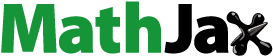
Abstract
Zearalenone is an estrogenic mycotoxin which is a common food contaminant and has been implicated in increasing the incidence of carcinogenesis and other reproductive health ailments through the estrogen receptor alpha (ERα) pathway. Competitive ERα blockers such as 4-Hydroxytamoxifen (OHT), are synthetic FDA approved drugs which, albeit being an effective anticancer agent, induces life altering side effects. For this reason, there is an increased interest in the use of naturally occurring medicinal plant products such as flavonoids. This study aimed to identity flavonoid ERα inhibitors and provide insights into the mechanism of inhibition using computational techniques. The Molecular Mechanics/Generalized Born Surface Area calculations revealed that quercetrin, hesperidin, epigallocatechin 3-gallate and kaempferol 7-O-glucoside out of 14 flavonoids had higher binding affinity for ERα than OHT. The structural analysis revealed that the binding of the compounds to the receptor lead to dynamic alterations, which induced conformational shift in the structure and orientation of the receptor resulting in stabilised, compact and low energy systems. The results of this study provide imperative information that supports the use of flavonoids in the inhibition of ERα to prevent or ameliorate the consequential adverse effects associated with zearalenone exposure.
Communicated by Ramaswamy H. Sarma
Introduction
Mycotoxins are secondary metabolites produced by fungi that can be toxic to man and animals and are ubiquitously found in the environment. Common ones include aflatoxin, fumonisins, ochratoxin A, citrinin, ergot alkaloids, trichothecenes, patulin, and zearalenone (Andersen et al., Citation2018). Unlike the other mycotoxins, zearalenone exhibits estrogenicity and hence referred to as a mycoestrogen (Andersen et al., Citation2018).
Zearalenone (ZEA) is a non-steroidal mycoestrogen produced by several Fusarium fungi species especially F. graminearum and F. cuolmorum (Bennett & Klich, Citation2003; Kuiper-Goodman et al., Citation1987). These species of fungi are commonly found in human and animal foodstuffs such as cereal crops, dried fruits, spices, wine, beer and meat products. Contamination of cereal crops with ZEA generally occurs during pre-harvest and persists thereafter due to the mycotoxin’s high resistance to elevated food processing temperature and pressure conditions. This mechanism allows the bioaccumulation of ZEA in humans and animals that consume the contaminated produce (Andersen et al., Citation2018).
Chemically described as 6-(10-hydroxy-6-oxo-trans-1-undecenyl)-β-resocyclic acid lactone, the structural similarity of ZEA () to the endogenous estrogens such as 17β-estradiol () plus the high binding affinity to the estrogen receptors are the reasons for its harmful estrogenic activities. ZEA has been implicated in carcinogenesis (breast cancer, oesophageal cancer, cervical cancer, prostate cancer) through estrogen signaling which influences DNA synthesis and cell reproduction (Ahmed et al., Citation2017; Claeys et al., Citation2020; Kowalska et al., Citation2018; Luo et al., Citation1990). Animal studies have reported ZEA induced infertility, spontaneous abortions and still births. ZEA also modulates the synthesis of hormones (estradiol, cortisol, testosterone and progesterone) by binding to estrogen receptors (ERα and ERβ), leading to the transcriptional activation of estrogen responsive genes (Andersen et al., Citation2018).
Figure 1. Chemical structures of the ERα ligands. (A) Estradiol, the endogenous stimulatory ERα-ligand (ChemSpider ID: 5554). (B) ZEA, the stimulatory mycoestrogen (ChemSpider ID: 4444897). (C) 4-Hydroxytamoxifen, the synthetic selective estrogen-receptor modulator (ChemSpider ID: 395987).
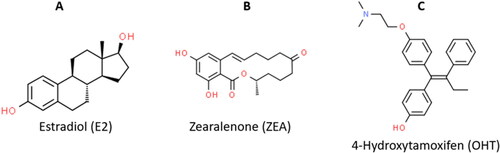
Selective estrogen receptor modulators (SERMs) such as 4-Hydroxytamoxifen (used to treat breast cancer, ), Clomiphene (used to treat infertility), and Raloxifene (used to treat osteoporosis), which acts as competitive blockers of the estrogen/ZEA binding to the ER, have been used successfully (Y. Kim et al., 2020; Neven et al., 2018; Qin et al., Citation2020). Although these drugs are efficacious, they are associated with drug resistance and life altering side effects such as uterine cancer, endometrial cancer, menopausal symptoms, edema, pulmonary embolism and so on (Ignatov & Ortmann, Citation2020; Jeon et al., Citation2020; Zhang et al., Citation2016). Hence, the worldwide interest in the use of naturally occurring medicinal plant compounds such as flavonoids to circumvent debilitating side effects.
Flavonoids are phytochemicals synthesised as secondary metabolites in several parts of plants where they function as growth factors and a defence mechanism from infection and other stressors (Santos et al., Citation2017). They have been associated with a broad spectrum of health-promoting effects and are an indispensable component in a variety of nutraceutical, pharmaceutical, medicinal and cosmetic applications (Panche et al., Citation2016). They have antioxidative, anti-inflammatory, anti-mutagenic and anti-carcinogenic properties coupled with their capacity to modulate key cellular enzyme functions and are potent SERMs (Panche et al., Citation2016). Flavonoids are polyphenolic compounds consisting of 15-carbon skeleton: two phenyl rings (A and B) and a heterocyclic ring (ring C) (see ). They are classified into 6 subgroups (Flavones, anthocyanidins, flavanones, flavanols, isoflavones, flavonols) based on the degree of oxidation and hydroxylation of the ring structures (Panche et al., Citation2016). The hydroxylation often occurs at positions 3,5,7,3´,4´ and 5´() and often these hydroxyl groups are sulphated, methylated or acetylated (Santos et al., Citation2017). Flavonoids naturally occur as aglycones or glycosides with the sugar (D-glucose, L-rhamnose, glucose rhamnose, arabinose or galactose) moiety conjugated at either position 3 or 7. (Santos et al., Citation2017). Under natural conditions, most of the flavonoids are present in glycoside form. The modification to their structures alters their stability, reactivity and solubility (Santos et al., Citation2017). The hydrophobic and polar components on the rings interact with amino acid residues within the ligand binding domain of Estrogen receptors.
Figure 2. Basic chemical structures of several different flavonoid classes (Panche et al., Citation2016).
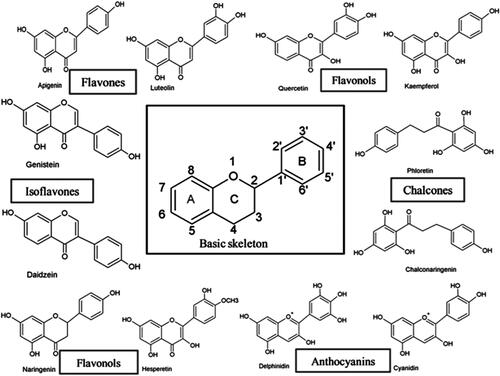
The estrogen receptor α (ERα) is a ligand activated transcription factor (Yaşar et al., Citation2017). The ERα has 6 functional domains: N-terminal (A/B) domain, the DNA-binding domain (C), the hinge domain (D) and the ligand binding domain (LBD, E/F).
The LBD is responsible for encompassing the ligand, it consists of a co-regulator interaction site (activation function 2, AF2), dimerisation interface and a binding pocket (Kumar et al., Citation2011). This domain has 12 alpha helices (H1-H12) and two beta sheets (S1 and S2) (see ) (Brzozowski et al., Citation1997). The binding of a ligand to the LBD induces dynamic conformational changes in the helical structures of the binding pocket (BP), H12 conformational changes are mainly responsible for overall receptor activation (Puranik et al., Citation2019).
Figure 3. X-ray crystallography structures of the ERα ligand binding domain (LBD). (A) The LBD encompasses helices: H1-H12. The ligand binding pocket is comprised of H3, H6, H8, H11 and H2, PDB ID: 3ert. (B) ERα LBD complexed with zearalenone in the active/closed conformation, PDB ID: 5krc. (C) ERα LBD complexed with 4-hydroxytamoxifen in the inactive/open conformation, PDB ID: 3ert. (D) The apo form of ERα LBD complexed with 17β-estradiol, PDB ID: IA52.
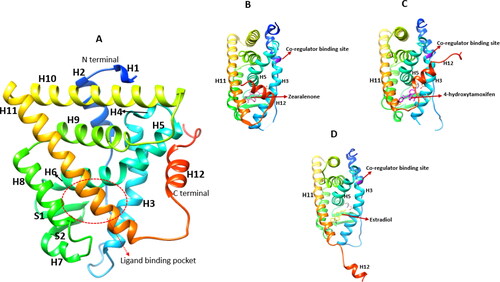
When 17β-estradiol (E2) binds to the BP, it rests within the hydrophobic core of the BP formed by H3, H6, H8, H11 and H12 (see ). The hydrophobic core of E2 forms non-polar interactions with the hydrophobic residues of the BP, the phenolic hydroxyl group from the A ring forms hydrogen bonds with Glu353 in H3 and Arg394 in H6 while the 17β-hydroxyl group of the D ring forms a hydrogen bond with His524 (H11) (Brzozowski et al., Citation1997). A similar picture is seen upon ZEA binding to the BP, the hydroxyl group on position 14 forms a hydrogen bond with Glu353 while the keto/hydroxyl group in 6th position is bonded with Hie524 (Ehrlich et al., Citation2015; Zhang et al., Citation2019). The combination of the hydrophobic and polar interactions of ZEA and E2 within the BP results in an active/closed conformation. This shift in conformation causes the inner hydrophobic surface of helix H12 to be positioned across H3 and H11 thus forming a lid over the cavity to accommodate co-regulator binding (), which ultimately leads to enhanced gene transcription (Brzozowski et al., Citation1997; Shtaiwi et al., Citation2019).
Conversely, ERα competitive blockers such as 4-hydroxytamoxifen (OHT) and raloxifene occupy the same hydrophobic BP as ZEA and E2. The phenolic hydroxyl group of OHT interacts with Glu353 and Arg394, while the dimethylaminoethoxy group side chain lies near Asp351 and forms a direct hydrogen bond. The bulky side chain protrudes from the BP between H3 and H11 preventing the hydrophobic interactions of H12 with H3/H11 thus attaining an inactive/open conformation where H12 is positioned directly opposite H3 and H5, occupying the co-regulator binding site (), which reduces the level of estrogen-regulated gene transcription (Pike et al., Citation1999; Shtaiwi et al., Citation2019). However, the unbound form of ERα exhibits a unique conformation in which H12 does not share hydrophobic interactions with the BP instead it is directed away from the LBD core, as depicted in (Shtaiwi et al., Citation2019).
Over the last few decades, computer-aided drug design (CADD) strategic methods have greatly contributed toward the drug discovery, design and development that act against a wide diversity of bacteria, viruses and diseases (Yu & MacKerell, Citation2017). Computational techniques are frequently used to rapidly facilitate the discovery process and intentionally direct focus toward drug compounds that express potential pharmacological activities, provide enhancements for promising candidates and intricately details enzyme-drug interactions (Das et al., Citation2017; Kore et al., Citation2012).
Virtual screening (VS) is a process that utilises a series of computational tools to search through compound databases/libraries for suitable small molecules that have similar structuring to the template scaffold. Structures identified through VS should bind to the amino acid residues within the target’s active site (Appiah-Kubi & Soliman, Citation2017; Berinyuy & Soliman, Citation2017; N Mhlongo & ES Soliman, 2016). Structure-based VS searches for potential ligand candidates primarily based on the 3 D-structure of a target enzyme’s active binding pocket. On the other hand, ligand-based VS searches for favourable ligand features with known bioactive properties for pharmacophore model generation (Cele et al., Citation2016). Pharmacophore/ligand-based VS involve intermolecular interactions, which include hydrogen bonding, electrostatic energies and charge. This approach also screens for potential drug candidates that form interactions with highly contributing residues based on the binding free energy (Islam & Pillay, Citation2015).
Herein, we implemented molecular dynamics (MD) simulations, and post dynamic analysis to identify potential ERα inhibitors. This study provides the fundamental basis that will facilitate the selection of natural products with better suited antiestrogenic properties to ameliorate ZEA-induced pathologies.
Computational methodology
System preparation
The X-ray crystallographic structure of human ERα LBD complexed with OHT was obtained from the protein data bank (PDB code: 3ERT) (Burley et al., 2019). The complex was then processed using the UCSF Chimera software (Pettersen et al., Citation2004). The list of flavonoids was obtained from the Biological Magnetic Resonance Data Bank (BMRB) (Romero et al., Citation2020). We obtained 30 flavonoids (: supplementary materials) from the BMRB and docked all of them against the estrogen receptor. Furthermore, all the docked complexes with dock scores more than 8 were selected for further analysis (Molecular Mechanics/GB Surface Area method-MM/GBSA).
Table 1. Flavonoid compounds obtained from the Biological Magnetic Resonance Data Bank.
All 2-D () and 3-D ligand structures were obtained from PubChem (S. Kim et al., Citation2019) and processed using the chimera software (Pettersen et al., Citation2004). All 14 ligands including OHT, which was used as a ERα inhibitor reference, were docked to the Erα-LBD.
Molecular docking
The compounds were docked into the LBD of ERα. The docking protocol was validated by redocking the original substrate OHT to the processed LBD and superimposing the redocked complex to the original crystal structure. The RMSD obtained was 0.004 Å (). In preparation for docking, non-polar hydrogen atoms were merged to carbon atoms and Gasteiger charges were added to all ligands docked into the binding pocket of the ERα LBD through UCSF Chimera (Pettersen et al., Citation2004), using the AutoDock Vina plugin (Pettersen et al., Citation2004), compounds were docked by means of the grid box function to express the binding site (spacing of 1Å was marked out at a size of 22.3554 × 22.7154 × 26.9884 in the x, y and z three-dimensional axis respectively. All the docked complexes were then subjected to molecular dynamic simulations.
Molecular dynamic (MD) simulations
Molecular dynamics (MD) simulation can provide not only plentiful dynamical structural information on biomacromolecules but also a wealth of information concerning the energetics of protein and ligand interactions. Such information is very important to understanding the structure-function relationship of the target molecules, the essence of protein–ligand interactions and to guiding the drug discovery and design process. Thus, MD simulations have been applied widely and successfully in each step of modern drug discovery (Liu et al., Citation2018).
The MD simulations were performed using AMBER18 with GPU acceleration (Hollingsworth & Dror, Citation2018). The enzyme was dehydrogenated and parameterized using the AMBER FF18SB force field (Hollingsworth & Dror, Citation2018). Hydrogen atoms were added to the ligands and charged via Gasteiger charges. The antechamber module was used to generate partial atomic charges for the ligands by applying General Amber Force Field (GAFF) and restrained electrostatic potential (RESP) procedures (Hollingsworth & Dror, Citation2018). The LEAP module was applied to neutralise and solvate the systems (Le Grand et al., Citation2013) by adding hydrogen, sodium and chloride atoms, whilst suspending them in an orthorhombic box of TIP3P water molecules so that all atoms were within 8 Å of the box edges. The amino acid residues of the LBD were numbered 305-554.A 2000 step partial minimisation was performed, while applying a 1000 step restraint potential of 500 Kcal/mol, using the steepest descent method followed by 1000 steps of conjugate gradients. Furthermore, 1000 steps of full minimisation were executed using conjugate gradient algorithm without restraint. A heating MD simulation from 0 K to 300 K was gradually carried out for 50 ps, maintaining a fixed number of atoms and volume. A potential harmonic restraint of 10 kcal/mol and collision frequency of 1.0ps were applied on to all the solutes in the system and Langevin thermostat with collision frequency of 1.0 ps−1 was applied to each system. After heating, about 500ps of equilibration was executed while keeping a constant operating temperature at 300 K. Features such as several atoms and pressure were also kept constant mimicking an isobaric-isothermal ensemble. The Berendsen barostat was used to preserve the system pressure at 1 bar and the SHAKE algorithm was utilized for hydrogen bond constraint (Gonnet, Citation2007; Le Grand et al., Citation2013). SPFP (Single precision with fixed point integer arithmatic) precision model was used and the step size of each simulation was 2fs (Le Grand et al., Citation2013).
Post simulation analysis
The coordinates of the systems were saved, and the trajectories were analyzed for every ps using CPPTRAJ and PTRAJ modules in the Amber18 package (Shunmugam & Soliman, Citation2018). The following post analysis were conducted root mean square deviation (RMSD), root means square fluctuation (RMSF) and radius of gyration (RoG). The Origin data analysis software (Seifert, Citation2014) was used to plot data and generate graphs.
RMSD
The root mean square deviation (RMSD) is a similarity measure widely used to estimate the deviation in the Cα (alpha carbon)-atoms of protein residues indicative of the degree of protein stability (Dixit et al., Citation2006). The Cα RMSD plots of the apo and bound systems were analysed to assess the effect of flavonoid binding on the structural stability of the ERα-LBD.
RoG
Radius of gyration (RoG) is defined as the mass weighted root mean square distance of a collection of atoms from their common centre of mass. It is an indicator of protein structural compactness (Baig et al., Citation2014). This method of analysis allowed the assessment of ERα-LBD compactness upon flavonoid binding along the 200 ns trajectory in the MD simulation.
RMSF
Root means square fluctuation (RMSF) is a measure of the displacement of a particular atom, or group of atoms, relative to the reference structure, averaged over the number of atoms. It is frequently used to discern whether a structure is stable in the timescale of the simulations or if it is diverging from the initial coordinates (Martínez, Citation2015). The RMSF analysis provides insights into the flexibility of the ERα-LBD amino acid residues upon binding to the flavonoids.
Binding free energy calculations
The MD allows free energy differences to be accepted, which regulate the underlying mechanism of all biological processes (Gapsys et al., Citation2015).Binding free energy (BFE) calculations are a key tool for studying the in-depth binding mechanism between a protein and ligand, which involves both entropic and enthalpic contributions (Schauperl et al., Citation2017). The BFE is used in combination with the Molecular Mechanics/Generalized Born Surface Area (MM/GBSA) technique to estimate the binding affinity within a docked system. In contrast to a full-scale MD free energy perturbation/thermodynamic simulation, this approach can be used to provide reproducible relative binding affinities of compounds with good precision and needs significantly less computational resources (Shunmugam & Soliman, Citation2018).
The free binding energy was calculated using the Molecular Mechanics/GB Surface Area method (MM/GBSA) to estimate and compare the binding affinity of the systems. This method was employed as previously described (Idowu et al., Citation2020). Binding free energy was averaged over 200 000 snapshots extracted from the 200 ns trajectory. The free binding energy (ΔG) computed by this method for each molecular species (complex, ligand, and receptor) can be represented as:
(1)
(1)
(2)
(2)
(3)
(3)
(4)
(4)
(5)
(5)
The term Egas denotes the gas-phase energy, which consists of the internal energy Eint; Coulomb energy Eele and the van der Waals energies Evdw. The Egas was directly estimated from the FF14SB force field terms. Solvation free energy, Gsol, was estimated from the energy contribution from the polar states, GGB, and non-polar states, G. The non-polar solvation energy, SA. GSA, was determined from the solvent-accessible surface area (SASA), using a water probe radius of 1.4 Å. In contrast, the polar solvation, GGB, the contribution was estimated by solving the GB equation. S and T denote the total entropy of the solute and temperature, respectively.
Results and discussion
Thermodynamic binding free energy of the flavonoids to ERα
To determine the inhibitory potential of the flavonoids, the binding affinities of the flavonoids to the ERα was estimated by calculating their binding free energy (ΔGbind) using the MM/GBSA method. This method was previously used to calculate ΔGbind of ligands to receptors in several computational studies (Idowu et al., Citation2020; Mazurek et al., Citation2020; Shunmugam & Soliman, Citation2018). Following MD simulation of 200 ns, the binding energies of all the bound systems were calculated ().
Table 2. Thermodynamic binding free energy components (Kcal/mol) for all the flavonoids compared to OHT (reference drug).
The binding affinities of flavonoid bound systems were compared to the OHT complex. We postulated that compounds with the lowest ΔGbind are considered to have better binding affinity and enhanced inhibitory potential. HPN (-56.07 Kcal/mol), QRM (-55.63 Kcal/mol), EGCG (-48.09 Kcal/mol) and K7G (-46.51 Kcal/mol) had lower ΔGbind compared to OHT (-44.72 Kcal/mol). This finding may imply that these specific compounds express a much more favourable binding affinity for ERα than the reference synthetic drug, OHT, ultimately suggesting that HPN, QRM, EGCG and K7G are potentially better ERα Inhibitors.
Majority of previous studies based on the binding of flavonoids use docking scores to predict the binding affinity of flavonoids to ERα (Indira et al., Citation2020; Maruthanila et al., Citation2019; Pang et al., Citation2018; Powers & Setzer, Citation2015; Puranik et al., Citation2019; Widowati et al., Citation2019). To the best of our knowledge, this is one of a few studies to use the MM/GBSA method to estimate the binding affinity of flavonoids to ERα. As a consequence, to HPN, QRM, EGCG and K7G superior binding affinity, the compounds were selected for further structural and dynamical analysis.
ERα- ligand complex stability
The RMSD readings of alpha carbon (Cα) atoms throughout the 200 ns MD simulation of the apo and bound systems were analysed to assess the effect of the binding mechanisms of QRM, HPN, K7G and EGCG on the structural stability of the ER (). The RMSD analysis serves as a measure of system convergence and stability, which influences overall receptor function (Idowu et al., Citation2020). These measurements are determined by ligand-receptor interactions that arise from ligand movements in the binding pocket (BP) to achieve optimum geometric orientation (Mazurek et al., Citation2020).
Figure 6. (A) The RMSD plots of Cα for the apo (black), OHT (red), HPN (cyan) and QRM (purple). (B) The RMSD plots of Cα apo (black), OHT (red), K7G (green) and QRM (blue).
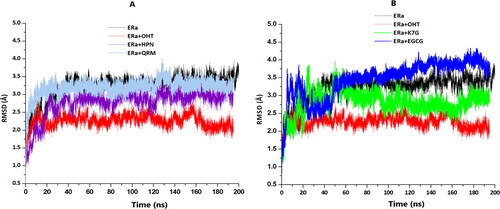
The RMSD plots showed that OHT, HPN and QRM complexes each converged at approximately 20 ns (), while the apo reached stability at 50 ns and the remaining systems converged at 60 ns (EGCG and K7G) ().
The findings of this study are similar to those observed in previous studies conducted by Celik et al. (Citation2007) and Shtaiwai et al. (2019) in which the Cα atom RMSD of a OHT complex converged at 20 ns and the apo system showed greater Cα atom deviations than the bound systems.
The results suggest that the binding of the ligands to the receptor stabilised the structural complexes following an initial period of high fluctuations in order to achieve the optimum geometric conformation (Mazurek et al., Citation2020).
To gain more insight into the stability and structural conformation of the flavonoid complexes, the radii of gyration (RoG) were analysed and graphically represented in . RoG is a measure of protein folding, stability and structural compactness. According to relevant figure, it is seen that the RoG value for the apo and OHT, HPN, EGCG, QRM and K7G-bound systems are reasonably similar, with an average value of 18.8Å, 18.6Å, 18.5Å,18.6Å, 18.7Å and 18.7Å respectively. These findings suggest that compound binding to the LBD of ERα induced a conformational shift to a slightly more compact structure, despite there only being a minimal change in distance, [OHT (0.2Å), HPN (0.3Å), EGCG (0.2Å), QRM (0.1Å) and K7G (0.1Å)], resulting in a steady and lower energy system. This will ultimately form a relatively stable protein (Shunmugam and Soliman. 2018).
Figure 7. Radii of gyration (RoG)of apo and bound systems were measured over a 200 ns simulation. Data obtained were presented as a plot exhibiting the differences arising in radius deviation between the apo and bound systems. (A) The RoG plots for apo(black), OHT (red), HPN (cyan) and QRM (purple). (B) The RoG plots for apo (black), OHT (red), K7G (green) and QRM (blue).
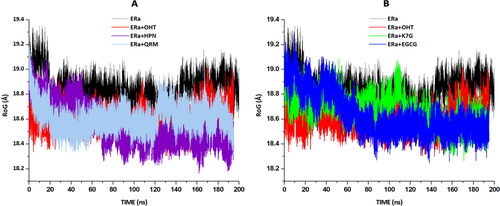
ERα- ligand complex residue fluctuations
The RMSF values of the apo and bound systems were analysed to determine the binding effects of OHT, HPN, EGCG, QRM and K7G on the dynamic residue behaviour within the ERα-LBD. The RMSF with respect to the averaged MD simulation conformation was used to demonstrate the differences occurring in residue flexibility.
The RMSF of all residues were calculated for flexibility of the structure backbone and graphically depicted in . The RMSF values of HPN, EGCG, QRM and K7G were compared to OHT.
Figure 8. (A) The RMSF plots for the apo system (black), OHT complex (red), HPN (cyan) complex and QRM (purple) complex. (B) The RMSF plots for the apo system (black), OHT (red) complex, K7G (green) complex and QRM (blue) complex.
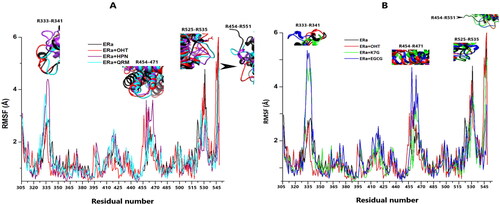
All systems, free and bound, exhibited increased dynamic fluctuations of the amino acid residues (R) in four specific regions corresponding to: loops preceding H3 (R333-R341), H9 (R454-R471) and those surrounding the terminal helix H12 (R525-R535 and R545-R551). This finding is congruent with previous studies in literature (Fratev, Citation2015; McGee et al., Citation2008; Ng, Citation2016; Shtaiwi et al., Citation2019)
The most evident amino acid fluctuations observed in the apo system was identified as residues that surround H12 (). These findings are consistent with the RMSD and RoG data obtained. The flexibility of the Cα atoms as reflected in the RMSD plots and the loose structural configuration represented in the RoG graphs is attributed to the amino acid residue movements in these regions () (Ng, Citation2016). The residue variations in these regions could be due to the lack of ligand interactions, which lock to keep the helices in place for appropriate receptor function (Ye et al., Citation2018). This occurrence was also observed in a previous study whereby removal of the ligand from the ERα BP led to increased residue fluctuations specifically in the loop region located at the head of H12 (McGee et al., Citation2008).
Table 3. The average Cα RMSF values of specific regions of the ER LBC.
Similarly, the OHT, QRM and K7G bound system residues exhibited their highest movements in the terminal H12 regions while that of the EGCG complex were lowest in these regions. The flexibility seen in the OHT bound system is attributed to the conformation of H12 attained when the bulky side chain for OHT protrudes from the BP between H3 and H11, thus preventing hydrophobic interactions of H12 with the core of the binding pocket (Brzozowski et al., Citation1997). All the polar and hydrophobic interactions in the QRM and K7G complexes were localised in H3 and H5 (). The lack of interactions with the residues of H11 and H12 accounts for increased movements in the loops surrounding these helices because they are devoid of the ligand interactions which would restrict their flexibility (Ye et al., Citation2018). However, EGCG formed 6 out of its 8 hydrogen bonds with residues of H11 and H12. The presence of the EGCG hydrogen bonds pulls the helices inwards, and locks them in place, hence reducing their movement.
Figure 9. The graphical representation of HPN, QRM, EGCG and K7G residue interactions within the LBD of ERα.
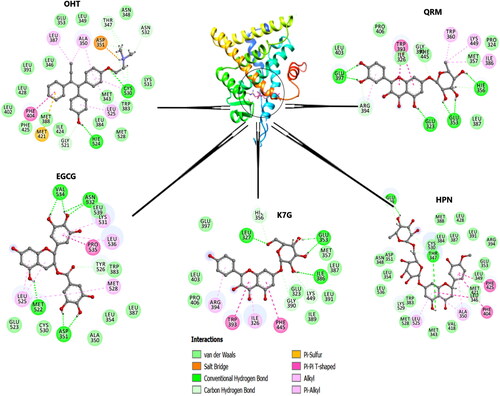
Contrastingly, the residue fluctuations of the HPN complex were highest in the H3 and H9 regions (). Six out of the seven hydrogen bonds in the HPN complex are in H3 and H6 (). The presence of these interactions are likely to reposition the helical residues towards HPN (Ye et al., Citation2018) and this indicates that during this phenomenon, the loops that surround the affected helices are more than likely to exhibit increased dynamic fluctuations (Brzozowski et al., Citation1997).
Table 4. The flavonoid- ERα interaction details. The number of interactions and the interacting residues.
ERα and ligand interactions
The structural properties of flavonoids determine the nature of their interactions with the amino acid residues of the Erα-LBD pocket. To assess the binding mechanisms of QRM, HPN, K7G and EGCG with the receptor’s amino acid residues, snapshots of each compound were captured at 200 ns of the MD simulation and subjected to analysis ().
As observed in , HPN had the highest number of interactions (26) and carbon hydrogen: conventional hydrogen bond ratio (5:2), which is comparable to OHT with 26 interactions and a 7:2 carbon hydrogen: conventional hydrogen bond ratio. It is well established in literature that HPN has a double sugar side chain with multiple hydroxyl groups, which are responsible for most of the hydrogen bonds (Binkowska, Citation2020; Kuntić et al., Citation2014). QRM and EGCG have 17 interactions each and carbon hydrogen: conventional hydrogen bond ratio of 3:6 and 1:7, respectively. K7G has the lowest overall number of hydrogen bonds. QRM and K7G are glycosides while EGCG is aglycone (Panche et al., Citation2016). However, the most reactive hydroxyl groups (7-OH in flavones or the 3-OH in flavonols) in flavonoids are glycosylated (Panche et al., Citation2016). Hence, this explains the lower overall number of interactions, number of carbon hydrogen bonds and the binding affinities observed ().
The amino acids residues Glu353, Arg394, and Hie524 form the binding mode of the endogenous ERα stimulator 17β-estradiol (E2) and are therefore considered as estrogenic residues as they are important in the recognition of estrogenic ligands (Brzozowski et al., Citation1997; Shtaiwi et al., Citation2019). However, the estrogenic residues in addition to Asp351 (Brzozowski et al., Citation1997; Celik et al., Citation2007; Shtaiwi et al., Citation2019) Thr347 and Leu346 form the binding modes of most SERMs (such as OHT and raloxifene) and they are therefore considered as antiestrogenic residues which are important in recognising antiestrogenic ligands (ERα inhibitors) (Li et al., Citation2013).
The alkyl side chain of OHT forms hydrogen bonds with Thr347, Asp351, Gly521, Hie524, Cys530 and Asn532. Notably, OHT formed strong C-H covalent bonds with the antiestrogenic residues Thr347 and Asp351.The hydroxyl groups on the rhamnoglucoside chain of HPN formed hydrogen bonds with Glu339, Leu346, Thr347, Trp383, and Cys530; and a covalent bond with Thr347 and Leu346. These results are consistent with previous docking studies that reported OHT and HPN binding to Asp351 and Thr347 (Puranik et al., Citation2019). The glucoside chain of QRM formed direct hydrogen bonds with Glu323, Glu353, Hie356, Arg394, Phe445 similar to Puranik’s (Citation2019) team who reported the binding of the aglycon version of QRM to Glu353 and Arg394. On the other hand, EGCG and K7G formed hydrogen bonds with Asp351, Met522, Asn532, Val534, Tyr526 and Leu327, Glu353, Ile386, Gly390, Lys444, respectively. EGCG also shared a conventional hydrogen bond with Asp351. Contrary to the findings of this study, Pang et al. (Citation2018) reported a lack of binding interactions between EGCG and Asp351
Interestingly, QRM and K7G formed hydrophobic interactions with the same amino acid residues. These interactions may be due to similarities arising in the unconjugated skeleton structures of both compounds. The only difference observed is the OH group on C-3 of the B- ring in QRM, which is replaced with H in K7G (Dabeek & Marra, Citation2019). However, the sugar sidechains of both compounds formed double conventional hydrogen bonds with Glu353, but none were observed to be formed with the antiestrogenic residues. These findings contradict those expressed by Puranik et al. (Citation2019) in which K7G and QRM interacted with Asp351 and Thr347, respectively (Puranik et al., Citation2019). This difference may be accredited to the use of aglycon versions of K7G and QRM in the present study. (Martínez, Citation2015)
Conclusions
Zearalenone is an estrogenic mycotoxin which is a common human and animal food contaminant. It has been implicated in carcinogenesis and other reproductive ailments through the estrogen receptor (ER) pathway. FDA approved synthetic selective estrogen receptor modulators (SERM) such as tamoxifen (OHT) which are used to treat these ailments induce life altering side effects albeit being effective. Hence the need for natural occurring ER inhibitors as medicinal alternatives such as flavonoids to circumvent side-effects. This study aimed to identify flavonoids ERα inhibitors.
Inhibitors of ERα extrapolated from a library of flavonoids were identified and the binding mechanism of the inhibition were investigated using molecular mechanics/GB surface area method (MM/GBSA) calculations and molecular dynamic simulations. The MM/GBSA binding free energy calculations revealed that HPN QRM, K7G and EGCG had a higher binding affinity for the receptor than reference drug, OHT. The ligand protein interactions revealed that HPN had the greatest total number of interactions and carbon-hydrogen bonds while QRM and K7G interacted with the same amino acid residues. Collectively the results revealed that the binding of the compounds to the receptor lead to dynamic alterations, which induced conformational shift in the structure and orientation of the receptor resulting in stabilised, compact and low energy systems.
Cumulatively, the results of this study support the use of flavonoids, especially HPN and QRM, as a naturally sourced alternative therapeutic agents against the pathological effects of zearalenone exposure in humans.
Abbreviations | ||
ΔGbind | = | Binding free energy |
BP | = | Binding pocket |
Cα | = | Alpha carbon |
EGCG | = | Epigallocatechin 3-gallate |
ERα | = | Estrogen receptor alpha |
HPN | = | Hesperidin |
K7G | = | Kaempferol 7-O-glucoside |
LBD | = | Ligand binding domain |
MD | = | Molecular dynamics |
MM/GBSA | = | Molecular Mechanics/Generalized Born Surface Area |
OHT | = | 4-Hydroxytamoxifen |
QRM | = | Quercetrin |
RMSD | = | Root mean square deviation |
RMSF | = | Root mean square fluctuation |
RoG | = | Radius of gyration |
SERMs | = | Selective estrogen receptor modulators |
ZEA | = | Zearalenone |
Supplemental Material
Download MS Word (19.9 KB)Acknowledgements
The authors wish to acknowledge the College of Health Science at the University of KwaZulu-Natal and the National Research Foundation (NRF) for the financial support and The Centre of High-Performance Computing (CHPC, https://www.chpc.ac.za), Cape Town, RSA, for computational resources.
Disclosure statement
The authors declare no potential conflicts of interest.
Funding
This work did not receive direct funding however the student and supervisor involved received some financial support from the parties mentioned.
References
- Ahmad, I. (2018). Tamoxifen a pioneering drug: An update on the therapeutic potential of tamoxifen derivatives. European Journal of Medicinal Chemistry, 143, 515–531. https://doi.org/10.1016/j.ejmech.2017.11.056
- Ahmed Adam, M. A., Tabana, Y. M., Musa, K. B., & Sandai, D. A. (2017). Effects of different mycotoxins on humans, cell genome and their involvement in cancer. Oncology Reports, 37(3), 1321–1336.
- Andersen, C. L., Zhao, F., & Ye, X.-Q. (2018). Effects of mycoestrogens on female reproduction. Reproductive and Developmental Medicine, 2(1), 52.
- Appiah-Kubi, P., & Soliman, M. (2017). Hybrid receptor-bound/MM-GBSA-per-residue energy-based pharmacophore modelling: Enhanced approach for identification of selective LTA4H inhibitors as potential anti-inflammatory drugs. Cell Biochemistry and Biophysics, 75(1), 35–48. https://doi.org/10.1007/s12013-016-0772-3
- Ashrafizadeh, M., Ahmadi, Z., Mohammadinejad, R., & Afshar, E. G. (2020). Tangeretin: A mechanistic review of its pharmacological and therapeutic effects. Journal of Basic and Clinical Physiology and Pharmacology, 31(4) https://doi.org/10.1515/jbcpp-2019-0191
- Baig, M. H., Sudhakar, D. R., Kalaiarasan, P., Subbarao, N., Wadhawa, G., Lohani, M., Khan, M. K. A., & Khan, A. U. (2014). Insight into the effect of inhibitor resistant S130G mutant on physico-chemical properties of SHV type beta-lactamase: A molecular dynamics study. PloS One, 9(12), e112456. https://doi.org/10.1371/journal.pone.0112456
- Behzad, S., Sureda, A., Barreca, D., Nabavi, S. F., Rastrelli, L., & Nabavi, S. M. (2017). Health effects of phloretin: From chemistry to medicine. Phytochemistry Reviews, 16(3), 527–533. https://doi.org/10.1007/s11101-017-9500-x
- Bennett, J. W., & Klich, M. (2003). Mycotoxins. Clinical Microbiology Reviews, 16(3), 497–516. https://doi.org/10.1128/cmr.16.3.497-516.2003
- Berinyuy, E., & Soliman, M. E. (2017). Identification of novel potential GP120 of HIV-1 antagonist using per-residue energy contribution-based pharmacophore modelling. Interdisciplinary Sciences: Computational Life Sciences, 9(3), 406–418.
- Binkowska, I. (2020). Hesperidin: Synthesis and characterization of bioflavonoid complex. SN Applied Sciences, 2(3), 1–9. https://doi.org/10.1007/s42452-020-2256-8
- Brzozowski, A. M., Pike, A. C., Dauter, Z., Hubbard, R. E., Bonn, T., Engström, O., Ohman, L., Greene, G. L., Gustafsson, J. A., & Carlquist, M. (1997). Molecular basis of agonism and antagonism in the oestrogen receptor. Nature, 389(6652), 753–758.
- Burley, S. K., Berman, H. M., Bhikadiya, C., Bi, C., Chen, L., Di Costanzo, L., Christie, C., Dalenberg, K., Duarte, J. M., Dutta, S., Feng, Z., Ghosh, S., Goodsell, D. S., Green, R. K., Guranovic, V., Guzenko, D., Hudson, B. P., Kalro, T., Liang, Y., … Zardecki, C. (2019). RCSB Protein Data Bank: Biological macromolecular structures enabling research and education in fundamental biology, biomedicine, biotechnology and energy. Nucleic Acids Research, 47(D1), D464–D474. https://doi.org/10.1093/nar/gky1004
- Cele, F. N., Ramesh, M., & Soliman, M. E. (2016). Per-residue energy decomposition pharmacophore model to enhance virtual screening in drug discovery: A study for identification of reverse transcriptase inhibitors as potential anti-HIV agents. Drug Design, Development and Therapy, 10, 1365–1377.
- Celik, L., Lund, J. D. D., & Schiøtt, B. (2007). Conformational Dynamics of the Estrogen Receptor alpha: Molecular dynamics simulations of the influence of binding site structure on protein dynamics. Biochemistry, 46(7), 1743–1758. https://doi.org/10.1021/bi061656t
- Cid-Ortega, S., & Monroy-Rivera, J. A. (2018). Extraction of kaempferol and its glycosides using supercritical fluids from plant sources: A review. Food Technology and Biotechnology, 56(4), 480–493.
- Claeys, L., Romano, C., De Ruyck, K., Wilson, H., Fervers, B., Korenjak, M., Zavadil, J., Gunter, M. J., De Saeger, S., De Boevre, M., & Huybrechts, I. (2020). Mycotoxin exposure and human cancer risk: A systematic review of epidemiological studies. Comprehensive Reviews in Food Science and Food Safety, 19(4), 1449–1464. https://doi.org/10.1111/1541-4337.12567
- Cvetanović, A., Švarc-Gajić, J., Gašić, U., Tešić, Ž., Zengin, G., Zeković, Z., & Đurović, S. (2017). Isolation of apigenin from subcritical water extracts: Optimization of the process. The Journal of Supercritical Fluids, 120, 32–42. https://doi.org/10.1016/j.supflu.2016.10.012
- Dabeek, W., & Marra, M. (2019). Dietary Quercetin and Kaempferol: Bioavailability and Potential Cardiovascular-Related Bioactivity in Humans. Nutrients, 11(10), 2288. https://doi.org/10.3390/nu11102288
- Das, P. S., Saha, P., & Abdul, A. (2017). A review on computer aided drug design in drug discovery. World J. Pharm. Pharm. Sci, 6(7), 279–291.
- de Oliveira, M. R., Nabavi, S. F., Habtemariam, S., Orhan, I. E., Daglia, M., & Nabavi, S. M. (2015). The effects of baicalein and baicalin on mitochondrial function and dynamics: A review. Pharmacological Research, 100, 296–308.
- Dinda, B., Dinda, S., DasSharma, S., Banik, R., Chakraborty, A., & Dinda, M. (2017). Therapeutic potentials of baicalin and its aglycone, baicalein against inflammatory disorders. European Journal of Medicinal Chemistry, 131, 68–80. https://doi.org/10.1016/j.ejmech.2017.03.004
- Dixit, S. B., Ponomarev, S. Y., & Beveridge, D. L. (2006). Root mean square deviation probability analysis of molecular dynamics trajectories on DNA. Journal of Chemical Information and Modeling, 46(3), 1084–1093.
- Ehrlich, V. A., Dellafiora, L., Mollergues, J., Dall’Asta, C., Serrant, P., Marin-Kuan, M., … Cozzini, P. (2015). Hazard assessment through hybrid in vitro/in silico approach: The case of zearalenone. ALTEX-Alternatives to Animal Experimentation, 32(4), 275–286.
- Fratev, F. (2015). Activation helix orientation of the estrogen receptor is mediated by receptor dimerization: Evidence from molecular dynamics simulations. Physical Chemistry Chemical Physics : PCCP, 17(20), 13403–13420. https://doi.org/10.1039/c5cp00327j
- Ganeshpurkar, A., & Saluja, A. (2019). The pharmacological potential of hesperidin. Indian Journal of Biochemistry and Biophysics (IJBB), 56(4), 287–300.
- Gapsys, V., Michielssens, S., Peters, J. H., de Groot, B. L., & Leonov, H. (2015). Calculation of binding free energies. In Molecular Modeling of Proteins (pp. 173–209): Springer.
- Gonnet, P. (2007). P-SHAKE: A quadratically convergent SHAKE in O (n2). Journal of Computational Physics, 220(2), 740–750. https://doi.org/10.1016/j.jcp.2006.05.032
- Grynkiewicz, G., & Demchuk, O. M. (2019). New perspectives for fisetin. Frontiers in Chemistry, 7, 697.
- Gupta, G., Siddiqui, M. A., Khan, M. M., Ajmal, M., Ahsan, R., Rahaman, M. A., Ahmad, M. A., Arshad, M., & Khushtar, M. (2020). Current pharmacological trends on myricetin. Drug Research, 70(10), 448–454. https://doi.org/10.1055/a-1224-3625
- Hollingsworth, S. A., & Dror, R. O. (2018). Molecular Dynamics Simulation for All. Neuron, 99(6), 1129–1143. https://doi.org/10.1016/j.neuron.2018.08.011
- Huynh, N. T., Smagghe, G., Gonzales, G. B., Van Camp, J., & Raes, K. (2018). Bioconversion of Kaempferol and Quercetin Glucosides from Plant Sources Using Rhizopus spp. Fermentation, 4(4), 102. https://doi.org/10.3390/fermentation4040102
- Idowu, K., Ramharack, P., Nlooto, M., & Gordon, M. (2020). Molecular Dynamic Mechanism(s) of Inhibition of Bioactive Antiviral Phytochemical Compounds Targeting Cytochrome P450 3A4 and P-Glycoprotein, https://doi.org/10.1080/07391102.2020.1821780
- Ignatov, A., & Ortmann, O. (2020). Endocrine Risk Factors of Endometrial Cancer: Polycystic Ovary Syndrome, Oral Contraceptives, Infertility, Tamoxifen. Cancers, 12(7), 1766. https://doi.org/10.3390/cancers12071766
- Indira, M., Krupanidhi, S., & Bobby, M. N. (2020). Docking study of selected citrus flavonoids towards exploring the potential anti estrogen ac. tivity.
- Islam, M. A., & Pillay, T. S. (2015). Exploration of the structural requirements of HIV-protease inhibitors using pharmacophore, virtual screening and molecular docking approaches for lead identification. Journal of Molecular Graphics & Modelling, 56, 20–30.
- Jeon, J., Kim, S. E., Lee, D.-Y., & Choi, D. (2020). Factors associated with endometrial pathology during tamoxifen therapy in women with breast cancer: A retrospective analysis of 821 biopsies. Breast Cancer Research and Treatment, 179(1), 125–130.
- Karim, N., Jia, Z., Zheng, X., Cui, S., & Chen, W. (2018). A recent review of citrus flavanone naringenin on metabolic diseases and its potential sources for high yield-production. Trends in Food Science & Technology, 79, 35–54. https://doi.org/10.1016/j.tifs.2018.06.012
- Khan, N., Syed, D. N., Ahmad, N., & Mukhtar, H. (2013). Fisetin: A dietary antioxidant for health promotion. Antioxidants & Redox Signaling, 19(2), 151–162.
- Kim, S., Chen, J., Cheng, T., Gindulyte, A., He, J., He, S., Li, Q., Shoemaker, B. A., Thiessen, P. A., Yu, B., Zaslavsky, L., Zhang, J., & Bolton, E. E. (2019). PubChem 2019 update: Improved access to chemical data. Nucleic Acids Research, 47(D1), D1102–d1109. https://doi.org/10.1093/nar/gky1033
- Kim, Y., Tian, Y., Yang, J., Huser, V., Jin, P., Lambert, C. G., Park, H., You, S. C., Park, R. W., Rijnbeek, P. R., Van Zandt, M., Reich, C., Vashisht, R., Wu, Y., Duke, J., Hripcsak, G., Madigan, D., Shah, N. H., Ryan, P. B., Schuemie, M. J., & Suchard, M. A. (2020). Comparative safety and effectiveness of alendronate versus raloxifene in women with osteoporosis. Scientific Reports, 10(1), 11115. https://doi.org/10.1038/s41598-020-68037-8
- Kore, P. P., Mutha, M. M., Antre, R. V., Oswal, R. J., & Kshirsagar, S. S. (2012). Computer-aided drug design: An innovative tool for modeling.
- Kowalska, K., Habrowska-Górczyńska, D. E., Urbanek, K. A., Domińska, K., & Piastowska-Ciesielska, A. W. (2018). Estrogen receptor α is crucial in zearalenone-induced invasion and migration of prostate cancer cells. Toxins, 10(3), 98. https://doi.org/10.3390/toxins10030098
- Kuiper-Goodman, T., Scott, P., & Watanabe, H. (1987). Risk assessment of the mycotoxin zearalenone. Regulatory Toxicology and Pharmacology: RTP, 7(3), 253–306.
- Kumar, R., Zakharov, M. N., Khan, S. H., Miki, R., Jang, H., Toraldo, G., Singh, R., Bhasin, S., & Jasuja, R. (2011). The dynamic structure of the estrogen receptor. Journal of Amino Acids, 2011, 812540. https://doi.org/10.4061/2011/812540
- Kuntić, V., Brborić, J., Holclajtner-Antunović, I., & Uskoković-Marković, S. (2014). Evaluating the bioactive effects of flavonoid hesperidin: A new literature data survey. Vojnosanitetski Pregled, 71(1), 60–65.
- Le Grand, S., Götz, A. W., & Walker, R. C. (2013). SPFP: Speed without compromise—A mixed precision model for GPU accelerated molecular dynamics simulations. Computer Physics Communications, 184(2), 374–380. https://doi.org/10.1016/j.cpc.2012.09.022
- Li, W.-M., Li, X.-B., Sun, S.-X., Liang, J., Wang, R.-L., & Wang, S.-Q. (2013). Agonist and antagonist recognition studies for oestrogen receptor by molecular dynamics simulation. Molecular Simulation, 39(3), 228–233. https://doi.org/10.1080/08927022.2012.717281
- Liu, X., Shi, D., Zhou, S., Liu, H., Liu, H., & Yao, X. (2018). Molecular dynamics simulations and novel drug discovery. Expert Opinion on Drug Discovery, 13(1), 23–37. https://doi.org/10.1080/17460441.2018.1403419
- Luo, Y., Yoshizawa, T., & Katayama, T. (1990). Comparative study on the natural occurrence of Fusarium mycotoxins (trichothecenes and zearalenone) in corn and wheat from high- and low-risk areas for human esophageal cancer in China. Applied and Environmental Microbiology, 56(12), 3723–3726. https://doi.org/10.1128/AEM.56.12.3723-3726.1990
- Mariadoss, A. V., Vinyagam, R., Rajamanickam, V., Sankaran, V., Venkatesan, S., & David, E. (2019). Pharmacological aspects and potential use of phloretin: A systemic review. Mini-Reviews in Medicinal Chemistry, 19(13), 1060–1067. https://doi.org/10.2174/1389557519666190311154425
- Martínez, L. (2015). Automatic identification of mobile and rigid substructures in molecular dynamics simulations and fractional structural fluctuation analysis. PloS One, 10(3), e0119264. https://doi.org/10.1371/journal.pone.0119264
- Maruthanila, V., Elancheran, R., Roy, N. K., Bhattacharya, A., Kunnumakkara, A. B., Kabilan, S., & Kotoky, J. (2019). In silico molecular modelling of selected natural ligands and their binding features with estrogen receptor alpha. Current Computer-Aided Drug Design, 15(1), 89–96.
- Mazurek, A. H., Szeleszczuk, Ł., Simonson, T., & Pisklak, D. M. (2020). Application of Various Molecular Modelling Methods in the Study of Estrogens and Xenoestrogens. International Journal of Molecular Sciences, 21(17), 6411. https://doi.org/10.3390/ijms21176411
- McGee, T. D., Edwards, J., & Roitberg, A. E. (2008). Preliminary molecular dynamic simulations of the estrogen receptor alpha ligand binding domain from antagonist to apo. Int J Environ Res Public Health, 5(2), 111–114. https://doi.org/10.3390/ijerph5020111
- N, N., Mhlongo, E. S., & Soliman, M. (2016). Binding free energy-based footprint pharmacophore model to enhance virtual screening and drug discovery: A case on glycosidases as anti-influenza drug targets. Letters in Drug Design & Discovery, 13(10), 1033–1046.
- Neven, P., Jongen, L., Lintermans, A., Van Asten, K., Blomme, C., Lambrechts, D., Poppe, A., Wildiers, H., Dieudonné, A.-S., Brouckaert, O., Decloedt, J., Berteloot, P., Verhoeven, D., Joerger, M., Vuylsteke, P., Wynendaele, W., Casteels, M., Van Huffel, S., Lybaert, W., … Guchelaar, H.-J. (2018). Tamoxifen metabolism and efficacy in breast cancer: A prospective multicenter trial. Clinical Cancer Research: An Official Journal of the American Association for Cancer Research, 24(10), 2312–2318. https://doi.org/10.1158/1078-0432.CCR-17-3028
- Ng, H. L. (2016). Simulations reveal increased fluctuations in estrogen receptor-alpha conformation upon antagonist binding. Journal of Molecular Graphics & Modelling, 69, 72–77. https://doi.org/10.1016/j.jmgm.2016.08.009
- Panche, A., Diwan, A., & Chandra, S. (2016). Flavonoids: An overview. Journal of Nutritional Science, 5 https://doi.org/10.1017/jns.2016.41
- Pang, X., Fu, W., Wang, J., Kang, D., Xu, L., Zhao, Y., Liu, A.-L., & Du, G.-H. (2018). Identification of estrogen receptor α antagonists from natural products via in vitro and in silico approaches. Oxidative Medicine and Cellular Longevity, 2018, 6040149. https://doi.org/10.1155/2018/6040149
- Pettersen, E. F., Goddard, T. D., Huang, C. C., Couch, G. S., Greenblatt, D. M., Meng, E. C., & Ferrin, T. E. (2004). UCSF Chimera—a visualization system for exploratory research and analysis. Journal of Computational Chemistry, 25(13), 1605–1612.
- Pike, A. C., Brzozowski, A. M., Hubbard, R. E., Bonn, T., Thorsell, A. G., Engström, O., Ljunggren, J., Gustafsson, J. A., & Carlquist, M. (1999). Structure of the ligand-binding domain of oestrogen receptor beta in the presence of a partial agonist and a full antagonist . The EMBO Journal, 18(17), 4608–4618. https://doi.org/10.1093/emboj/18.17.4608
- Powers, C. N., & Setzer, W. N. (2015). A molecular docking study of phytochemical estrogen mimics from dietary herbal supplements. In Silico Pharmacology, 3(1), 4. https://doi.org/10.1186/s40203-015-0008-z
- Puranik, N. V., Srivastava, P., Bhatt, G., John Mary, D. J. S., Limaye, A. M., & Sivaraman, J. (2019). Determination and analysis of agonist and antagonist potential of naturally occurring flavonoids for estrogen receptor (ERα) by various parameters and molecular modelling approach. Scientific Reports, 9(1), 7450. https://doi.org/10.1038/s41598-019-43768-5
- Qin, F., Zhou, Y., Huan, L., & Gui, W. (2020). Comparison of clomiphene and letrozole for superovulation in patients with unexplained infertility undergoing intrauterine insemination: A systematic review and meta-analysis. Medicine, 99(31), e21006. https://doi.org/10.1097/MD.0000000000021006
- Romero, P. R., Kobayashi, N., Wedell, J. R., Baskaran, K., Iwata, T., Yokochi, M., Maziuk, D., Yao, H., Fujiwara, T., Kurusu, G., Ulrich, E. L., Hoch, J. C., & Markley, J. L. (2020). BioMagResBank (BMRB) as a Resource for Structural Biology. Methods Mol Biol.), 2112, 187–218. https://doi.org/10.1007/978-1-0716-0270-6_14
- Sanchez-Spitman, A., Dezentjé, V., Swen, J., Moes, D. J. A. R., Böhringer, S., Batman, E., van Druten, E., Smorenburg, C., van Bochove, A., Zeillemaker, A., Jongen, L., Los, M., Neven, P., Gelderblom, H., & Guchelaar, H.-J. (2019). Tamoxifen pharmacogenetics and metabolism: Results from the prospective CYPTAM study. Journal of Clinical Oncology: Official Journal of the American Society of Clinical Oncology, 37(8), 636–646. +. https://doi.org/10.1200/JCO.18.00307
- Santos, E. L., Maia, B., Ferriani, A. P., & Teixeira, S. D. (2017). Flavonoids: Classification, biosynthesis and chemical ecology (Vol. 6). InTech.
- Schauperl, M., Czodrowski, P., Fuchs, J. E., Huber, R. G., Waldner, B. J., Podewitz, M., Kramer, C., & Liedl, K. R. (2017). Binding pose flip explained via enthalpic and entropic contributions. Journal of Chemical Information and Modeling, 57(2), 345–354. https://doi.org/10.1021/acs.jcim.6b00483
- Seifert, E. (2014). OriginPro 9.1: Scientific Data Analysis and Graphing Software — Software Review. ACS Publications.
- Selmi, S., Rtibi, K., Grami, D., Sebai, H., & Marzouki, L. (2017). Protective effects of orange (Citrus sinensis L.) peel aqueous extract and hesperidin on oxidative stress and peptic ulcer induced by alcohol in rat. Lipids in Health and Disease, 16(1), 1–12. https://doi.org/10.1186/s12944-017-0546-y
- Shtaiwi, A., Adnan, R., Khairuddean, M., & Khan, S. U. (2019). Computational investigations of the binding mechanism of novel benzophenone imine inhibitors for the treatment of breast cancer. RSC Advances, 9(61), 35401–35416. https://doi.org/10.1039/C9RA04759J
- Shunmugam, L., & Soliman, M. (2018). Targeting HCV polymerase: A structural and dynamic perspective into the mechanism of selective covalent inhibition. RSC Advances, 8(73), 42210–42222. https://doi.org/10.1039/C8RA07346E
- Somerset, S. M., & Johannot, L. (2008). Dietary flavonoid sources in Australian adults. Nutrition and Cancer, 60(4), 442–449. https://doi.org/10.1080/01635580802143836
- Sumardi, D., Pancoro, A., Yulia, E., Musfiroh, I., Prasetiyono, J., Karuniawan, A., & Syamsudin, T. (2017). Potential of local black soybean as a source of the isoflavones daidzein and genistein. International Food Research Journal, 24(5), 2140–2145.
- Ulusoy, H. G., & Sanlier, N. (2020). A minireview of quercetin: From its metabolism to possible mechanisms of its biological activities. Critical Reviews in Food Science and Nutrition, 60(19), 3290–3303.
- Vázquez Cisneros, L. C., López-Uriarte, P., López-Espinoza, A., Navarro Meza, M., Espinoza-Gallardo, A. C., & Guzmán Aburto, M. B. (2017). Effects of green tea and its epigallocatechin (EGCG) content on body weight and fat mass in humans: A systematic review. Nutricion Hospitalaria, 34(3), 731–737.
- Venkateswara Rao, P., Kiran, S., Rohini, P., & Bhagyasree, P. (2017). Flavonoid: A review on Naringenin. J. Pharmacogn. Phytochem, 6, 2778–2783.
- Wang, M., Firrman, J., Liu, L., & Yam, K. (2019). A review on flavonoid apigenin: Dietary intake, ADME, antimicrobial effects, and interactions with human gut microbiota. BioMed Research International, 2019, 7010467. https://doi.org/10.1155/2019/7010467
- Widowati, W., Jasaputra, D. K., Heriady, Y., Faried, A., Rizal, R., Widodo, W. S., Benowo Wibowo, S. H., W Kusuma, H. S., Girsang, E., & Ehrich Lister, I. N. (2019). Dietary flavonoids against various breast cancer subtypes: A molecular docking study. ScienceAsia, 45(5), 452. https://doi.org/10.2306/scienceasia1513-1874.2019.45.452
- Yaşar, P., Ayaz, G., User, S. D., Güpür, G., & Muyan, M. (2017). Molecular mechanism of estrogen-estrogen receptor signaling. Reproductive Medicine and Biology, 16(1), 4–20. https://doi.org/10.1002/rmb2.12006
- Ye, H., Dudley, S. Z., & Shaw, I. C. (2018). Intimate estrogen receptor-α/ligand relationships signal biological activity. Toxicology, 408, 80–87. https://doi.org/10.1016/j.tox.2018.07.003
- Yu, W., & MacKerell, A. D. (2017). Computer-aided drug design methods. In Antibiotics (pp. 85–106): Springer.
- Zhang, L., Li, Y., Lan, L., Liu, R., Wu, Y., Qu, Q., & Wen, K. (2016). Tamoxifen has a proliferative effect in endometrial carcinoma mediated via the GPER/EGFR/ERK/cyclin D1 pathway: A retrospective study and an in vitro study. Molecular and Cellular Endocrinology, 437, 51–61. https://doi.org/10.1016/j.mce.2016.08.011
- Zhang, J., Wu, W., Song, Y., Hou, L., Li, T., Guan, T., Zhang, T., & Wang, Y. (2019). Homogeneous assay for zearalenone analogues and their docking studies with apo-/holo-estrogen receptors. Analytical Methods, 11(2), 192–199. https://doi.org/10.1039/C8AY01940A