe_3. Root-mean-square fluctuations (RMSF) for individual amino acids exhibit similar behaviour, as shown in (data in Table S3, supplementary material). Metal-binding residues exhibit the lowest RMSF values – the most mobile being Glu3 in Fe_3, with an RMSF of 5.69 Å – while larger values of up to 10 Å are found for aromatic residues Phe4 and Tyr10, and also for the C-terminal Lys16 residue.
Table 2. Percentage of secondary structure types observed over equilibrated trajectories.
Table 3. Statistics of hydrogen bond occupation.
Metal coordination can exert profound effects on the structure of Aβ, which has an important role in its neurobiology. shows secondary structure by residue for each Fe(II)–Aβ system, as well as Ramachandran maps for the equilibrated trajectories; shows total peptide secondary structure percentage. Plots are very similar, exhibiting large amounts of bend structure in residues 3–11 and a mix of α- and 310-helix for residues 12–16. The six-coordinate structure favours turn over bend in the N-terminus, but is otherwise similar. Very small amounts (<0.25%) of β-strand structure are found to be present in all three systems. Ramachandran maps reveal that all three complexes have the greatest concentration of conformations in the region expected for α- and 310-helix, along with smaller but significant populations of β-strand and left-handed helix, the former being more prevalent for the two five-coordinate complexes (Fe_1 and Fe_2). Residue contact maps are also broadly similar, exhibiting relatively short contacts between coordinated residues and longer distances between central and C-terminal ones.
Clustering analysis of all equilibrated trajectories on the basis of peptide backbone dihedrals was also performed, leading to the data in , including the minimised structures of the most representative clusters. For both Fe_1 and Fe_3, a single cluster accounts for over 90% of conformations, while for Fe_2, the dominant cluster accounts for 57% of simulation. All three show similar properties, with coil/bend/turn throughout the N-terminal region and a small amount of helix towards the C-terminus. These aspects of the secondary structure are summarised in , which reports that each simulation spends between 15 and 20% of the time in helix-like conformations, less than 0.25% in strand-like, and over 80% in turn/bend/coil conformations. Overall, we surmise that the specifics of coordinating residues do not strongly affect the conformations visited by or the flexibility of the peptide chain.
3.2. Comparison of Cu, Fe and Zn binding
We therefore decided to compare data from the Fe_1 coordination mode to analogous structures bound to Cu(II) and Zn(II), since these share many of the same binding residues. All three metals are bound through Asp1, His6 and His14, with Glu11 as an additional binding residue for Zn(II) only. shows backbone RMSD values for each simulation, while Table S4, supplementary material, reports RMSD and Rg values for post-equilibration frames for the three systems studied. In each case, RMSD values are relatively small (2–3.5 Å) with small standard deviations (0.2–0.35 Å). Maximum values are very slightly larger for Zn than for Cu and Fe, but differences are rather small. A similar pattern is seen for Rg data, in which Fe is notably more compact than the others and Zn slightly larger than Cu. These data suggest that the five-coordinate nature of the Fe complex restricts the peptide to a smaller, more compact set of structures. As expected, given the harmonic bonding model used for metal coordination, bond lengths involving metal ions oscillate around equilibrium values of around 2.0 Å with standard deviations of ca. 0.05 Å. Bond angles exhibit a similar pattern, exhibiting standard deviations of 2–3°, and reflect the approximately square-pyramidal, distorted square-planar and tetrahedral coordination geometries of Fe, Cu and Zn, respectively. Full data are reported in Table S5, supplementary material.
Table 4. Summary of bond critical point propertiesTable Footnotea for metal–ligand bonds (au).
Further information on the impact of metal coordination on peptide conformational freedom can be drawn from RMSF data, as reported in Table S6, supplementary material, and . The overall pattern for all three metals is similar: metal-bound residues are the least mobile: His6 and His14 in particular exhibit lower RMSF values. Reflecting the values above, Fe_1 has the lowest average RMSF and the lowest value for 9 of the 16 residues, while Zn has the highest average RMSF and is highest for nine residues.
Metal binding has important effects on the structure and chemistry of Aβ: reports a summary of the secondary structure across equilibrated trajectories, and shows total peptide secondary structure percentage. Secondary structures show a good deal of similarity between metals: amino acids Asp1–Tyr10 exhibit mainly coil/turn/bend structures, while Glu11–Gln15 show a much more helical character, along with turn. Within this broad picture, however, subtle details differ: Zn induces more α-helical content than the other metals, which extends further towards the C-terminus of the peptide, whereas Cu and Fe show more 310 helix characters over a slightly shorter range. A small amount of parallel β-strand contact between Ala2 and Gln15 is also seen for Fe_1, and an even smaller extent of anti-parallel strand for Ser8 and Gly9 in the Cu complex. Ramachandran maps reflect this pattern, but also show more detail of conformations sampled by MD. All show the greatest concentration within the right-handed helix region of the maps, along with variable amounts of β-strand and left-handed helical conformations. Notably, Cu shows a large number of conformations in the PPII region (ca. −75, 150) (Adzhubei & Sternberg, Citation1993; Hollingsworth & Karplus, Citation2010; Woody, Citation2009). The PPII structure is relatively open and contains little/no internal hydrogen bonding (vide infra) and as such is likely characterised as turn/bend/coil. Fe_1 and Zn show progressively fewer conformations in this region. In addition, Fe_1 shows significant occupation of the bridge and ribbon regions of the plot, extending between the broad helical and β-sheet regions (Hollingsworth & Karplus, Citation2010; Karplus, Citation1996) Fe_1 also has a greater number of conformations in the β-sheet region of the Ramachandran map than either Cu or Zn, though this is not reflected in secondary structure analysis, likely due to their lack of requisite hydrogen bonds (vide infra). Overall though, we stress that the N-terminus of Aβ remains rather unstructured despite the metal coordination imposing structural restraints on certain residues, as exemplified by the data in .
Figure 8. Breakdown of secondary structure and Ramachandran maps for Cu, Fe_1 and Zn complexes with Aβ1–16.
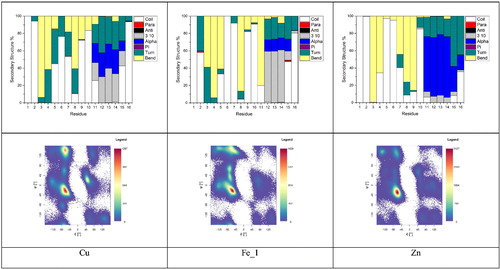
Salt bridges are crucial in determining peptide structure and stability: shows the percentage occupancy of all eight possible such contacts within Aβ1–16 (numerical data in Tables S7–S9, supplementary material). In all systems, the most commonly observed interaction is between Arg5 and Asp7, despite the fact that the metal ions are coordinated through His6 between these residues. This bridge is present for almost 100% of the sampled configurations of the Fe_1 complex, and between 75–85% for Cu and Zn. Glu3–Lys16 is also very prevalent (91%) for Fe_1, but is barely observed for Cu and Zn for which Glu3 tends to make contact with Arg5 (38 and 10%, respectively). Notably, the Asp7–Lys16 interaction is observed much more frequently (14%) in the Cu simulations than in either the iron or zinc. Asp1 and Glu11 rarely form salt bridge interactions in any of the metal systems.
The effect of these salt bridges, and of the metal coordination patterns, is evident in the contact maps shown in . Fe_1 contains rather short distances for distant amino acids that share coordination, for instance, between Asp1 and His14 and also between Glu3 and Lys16 that participate in a high-occupancy salt bridge. In contrast, Cu and Zn complexes have rather fewer close contacts, reflecting the lower coordination number and fewer salt bridges in these complexes. Particularly long distances are observed for Glu3–Val12 in Cu, and Glu3–Tyr10, His13 and Lys16 in Zn.
As well as salt bridges, hydrogen bonds play an important role in determining stability and structure: summarises the overall H-bond patterns for the three metals. All are rather transient, with frames containing zero hydrogen bond interactions observed as well as others with as many as 15. On average, Fe_1 contains the most: contacts between backbone C = O of Glu11 and H-Nε of His14 (58% of trajectory) and between backbone C = O of Phe4 and H-Nε of His6 (57%) are the most prevalent. In contrast, the most occupied H-bond in the Zn complex is between Tyr10 C = O and H-Nε of His14 at just 33% of frames, while for Cu, the contact between Lys16 C = O and H-Nε of His6 is observed for 42% of frames. adds more detail to this picture and highlights significant differences between metals: both Cu and Zn contain frequent interactions between Arg5 donor and Glu3 and Asp7, whereas Fe contains only the former interaction. Both Cu and Fe display frequent His6 (donor) to Glu3 and Phe4 (acceptors), which are not present in Zn. In contrast, Zn Glu11 accepts H-bonds with four different residues with greater than 5% incidence, the most of any residue. Interestingly, Glu11 is coordinated to Zn in this case, while the uncoordinated Glu11 in Fe and Cu cases forms H-bond interactions with no more than three residues, though at greater incidence than for Zn.
Figure 10. Plots of hydrogen bond occupancy for Cu (left), Fe_1 (centre) and Zn (right). Plots only display data for hydrogen bonds present for greater than 5% of simulation. Black circles indicate multiple interactions between relevant residues.
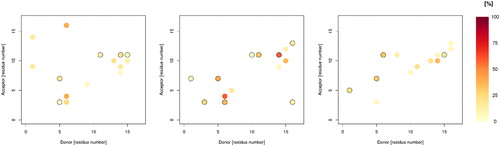
Clustering of all recorded frames on the basis of backbone dihedrals was also performed, leading to the data shown in , including ribbon diagrams of the most representative cluster following minimisation. For Cu and Zn, a single cluster accounts for over 60% of the trajectory, with the remaining frames spread across clusters encompassing no more than 17%. The Fe trajectory, however, leads to essentially a single cluster accounting for the entire set of post-equilibration frames recorded at 92%, with no other cluster covering more than 5%. This reinforces the picture that the extra metal–ligand bond in the five-coordinate iron complex limits the flexibility of the peptide more than is the case for four-coordinate metals. We also report the total MM energy after minimisation, which shows that the Cu and Zn complexes have similar energy and are markedly more stable than the Fe_1 complex. Further inspection of the breakdown of this energy (Table S10, supplementary material) indicates that bond stretch, angle bend and van der Waals terms are within 2 kcal/mol for all three systems and that the overall energy difference stems from changes in electrostatic and generalised Born solvation terms. It seems that higher coordination number of the iron complex prevents the polar side chains from interacting electrostatically with either the rest of the peptide or the implicit solvent, compared to the four-coordinate Cu and Zn complexes.
Figure 11. Summary of clustering analysis: minimised geometry, percentage occupancy and molecular mechanics energy (kcal/mol) after minimisation.
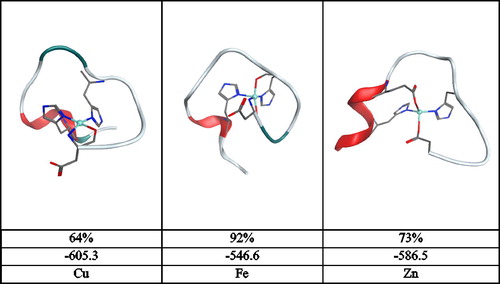
Optimised geometries of representative clusters were used to carry out AIM analysis, based on electron density from B3LYP/6-31G(d) calculations. This is focussed on the metal–ligand bonds: bond critical points (BCPs) are located for all coordination bonds: properties at these points are summarised in . This reveals a pattern of metal–His bonds being notably stronger than those of other atoms, exhibiting greater electron density (ρ), lesser positive Laplacian (∇2ρ) and more negative energy density (H). By these measures, the strongest of these bonds are between Cu and Nδ of His6 and His14, while those to Asp1 are around 10% weaker. This pattern is less marked for the other metals, but in general, these data indicate that coordination through oxygen is weaker than through N. Particularly weak, and hence more labile, bonds are observed for Fe—O(His6) and Zn—O(Glu11).
4. Conclusions
We have used atomistic MD to examine and compare the effect of coordination of copper, iron and zinc on the structure and dynamics of the N-terminal 1–16 fragments of Aβ. These ions are believed to play a role in the aggregation of Aβ and in the onset of AD, such that their effect on peptide conformational behaviour may be an important factor in disease. In order to compare different metals and coordination modes on an equal footing, we use Li and Merz’s MCPB.py approach, which extracts force constants and non-bonded parameters for metal ions from DFT calculations. These parameters were calculated for three different proposed coordination modes for Fe(II) and for comparable coordination of Cu(II) and Zn(II).
This method is used to obtain 1.5 μs of MD trajectories for two five-coordinate Fe(II) complexes, which differ in coordination through either His13 or His14, and a six-coordinate complex involving also the side chain carboxylate of Glu3. Each system is found to reach pseudo-equilibration after 100–300 ns, allowing us to extract over 900 ns of equilibrated data. Over the course of these trajectories, the conformations adopted by the peptide are broadly independent of the coordination mode: all exhibit turn/coil/bend structures in the N-terminus and a significant amount of helical content towards the C-terminus. Similar patterns in the flexibility of individual residues, as measured by RMSF, and inter-residue contact distances are also seen.
Comparison of one of these Fe(II) coordination modes with analogous ones to Cu(II) and Zn(II) was then made, from which we find more notable differences between metals. Equilibration again occurs within similar time scales, allowing extraction of at least 900 ns of equilibrated trajectories. The overall picture of turn/bend/coil in N-terminus and helix in C-terminus is retained, but the amounts of each vary more between metals. In particular, Cu coordination leads to greater occupancy of the PPII region of Ramachandran maps more than other metals, while Fe leads to significant occupation of bridge and ribbon regions. Salt bridges are also affected differently by different metals: Arg5–Asp7 is ubiquitous, whereas Glu3–Lys16 is common for Fe complexes, but is hardly present for Cu/Zn. These changes are evident in inter-residue contacts: the five-coordinate Fe complex is more compact than the four-coordinate Cu/Zn ones, with Zn in particular allowing greater peptide flexibility. Potential biological consequences of these changes are difficult to estimate, especially since we have only considered the N-terminal portion of the peptide. Nevertheless, we speculate that the rigidity of the Fe complex might provide a better seed for aggregation than the more flexible Cu and Zn complexes.
Clustering allows us to extract a single structure that is representative of the equilibrated trajectories: after minimisation, the energies of the Cu and Zn complexes are similar, but that of the Fe complex is significantly higher, apparently due to the strain imposed by the extra coordination bond for this metal and the lower solvation energy that results from coordination of the carboxylate of Glu3. DFT and AIM analysis of the metal–peptide bonds within these minimised clusters indicates that metal–histidine bonds are strongest, especially within the Cu complex, and that bonds to N are slightly stronger than those to O.
This work focusses on the N-terminal fragment of Aβ since this is known to be the site of metal binding, while the relatively compact nature of the complexes formed aid equilibration of MD trajectories. Having established suitable parameters for simulation of different transition metals, future work will address how the same methods can be applied to the full 1–40 or 1–42 sequences and their aggregation.
Data availability statement
The data that support the findings of this study are openly available via Zenodo at 10.5281/zenodo.1540579.
revised_SI_.docx
Download MS Word (125.2 KB)Acknowledgements
We are grateful to Cardiff University’s ARCCA facility for access to high-performance computing.
Disclosure statement
No potential conflict of interest was reported by the authors.
Additional information
Funding
References
- Adzhubei, A. A., & Sternberg, M. J. E. (1993). Left-handed polyproline II helices commonly occur in globular proteins. Journal of Molecular Biology, 229(2), 472–493. doi: 10.1006/jmbi.1993.1047
- Alí-Torres, J., Maréchal, J.-D., Rodríguez-Santiago, L., & Sodupe, M. (2011). Three dimensional models of Cu2+-Aβ(1–16) complexes from computational approaches. Journal of the American Chemical Society, 133(38), 15008–15014. doi: 10.1021/ja203407v
- Alí-Torres, J., Mirats, A., Maréchal, J.-D., Rodríguez-Santiago, L., & Sodupe, M. (2015). Modeling Cu2+-Aβ complexes from computational approaches. AIP Advances, 5(9), 092402. doi: 10.1063/1.4921072
- Anandakrishnan, R., Drozdetski, A., Walker, R. C., & Onufriev, A. V. (2015). Speed of conformational change: Comparing explicit and implicit solvent molecular dynamics simulations. Biophysical Journal, 108(5), 1153–1164. doi: 10.1016/j.bpj.2014.12.047
- Atwood, C. S., Scarpa, R. C., Huang, X., Moir, R. D., Jones, W. D., Fairlie, D. P., … Bush, A. I. (2000). Characterization of copper interactions with Alzheimer amyloid beta peptides: Identification of an attomolar-affinity copper binding site on amyloid beta 1-42. Journal of Neurochemistry, 75(3),1219–1233. doi: 10.1046/j.1471-4159.2000.0751219.x
- Boopathi, S., & Kolandaivel, P. (2016). Fe2+ binding on amyloid β-peptide promotes aggregation. Proteins: Structure, Function, and Bioinformatics, 84(9), 1257–1274. doi: 10.1002/prot.25075
- Bousejra-ElGarah, F., Bijani, C., Coppel, Y., Faller, P., & Hureau, C. (2011). Iron(II) binding to amyloid-β, the Alzheimer’s peptide. Inorganic Chemistry, 50(18), 9024–9030. doi: 10.1021/ic201233b
- Bush, A., Pettingell, W., Multhaup, G., D Paradis, M., Vonsattel, J., Gusella, J., … Tanzi, R. (1994). Rapid induction of Alzheimer A beta amyloid formation by zinc. Science, 265(5177), 1464–1467. doi: 10.1126/science.8073293
- Bush, A. I. (2013). The metal theory of Alzheimer’s disease. Journal of Alzheimer's Disease, 33(s1), S277–S281. doi: 10.3233/JAD-2012-129011
- Bush, A. I., & Tanzi, R. E. (2008). Therapeutics for Alzheimer's disease based on the metal hypothesis. Neurotherapeutics : The Journal of the American Society for Experimental Neurotherapeutics, 5(3), 421–432. https://doi.org/10.1016/j.nurt.2008.05.001
- Case, D. A., Betz, R. M., Cerutti, D. S., Cheatham, T. E., III, Darden, T. A., Duke, R. E., … Kollman, P. A. (2016). AMBER 2016. San Francisco, CA: University of California.
- Clark Still, W., Tempczyk, A., Hawley, R. C., & Hendrickson, T. (1990). Semianalytical treatment of solvation for molecular mechanics and dynamics. Journal of the American Chemical Society, 112(16), 6127–6129. doi: 10.1021/ja00172a038
- Cleary, J. P., Walsh, D. M., Hofmeister, J. J., Shankar, G. M., Kuskowski, M. A., Selkoe, D. J., & Ashe, K. H. (2005). Natural oligomers of the amyloid-protein specifically disrupt cognitive function. Nature Neuroscience, 8(1), 79–84. doi: 10.1038/nn1372
- Constancien, R., & Contreras, R. (1984). Self-consistent field-theory of solvent effects representation by continuum models – Introduction of desolvation contribution. Theoretica Chimica Acta, 65(1), 1–11. doi: 10.1007/BF02427575
- Curtain, C. C., Ali, F., Volitakis, I., Cherny, R. A., Norton, R. S., Beyreuther, K., … Barnham, K. J. (2001). Alzheimer’s disease amyloid-β binds copper and zinc to generate an allosterically ordered membrane-penetrating structure containing superoxide dismutase-like subunits. Journal of Biological Chemistry, 276(23), 20466–20473. doi: 10.1074/jbc.M100175200
- Duce, J. A., & Bush, A. I. (2010). Biological metals and Alzheimer’s disease: Implications for therapeutics and diagnostics. Progress in Neurobiology, 92(1), 1–18. doi: 10.1016/j.pneurobio.2010.04.003
- Esler, W. P., Stimson, E. R., Jennings, J. M., Ghilardi, J. R., Mantyh, P. W., & Maggio, J. E. (1996). Zinc-induced aggregation of human and rat β-amyloid peptides in vitro. Journal of Neurochemistry, 66(2),723–732. doi: 10.1046/j.1471-4159.1996.66020723.x
- Faller, P., & Hureau, C. (2009). Bioinorganic chemistry of copper and zinc ions coordinated to amyloid-β peptide. Dalton Transactions, 7(7), 1080–1094. doi: 10.1039/B813398K
- Faller, P., & Hureau, C. (2012). Metal ions in neurodegenerative diseases. Coordination Chemistry Reviews, 256(19-20), 2127–2128. doi: 10.1016/j.ccr.2012.04.006
- Faller, P., Hureau, C., & La Penna, G. (2014). Metal ions and intrinsically disordered proteins and peptides: From Cu/Zn amyloid-β to general principles. Accounts of Chemical Research, 47(8), 2252–2259. doi: 10.1021/ar400293h
- Frisch, M. J., Trucks, G. W., Schlegel, H. B., Scuseria, G. E., Robb, M. A., Cheeseman, J. R., … Sonnenber, D. J. (2009). Gaussian09. Wallingford, CT: Gaussian Inc.
- Furlan, S., Hureau, C., Faller, P., & La Penna, G. (2010). Modeling the Cu + binding in the 1 − 16 region of the amyloid-β peptide involved in Alzheimer’s disease. The Journal of Physical Chemistry B, 114(46), 15119–15133. doi: 10.1021/jp102928h
- Giannozzi, P., Jansen, K., La Penna, G., Minicozzi, V., Morante, S., Rossi, G., & Stellato, F. (2012). Zn induced structural aggregation patterns of beta-amyloid peptides by first-principle simulations and XAS measurements. Metallomics, 4(2), 156–165. doi: 10.1039/C2MT00148A
- Greenough, M. A., Camakaris, J., & Bush, A. I. (2013). Metal dyshomeostasis and oxidative stress in Alzheimer’s disease. Neurochemistry International, 62(5), 540–555. doi: 10.1016/j.neuint.2012.08.014
- Haass, C., & De Strooper, B. (1999). Review: Neurobiology – The presenilins in Alzheimer’s disease – Proteolysis holds the key. Science, 286(5441), 916–919. doi: 10.1126/science.286.5441.916
- Hardy, J., & Higgins, G. (1992). Alzheimers-disease – The amyloid cascade hypothesis. Science, 256(5054), 184–185. doi: 10.1126/science.1566067
- Hollingsworth, S. A., & Karplus, P. A. (2010). A fresh look at the Ramachandran plot and the occurrence of standard structures in proteins. BioMolecular Concepts, 1(3-4), 271–283. https://doi.org/10.1515/bmc.2010.022
- Honda, K., Casadesus, G., Petersen, R. B., Perry, G., & Smith, M. A. (2004). Oxidative stress and redox-active iron in Alzheimer’s disease. Annals of the New York Academy of Sciences, 1012(1), 179–182. doi: 10.1196/annals.1306.015
- Huang, X., Atwood, C. S., Moir, R. D., Hartshorn, M. A., Tanzi, R. E., & Bush, A. I. (2004). Trace metal contamination initiates the apparent auto-aggregation, amyloidosis, and oligomerization of Alzheimer’s Aβ peptides. JBIC Journal of Biological Inorganic Chemistry, 9(8), 954–960. doi: 10.1007/s00775-004-0602-8
- Humphrey, W., Dalke, A., & Schulten, K. (1996). VMD: Visual molecular dynamics. Journal of Molecular Graphics, 14(1), 27–28, 33–38. doi: 10.1016/0263-7855(96)00018-5
- Huy, P. D. Q., Vuong, Q. V., La Penna, G., Faller, P., & Li, M. S. (2016). Impact of Cu(II) binding on structures and dynamics of Aβ42 monomer and dimer: Molecular dynamics study. ACS Chemical Neuroscience, 7(10), 1348–1363. doi: 10.1021/acschemneuro.6b00109
- Kabsch, W., & Sander, C. (1983). Dictionary of protein secondary structure: Pattern recognition of hydrogen-bonded and geometrical features. Biopolymers, 22(12), 2577–2637. doi: 10.1002/bip.360221211
- Karantzoulis, S., & Galvin, J. E. (2011). Distinguishing Alzheimer’s disease from other major forms of dementia. Expert Review of Neurotherapeutics, 11(11), 1579–1591. doi: 10.1586/ern.11.155
- Karplus, P. A. (1996). Experimentally observed conformation-dependent geometry and hidden strain in proteins. Protein Science, 5(7), 1406–1420. doi: 10.1002/pro.5560050719
- Keith, T. (2017). AIMAll (Version 17.11.14). Overland Park, KS: TK Gristmill Software.
- Kepp, K. (2012). Bioinorganic chemistry of Alzheimer’s disease. Chemical Reviews, 112(10), 5193–5239. doi: 10.1021/cr300009x
- Kepp, K. (2017). Alzheimer’s disease: How metal ions define β-amyloid function. Coordination Chemistry Reviews, 351, 127–159. doi: 10.1016/j.ccr.2017.05.007
- Kosik, K. S., Joachim, C. L., & Selkoe, D. J. (1986). Microtubule-associated protein tau (tau) is a major antigenic component of paired helical filaments in Alzheimer disease. Proceedings of the National Academy of Sciences of the United States of America, 83(11), 4044–4048. doi: 10.1073/pnas.83.11.4044
- Kozin, S. A., Zirah, S., Rebuffat, S., Hui Bon Hoa, G., & Debey, P. (2001). Zinc binding to Alzheimer’s Aβ(1–16) peptide results in stable soluble complex. Biochemical and Biophysical Research Communications, 285(4), 959–964. doi: 10.1006/bbrc.2001.5284
- Kozlowski, H., Luczkowski, M., Remelli, M., & Valensin, D. (2012). Copper, zinc and iron in neurodegenerative diseases (Alzheimer’s, Parkinson’s and prion diseases). Coordination Chemistry Reviews, 256(19-20), 2129–2141. doi: 10.1016/j.ccr.2012.03.013
- La Penna, G., Hureau, C., Andreussi, O., & Faller, P. (2013). Identifying, by first-principles simulations, Cu[amyloid-β] species making Fenton-type reactions in Alzheimer’s disease. The Journal of Physical Chemistry B, 117(51), 16455–16467. doi: 10.1021/jp410046w
- Labute, P. (2010). LowModeMD – Implicit low-mode velocity filtering applied to conformational search of macrocycles and protein loops. Journal of Chemical Information and Modeling, 50(5), 792–800. https://doi.org/10.1021/ci900508k doi: 10.1021/ci900508k
- Li, P., & Merz, K. M. (2016). MCPB.py: A python based metal center parameter builder. Journal of Chemical Information and Modeling, 56(4), 599–604. doi: 10.1021/acs.jcim.5b00674
- Lovell, M. A., Robertson, J. D., Teesdale, W. J., Campbell, J. L., & Markesbery, W. R. (1998). Copper, iron and zinc in Alzheimer’s disease senile plaques. Journal of the Neurological Sciences, 158(1), 47–52. doi: 10.1016/S0022-510X(98)00092-6
- Maier, J. A., Martinez, C., Kasavajhala, K., Wickstrom, L., Hauser, K. E., & Simmerling, C. (2015). ff14SB: Improving the accuracy of protein side chain and backbone parameters from ff99SB. Journal of Chemical Theory and Computation, 11(8), 3696–3713. doi: 10.1021/acs.jctc.5b00255
- Margreitter, C., & Oostenbrink, C. (2017). MDplot: Visualise molecular dynamics. The R Journal, 9(1), 164–186.
- Miller, Y., Ma, B., & Nussinov, R. (2012). Metal binding sites in amyloid oligomers: Complexes and mechanisms. Coordination Chemistry Reviews, 256(19-20), 2245–2252. doi: 10.1016/j.ccr.2011.12.022
- Molecular Operating Environment (MOE) (2013.08). (2017). Montreal, QC, Canada: Chemical Computing Group Inc.
- Mutter, S. T., Turner, M., Deeth, R. J., & Platts, J. A. (2018). Metal binding to amyloid-β1–42: A ligand field molecular dynamics study. ACS Chemical Neuroscience, 9(11), 2795–2806. https://doi.org/10.1021/acschemneuro.8b00210
- Nasica-Labouze, J., Nguyen, P. H., Sterpone, F., Berthoumieu, O., Buchete, N.-V., Coté, S., … Derreumaux, P. (2015). Amyloid β protein and Alzheimer’s disease: When computer simulations complement experimental studies. Chemical Reviews, 115(9), 3518–3563. doi: 10.1021/cr500638n
- Pan, L., & Patterson, J. C. (2013). Molecular dynamics study of Zn(Aβ) and Zn(Aβ)2. Plos One, 8(9), e70681. doi: 10.1371/journal.pone.0070681
- Pithadia, A. S., & Lim, M. H. (2012). Metal-associated amyloid-β species in Alzheimer’s disease. Current Opinion in Chemical Biology, 16(1-2), 67–73. doi: 10.1016/j.cbpa.2012.01.016
- Raffa, D. F., & Rauk, A. (2007). Molecular dynamics study of the beta amyloid peptide of Alzheimer’s disease and its divalent copper complexes. The Journal of Physical Chemistry B, 111(14), 3789–3799. doi: 10.1021/jp0689621
- Roe, D. R., & Cheatham, T. E. (2013). PTRAJ and CPPTRAJ: Software for processing and analysis of molecular dynamics trajectory data. Journal of Chemical Theory and Computation, 9(7), 3084–3095. doi: 10.1021/ct400341p
- Ryckaert, J.-P., Ciccotti, G., & Berendsen, H. J. C. (1977). Numerical integration of the cartesian equations of motion of a system with constraints: Molecular dynamics of n-alkanes. Journal of Computational Physics, 23(3), 327–341. doi: 10.1016/0021-9991(77)90098-5
- Schaefer, M., & Karplus, M. (1996). A comprehensive analytical treatment of continuum electrostatics. The Journal of Physical Chemistry, 100(5), 1578–1599. doi: 10.1021/jp9521621
- Selkoe, D. (1991). The molecular pathology of Alzheimers-disease. Neuron, 6(4), 487–498. doi: 10.1016/0896-6273(91)90052-2
- Tõugu, V., Karafin, A., & Palumaa, P. (2008). Binding of zinc(II) and copper(II) to the full-length Alzheimer’s amyloid-β peptide. Journal of Neurochemistry, 104(5), 1249–1259. doi: 10.1111/j.1471-4159.2007.05061.x
- Tõugu, V., & Palumaa, P. (2012). Coordination of zinc ions to the key proteins of neurodegenerative diseases: Aβ, APP, α-synuclein and PrP. Coordination Chemistry Reviews, 256(19-20), 2219–2224. doi: 10.1016/j.ccr.2011.12.008
- Turner, M., Mutter, S. T., Deeth, R. J., & Platts, J. A. (2018). Ligand field molecular dynamics simulation of Pt(II)-phenanthroline binding to N-terminal fragment of amyloid-β peptide. Plos One, 13(3), e0193668. doi: 10.1371/journal.pone.0193668
- Walsh, D. M., Klyubin, I., Fadeeva, J. V., Cullen, W. K., Anwyl, R., Wolfe, M. S., … Selkoe, D. J. (2002). Naturally secreted oligomers of amyloid beta protein potently inhibit hippocampal long-term potentiation in vivo. Nature, 416(6880), 535–539. doi: 10.1038/416535a
- WHO. (2017). Dementia. Retrieved December 14, 2017, from http://www.who.int/mediacentre/factsheets/fs362/en/
- Wise-Scira, O., Xu, L., Perry, G., & Coskuner, O. (2012). Structures and free energy landscapes of aqueous zinc(II)-bound amyloid-β(1–40) and zinc(II)-bound amyloid-β(1–42) with dynamics. JBIC Journal of Biological Inorganic Chemistry, 17(6), 927–938. doi: 10.1007/s00775-012-0909-9
- Woody, R. W. (2009). Circular dichroism spectrum of peptides in the poly(pro)II conformation. Journal of the American Chemical Society, 131(23), 8234–8245. doi: 10.1021/ja901218m
- World Alzheimer Report 2015. (2015). Retrieved December 14, 2017, from http://www.worldalzreport2015.org/