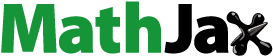
Abstract
Zika virus (ZIKV) is an emerging mosquito-borne flavivirus and infection by ZIKV Asian lineage is known to cause fetal brain anomalies and Guillain-Barrés syndrome. The WHO declared ZIKV a global public health emergency in 2016. However, currently neither vaccines nor antiviral prophylaxis/treatments are available. In this study, we report the identification of a C2‐symmetric diol‐based Human immunodeficiency virus type-1 (HIV) protease inhibitor active against ZIKV NS2B-NS3 protease. The compound, referred to as 9b, was identified by in silico screening of a library of 6265 protease inhibitors. Molecular dynamics (MD) simulation studies revealed that compound 9b formed a stable complex with ZIKV protease. Interaction analysis of compound 9b’s binding pose from the cluster analysis of MD simulations trajectories predicted that 9b mostly interacted with ZIKV NS3. Although designed as an aspartyl protease inhibitor, compound 9b was found to inhibit ZIKV serine protease in vitro with IC50 = 143.25 ± 5.45 µM, in line with the in silico results. Additionally, linear interaction energy method (LIE) was used to estimate binding affinities of compounds 9b and 86 (a known panflavivirus peptide hybrid with IC50 = 1.64 ± 0.015 µM against ZIKV protease). The LIE method correctly predicted the binding affinity of compound 86 to be lower than that of 9b, proving to be superior to the molecular docking methods in scoring and ranking compounds. Since most of the reported ZIKV protease inhibitors are positively charged peptide-hybrids, with our without electrophilic warheads, compound 9b represents a less polar and more drug-like non-peptide hit compound useful for further optimization.
Communicated by Ramaswamy Sarma
Introduction
Zika virus (ZIKV) is a mosquito-borne virus belonging to the Flavivirus genus of the Flaviviridae family. This family also includes other relevant human pathogens like dengue virus, yellow fever virus, West Nile virus and tick-borne encephalitis virus (Simmonds et al., 2011). ZIKV is a spherical, enveloped virus with icosahedral symmetry (Sirohi et al., Citation2016), that encloses a positive-sense, single stranded RNA genome of approximately 10.8 kb (Kuno & Chang, Citation2007). The ZIKV genome follows the typical Flavivirus genome organization, consisting of a single open reading frame (ORF) and two untranslated regions at both 5’ and 3’ ends. (Chambers, Hahn, Galler, & Rice, Citation1990) The single ORF is translated into a single polyprotein of around 3500 amino acids (Chambers et al., Citation1990). The polyprotein is then cleaved by the viral NS2B-NS3 protease and host proteases into three structural proteins: C (capsid), prM (precursor membrane), env (envelope) and eight non-structural (NS) proteins: NS1, NS2A, NS2B, NS3, NS4A, NS4B, NS5 and 2K (Chambers et al., Citation1990).
Currently, ZIKV is broadly classified into Asian and African lineages. Since its first isolation from humans in 1954, during an epidemic of jaundice in Nigeria (MacNamara, Citation1954), ZIKV caused only sporadic episodes of infection. However, in the last twelve years, the Asian lineage of ZIKV has been found to be the cause for several epidemic infections in humans (Cao-Lormeau et al., Citation2014; Duffy et al., Citation2009; Roth et al., Citation2014). The most important epidemic event, occurred in Brazil (2014–2016), had between 0.4 and 1.3 million estimated cases of ZIKV infection (World Health Organization, Citation2016). The common symptoms of ZIKV infection in patients include mild fever, headaches, asthenia, arthralgia, rashes and conjunctivitis (Cao-Lormeau et al., Citation2014; Duffy et al., Citation2009). Severe manifestations like fetal brain abnormalities (World Health Organization, Citation2016) and Guillain-Barrés syndrome, were also connected with ZIKV infection in French Polynesia (Oehler et al., Citation2014) and the Americas (World Health Organization, Citation2016), thereby increasing public health concern towards the emergence and spread of ZIKV. To date, these severe clinical manifestations have been observed only in patients with ZIKV infection of the Asian lineage and not the African lineage.
ZIKV is mainly transmitted by different types of mosquito species of the Aedes genus. Vertical (Driggers et al., Citation2016) and sexual (D’Ortenzio et al., Citation2016) transmissions of the virus have also been documented. Currently, neither vaccine nor drug is available to prevent or treat this viral infection. Viral proteases play an important role in the replication cycle of viruses and have been successfully targeted for the development of antiviral treatments against HIV (Lv, Chu, & Wang, Citation2015) and HCV (Leuw & Stephan, Citation2018). In an earlier study by our group, we have shown that despite not having a high sequence similarity between HCV and ZIKV, on the structural level, they are highly similar (Palanisamy, Akaberi, & Lennerstrand, 2017). Therefore, ZIKV NS2B-NS3 protease also represent a potential target for treatment/prophylaxis of the ZIKV infection. Usually, initial serine protease inhibitors originate from the peptide substrate and contain C-terminal electrophilic warheads forming a covalent bond with the enzyme (Leung, Abbenante, & Fairlie, Citation2000). Such oligopeptide inhibitors have been developed also for the ZIKV NS2B-NS3 protease and are based on the basic amino acids like Arg and Lys in P1 and P2 positions (Li et al., Citation2017; Nitsche et al., Citation2017). These inhibitors are polar and reactive, and do not have the properties needed for an orally administrated drug. To date, only a handful of non-peptidic compounds, both clinically approved (Chan et al., Citation2017; Yuan et al., Citation2017) and not (Lee et al., Citation2017; Lim et al., Citation2017), active against the ZIKV NS2B-NS3 protease have been reported. Thus, there is a need for non-covalent, less basic and overall more drug-like ZIKV NS2B-NS3 protease inhibitors. Crystal structures of ZIKV NS2B-NS3 protease, both bound to an inhibitor (Lei et al., Citation2016) and in the free (Phoo et al., Citation2016) form, have been resolved by the X-ray diffraction method, thereby allowing screening for potential inhibitors by in silico methods prior to performing in vitro assays; saving the additional time and the costs involved. Our aim in the present study is to use in silico tools to screen for potential hits that bind to the active site of ZIKV protease, and further validate these potential hits with the in vitro assay established for the ZIKV protease. To this purpose, a library of 6265 known or possible protease inhibitors was screened in silico and the potential hit was further validated experimentally.
Materials and methods
Compounds library preparation
The PubChem ID of 8222 protease inhibitors (PIs) known to inhibit different viral and non-viral proteases were first collected from the MEROPS small-molecule inhibitor database (Rawlings, Barrett, & Finn, Citation2016) and from the PubChem database (Kim et al., Citation2016). In the PubChem database, PIs were filtered from rest of the compounds by providing ‘protease inhibitor’ as input in the search field. Simplified molecular-input line-entry system (SMILES) of these 8222 unique PIs were converted into corresponding 3D structures using MolConverter v16.7.4.0 ChemAxon (http://www.chemaxon.com). While using the MolConverter, the ‘hyperfine’ option was used for the 3D conversion and MMFF94 (Halgren, Citation1996) force field was used for the optimization of the 3D structure. OpenBabel v2.3.2 (https://openbabel.org) (O’Boyle et al., Citation2011) was used to filter small fragments, of no interest for this study, with molecular weight lower than 180 Da from the library. This resulted in a new library consisting of 6265 unique PIs. Subsequently, the library was converted into different file formats to be used with different docking programs.
In silico docking
In silico docking was performed, in parallel, using two different docking programs namely AutoDock Vina v1.1.2 (Trott & Olson, Citation2010) and iGEMDOCK v2.1 (Hsu, Chen, Lin, & Yang, Citation2011). To perform an automated screening of the entire library with Autodock Vina, AUDocker v1.1.2 (Sandeep, Nagasree, Hanisha, & Kumar, Citation2011) (https://sourceforge.net/projects/audocker/files/) was used. The search space for AutoDock Vina was defined by a box with dimensions 30 x 30 x 30 Å3 and the exhaustiveness was set to ‘16’. For iGEMDOCK, the search space was automatically extracted from the ZIKV protease crystal structure 5CL0 (Lei et al., Citation2016) and the population size, the number of generations and the number of solution were set to 200, 70 and 3, respectively. For the compounds with PubChem IDs 449117, 449114, 449115, 445306 and 5484730, AutoDock Vina docking was repeated with an exhaustiveness of 80, while iGEMDOCK docking was repeated with the population size, the number of generations and the number of solution set to 800, 80 and 10, respectively.
Molecular dynamics simulations
Molecular dynamics (MD) simulations were carried out using GROMACS version 5.1.1 (Hess, Kutzner, van der Spoel, & Lindahl, Citation2008) on the ‘Tintin’ cluster composed of 160 nodes for a total of 2624 CPU cores. Each simulation was performed using one node composed of two 8 core Opteron 6220 processors (3 GHz) with 64 GB of RAM memory. Each system composing of ZIKV NS2B-NS3 protease (with or without the docked PI) was prepared as previously described (Akaberi et al., Citation2018). A total of three simulation replicates were performed with randomly generated new starting velocities. All PIs were simulated in complex with ZIKV protease crystal structure 5LC0 (Lei et al., Citation2016) and only compound 9b was also simulated in complex with ZIKV crystal structures 5GPI (Zhang et al., Citation2016) and 5GJ4 (Phoo et al., Citation2016). 5LC0, 5GPI and 5GJ4 are PDB IDs (https://www.rcsb.org/) of corresponding crystal structures. GROMACS built-in tools were used for root mean square deviation (RMSD), radius of gyration and cluster analyses of the MD simulations’ trajectories. Prediction analysis of possible interactions between compounds 9b (Pyring et al., Citation2001), 86 (Behnam, Graf, Bartenschlager, Zlotos, & Klein, Citation2015) and ZIKV protease 5LC0 was performed using Discovery Studio v16.1.0.15350 (Dassault Systèmes BIOVIA, San Diego).
The Q6 program (Bauer et al., Citation2018) was used to run MD simulations from which ligand-surrounding interaction energies were extracted and used for estimating a compound’s binding free energy. Compounds 9b and 86 were simulated bound to the ZIKV protease structures (5LC0 and 5GPI) and in the unbound free form in water. Q6 MD simulations were performed on the ‘‘Rackham’’ cluster composed of 486 nodes for a total of 9720 CPU cores. Each simulation was performed using one node composed of two 10 core intel Xeon VA CPUs processors with 128 GB of RAM memory. ZIKV proteases (PDB IDs: 5LC0 (Lei et al., Citation2016) and 5GPI (Zhang et al., Citation2016)) were assigned parameters from the OPLS-AA force field using the Q6 program. The parametrization of compounds, using the OPLS-AA force field (Jorgensen, Maxwell, & Tirado-Rives, Citation1996), was performed with the LigParGen webserver (Dodda, Cabeza de Vaca, Tirado-Rives, & Jorgensen, Citation2017) applying the 1.14*CM1A-LBCC and the 1.14*CM1A charge models for the compound 9b (net charge = 0) and for the compound 86 (net charge = +2), respectively. No optimization cycles were applied. All simulations were performed using spherical boundary conditions with a radius of 30 Å for the ligand-protease complex and a radius of 25 Å for the unbound ligands in water. All systems were solvated with TIP3P water and equilibrated for 200 ps during which the temperature was sequentially increased to 310 K, while the solute heavy atom restrains were gradually released. Five MD simulations of 5 ns each with different starting velocities were performed with an integration time of 1 fs. No restraints were applied in the ligand-protease complex simulations while a 10 kcal/mol·Å2 harmonic restraint was applied to the ligand during the unbound simulations in water to keep its center of mass in the center of the simulation’s sphere. A cut-off of 10 Å was used to calculate non-bonded interactions and the ligand-surrounding energies were saved every 25 steps. Residues Asp 50, 64, 91, 147 and Glu 62, 66, 88, 165 of the PDB ID: 5LC0 structure were changed into their neutral form. The same was done for the residues Asp 64, 86 and Glu 62, 94 of the 5GPI structure to keep the protease and the solvent net charge equal to zero.
Free binding energy calculation
Free binding energy was calculated with the linear interaction energy method (LIE) (Hansson, Marelius, & Åqvist, Citation1998) adopting the equation:
(1)
(1)
Where the estimated binding free energy ( is calculated as the sum of the average non-polar (
and polar (
ligand-surrounding (l-s) interaction energies. These average values are calculated as the difference of the average non-polar (2) and polar (3) interaction energies from the ligand-receptor complex and the free ligand in water, using the Q6 MD simulations data:
(2)
(2)
(3)
(3)
The scaling factor α was set to 0.18 and β was set to 0.33 for the compound 9b and 0.5 for the charged compound 86 (Almlöf, Carlsson, & Åqvist, Citation2007; Hansson et al., Citation1998). The constant term γ was set to 0.
The experimental free binding energy of the compounds 9b and 86 was calculated using the equation:
(4)
(4)
The inhibition constant (Ki) was substituted as shown in EquationEquation (5)(5)
(5) (Cheng & Prusoff, Citation1973), with T = 310 K, Km = 8.59 µM and the substrate concentration (S) equal to 20 µM for the compounds 9b and 15 µM for the compound 86.
(5)
(5)
Per-residue energy decomposition analysis
The interaction energies between the residues of ZIKV protease 5LC0 and the compound 9b were extracted fromQ6 MD simulations results. The polar and apolar interaction energies form the five simulation replicates were summed, averaged and plotted using Microsoft Excel.
Expression and purification of ZIKV NS2B-NS3 protease complex
Unlinked ZIKV protease NS2B-NS3 complex was expressed and purified following an earlier published protocol, adopting the bZiPro construct described by the Kang group (Phoo et al., Citation2016), containing nucleotide sequences corresponding to the NS2B residues 45–96 and the NS3 residues 3–179 of the ZIKV isolate ‘Brazil-ZKV2015’ (NCBI accession number KU497555.1). Detailed protocol is described in the supporting information.
The ZIKV NS2B and NS3 cDNAs were synthesized and cloned into NdeI/XhoI and NcoI/HindIII cloning sites of the pETDuet-1 vector by Genescript (GenScript Biotech, the Netherlands). The vector was transformed into E. coli BL21 (DE3)-T1R competent cells carrying the pRARE2 plasmid and cells were grown at 37 °C in terrific broth (TB) containing 1% glycerol supplemented with carbenicillin (50 μg/ml) and chloramphenicol (34 μg/ml). When the OD600 reached 2, the cultures were shifted to 18 °C. One hour later, the expressions of His-tagged NS2B and NS3 (no His tag) were induced by addition of 0.5 mM β-D-1-thiogalactopyranoside (IPTG) and allowed to grow overnight at 18 °C. Cells were harvested by centrifugation (10 min at 4500 xg), re-suspended the cells in IMAC lysis buffer (100 mM HEPES-NaOH pH 8.0, 500 mM NaCl, 10% glycerol, 10 mM imidazole, 0.5 mM TCEP), additionally containing benzonase, and disrupted by sonication (Sonics Vibracell). Lysates were centrifuged at 49,000 × g for 20 min at 4 °C. The supernatants were filtered before loading on the ATKA Xpress (GE Healthcare) protein purification system. Protein purification was performed using an IMAC HisTrap HP 5 ml column (GE Healthcare). The column was washed with wash buffer (20 mM HEPES-NaOH pH7.5, 500 mM NaCl, 10% glycerol, 50 mM imidazole, 0.5 mM TCEP) and the bound protein was eluted with elution buffer (20 mM HEPES-NaOH pH7.5, 500 mM NaCl, 10% glycerol, 500 mM imidazole, 0.5 mM TCEP), followed by a size exclusion chromatography step using a HiLoad 16/60 Superdex 75 preparative grade column (GE Healthcare) to replace the elution buffer with chromatography buffer (20 mM HEPES-NaOH pH 7.5, 300 mM NaCl, 10% glycerol, 0.5 mM TCEP). Fractions containing the target protein were examined on a SDS PAGE gel, pooled together, flashed frozen in liquid nitrogen and stored at −80 °C in storage buffer (20 mM HEPES-NaOH pH 7.5, 300 mM NaCl, 10% glycerol and 2 mM TCEP).
In vitro enzymatic assay
Indinavir was purchased from Sigma-Aldrich (product number: Y0000788). Compound 9b (Pyring et al., Citation2001) and compound 86 (Behnam et al., Citation2015; Kuiper et al., Citation2017) were synthesized according to earlier published data. Synthesized compounds were validated by NMR and mass spectroscopy (Supporting information).
The enzymatic assay was performed as described previously (Li et al., Citation2017). All experiments were carried out in black flat-bottomed 96 well plates (Nunc, Thermo Fisher Scientific) with a final volume of 100 µl. ZIKV NS2B-NS3 protease, at a final concentration of 3 nM, was incubated with either Compound 9b, 86 or indinavir at different concentrations in the assay buffer (20 mM Tris-HCl pH 8.5, 10% glycerol and 0.01% Triton X-100) for 10 min. The FRET substrate Bz-Nle-Lys-Arg-Arg-AMC (Bachem Holding AG, Switzerland) was then added at a final concentration of 20 µM to start the enzymatic reaction. The fluorescence emission was monitored every 60 s for 30 min at 37 °C using a Tecan infinite M200 PRO plate reader (Tecan Trading AG, Switzerland) with excitation wavelength set to 380 nm and emission wavelength set to 460 nm. Compound 9b was tested at concentrations ranging from 7.8 µM to 1 mM. It was dissolved in DMSO and serially diluted (2-fold) as 20x working solutions in DMSO. Five microliters of the 20× working solution were added to the wells (final volume 100 µl) to reach the 1x working concentration and keep the final DMSO concentration to 5%. Compound 86 was dissolved and diluted (5-fold) in the assay buffer and tested at final concentrations ranging from 0.032 µM to 100 µM. The relative fluorescence units (RFU) per second was plotted and the initial velocities were calculated and normalized, and converted to enzyme activity (in %). The enzyme activity (in %) values (control wells with no substrate = 100% inhibition and control wells with no inhibitor = 0% inhibition) were plotted against the log of the compound’s concentration used and IC50 values were fitted by nonlinear regression analysis performed using GraphPad Prism version 6 (graphPad Sofware, La Jolla California, USA). All compounds were independently tested twice and during each independent experiment, all concentrations were tested in triplicates.
Results
HIV protease inhibitors bind with high affinity to ZIKV NS2B-NS3 protease in silico
ZIKV NS2B-NS3 serine protease represent a promising target for the development of antiviral drugs against ZIKV infection. To find potential ZIKV NS2B-NS3 protease inhibitors (PIs), a library of 6265 known PIs retrieved from the MEROPS (Rawlings et al., Citation2016) and PubChem (Kim et al., Citation2016) databases, was screened using in silico molecular docking techniques. To facilitate the near accurate identification of the potential PIs, two programs namely, AutoDock Vina (Trott & Olson, Citation2010) and iGEMDOCK (Hsu et al., Citation2011), were used to screen the library. The PIs that both programs predicted to have high binding affinity were selected for further studies. Surprisingly, five non-basic HIV aspartyl protease inhibitors were found in the top 25 best scoring compounds from both AutoDock Vina and iGEMDOCK. Four of these HIV protease inhibitors were C2‐symmetric diol‐based compounds (Pyring et al., Citation2001) and the other one being indinavir. The selected HIV PIs structures and the predicted binding affinities of the best scoring binding poses are shown in .
Table 1. HIV protease inhibitors identified as potential ZIKV protease inhibitors.
Compound 86, a known panflavivirus PI with activities against DENV (IC50 = 0.028 µM) (Behnam et al., Citation2015), WNV (IC50 = 0.117 µM) (Behnam et al., Citation2015) and ZIKV (IC50 = 1.06 µM) (Kuiper et al., Citation2017), was used as a positive control in this study.
The reported binding affinity of compound 86 for ZIKV here is from manually selected binding poses of our Vina and iGEMDOCK results. Despite the fact that compound 86 is a basic peptide-hybrid similar to the ZIKV protease’s natural substrate (Chappell, Stoermer, Fairlie, & Young, Citation2006), the five HIV protease inhibitors identified had higher Vina scores and similar iGEMDOCK scores. Notably, the selected HIV inhibitors do not present any positively charged groups that are present in all sub-micromolar inhibitors of flavivirus proteases reported so far. The compounds 9f, 9b, 9e, and 9a differs only for the presence and positioning of one or two fluorine substitutes and shared very similar binding poses. In particular, the indanolamine groups were placed in the sub-site 1 (S1) and sub-site 3 (S3) pockets, one fluoro-substituted benzyloxy group in the sub-site 2 (S2) pocket and the other fluoro-substituted benzyloxy group either in the sub-site 1’ (S1’) pocket (compounds 9f and 9b) or in between the sub-site 2 (S2) and S1’ (compounds 9e and 9a). The binding poses of the compounds 9f and 9e from the Vina docking results are shown in as an example and the HIV protease-compound 9b complex is shown in the supplementary material for comparison. Indinavir also had an indalonamine group in the S1 pocket, while the S1’, S2 and S3 pockets were occupied by a benzene, a pyridine and a 2-methylpropane group, respectively. In the selected binding pose of compound 86, () the arginine side chain is positioned in the S2, the lysine side chain in the S1, the thiophene N-cap in the S3 and the C-terminal (4-hydroxy)-D-phenylglycine in the S1’ with the 4-trifluoromethylbenzyl ether extending to the right.
Figure 1. Docking poses of compounds 9f, 9e (A), indinavir (B) and compound 86 (C) from Vina docking results. The compound 9f (colored in purple) and compound 9e (colored in yellow) are shown as an example of the two different binding poses observed. The ZIKV NS2B-NS3 protease (PDB ID 5LC0) is colored in cyan. While the compounds 9f, 9e and indinavir nicely fit the ZIKV NS2B-NS3 protease’s binding site, they lack key positively charged groups present in the compound 86. As shown in C, the positively charged arginine and lysine side chains of the compound 86 interact with negatively charged aspartate residues (colored in green) in the S2 and S1 pockets.
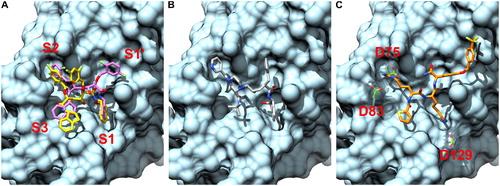
ZIKV NS2B-NS3 protease-9b complex is stable in MD simulations
The stability of the receptor-ligand poses generated by Vina, and their evolution over time, were investigated by performing 40 ns MD simulations in triplicates using GROMACS vr-5.1.1. The best scoring binding poses of the compounds 9f, 9b, 9e, 9a and indinavir with ZIKV protease, as well as the unbound form of the ZIKV protease from the PDB file 5LC0, were used as initial conformations for the MD simulations. MD simulations of compound 86 in complex with ZIKV protease were also performed as a positive control for comparison. The MD simulations’ trajectories were first visually inspected. Compound 9e and indinavir were found to dissociate from ZIKV protease during the MD simulations and were both excluded from further analyses. The MD simulations’ trajectories of the remaining compounds were used for canonical analyses to measure and compare the unbound protease and the protease-ligand complexes’ stability and compactness as a function of time. The structural stability of the protease was measured by calculating the RMSD of atomic coordinates while the radius of gyration (Rg) was calculated to measure the compactness of the free protein and protein-ligand complexes. ZIKV protease in complex with compound 9f had the highest average RMSD of 0.24 ± 0.04 nm () compared to the average RMSD of the ZIKV protease free form, which was 0.20 nm with a standard deviation (SD) of ±0.03 nm. Overall, the average RMSDs and SDs of the ZIKV protease free form and in complex with all compounds were similar suggesting that none of the compounds had any destabilizing effect on the enzyme structure. The same observation could be made for the calculated average Rgs. The ZIKV protease unbound and ligand-bound complex forms had near identical average Rgs with minor deviations (). These results also suggested that the compounds did not affect the enzyme compactness. The compounds’ RMSDs were also analyzed to understand how stable they were during the MD simulations. The compounds 9f and 9a (, red and green bars) presented slightly higher fluctuations of both the average RMSDs and SDs compared to the compound 9b that was the most stable compound with an average RMSD of 0.22 ± 0.03 nm (, blue bars). Since the compound 9b was the most stable of the HIV protease inhibitors studied, it was selected to predict possible interactions formed with the ZIKV protease.
Figure 2. Structural stability of bound/free ZIKV protease and compounds during the MD simulations. The average RMSD (A) and radius of gyration (B) of ZIKV protease bound to PI and free form are shown in the bar graphs. The average RMSD of compounds alone is shown in the bar graph (C). Error bars show the standard deviation.
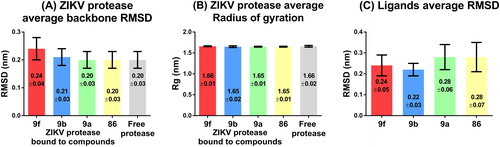
The compound 86 was again used as the positive control. MD simulations of the most representative conformation of compound 9b and compound 86 () were identified by cluster analysis of last 30 ns of the simulation period for a total of 90 ns simulation period.
Figure 3. Cluster analysis and predicted possible interactions between ZIKV protease and ligands. The average structure of compounds 9b and 86 extracted from the most populated cluster are shown in (A) and (B). The 2D diagrams show possible interactions formed between the compound 9b (C) and the compound 86 (D) with the ZIKV protease. Amino acids belonging to NS2B or NS3 are reported with the chain identifier ‘A’ and ‘B’ respectively. Hydrogen bonds, salt bridges and non-bonded compound interactions were contributing to the compounds binding.
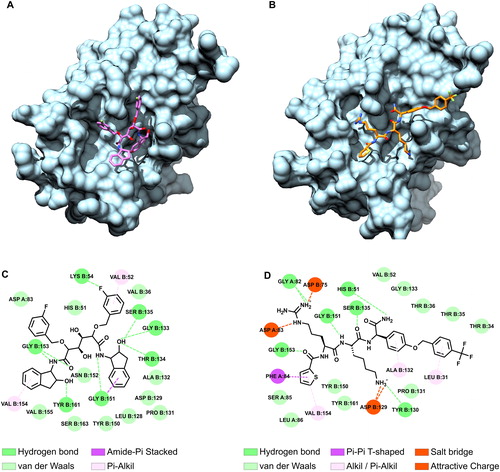
The compound 9b mostly interacted through the formation of hydrogen bonds with the ZIKV protease binding site. However, the compound 86 could also form salt bridges between the positively charged arginine and lysine side chains and the negatively charged Asp83 (NS2B), Asp75 (NS3) and Asp129 (NS3). Interestingly, both compounds were predicted to form hydrogen bonds with the residues Gly 151, Gly 153 and Ser 135 of the ZIKV NS3.
In order to further assess the binding stability of the compound 9b, we performed more MD simulations with two different ZIKV protease crystal structures, namely 5GJ4 (Phoo et al., Citation2016) and 5GPI (Zhang et al., Citation2016). While the ZIKV proteases 5LC0 was co-crystallized with a peptide-boronic acid irreversible inhibitor and the crystal structure 5GJ4 with a tetrapeptide ‘TGKR’, the crystal structure 5GPI represent the ZIKV protease in its unbound state. As previously described, Autodock Vina was again used to dock the compound 9b against 5GJ4 (Vina score = −8.2 kcal/mol) and 5GPI (Vina score = −8.4 kcal/mol) ZIKV protease structures. Binding poses similar to the one shown in were selected for the MD simulations using GROMACS. Analyses of the MD simulations’ trajectories proved that the compound 9b was stably bound with ZIKV protease crystal structures 5GJ4 and 5GPI as previously described for 5LC0 crystal structure (supplementary results).
Compound 9b impairs the function of ZIKV protease in an in vitro assay
In order to confirm if the compound 9b is a real ZIKV protease inhibitor, the compound was synthesized and tested in vitro using a FRET-based enzymatic assay (Li et al., Citation2017). The compound 86 was used as a positive control, and indinavir that was not stable during the MD simulations was used as a negative control. For the assay, unlinked recombinant ZIKV protease with or without the ligand was co-incubated with the FRET-substrate Bz-Nle-Lys-Arg-Arg-AMC. The cleavage of the substrate and the relative increase in emitted fluorescence was monitored in presence of different concentrations of the inhibitors. The compound 9b was indeed inhibiting the ZIKV protease with an IC50 = 143.25 ± 5.45 µM (). Indinavir, on the other hand, appeared to be a suitable negative control and had no inhibitory activity against ZIKV protease up to the highest concentration studied i.e. 1 mM (supplementary results), in line with the MD simulations’ results. As expected, the positively charged compound 86 had an IC50 = 1.64 ± 0.015 µM () similar to an IC50 value reported in a previous study by an another group (Kuiper et al., Citation2017) and was almost 100 times more potent than compound 9b.
Free binding energies of compounds 9b and 86 with the ZIKV NS2B-NS3 protease
Since the accuracy of the molecular docking programs used was not sufficient to correctly score and rank the screened PIs, we decided to evaluate other methods to estimate compounds binding affinity. In silico estimation of a ligand’s binding free energy from an ensemble of binding poses sampled using MD simulations can be extremely useful to select compounds for synthesis and in vitro testing. Since the compound 9b and 86 were tested in vitro, we were able to calculate their experimental binding free energy (see the EquationEquation (4)(4)
(4) in the materials and methods). We then used the linear interaction energy method (LIE) (Hansson et al., Citation1998) in combination with the MD simulations, to estimate the in silico binding free energy of the compounds 9b and 86. MD simulations of the compounds in complex with ZIKV crystal structure 5LC0, and in the unbound form were performed with the Q6 program (Bauer et al., Citation2018) using water as the solvent. Since the 5LC0 ZIKV protease was co-crystallized with a peptide-boronic acid inhibitor, the 5GPI ZIKV free protease crystal structure was also used. The compound 86’s binding affinity (
was correctly estimated to be higher than the one for the compound 9b regardless of the ZIKV protease crystal structures used (), proving that in this case, the LIE method was able to discriminate a compound with higher binding affinity from a compound with a lower binding affinity.
It was also noticeable that the estimated compounds’ binding affinities and standard errors were higher when the ZIKV protease 5LC0 was used. In the case of the compound 86, the estimated binding affinity was −2.77 kcal/mol lower than the calculated experimental binding affinity (). On the other hand, the estimated binding affinities of both the compounds were higher than the experimental values when using the ZIKV protease crystal structure 5GPI and the relative standard errors were improved. The difference between estimated and experimental binding affinities of the compounds 9b and 86 were −4.34 and −4.71 kcal/mol, respectively, with an average difference of −4.53 ± 0.26 kcal/mol. By using this value as the γ constant of EquationEquation (1)
(1)
(1) (see material and methods), the estimated binding energies of the compounds 9b and 86 (relative to the simulations performed with the ZIKV protease 5GPI) would be −5.98 and −9.04 kcal/mol, respectively; well in agreement with the calculated experimental binding free energy values.
Per-residue energy decomposition
The per-residue free energy decomposition was computed from the Q6 MD simulations results, performed using the ZIKV protease (PDB ID: 5LC0) in complex with the compound 9b, to identify the residues that contributed the most to the stabilization of the compound 9b with the protease. The residues with energy contributions ≤ −3 kcal/mol are shown in . All the eight identified residues belonged to the NS3 protease’s active site. Of these eight residues, six were also identified to potentially form hydrogen bonds with the compound 9b in the interaction analysis performed on the GROMACS MD simulations’ results (). This further proves the stability of the selected binding pose. Again, two of the three residues of the ZIKV NS3 protease’s catalytic triad, namely His51 and Ser135, were contributing to the stabilization of the compound 9b in the ZIKV active site.
Figure 5. Graph of average interaction energy of the ZIKV protease (PDB ID: 5LC0) residues with the compound 9b calculated from five independent MD simulations. Residues that interact with an energy of ≤ -3 kcal/mol are listed. The residues, which were previously found by us in this study, to potentially form hydrogen bonds with the compound 9b are reported in bold font.
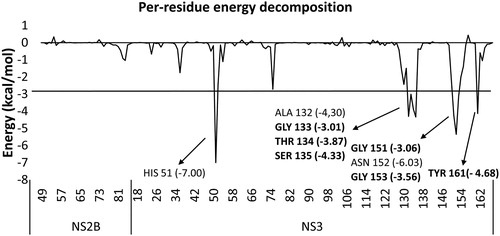
Discussion
In this study, we report the in silico identification of a HIV protease inhibitor, with proven in vitro inhibitory activity against the ZIKV protease. The compound 9b, together with three variants (compounds 9f, 9e and 9a), and the first generation HIV compound indinavir (), were identified by both Autodock Vina and iGEMDOCK during the in silico screening of a library containing 6265 protease inhibitors. Although the ZIKV NS2B-NS3 protease is a chymotrypsin like serine protease, and the HIV protease is an aspartyl protease, this is the second study that reports the identification of a HIV PI active against ZIKV protease. In the study performed by Yuan et al. (Citation2017), the HIV PIs lopinavir and ritonavir were selected by in silico screening and were found to have in vitro and in vivo activities against ZIKV protease. MD simulations by us of the PIs in complex with the ZIKV protease 5LC0, found that the compounds 9f, 9b, and 9a were stably bound to the ZIKV protease’s active site while having no destabilizing effect on the ZIKV protease structure stability and compactness (). The compounds were also found to be structurally stable during the entire course of the simulations, and in particular, the compound 9b had the lowest average RMSD value (0.22 ± 0.03 nm) as shown in . Similar results were replicated when performing MD simulations of the compound 9b in complex with the ZIKV protease crystal structures 5GPI and 5GJ4, which further proves the predicted binding stability of the compound. The compound 9b was therefore synthesized and tested in vitro using a FRET-based protease assay. Indinavir, which did not stably bind to the ZIKV protease during the MD simulations, and the compound 86, a known panflaviviral protease inhibitor, were also tested as negative and positive controls, respectively. As predicted by the in silico results, the compound 9b was active and inhibited the ZIKV protease with an IC50 = 143.25 ± 5.45 µM, while indinavir showed no activity. The compound 86 inhibited ZIKV protease with an IC50 of 1.64 ± 0.015 µM similar to the value of 1.06 µM reported by Kupier et al. (Kuiper et al., Citation2017). Although the compounds 9b had a modest IC50 in the high micromolar range, the results proved (in this case) the ability and usage of MD simulations to discriminate between active and inactive compounds. When comparing the IC50 values of the compounds 86 and 9b, compound 86 had almost 100 times higher potency than compound 9b, albeit the same was not reflected in the in silico docking scoring (). In the case of AutoDock Vina, this discrepancy could be due to the fact that molecule’s partial charges are not directly accounted for in the program scoring function (Trott & Olson, Citation2010). Thus, possibly leading to the overestimation of the binding affinity of bigger molecules that can make extensive hydrophobic interaction with the shallow binding site of ZIKV protease. It is also worth noticing that while the use of different ZIKV crystal structures had no effect on the outcomes of the MD simulations’ results; differences were observed in the docking results. The compound 9b had the lowest AutoDock Vina binding affinity when docked with the ZIKV protease crystal structure 5LC0 (−9.2 kcal/mol), while the binding affinities were slightly higher when the crystal structures 5GJ4 (−8.2 kcal/mol) and 5GPI (−8.4 kcal/mol) were used. On the contrary, the AutoDock Vina binding affinities of the compound 86 was lower when docked to 5LC0 (−7.4 kcal/mol) compared to 5GJ4 (−8.3 kcal/mol) and 5GPI (−8.2 kcal/mol), indicating that the ZIKV protease crystal structure 5GPI or 5GJ4 could be more suitable for the in silico screening. This could be due to the fact that the 5GPI structure was crystallized in the unbound form and 5GJ4 was crystallized while bound to the tetra peptide TGKR released after the protease self-cleavage. 5LC0, on the other hand, was co-crystallized with an irreversible inhibitor making the binding site more prone to fit particular ligands. Finally, we used the LIE method in combination with MD simulations performed with the Q6 program, to estimate the binding free energies of the compounds 9b and 86 in complex with the ZIKV proteases 5CL0 and 5GPI. The LIE method, while not able to exactly reproduce the calculated experimental binding affinities, it correctly predicted that compound 9b to have higher binding affinity than compound 86 independently from the ZIKV proteases used (). Using of ZIKV protease 5GPI resulted in average binding energies with lower standard errors compared to the results obtained from the ZIKV protease 5LC0 (). Moreover, when using the ZIKV protease 5LC0, the binding free energy of the compound 86 was overestimated by −2.77 kcal/mol, thus suggesting again that the ZIKV protease 5GPI could be more suited for the in silico applications. When substituting the average differences between the estimated and the experimental binding free energies (4.53 ± 0.26 kcal/mol) for the compounds 9b and 86 in complex with 5GPI, to the constant γ, the accuracy of the results were greatly increased. It therefore appears that for the accurate prediction of absolute binding free energy of ligands, the empirical parameterization of γ, through modeling of binding free energies calculated from several different known ZIKV protease inhibitors, is necessary. Overall, the in silico methods used herein allowed us to discover a non-covalent, non-peptide, and less polar hit compound as compared to previously discovered positively charged substrate analogues for the ZIKV protease. This symmetrical diol compound that was originally developed against the HIV protease, can now serve as a good starting point for the development of an orally available ZIKV protease inhibitor. Compound 9b, and other diol based compounds, could also serve as a starting point for the development of treatment against other members of the flavivirus genus. However, the potential panflavivirus inhibitory effects of these compounds remain to be assessed.
Table 2. Ligands Average energies values and standard errors (kcal/mol) calculated from the Q6 MD simulations.
Author contributions
D.A., N.P. and J.L. conceived and designed the study. D.A performed docking and molecular dynamics simulations experiments. D.A performed in vitro enzymatic assays. P.K.C and A.S synthesized compound 9b. A.B and A.S synthesized compound 86. All authors analyzed the data and contributed to the scientific discussion. The manuscript was written through contributions of all authors and all authors have given approval to the final version of the manuscript.
Abbreviations | ||
FRET | = | Fluorescence energy transfer |
HIV | = | Human immunodeficiency virus |
LIE | = | Linear interaction energy |
MD | = | Molecular dynamics |
NS | = | Non-structural |
ORF | = | Open reading frame |
PI | = | Protease inhibitor |
RFU | = | Reference fluorescence units |
Rg | = | Radius of gyration |
RMSD | = | Root mean square deviation |
ZIKV | = | Zika virus |
Supplementary_information_JBSD.docx
Download MS Word (13.7 MB)Acknowledgements
We thank the Protein Science Facility (PSF) at the Karolinska Institute, SciLifeLab for help with the production of the Zika virus protease. We also thank the Swedish National Infrastructure (SNIC) for providing the advanced computational resources through the Uppsala Multidisciplinary center for Advanced Computational Science (UPPMAX) under the projects SNIC2016-1-535, SNIC2018-3-252 and SNIC2019-3-312. P. K. Chinthakindi and A. Båhlström acknowledge the Department of Medicinal Chemistry, Uppsala University, Sweden, for fellowships. Anders Bergkvist (Uppsala University) is acknowledge for the help provided with the statistical calculations and Lucas Brock (Uppsala University) for his help with the enzymatic assay.
Disclosure statement
The authors declare no competing financial interest.
Additional information
Funding
References
- Akaberi, D., Bergfors, A., Kjellin, M., Kameli, N., Lidemalm, L., Kolli, B., … Lennerstrand, J. (2018). Baseline dasabuvir resistance in Hepatitis C virus from the genotypes 1, 2 and 3 and modeling of the NS5B-dasabuvir complex by the in silico approach. Infection Ecology & Epidemiology, 8(1). doi:10.1080/20008686.2018.1528117
- Almlöf, M., Carlsson, J., & Åqvist, J. (2007). Improving the accuracy of the linear interaction energy method for solvation free energies. Journal of Chemical Theory and Computation, 3(6), 2162–2175. doi:10.1021/ct700106b
- Bauer, P., Barrozo, A., Purg, M., Amrein, B. A., Esguerra, M., Wilson, P. B., … Kamerlin, S. C. L. (2018). Q6: A comprehensive toolkit for empirical valence bond and related free energy calculations. SoftwareX, 7, 388–395. doi:10.1016/j.softx.2017.12.001
- Behnam, M. A. M., Graf, D., Bartenschlager, R., Zlotos, D. P., & Klein, C. D. (2015). Discovery of nanomolar Dengue and West Nile virus protease inhibitors containing a 4-benzyloxyphenylglycine residue. Journal of Medicinal Chemistry, 58(23), 9354–9370. doi:10.1021/acs.jmedchem.5b01441
- Cao-Lormeau, V.-M., Roche, C., Teissier, A., Robin, E., Berry, A.-L., Mallet, H.-P., … Musso, D. (2014). Zika Virus, French Polynesia, South Pacific, 2013. Emerging Infectious Diseases, 20(6), 1084–1086. doi:10.3201/eid2006.140138
- Chambers, T. J., Hahn, C. S., Galler, R., & Rice, C. M. (1990). Flavivirus genome organization, expression, and replication. Annual Review of Microbiology, 44(1), 649–688. doi:10.1146/annurev.mi.44.100190.003245
- Chan, J. F.-W., Chik, K. K.-H., Yuan, S., Yip, C. C.-Y., Zhu, Z., Tee, K.-M., … Yuen, K.-Y. (2017). Novel antiviral activity and mechanism of bromocriptine as a Zika virus NS2B-NS3 protease inhibitor. Antiviral Research, 141, 29–37. doi:10.1016/j.antiviral.2017.02.002
- Chappell, K. J., Stoermer, M. J., Fairlie, D. P., & Young, P. R. (2006). Insights to substrate binding and processing by West Nile Virus NS3 protease through combined modeling, protease mutagenesis, and kinetic studies. Journal of Biological Chemistry, 281(50), 38448–38458. doi:10.1074/jbc.M607641200
- Cheng, Y., & Prusoff, W. H. (1973). Relationship between the inhibition constant (K1) and the concentration of inhibitor which causes 50 per cent inhibition (I50) of an enzymatic reaction. Biochemical Pharmacology, 22(23), 3099–3108. doi:10.1016/0006-2952(73)90196-2
- Dodda, L. S., Cabeza de Vaca, I., Tirado-Rives, J., & Jorgensen, W. L. (2017). LigParGen web server: An automatic OPLS-AA parameter generator for organic ligands. Nucleic Acids Research, 45(W1), W331–W336. doi:10.1093/nar/gkx312
- D’Ortenzio, E., Matheron, S., Yazdanpanah, Y., de Lamballerie, X., Hubert, B., Piorkowski, G., … Leparc-Goffart, I. (2016). Evidence of sexual transmission of Zika virus. New England Journal of Medicine, 374(22), 2195–2198. doi:10.1056/NEJMc1604449
- Driggers, R. W., Ho, C.-Y., Korhonen, E. M., Kuivanen, S., Jääskeläinen, A. J., Smura, T., … Vapalahti, O. (2016). Zika virus infection with prolonged maternal viremia and fetal brain abnormalities. New England Journal of Medicine, 374(22), 2142–2151. doi:10.1056/NEJMoa1601824
- Duffy, M. R., Chen, T.-H., Hancock, W. T., Powers, A. M., Kool, J. L., Lanciotti, R. S., … Hayes, E. B. (2009). Zika virus outbreak on Yap Island, Federated States of Micronesia. New England Journal of Medicine, 360(24), 2536–2543. doi:10.1056/NEJMoa0805715
- Halgren, T. A. (1996). Merck molecular force field. I. Basis, form, scope, parameterization, and performance of MMFF94. Journal of Computational Chemistry, 17(5-6), 490–519. doi:10.1002/(SICI)1096-987X(199604)17:5/6<490::AID-JCC1>3.0.CO;2-P
- Hansson, T., Marelius, J., & Åqvist, J. (1998). Ligand binding affinity prediction by linear interaction energy methods. Journal of Computer-Aided Molecular Design, 12(1), 27–35. doi:10.1023/A:1007930623000
- Hess, B., Kutzner, C., van der Spoel, D., & Lindahl, E. (2008). GROMACS 4: Algorithms for highly efficient, load-balanced, and scalable molecular simulation. Journal of Chemical Theory and Computation, 4(3), 435–447. doi:10.1021/ct700301q
- Hsu, K.-C., Chen, Y.-F., Lin, S.-R., & Yang, J.-M. (2011). iGEMDOCK: A graphical environment of enhancing GEMDOCK using pharmacological interactions and post-screening analysis. BMC Bioinformatics, 12(Suppl 1), S33. doi:10.1186/1471-2105-12-S1-S33
- Jorgensen, W. L., Maxwell, D. S., & Tirado-Rives, J. (1996). Development and testing of the OPLS All-atom force field on conformational energetics and properties of organic liquids. Journal of the American Chemical Society, 118(45), 11225–11236. doi:10.1021/ja9621760
- Kim, S., Thiessen, P. A., Bolton, E. E., Chen, J., Fu, G., Gindulyte, A., … Bryant, S. H. (2016). PubChem substance and compound databases. Nucleic Acids Research, 44(D1), D1202–1213. doi:10.1093/nar/gkv951
- Kuiper, B. D., Slater, K., Spellmon, N., Holcomb, J., Medapureddy, P., Muzzarelli, K. M., … Kovari, L. C. (2017). Increased activity of unlinked Zika virus NS2B/NS3 protease compared to linked Zika virus protease. Biochemical and Biophysical Research Communications, 492(4), 668–673. doi:10.1016/j.bbrc.2017.03.108
- Kuno, G., & Chang, G.-J. J. (2007). Full-length sequencing and genomic characterization of Bagaza, Kedougou, and Zika viruses. Archives of Virology, 152(4), 687–696. doi:10.1007/s00705-006-0903-z
- Lee, H., Ren, J., Nocadello, S., Rice, A. J., Ojeda, I., Light, S., … Johnson, M. E. (2017). Identification of novel small molecule inhibitors against NS2B/NS3 serine protease from Zika virus. Antiviral Research, 139, 49–58. doi:10.1016/j.antiviral.2016.12.016
- Lei, J., Hansen, G., Nitsche, C., Klein, C. D., Zhang, L., & Hilgenfeld, R. (2016). Crystal structure of Zika virus NS2B-NS3 protease in complex with a boronate inhibitor. Science, 353(6298), 503–505. doi:10.1126/science.aag2419
- Leung, D., Abbenante, G., & Fairlie, D. P. (2000). Protease inhibitors: Current status and future prospects. Journal of Medicinal Chemistry, 43(3), 305–341. doi:10.1021/jm990412m
- Leuw, P. D., & Stephan, C. (2018). Protease inhibitor therapy for hepatitis C virus-infection. Expert Opinion on Pharmacotherapy, 19(6), 577–587. doi:10.1080/14656566.2018.1454428
- Li, Y., Zhang, Z., Phoo, W. W., Loh, Y. R., Wang, W., Liu, S., … Kang, C. (2017). Structural dynamics of Zika virus NS2B-NS3 protease binding to dipeptide inhibitors. Structure, 25(8), 1242–1250.e3. doi:10.1016/j.str.2017.06.006
- Lim, H., Nguyen, T. T. H., Kim, N. M., Park, J.-S., Jang, T.-S., & Kim, D. (2017). Inhibitory effect of flavonoids against NS2B-NS3 protease of ZIKA virus and their structure activity relationship. Biotechnology Letters, 39(3), 415–421. doi:10.1007/s10529-016-2261-6
- Lv, Z., Chu, Y., & Wang, Y. (2015). HIV protease inhibitors: A review of molecular selectivity and toxicity. HIV/AIDS - Research and Palliative Care, 7, 95–104. 10.2147/HIV.S79956.
- MacNamara, F. N. (1954). Zika virus: A report on three cases of human infection during an epidemic of jaundice in Nigeria. Transactions of the Royal Society of Tropical Medicine and Hygiene, 48(2), 139–145. doi:10.1016/0035-9203(54)90006-1
- Nitsche, C., Zhang, L., Weigel, L. F., Schilz, J., Graf, D., Bartenschlager, R., … Klein, C. D. (2017). Peptide-boronic acid inhibitors of flaviviral proteases: Medicinal chemistry and structural biology. Journal of Medicinal Chemistry, 60(1), 511–516. doi:10.1021/acs.jmedchem.6b01021
- O’Boyle, N. M., Banck, M., James, C. A., Morley, C., Vandermeersch, T., & Hutchison, G. R. (2011). Open Babel: An open chemical toolbox. Journal of Cheminformatics, 3, 33. doi:10.1186/1758-2946-3-33
- Oehler, E., Watrin, L., Larre, P., Leparc-Goffart, I., Lastere, S., Valour, F., … Ghawche, F. (2014). Zika virus infection complicated by Guillain-Barre syndrome–case report, French Polynesia, December 2013. Eurosurveillance, 19(9). 10.2807/1560-7917.es2014.19.9.20720.
- Palanisamy, N., Akaberi, D., & Lennerstrand, J. (2017). Protein backbone flexibility pattern is evolutionarily conserved in the Flaviviridae family: A case of NS3 protease in Flavivirus and Hepacivirus. Molecular Phylogenetics and Evolution, 118, 58–63. doi:10.1016/j.ympev.2017.09.015
- Phoo, W. W., Li, Y., Zhang, Z., Lee, M. Y., Loh, Y. R., Tan, Y. B., … Luo, D. (2016). Structure of the NS2B-NS3 protease from Zika virus after self-cleavage. Nature Communications, 7(1), 13410. doi:10.1038/ncomms13410
- Pyring, D., Lindberg, J., Rosenquist, Å., Zuccarello, G., Kvarnström, I., Zhang, H., … Samuelsson, B. (2001). Design and synthesis of potent C2-symmetric diol-based HIV-1 protease inhibitors: Effects of fluoro substitution. Journal of Medicinal Chemistry, 44(19), 3083–3091. doi:10.1021/jm001134q
- Rawlings, N. D., Barrett, A. J., & Finn, R. (2016). Twenty years of the MEROPS database of proteolytic enzymes, their substrates and inhibitors. Nucleic Acids Research, 44(D1), D343–D350. doi:10.1093/nar/gkv1118
- Roth, A., Mercier, A., Lepers, C., Hoy, D., Duituturaga, S., Benyon, E., … Souarès, Y. (2014). Concurrent outbreaks of dengue, chikungunya and Zika virus infections – an unprecedented epidemic wave of mosquito-borne viruses in the Pacific 2012–2014. Eurosurveillance, 19(41), 20929. doi:10.2807/1560-7917.ES2014.19.41.20929
- Sandeep, G., Nagasree, K. P., Hanisha, M., & Kumar, M. M. K. (2011). AUDocker LE: A GUI for virtual screening with AUTODOCK Vina. BMC Research Notes, 4 (1), 445. doi:10.1186/1756-0500-4-445
- Simmonds, P., Becher, P., Collett, M. S., Gould, E. A., Heinz, F. X., Meyers, G., … Bukh, J. (2011). Family Flaviviridae. In Virus taxonomy, classification and nomenclature of viruses. Ninth report of the International Committee on Taxonomy of Viruses (1st ed., pp. 1004–1010). The Netherlands: Elsevier.
- Sirohi, D., Chen, Z., Sun, L., Klose, T., Pierson, T. C., Rossmann, M. G., & Kuhn, R. J. (2016). The 3.8 Å resolution cryo-EM structure of Zika virus. Science, 352(6284), 467–470. doi:10.1126/science.aaf5316
- Trott, O., & Olson, A. J. (2010). AutoDock Vina: Improving the speed and accuracy of docking with a new scoring function, efficient optimization and multithreading. Journal of Computational Chemistry, 31(2), 455–461. doi:10.1002/jcc.21334
- World Health Organization. (2016). Zika situation report: Zika virus, microcephaly and Guillain Barré syndrome. Retrieved from https://apps.who.int/iris/bitstream/handle/10665/204491/zikasitrep_26Feb2016_eng.pdf;jsessionid=0AD32F347339C536879C0EC1777A779A?sequence=1
- Yuan, S., Chan, J. F.-W., den-Haan, H., Chik, K. K.-H., Zhang, A. J., Chan, C. C.-S., … Yuen, K.-Y. (2017). Structure-based discovery of clinically approved drugs as Zika virus NS2B-NS3 protease inhibitors that potently inhibit Zika virus infection in vitro and in vivo. Antiviral Research, 145, 33–43. doi:10.1016/j.antiviral.2017.07.007
- Zhang, Z., Li, Y., Loh, Y. R., Phoo, W. W., Hung, A. W., Kang, C., & Luo, D. (2016). Crystal structure of unlinked NS2B-NS3 protease from Zika virus. Science, 354(6319), 1597–1600. doi:10.1126/science.aai9309