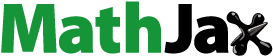
Abstract
Protein flexible loop regions were once thought to be simple linkers between other more functional secondary structural elements. However, as it becomes clearer that these loop domains are critical players in a plethora of biological processes, accurate conformational sampling of 3D loop structures is vital to the advancement of drug design techniques and the overall growth of knowledge surrounding molecular systems. While experimental techniques provide a wealth of structural information, the resolution of flexible loop domains is sometimes low or entirely absent due to their complex and dynamic nature. This highlights an opportunity for de novo structure prediction using in silico methods with molecular dynamics (MDs). This study evaluates some of the AMBER protein force field’s (ffs) ability to accurately model dihydrofolate reductase (DHFR) conformations, a protein complex characterized by specific arrangements and interactions of multiple flexible loops whose conformations are determined by the presence or absence of bound ligands and cofactors. Although the AMBER ffs, including ff19SB, studied well model most protein structures with rich secondary structure, results obtained here suggest the inability to significantly sample the expected DHFR loop–loop conformations – of the six distinct protein-ligand systems simulated, a majority lacked consistent stabilization of experimentally derived metrics definitive the three enzyme conformations. Although under-sampling and the chosen ff parameter combinations could be the cause, given past successes with these MD approaches for many protein systems, this suggests a potential misbalance in available ff parameters required to accurately predict the structure of multiple flexible loop regions present in proteins.
Communicated by Ramaswamy H. Sarma
Introduction
Flexibility and dynamics are integral to biological and enzymatic function, recognition, and protein-ligand interaction. To accurately model induced fit and/or conformational selection processes involved in ligand-receptor binding, there must be the inclusion of flexibility in both the ligand and the receptor (Koshland, Citation1995; Perola et al., Citation2004) – this is imperative in situations where multiple structures are populated depending on the environment (Steuber et al., Citation2006) and for docking apo (McGovern & Shoichet, Citation2003) (ligand-free structures which need to relax or respond to the docked ligand) or homology structures (Kairys et al., Citation2006). Dynamic processes in enzymes often utilize complex reorganizations of the protein through flexible loop domains, which themselves contribute to a plethora of biological processes (Lee et al., Citation2010; Espadaler et al., Citation2006; McElheny et al., Citation2005). As the known functions and involvement of these loop regions expand, the prediction of their 3D structure should be correct to produce accurate, biologically relevant data and reliable conclusions.
Challenges in predicting these flexible loop domains are seen in experimental structure determinations. Although NMR and protein crystallographic techniques provide an abundance of structural information, sometimes the structural data excludes or lacks resolution of these intricate loop regions as characterizing them is notoriously difficult due to their disordered nature (Fukuchi et al., Citation2006). On the computational side, homology modeling can address structural gaps in proteins and is utilized to predict 3D structures with a high degree of accuracy (Nikolaev et al., Citation2018; Martí-Renom et al., Citation2000). Unfortunately, determining the structure of flexible loop regions can be substantially less accurate using homology modeling due to the extensive variability in sequence and structure (Martí-Renom et al., Citation2000). All this provides motivation to pursue a comprehensive model using in silico techniques to expand understanding of dynamic molecular systems, specifically protein-ligand complexes, for the advancement of structure prediction and computer-aided drug design methodologies. However, prior to deeming de novo computational modeling as an effective tool to provide information of such elaborate movements, these modeling methodologies must be validated against existing experimental data.
The current generation of AMBER force fields (ffs) has shown the ability to fold and maintain the experimental structure of a wide variety of well-structured protein systems, including protein-ligand systems in varied molecular dynamics (MDs) simulation approaches, both in solution and in crystals (Tian et al., Citation2020; Wang et al., Citation2004; Pendley et al., Citation2009; Zouridakis et al., Citation2019; Shao & Zhu, Citation2018; Mittal et al., Citation2021; Rebollar-Ramos et al., Citation2021; Janowski et al., Citation2016). The AMBER ‘SB’ series of protein ffs – ff99SB, ff14SB and ff19SB – have become widely accepted amongst biomolecular simulation users. Broadly speaking, where these ffs differ from previous generations – ff94 and ff99 – is in the adjustment and corrections involving energy profiles for rotation around bonds (Maier et al., Citation2015). More specifically, the ff99SB protein ff refit protein backbone dihedrals, expanding on methods used in the ff94/99 parameters (Maier et al., Citation2015). Further improvements were made in ff14SB with the addition of new QM-based side-chain dihedral parameters and empirical adjustment to the backbone φ energy profile which improved secondary structure in small peptides (Tian et al., Citation2020; Maier et al., Citation2015). The most recent AMBER protein ff ff19SB, released in 2019, has significantly improved the backbone profiles for all 20 amino acids using a coupled scan for 2D parameters φ/ψ conformations of multiple amino acids using as reference the 2D quantum mechanics energy surface (Tian et al., Citation2020; Abriata & Dal Peraro, Citation2021). All the aforementioned protein ffs have shown to be successful in modeling the dynamics of protein systems with robust secondary structural elements (i.e. beta sheets and alpha helices), both in terms of interactions with ligands and other proteins, including in simulations with GAFF parameterization of the ligands and the OPC water model (Mustafa et al., Citation2020; Hornak et al., Citation2006; Schneider et al., Citation2020; Hoffmann et al., Citation2018; Zou et al., Citation2019). Where the ffs may break down is with intrinsically disordered proteins and in proteins having considerable non-classical secondary structural elements, such as dynamic loop–loop interactions (Mustafa et al., Citation2020; Feng et al., Citation2021; Barozet et al., Citation2021; Trbovic et al., Citation2008). A protein our lab has previously used to examine AMBER protein ffs’ ability to model dynamic loop regions is the enzyme dihydrofolate reductase (DHFR). DHFR is special in that it has three relatively large and interacting loops whose conformations and interactions depend on the presence or absence of bound ligand and/or co-factor.
DHFR − 5,6,7,8-tetrahydrofolate (THF):NADP+oxoreductase, E.C. 1.5.1.3 – is an experimentally well-studied model system for examining protein dynamics in enzyme catalysis as well as a model system for describing conformation selection in catalysis (McElheny et al., Citation2005; Venkitakrishnan et al., Citation2004; Sawaya & Kraut, Citation1997; Osborne et al., Citation2001; Boehr et al., Citation2006; Hughes et al., Citation2017; Kohen, Citation2015; Behiry et al., Citation2014). DHFR catalyzes the reduction of 7,8-dihydrofolate (DHF) to 5,6,7,8-THF via a hydride transfer using its cofactor reduced nicotinamide adenine dinucleotide phosphate (NADPH), a process essential for purine and thymidylate biosynthesis and subsequent cell growth and proliferation (Sawaya & Kraut, Citation1997; Osborne et al., Citation2001; Behiry et al., Citation2014; Boehr et al., Citation2010; Hawser et al., Citation2006). The enzyme has 159 residues and three well-characterized experimentally derived conformations – open (PDB: 1RD7; Sawaya & Kraut, Citation1997), closed (PDB: 1RF7; Sawaya & Kraut, Citation1997), and occluded (PDB: 1RX1; Sawaya & Kraut, Citation1997). These conformations are largely differentiated by the structure of three flexible loops: the active site M20 loop (residues 9–24), the neighboring FG loop (residues 116–132), and the GH (residues 142–149) loop domains () (McElheny et al., Citation2005; Venkitakrishnan et al., Citation2004; Sawaya & Kraut, Citation1997; Osborne et al., Citation2001; Behiry et al., Citation2014).
Figure 1. Molecular graphics depicting the flexible loop domains involved in the DHFR catalytic cycle modeled on the opened (PDB: 1RD7; Sawaya & Kraut, Citation1997), occluded (PDB: 1RF7; Sawaya & Kraut, Citation1997) and closed (PDB: 1RX1; Sawaya & Kraut, Citation1997) conformations. The opened conformation is complexed with the FOL ligand (Folic Acid), the occluded conformation with the THF (Tetrahydrofolate) ligand, and closed with the NADPH cofactor. The M20 loop (residues 9–24) is shown in violet, FG loop (residues 116 − 132) in green and GH loop (residues 142–149) in red.
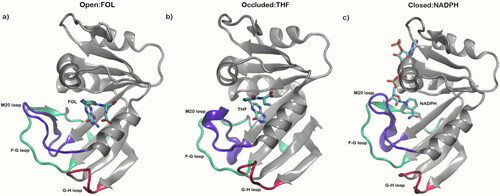
DHFR moves through five intermediates where the active site loop moves twice between closed and occluded conformations: first following a hydride transfer and again after product release as described in . Enzyme reorganization is proposed to occur through an open conformation intermediate () and NMR relaxation dispersion experiments suggest the interconversion between DHFR conformations occurs on the microsecond to millisecond timescale (McElheny et al., Citation2005; Sawaya & Kraut, Citation1997; Boehr et al., Citation2010). The M20 loop domain plays an important role in separating the closed conformation from the occluded (Venkitakrishnan et al., Citation2004). In the closed conformation, the M20 loop seals the active site through the positioning of the nicotinamide-ribose moiety of the bound cofactor (). This is stabilized via a hydrogen-bonding network between the M20 and FG loop domains in which the M20 loop adopts an orientation that sterically hinders the nicotinamide ring of the cofactor from interacting with the active site (McElheny et al., Citation2005; Sawaya & Kraut, Citation1997; Osborne et al., Citation2001; Boehr et al., Citation2010). Directly following hydride transfer and product formation, the enzyme is subjected to large-scale re-organization to the occluded conformation (). This involves breaking of the previously mentioned hydrogen bonding network to form a new hydrogen-bonding network between the M20 and GH loops. The catalytic cycle of this process is summarized in .
Figure 2. The various states observed in the DHFR catalytic cycle (McElheny et al., Citation2005; Cao et al., Citation2018). Closed conformational states are shown in pink, occluded in purple and DHFR is represented by ‘E’. Figure adapted from Kohen (Citation2015).
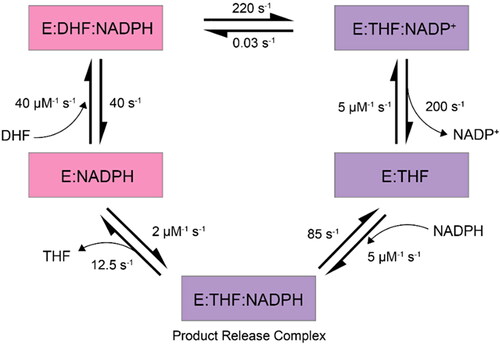
With an abundance of experimental structural data from NMR and protein x-ray crystallography, DHFR is a superb model system in evaluating and validating the AMBER ffs’ capability to model the enzyme structures accurately (Venkitakrishnan et al., Citation2004; Sawaya & Kraut, Citation1997; Osborne et al., Citation2001; Boehr et al., Citation2006; Kohen, Citation2015; Behiry et al., Citation2014; Boehr et al., Citation2010; Cao et al., Citation2018). Our initial investigations of DHFR began in 2005 with the evaluation of the ability of the AMBER ff99SB parameters to accurately resolve the complicated dynamics of the DHFR flexible loop domains on the 150–300 ns timescale (Hornak et al., Citation2006). The aim was to start both the correct structures and also decoy structures in the wrong or swapped state and hope, in the case of the decoys, that the MD simulations would converge to the correct loop conformations (open, occluded or closed) depending on the conditions (cofactor, ligand, both or none). The results of these simulations were unsuccessful. With the release of the AMBER ff14SB ff in 2015, we revisited the DHFR MD simulations with a series of ∼5 μs length simulations. Although the secondary structure largely remained intact, surprisingly similar conclusions to the earlier MD simulations were observed in the sense that none of the three flexible loop region states are sampled to the expected degree in simulations on the microsecond timescale (Figure S1).
In this work, we revisit the DHFR MD simulations using this latest ff (ff19SB) with more prolonged MD simulation times (three replicas, each ∼50 μs), and analyze the MD trajectories with a focus on the loop–loop interactions. In the DHFR simulations on a shorter timescale (∼50–100 ns), decent structural agreement and conformational shifts have been observed with various protein ffs including ff14SB (Mustafa et al., Citation2020), AMBER03 (Amusengeri et al., Citation2020) and CHARMM 36 (Huang et al., Citation2019). Mimicking this with the updated ff19SB protein ff, we noticed consistent agreement when only the first 10 ns of the simulation were analyzed using root-mean-square deviation (RMSD; Figure S2). However, there was already an increase in variation in the RMSD between identical replicas when looking at the first 100 ns (Figure S3). This highlights the importance of lengthened MD productions in order to obtain a full picture of protein loop–loop interactions, especially on a system where conformational shifts are expected to occur on the micro to millisecond timescale (McElheny et al., Citation2005; Sawaya & Kraut, Citation1997; Boehr et al., Citation2010). To thoroughly analyze the DHFR:ligand systems, we analyzed key interactions characteristic of experimental DHFR structures – including the formation of a characteristic 310 helix, close distance contacts, and hydrogen bonding interactions that all result from a psi angle torsion in the protein’s binding pocket. Overall, we observed that although the secondary structures are largely intact, the latest AMBER protein ff unfortunately does not maintain the expected loop–loop conformations as observed by NMR and x-ray crystallography.
Figure 3. RMSD analysis excluding DHFR loop regions residues: 9–24, 116–132 and 142–149. The analysis monitors structural variances as a function of time. Structures were analyzed against a reference structure which was the starting structure for that respective DHFR conformation. Each colored line represents a single replica for that system.
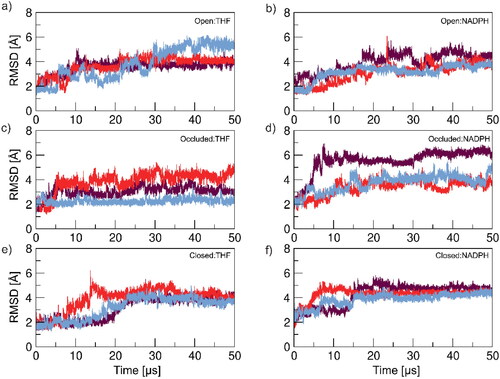
Methods
System building
Three distinct Escherichia coli DHFR structures were obtained from the RCSB Protein Databank (Berman et al., Citation2000) – open (PDB: 1RD7; Sawaya & Kraut, Citation1997), occluded (PDB: 1RF7; Sawaya & Kraut, Citation1997) and closed (PDB: 1RX1; Sawaya & Kraut, Citation1997). Model systems were built using the three conformations and complexing them individually with the THF ligand or NADPH cofactor, making for a total of six protein-ligand systems: Open:THF, Open:NADPH, Occluded:THF, Occluded:NADPH, Closed:THF and Closed:NADPH. THF coordinates were obtained from PDB 1RD7, and four hydrogen atoms were added using Gaussview version 5.0 (Mission, Kansas, United States of America) (Dennington et al., Citation2009) to the ligand in order to generate the proper THF product. The geometry of THF was optimized at an HF 6–31 G* theory level and partial charges were obtained based on the restrained electrostatic potential (RESP) methodology (Bayly et al., Citation1993; Cornell et al., Citation1993). To position the THF in the active site, the THF was aligned with the ligand from PDB 1RD7(DHF) coordinates. NADPH-containing systems were generated in the same manner, apart from using the PDB 1RX1(NADPH) coordinates for alignment and parameters for the NADPH cofactor were sourced from the Bryce AMBER parameter database (Bryce, Citation1998). The mol2 files containing parameters for both THF and NADPH and PDBs of the compounds complexed with DHFR can be found at https://amber.utah.edu/DHFR-ff19SB/.
Each model system was built using the AMBER ff19SB protein ff (Tian et al., Citation2020) to parameterize DHFR conformations and the AMBER General Force Field (GAFF) (Wang et al., Citation2004) for ligand parameterization. The systems were explicitly solvated in a truncated octahedron with 10 Å surrounding buffer of OPC water (Tian et al., Citation2020; Abriata & Dal Peraro, Citation2021; Izadi et al., Citation2014) using the Joung and Cheatham parameters for ions (Joung & Cheatham, Citation2008). Finally, net-neutralizing counterions were incorporated and excess NaCl was added to obtain a biologically representative ion concentration of ∼200 mM (Ross et al., Citation2018). To note, the conditions used in this evaluation of ff19SB using DHFR mimic the conditions our group has used in evaluations of previous AMBER protein ffs using DHFR.
Minimization and equilibration
The initial minimization consisted of 5000 steps with a 10 kcal/mol·Å2 harmonic positional restraint applied to the DHFR protein with no restraints to the respective ligand, water and other ions present in the complex. The first 1000 steps adhered to the steepest descent algorithm with strong restraints on heavy atoms and no SHAKE. The following minimization steps steadily released restraints on heavy atoms, still with no SHAKE, using the steepest descent algorithm. Equilibration occurred in eight steps, each 5000 ps, through which the restraints were reduced on backbone atoms and SHAKE (Götz et al., Citation2012) was introduced. The concluding equilibration phase was 1000 ps without restraints in an NTP ensemble. The Berendsen barostat was used for pressure scaling and Langevin dynamics were utilized for temperature control at 300 K with a 1.0 ps−1 collision frequency (Loncharich et al., Citation1992; Pastor et al., Citation1988) and relaxation time of 1.0 ps. Additionally, default parameters of the particle-mesh Ewald method (Essmann et al., Citation1995) were used to determine long-range electrostatics with a 9 Å cut-off (Salomon-Ferrer et al., Citation2013). It should be noted that the conditions of the DHFR:ligand systems – namely salt concentration (∼200 mM) and temperature (300 K) – aim to mimic both a biologically relevant environment and the environment of experimental studies of DHFR loop domains (McElheny et al., Citation2005; Venkitakrishnan et al., Citation2004; Ross et al., Citation2018; Tosso et al., Citation2013).
MD production
Following minimization and equilibration, all solute hydrogen masses were repartitioned from the bound heavy atom to 3.024 Da, in order to double the MD time integration step (to 4 fs) for production (Hopkins et al., Citation2015). All simulations were performed with the parallelized GPU (pmemd.CUDA) of the Amber20 modeling package (Le Grand et al., Citation2013). For all enzyme:ligand scenarios – Open:THF, Open:NADPH, Occluded:THF, Occluded:NADPH, Closed:THF and Closed:NADPH – three replicas were performed (each with a different initial ion distribution), and each replica run to obtain 50 µs simulation time. This totals 18 individual simulations and ∼900 µs of simulation time collectively. Simulations and analyses were performed at the Texas Advanced Computing Center and locally at University of Utah’s Center for High Performance Computing with the analyses utilizing AmberTools20 CPPTRAJ package (Roe & Cheatham, Citation2018). Prior to analyses, the systems were stripped of water present to create water-free trajectories in order to more clearly observe interactions and minimize computational demand. Additionally, all trajectory files for a given replica were compiled into a single file for ease of analysis and to reduce computational demand, every 10th frame (of the 900,000+ frames in the simulation) was collected. Analysis of the DHFR:ligand systems highlighted discrepancies between the computationally derived data collected here and established experimental metrics for defining DHFR conformational states. The data collected from the MD underwent a clustering analysis in order to more thoroughly analyze structures being sampled throughout the simulations. The method used followed the hierarchical (bottom-up) clustering algorithm which groups similar ‘objects’, in this case DHFR:ligand conformations, into clusters where each cluster is unique from each other but objects within a cluster are broadly similar. The conformational characteristics analyzed of each DHFR:ligand combination included evaluating system dynamics, the formation of an integral 310 helix, close distance contacts, and intramolecular hydrogen bonding interactions that all result from a psi angle torsion in the protein’s binding pocket (Venkitakrishnan et al., Citation2004; Sawaya & Kraut, Citation1997; Osborne et al., Citation2001; Behiry et al., Citation2014).
Results
Root-mean-square deviation
To get a broad look at system dynamics, a RMSD analysis was performed on all protein-ligand systems. An RMSD analysis provides the average deviation between the corresponding atoms of two structures – in this case between a reference structure (the experimental starting structure) and the coordinates of the simulated DHFR:ligand system over the duration of the MD production. A whole-protein (residues 1–159) RMSD was performed and results can be found in the Supporting Information (Figure S4). Observations from that analysis showed high fluctuation, and lack of convergence between replicas in many of the DHFR:ligand systems, furthermore, those systems that reach a level of convergence converge at a relatively higher RMSD value (∼5–6 Å) – this was attributed to the inclusion of the enzyme loop domains and their dynamic nature. As a result, an RMSD analysis was performed on all DHFR:ligand systems excluding the three-loop regions – the M20 (residues 9–24), FG (residues 116–132) and GH (residues 142–149) loops. Generally, replicas in the same protein-ligand scenario follow similar RMSD patterns throughout the respective simulation () with the RMSD value in converging systems being slightly lower (∼3–4 Å) but still higher than ideal (< 2 Å). The Occluded:NADPH and Closed:THF systems have some variance in RMSD behavior, however this is to be expected as these are the systems complexed with the ligand that promotes the opposite enzyme conformation (THF is native to the occluded and NADPH to the closed conformations).
Figure 4. RMSD analysis of the M20 loop domain (residues 9–24) of the various DHFR conformations in the presence of THF and NADPH. In each analysis, trajectories from the MD production are referenced against respective DHFR starting structures and each colored line describes an individual replica for that system.
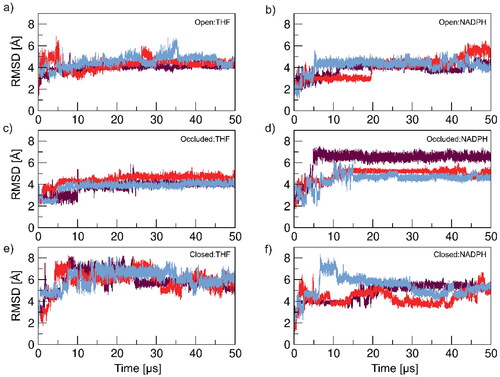
Though loop domains are less structured, more dynamic, and would be expected to greatly increase the RMSD, one particular DHFR loop holds particular significance in defining enzyme conformations. With the varied conformational states of the M20 loop being integral to DHFR function (McElheny et al., Citation2005; Venkitakrishnan et al., Citation2004; Sawaya & Kraut, Citation1997; Osborne et al., Citation2001), the RMSD analysis was honed in on just residues 9–24 to observe the system dynamics of this important domain ().
The pattern observed in previous RMSD analyses continues with the analysis focused on the M20 loop region – some systems converge but at relatively increased RMSD values. Higher RMSD values in general would be expected here due to the dynamic nature of the loop region. To get a full view of enzyme behavior and help further explore the RMSD results, we needed to look at other motions of the systems – e.g. internal motions.
Principle component analysis
A principle component analysis (PCA) can be utilized to evaluate dynamic motions of a given system; here, a combined PCA was used to analyze the internal motion of the DHFR systems. The PCA transforms a series of coordinated observations into a set of orthogonal vectors known as principal components (PCs) that partition the major modes of motion. These PCs help divulge variance in data and are used here to further investigate the lack of convergence observed in the RMSD analysis. Combined PCA refers to calculating the PCA across the aggregated three replicas, but with independent projection of PCs within each replica – this means that PC mode 1 corresponds between the three replicas which may not happen if independent PCA is performed on each replica. Figure S5 shows a combined PCA performed on the entirety of the DHFR enzyme, residues 1–159. Similar observations were made here as in the whole-protein RMSD analysis (Figure S4): inconsistency between replicas in sampling modes of motion. Following the same logic from the RMSD, the PCA was performed on the DHFR:ligand systems excluding residues involved in the flexible loop regions (residues 9–24 of the M20 loop, 116–132 of the FG loop, and 142–149 of the GH loop). describes the first PC, also known as the first mode of motion, of the DHFR systems and shows the largest variance in the data.
Figure 5. PCA depicting the first PC of motion of each simulation replica for all DHFR:ligand systems excluding M20, FG and GH loop domains. Each colored line denotes an individual replica for its respective simulation scenario.
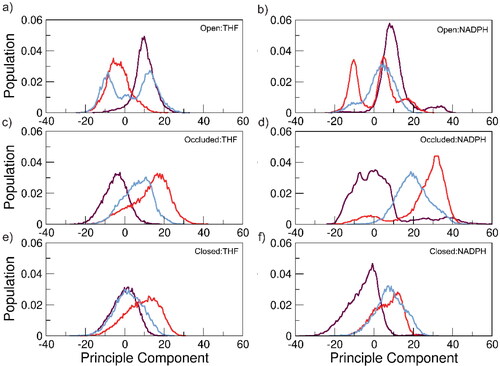
Between all the RMSD and PCA analyses, there is overall less convergence among simulation copies in all systems than we would like to see given the extended simulation time of 50 μs. Potential causes of this are insufficient sampling, as DHFR loop domain motions are proposed to occur on the microsecond to millisecond timescale, or, more likely, an inadequacy of AMBER ff19SB parameters to capture the elaborate arrangement of the loop regions during substrate insertion.
Secondary structure analyses were performed on the more defined structural regions of the DHFR enzyme to analyze the integrity of the structure throughout the simulation and ensure it is being conserved (). We observe preservation of the secondary structure in all analyzed portions of the protein in all of the DHFR:ligand combinations apart from residues 136–141. A plausible source of this is that these residues are located between the FG and GH loop regions and appear to have increased mobility due to the conformational shifts that occur in these domains. Nonetheless, knowing the DHFR secondary structure is well maintained but noting the variations observed in both the RMSD and PCA analyses, we suspect that each replica is likely sampling a distinct enzyme structure resulting in increased fluctuation between identical simulation copies. We aimed to dissect these varying enzyme structures in the following analyses and observe if any fulfilled experimentally defined metrics for the DHFR conformations.
Cluster analysis
A clustering analysis using the hierarchical (bottom-up) algorithm was performed to observe what structures are being sampled during the simulation. Being a highly mobile domain and a significant defining conformational characteristic, the clustering was performed on each replica of all systems highlighting the M20 loop. The number of clusters and their respective populations for all replicas of every DHFR:ligand combination modeled can be found in Table S1. Data shown here represent analyses performed on the top two clusters to study the most populated representative structures and how they satisfy experimental conformational metrics; comprehensive data for all clusters showing that none of the clusters fulfill these metrics can be found in the SI. An overlay of the most populated structures is shown in and already hints at the sampling of different structures between replicas.
Figure 6. Overlay of the representative structures from the most populated clusters (C0) of each DHFR:ligand scenario highlighting the M20 loop. The green, yellow, and orange coloring represents an individual replica for that respective system.
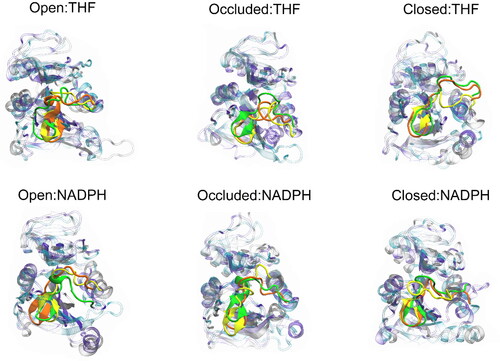
Table 1. Hydrogen bond occupancy of defining interactions reported in percentage of frames from the processed trajectories of the top two clusters (C0 and C1).
We see the lack of consistency between replicas persist both in cluster population distribution and in the configuration of the most populated structures, largely in the flexible loop regions. It is important to remember that the M20 loop plays a fundamental role in enzyme reorganization. Thus, the ff needs to be able the describe secondary structure and loop structures correctly. In the next section, we continue to analyze the M20 loop domain and validate against experimental data.
310 Helix formation
A defining characteristic of the occluded DHFR conformation (PDB: 1RF7; Sawaya & Kraut, Citation1997) is the formation of a 310 helix in the M20 loop domain, specifically in residues Glu17-Ala19 (Venkitakrishnan et al., Citation2004; Sawaya & Kraut, Citation1997; Behiry et al., Citation2014) (). When this helix is present, it prevents the cofactor from interacting with the active site through physical occlusion of the binding pocket (Sawaya & Kraut, Citation1997). Analysis of the secondary structure revealed DHFR conformations complexed with NADPH displayed minimal population of the 310 helix as expected from experiment. In the THF-complexed systems, the fraction of frames in which this helix was formed and reformed throughout the simulation was also considerably low () contrary to what would be expected given the prolonged ∼50 μs simulation time per replica.
Figure 7. a) Overlay of the three DHFR conformations, highlighting the 310 helix (purple) in the M20 loop residues Glu17-Ala19 that is characteristic of the occluded conformation. This region is represented for the open conformation (PDB: 1RD7) in blue, occluded (PDB: 1RF7) in purple and closed (PDB: 1RX1) in red; b) M20 loop 310 helix formation measured in central residues Glu17-Ala19 from the top two representative clusters. Each column describes a replica for that respective system and values are reported in percentage of frames. The 310 helix is a defining characteristic of the occluded DHFR conformation, so it would be expected that the percentage of frames that this moiety exists in would be significant in the THF-containing systems.
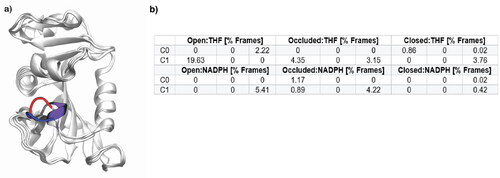
Comprehensive results from all clusters can be found in Table S2. With 310 helix formation being a staple of the occluded conformation and its presence being < 30% of simulation frames, indicates the occluded enzyme conformation is not substantially long-lived or stabilized in any of the THF-bound systems. Additionally, the discrepancy in population of this key secondary structure amongst replicas further indicates that the systems are sampling different conformations, pointing to a weakness in the ff to consistently predict these flexible loop regions. Alternative secondary structures that this region takes up are less ordered bends and turns (Figure S12).
Ψ Angle torsion
Closed and occluded DHFR conformations can be differentiated by a 180° change in psi (Ψ) torsion centering around residue Ile14 (Sawaya & Kraut, Citation1997). This structural change looks to be a result of Met16 in the M20 loop shifting to block the binding pocket in the occluded conformation (). The ΨIle14 torsion throughout the MD productions () displayed minimal change when the open and occluded conformations are complexed with either ligand, but the closed conformation does undergo a significant torsional change when complexed with THF compared to its reference value of −13.4°. Average ΨIle14 torsions were measured and reported in . Though the values measured are far from the reference ΨIle14 angles, the large error bars indicate the systems are changing in the presence of the two ligands but not concretely establishing the ΨIle14 appropriate for a defined DHFR conformation with respect to either ligand.
Figure 8. Histograms of ΨIle14 angle versus population for the top two clusters of all DHFR:ligand systems. Dashed lines designate reference ΨIle14 angle values measured from starting structures of respective reference systems – Occluded:THF (162.2°) and Closed:NADPH (−13.4°). Each colored line is an individual replica for that respective system.
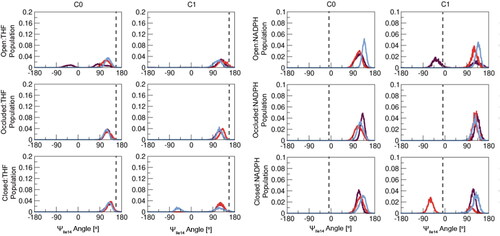
Figure 9. a) Overlay of the DHFR conformations highlighting Met16 blocking the binding pocket in the occluded conformation. b) Median ΨIle14 angle values from the most populated cluster for each complex with each bar representing a single replica for that system. Reference values were measure from starting reference structures – Occluded:THF and Closed:NADPH – and are described by the black dashed line; c) Overlay of the DHFR conformations highlighting Ile14 used to track the ΨIle14 angle to distinguish conformations. The residues from images a) and c) are represented for the open conformation (PDB: 1RD7) in blue, occluded (PDB: 1RF7) in purple and closed (PDB: 1RX1) in red.
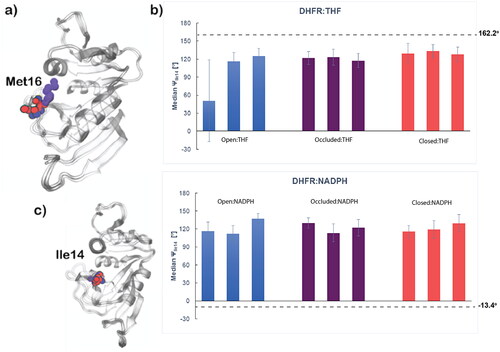
Generally, the torsion behavior of ΨIle14 is relatively consistent amongst the replicas for each of the DHFR:ligand systems and their clusters. THF-containing systems should have a ΨIle14 measurement of 162.2° and the systems with this ligand achieve ΨIle14 angles near this reference value. However, when complexed with NADPH, none of the systems shift to the −13.4° ΨIle14 angle characteristic of the reference closed enzyme conformation. Furthermore, the open DHFR systems only sampled ΨIle14 angle values less than zero briefly when complexed with THF. It is important to note that and only contain data from the top clusters of the enzyme-ligand systems and comprehensive results from all clusters for these analyses can be found in Figure S13 and Table S3, respectively. Observations made here are maintained in all clusters with a minimal change in ΨIle14 torsion in NADPH-complexed systems and THF-complexed systems being more successful in achieving the expected ΨIle14 angle.
Average values for the THF-bound systems achieve values generally closer to the reference ΨIle14 values (162.2°). This is rather significant in the Closed:THF system as the closed conformation begins at a −13.4°, so a considerable amount of the expected ∼180° ΨIle14 torsion angle change is taking place. Unfortunately, that is not the case in the NADPH-containing systems as all average ΨIle14 remain positive in value with the expected angle being −13.4°. The lack of ΨIle14 torsion in the DHFR:NADPH systems suggests that Met16 is not rotated out of the binding pocket and the pocket remains blocked, maintaining a characteristic of the occluded conformation rather than shifting to the closed conformation as expected in these systems. This is likely a result of an insufficiency in ff19SB to adequately model the dynamics of the M20 flexible loop domain and has the potential to impact other intramolecular protein interactions as we see in further analyses of these systems.
Hydrogen bond occupancy
The ΨIle14 torsion impacts hydrogen bonding interactions between loop regions in DHFR which help further distinguish between conformations. The closed DHFR conformation (PDB: 1RX1; Sawaya & Kraut, Citation1997) can be defined by hydrogen bonding interactions between the M20 loop and FG loop, the M20 loop and the αC helical region (residues 43–50), and an intra-loop hydrogen bond. When presented with DHF, a hydride transfer instigates product formation and the enzyme undergoes reorganization to the occluded conformation (). This involves breaking the hydrogen bonding network between the M20 and FG loops in favor of hydrogen bond formation between the M20 and GH loops as a result of the ΨIle14 torsion (McElheny et al., Citation2005; Venkitakrishnan et al., Citation2004; Osborne et al., Citation2001; Boehr et al., Citation2010). The average occupancy of defining hydrogen bonds can be found in . CPPTRAJ (Roe & Cheatham, Citation2018), the internal analysis program in AmberTools21, uses a distance cut-off and angle criteria to classify a hydrogen bond between selected atoms – that is the heavy atom hydrogen bond donor and the heavy atom hydrogen bond acceptor atom being 2.7–3.3 Å apart. Overall, there is a lack in consistent formation of hydrogen bonds that indicate stabilization of the various enzyme conformations. This is likely due to the change in ΨIle14 being less than the necessary 180° as seen in – to break and form hydrogen bonding networks necessary to stabilize alternate DHFR conformations.
There is a near complete absence in hydrogen bonding interactions that describe both the occluded and closed DHFR conformations in the top two clusters. In THF-containing systems, higher percentages of hydrogen bonding between the M20 loop and GH loop is expected. However, in the Open:THF and Closed:THF systems, in the interactions that do exist, there is increased interaction between the M20 and FG loops compared to M20 and GH domains, meaning that the former hydrogen bonding network was not sufficiently broken to instigate the latter. This pattern continues in the NADPH-containing simulations as the Open:NADPH and Occluded:NADPH systems contain a higher amount of hydrogen bonding interactions between the M20 and GH loop domain compared to M20 and FG loop domain, the opposite behavior to what is expected. There is a general increase in M20-αC, M20-M20 and M20-FG hydrogen bonding interactions when comparing the NADPH systems to the THF systems, which suggests minor movement toward alternate hydrogen-bonding networks. Complete data for all system clusters can be found in Tables S4 and S5. Additionally, the hydrogen bonds in the reference systems – Occluded:THF and Closed:NADPH – are generally absent, suggesting a lack in stabilization of those conformationally defining hydrogen bonding networks. Overall, the systems fail to establish substantial hydrogen bonding interactions that are definitive to either the occluded or closed DHFR conformations.
Distance analysis
Along with impacting hydrogen bond interactions (), the ΨIle14 torsion effects positioning of the M20 loop, specifically residue Met16 being in close contact with αC helical residues. The presence or absence of four close contacts helps to differentiate the occluded from the closed conformation. In the occluded conformation, M20 loop residue Met16 has close contact with the Thr46 and Ser49 residues in the αC helical region (). With the 180° ΨIle14 angle torsion change, the M20 loop residue Asn18 is repositioned to be in close contact with αC helical residues His45 and Ser49 in the closed conformation (Sawaya & Kraut, Citation1997) (). These four key distances were measured and the results can be found in . Generally, the open DHFR conformation did not appear to change drastically in terms of these defined distances, however, both the occluded and closed conformation shifted away from their reference distances when complexed with the opposing ligand.
Figure 10. Graphic highlighting the close contacts key to occluded and closed DHFR conformations: (A) Occluded DHFR conformation (PDB: 1RF7) shown in silver complexed with THF in pink. Key distances in this interaction are between the sulfur atom of Met16 and the alpha carbon of Thr46 (5.35 Å) and Met16 and the beta carbon of Ser49 (4.23 Å). (B) Closed DHFR conformation (PDB: 1RX1) represented in silver complexed with NADPH shown in purple. Close distances interactions occur between the gamma carbon of Asn18 and the beta carbons of His45 (4.07 Å) and Ser49 (3.90 Å).
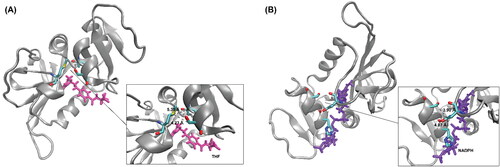
Figure 11. Each chart describes the average key distance measurements between the flexible M20 loop domain and the αC helical region. Reference systems’ – Occluded:THF and Closed:NADPH – close distance values are their respective colors, bolded and were measured from their respective PDBs. Data shown is representative of the most populated cluster (C0), and complete data for all clusters can be found in Tables S6 and S7 for DHFR:THF and DHFR:NADPH, respectively.
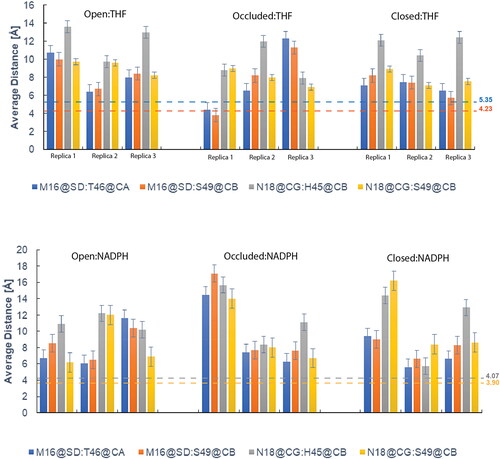
Comprehensive data from all clusters can be found in Tables S6 and S7 for the DHFR:THF and DHFR:NADPH systems respectively. Reference systems underwent MD simulation – Occluded:THF and Closed:NADPH – and it would be expected that these would maintain these critical distances at or close to that of the measured distance. Results indicate that neither the THF-bound nor NADPH-bound systems adapted conformations in which reference close contact distances were obtained. This could be a result of the ΨIle14 torsion not being enough to achieve these definitive contacts between the M20 loop and αC helical region, confirming previous observations from the analysis of that metric (). This continues to point out a weakness in AMBER ff19SB in accurately and consistently modeling flexible loop domains.
It should be noted that here we focus our analysis on representative structures obtained from the clustering analysis (). Traditionally, experimental studies would observe an average structure of a protein which, in an MD scenario, may look different than the representative structure. To comprehensively study the DHFR enzyme, we obtained an average structure for each cluster and measured the ΨIle14 angle to determine if these average structures better aligned with experimental data (Table S8). Our observations remained the same as with the representative structures: the average structures failed to meet experimental conformation criteria. Other conformational metrics studied in the representative enzyme structures – hydrogen bonding occupancy and formation of key contacts – are dependent on the torsion of the ΨIle14 angle. The lack of the necessary rotation indicates that these significant interactions also failed to be established.
Additionally, we explored the addition of restraints on the DHFR:ligand systems during MD productions with the intent of setting the systems up with desired conformational characteristics – THF-containing systems in occluded (PDB: 1RF7; Sawaya & Kraut, Citation1997) and NADPH-containing systems in the closed (PDB: 1RX1; Sawaya & Kraut, Citation1997) – and observing if the metrics were maintained. For THF-containing systems, distance and angle restraints were introduced. The distance restraint was intended to secure the 310 helix moiety in the M20 loop domain characteristic of the occluded DHFR conformation (). The values of the distance restraint were based on the close contact distances that were also used to define enzyme structures (). The angle restraint aimed to secure Ile14 in the appropriate Ψ angle (162.2°) for the occluded conformation (). NADPH-containing systems only had an angle restraint added to the systems with the objective of attaining the reference closed conformation ΨIle14 angle (−13.4°). The addition of these restraints did help improve convergence (Figures S14–S17), however, there was still a general failure in maintaining the restrained angles and distances as well as establishing other experimental conformational metrics (e.g. defined hydrogen bonding networks and formation of the 310 helix). Data from this set of simulations analyzing experimental metrics used throughout the manuscript to define DHFR conformations can be found in the SI (Tables S9–S14).
To see if the enzyme conformations were stabilized in the last 5 μs, we analyzed the last 10% of the 50 μs MD simulations. RMSD analyses were performed on the whole-protein (Figure S18), excluding the enzyme loop regions (Figure S19) and specifically on the M20 loop domain (Figure S20). This analysis highlighted improved sampling that occurs in prolonged MD simulations which can be observed with the increased convergence in the RMSD compared to the first 10 and 100 ns RMSDs ( and , respectively). Though the RMSD values are higher in the last 5 μs, we observe more stability and similarity in dynamics between replicas. Furthermore, a PCA analysis was performed to observe the internal motion of the systems during the last 5 μs (Figure S21), in which we did not observe significant variance or improvement from the initial PCA of the 50 μs simulations. Finally, we analyzed the ΨIle14 angle (Table S15) as it is the pivotal component in establishing other important DHFR conformational characteristics (hydrogen bonding networks, 310 helix formation and close contacts within the enzyme). As seen previously, all DHFR:ligand combinations fail to establish the appropriate ΨIle14 angle to establish, maintain and stabilize conformational structural characteristics.
Lastly, we wanted to evaluate our computational approach to ensure that was not the source of the issues in establishing DHFR conformations. In order to validate our methods, we simulated the Ac − GGG(KAAAA)3K − NH2 and Ac − GGG(KAAAA)3r − NH2 peptide systems, denoted by K19 and dR19, respectively, with AMBER ff19SB/OPC and ff14SB/TIP3P ff combinations to test if our results using our MD protocols match the observations from other groups. K19 and dR19 were chosen as they have been used in previous work to test the accuracy of both of the aforementioned AMBER protein ff/water parameter combinations through the comparison of the simulated helical content against experimental values (Tian et al., Citation2020; Maier et al., Citation2015; Song et al., Citation2008). Peptide structures were made utilizing the Chimera (Pettersen et al., Citation2004) software and modified using the tLEaP module of AMBER. For each of the peptide systems, three replicas were prepared using the ff14SB/TIP3P ff combination and three replicas were prepared using the ff19SB/OPC ff combination. The minimization and MD production processes for all replicas followed the same methods used for the DHFR systems with the simulations running to achieve ∼20 μs simulation time per replica. RMSD and fractional helicity analyses were performed using CPPTRAJ. The RMSD data (Figure S22) showed significant improvement in both lower RMSD values and increased convergence between replicas for both peptide systems between ff14SB/TIP3P and ff19SB/OPC – this greatly showcases the improvements made in the current ff19SB ff for modeling proteins. Additionally, the fractional helicity analysis (Figure S23) showed increased helicity in the ff19SB/OPC scenario compared to ff14SB/TIP3P in both dR19 and K19 systems, again exhibiting the improvements at work in the ff19SB/OPC ff combination in maintaining canonical protein structures. These results parallel previous studies on these systems, including those used to validate the ff14SB and ff19SB ffs (Tian et al., Citation2020; Maier et al., Citation2015; Song et al., Citation2008), which both emphasizes that ff19SB does well modeling robust canonical secondary structures and solidifies our approach to protein MD. These observations along with what we saw with the DHFR:ligand systems further suggest that there is room for improvement for AMBER protein ffs in modeling dynamic and complex protein loop domains.
Conclusion
The simulations presented aimed to evaluate AMBER ff19SB ff parameters in accurately and reproducibly modeling the complex and elaborate multiple loop domains of the enzyme DHFR when combined with the OPC water model and GAFF parameters in prolonged 50 µs MD productions. Results indicate a deficiency in adequate sampling of any of the three experimental conformations in any of the protein-ligand situations for DHFR. Generally, we observed less than ideal convergence given the 50 µs of simulation time, this may be addressed in future work using enhanced sampling methods, such as accelerated molecular dynamics (aMD) or using replica exchange molecular dynamics (REMD). The data consistently failed to fulfill experimentally-derived metrics and definitions of each distinct conformation. Additionally, reference systems – Occluded:THF and Closed:NADPH – did not substantially fulfill conformationally defining metrics that would be expected from experimental data in these mobile loop domains. The introduction of angle and distance restraints to the DHFR systems continued to fail in establishing conformationally definitive interactions. The observations in system dynamic motions, secondary structure aspects, and various loop − loop contacts suggest a misbalance in the ff that fails to accurately predict highly dynamic loop regions. Although ff19SB greatly improves on parameterizing the backbone for all amino acids and differentiating between amino-acid-dependent properties, such as helical propensities compared to previously published ff99SB, ff14SB and other non-Fourier ffs (Tian et al., Citation2020), further refinement of ff parameters may be necessary before ff19SB, and other parameters used here, can provide reliable de novo modeling of protein flexible loop domains.
Data and software availability
Trajectories and PDBs for all DHFR systems as well as ligand and cofactor mol2 files can be found at https://amber.utah.edu/DHFR-ff19SB/. Free software used can be accessed through the subsequent links: AmberTools21 (https://ambermd.org/AmberTools.php), Grace (https://plasma-gate.weizmann.ac.il/Grace/), VMD (https://www.ks.uiuc.edu/Development/Download/download.cgi?PackageName=VMD), UCSF Chimera (https://www.cgl.ucsf.edu/chimera/download.html).
Abbreviations | ||
AMBER | = | Assisted Model Building and Energy Refinement |
MD | = | Molecular Dynamics |
ff | = | Force Field |
DHFR | = | Dihydrofolate Reductase |
RESP | = | Restrained Electrostatic Potential |
QM | = | Quantum-mechanics |
DHF | = | Dihydrofolate |
T HF | = | Tetrahydrofolate |
NADP H | = | Nicotinamide Adenine Dinucleotide Phosphate |
RMSD | = | Root-Mean-Square Deviation |
PCA | = | Principle Component Analysis |
SI | = | Supplementary Information |
Supplemental Material
Download PDF (6.3 MB)Acknowledgments
We are thankful for considerable allocations of computer time on XSEDE MCA01S027, the Blue-waters Petascale Resource (NSF OCI-1036208, ACI-1440031, ACI-1443054 and ACI-1515572), Frontera and Longhorn at the Texas Advanced Computing Center (LRAC-MCB20008 and NSF OAC-1854828), and from the University of Utah Center for High Performance Computing. Funding from the NIH GM-081411 helped support this work and is gratefully acknowledged.
Disclosure statement
The authors report that there are no competing interests to declare.
Additional information
Funding
References
- Abriata, L. A., & Dal Peraro, M. (2021). Assessment of transferable forcefields for protein simulations attests improved description of disordered states and secondary structure propensities, and hints at multi-protein systems as the next challenge for optimization. Computational and Structural Biotechnology Journal, 19, 2626–2636. https://doi.org/10.1016/j.csbj.2021.04.050
- Amusengeri, A., Tata, R. B., & Tastan Bishop, Ö. (2020). Understanding the pyrimethamine drug resistance mechanism via combined molecular dynamics and dynamic residue network analysis. Molecules, 25(4), 904. https://doi.org/10.3390/molecules25040904
- Barozet, A., Chacón, P., & Cortés, J. (2021). Current approaches to flexible loop modeling. Current Research in Structural Biology, 3, 187–191. https://doi.org/10.1016/j.crstbi.2021.07.002
- Bayly, C. I., Cieplak, P., Cornell, W., & Kollman, P. A. (1993). A well-behaved electrostatic potential based method using charge restraints for deriving atomic charges: The RESP model. The Journal of Physical Chemistry, 97(40), 10269–10280. https://doi.org/10.1021/j100142a004
- Behiry, E. M., Evans, R. M., Guo, J., Loveridge, E. J., & Allemann, R. K. (2014). Loop interactions during catalysis by dihydrofolate reductase from Montella profunda. Biochemistry, 53(29), 4769–4774. https://doi.org/10.1021/bi500508z
- Berman, H. M., Westbrook, J., Feng, Z., Gilliland, G., Bhat, T. N., Weissig, H., Shindyalov, I. N., & Bourne, P. E. (2000). The protein data bank. Nucleic Acids Research, 28(1), 235–242. https://doi.org/10.1093/nar/28.1.235
- Boehr, D. D., McElheny, D., Dyson, H. J., & Wright, P. E. (2006). The dynamic energy landscape of dihydrofolate reductase catalysis. Science, 313(5793), 1638–1642. https://doi.org/10.1126/science.1130258
- Boehr, D. D., McElheny, D., Dyson, H. J., & Wright, P. E. (2010). Millisecond timescale fluctuations in dihydrofolate reductase are exquisitely sensitive to the bound ligands. Proceedings of the National Academy of Sciences of the United States of America, 107(4), 1373–1378. https://doi.org/10.1073/pnas.0914163107
- Bryce, R. (1998). AMBER parameter database. Bryce Group, Computational Biophysics and Drug Design. http://amber.manchester.ac.uk/
- Cao, H., Gao, M., Zhou, H., & Skolnick, J. (2018). The crystal structure of a tetrahydrofolate-bound dihydrofolate reductase reveals the origin of slow product release. Communications Biology, 1(1)10– 11. https://doi.org/10.1038/s42003-018-0236-y.
- Cornell, W. D., Cieplak, P., Bayly, C. I., & Kollman, P. A. (1993). Application of RESP charges to calculate conformational energies, hydrogen bond energies, and free energies of solvation. Journal of the American Chemical Society, 115(21), 9620–9631. https://doi.org/10.1021/ja00074a030
- Dennington, R., Keith, T., & Milliam, J. (2009). Gauss view, version 5. Semichem Inc.
- Espadaler, J., Querol, E., Aviles, F. X., & Oliva, B. (2006). Identification of function-associated loop motifs and application to protein function prediction. Bioinformatics (Oxford, England), 22(18), 2237–2243. https://doi.org/10.1093/bioinformatics/btl382
- Essmann, U., Perera, L., Berkowitz, M. L., Darden, T., Lee, H., & Pedersen, L. G. (1995). A smooth particle mesh Ewald method. Journal of Chemical Physics, 103(19), 8577–8593. https://doi.org/10.1063/1.470117
- Feng, J. J., Chen, J. N., Kang, W., & Wu, Y. D. (2021). Accurate structure prediction for protein loops based on molecular dynamics simulations with RSFF2C. Journal of Chemical Theory and Computation, 17(7), 4614–4628. https://doi.org/10.1021/acs.jctc.1c00341
- Fukuchi, S., Homma, K., Minezaki, Y., & Nishikawa, K. (2006). Intrinsically disordered loops inserted into the structural domains of human proteins. Journal of Molecular Biology, 355(4), 845–857. https://doi.org/10.1016/j.jmb.2005.10.037
- Götz, A. W., Williamson, M. J., Xu, D., Poole, D., Le Grand, S., & Walker, R. C. (2012). Routine microsecond molecular dynamics simulations with AMBER on GPUs. 1. Generalized born. Journal of Chemical Theory and Computation, 8(5), 1542–1555. https://doi.org/10.1021/ct200909j
- Hawser, S., Lociuro, S., & Islam, K. (2006). Dihydrofolate reductase inhibitors as antibacterial agents. Biochemical Pharmacology, 71(7), 941–948. https://doi.org/10.1016/j.bcp.2005.10.052
- Hoffmann, F., Mulder, F. A. A., & Schäfer, L. V. (2018). Accurate methyl group dynamics in protein simulations with AMBER force fields. The Journal of Physical Chemistry B, 122(19), 5038–5048. https://doi.org/10.1021/acs.jpcb.8b02769
- Hopkins, C. W., Le Grand, S., Walker, R. C., & Roitberg, A. E. (2015). Long-time-step molecular dynamics through hydrogen mass repartitioning. Journal of Chemical Theory and Computation, 11(4), 1864–1874. https://doi.org/10.1021/ct5010406
- Hornak, V., Abel, R., Okur, A., Strockbine, B., Roitberg, A., & Simmerling, C. (2006). Comparison of multiple AMBER force fields and development of improved protein backbone parameters. Proteins, 65(3), 712–725. https://doi.org/10.1002/prot.21123
- Huang, Q., Rodgers, J. M., Hemley, R. J., & Ichiye, T. (2019). Adaptations for pressure and temperature effects on loop motion in Escherichia Coli and Montella profunda dihydrofolate reductase. High Pressure Research, 39(2), 225–237. https://doi.org/10.1080/08957959.2019.1584799
- Hughes, R. L., Johnson, L. A., Behiry, E. M., Loveridge, E. J., & Allemann, R. K. (2017). A rapid analysis of variations in conformational behavior during dihydrofolate reductase catalysis. Biochemistry, 56(15), 2126–2133. https://doi.org/10.1021/acs.biochem.7b00045
- Izadi, S., Anandakrishnan, R., & Onufriev, A. V. (2014). Building water models: A different approach. The Journal of Physical Chemistry Letters, 5(21), 3863–3871. https://doi.org/10.1021/jz501780a
- Janowski, P. A., Liu, C., Deckman, J., & Case, D. A. (2016). Molecular dynamics simulation of triclinic lysozyme in a crystal lattice. Protein Science: A Publication of the Protein Society, 25(1), 87–102. https://doi.org/10.1002/pro.2713
- Joung, I. S., & Cheatham, T. E. (2008). Determination of alkali and halide monovalent ion parameters for use in explicitly solvated biomolecular simulations. The Journal of Physical Chemistry B, 112(30), 9020–9041. https://doi.org/10.1021/jp8001614
- Kairys, V., Fernandes, M. X., & Gilson, M. K. (2006). Screening drug-like compounds by docking to homology models: A systematic study. Journal of Chemical Information and Modeling, 46(1), 365–379. https://doi.org/10.1021/ci050238c
- Kohen, A. (2015). Dihydrofolate reductase as a model for studies of enzyme dynamics and catalysis. F1000Research, 4, 1464. https://doi.org/10.12688/f1000research.6968.1
- Koshland, D. E. (1995). The key–lock theory and the induced fit theory. Angewandte Chemie International Edition in English, 33(2324), 2375–2378. https://doi.org/10.1002/anie.199423751
- Le Grand, S., Götz, A. W., & Walker, R. C. (2013). SPFP: Speed without compromise—A mixed precision model for GPU accelerated molecular dynamics simulations. Computer Physics Communications, 184(2), 374–380. https://doi.org/10.1016/j.cpc.2012.09.022
- Lee, J., Lee, D., Park, H., Coutsias, E. A., & Seok, C. (2010). Protein loop modeling by using fragment assembly and analytical loop closure. Proteins, 78(16), 3428–3436. https://doi.org/10.1002/prot.22849
- Loncharich, R. J., Brooks, B. R., & Pastor, R. W. (1992). Langevin dynamics of peptides: The frictional dependence of isomerization rates of N-acetylalanyl-N’-methylamide. Biopolymers, 32(5), 523–535. https://doi.org/10.1002/bip.360320508
- Maier, J. A., Martinez, C., Kasavajhala, K., Wickstrom, L., Hauser, K. E., & Simmerling, C. (2015). Ff14SB: Improving the accuracy of protein side chain and backbone parameters from Ff99SB. Journal of Chemical Theory and Computation, 11(8), 3696–3713. https://doi.org/10.1021/acs.jctc.5b00255
- Martí-Renom, M. A., Stuart, A. C., Fiser, A., Sánchez, R., Melo, F., & Sali, A. (2000). Comparative protein structure modeling of genes and genomes. Annual Review of Biophysics and Biomolecular Structure, 29, 291–325. https://doi.org/10.1146/annurev.biophys.29.1.291
- McElheny, D., Schnell, J. R., Lansing, J. C., Dyson, H. J., & Wright, P. E. (2005). Defining the role of active-site loop fluctuations in dihydrofolate reductase catalysis. Proceedings of the National Academy of Sciences of the United States of America, 102(14), 5032–5037. https://doi.org/10.1073/pnas.0500699102
- McGovern, S. L., & Shoichet, B. K. (2003). Information decay in molecular docking screens against holo, apo, and modeled conformations of enzymes. Journal of Medicinal Chemistry, 46(14), 2895–2907. https://doi.org/10.1021/jm0300330
- Mittal, L., Srivastava, M., Kumari, A., Tonk, R. K., Awasthi, A., & Asthana, S. (2021). Interplay among structural stability, plasticity, and energetics determined by conformational attuning of flexible loops in PD-1. Journal of Chemical Information and Modeling, 61(1), 358–384. https://doi.org/10.1021/acs.jcim.0c01080
- Mustafa, G., Nandekar, P. P., Mukherjee, G., Bruce, N. J., & Wade, R. C. (2020). The effect of force-field parameters on cytochrome P450-membrane interactions: Structure and dynamics. Scientific Reports, 10(1), 7284. https://doi.org/10.1038/s41598-020-64129-7.
- Nikolaev, D. M., Shtyrov, A. A., Panov, M. S., Jamal, A., Chakchir, O. B., Kochemirovsky, V. A., Olivucci, M., & Ryazantsev, M. N. (2018). A comparative study of modern homology modeling algorithms for rhodopsin structure prediction. ACS Omega, 3(7), 7555–7566. https://doi.org/10.1021/acsomega.8b00721
- Osborne, M. J., Schnell, J., Benkovic, S. J., Dyson, H. J., & Wright, P. E. (2001). Backbone dynamics in dihydrofolate reductase complexes: Role of loop flexibility in the catalytic mechanism. Biochemistry, 40(33), 9846–9859. https://doi.org/10.1021/bi010621k
- Pastor, R. W., Brooks, B. R., & Szabo, A. (1988). An analysis of the accuracy of Langevin and molecular dynamics algorithms. Molecular Physics, 65(6), 1409–1419. https://doi.org/10.1080/00268978800101881
- Pendley, S. S., Yu, Y. B., & Cheatham, T. E. (2009). Molecular dynamics guided study of salt bridge length dependence in both fluorinated and non-fluorinated parallel dimeric coiled-coils. Proteins, 74(3), 612–629. https://doi.org/10.1002/prot.22177
- Perola, E., Walters, W. P., & Charifson, P. S. (2004). A detailed comparison of current docking and scoring methods on systems of pharmaceutical relevance. Proteins, 56(2), 235–249. https://doi.org/10.1002/prot.20088
- Pettersen, E. F., Goddard, T. D., Huang, C. C., Couch, G. S., Greenblatt, D. M., Meng, E. C., & Ferrin, T. E. (2004). UCSF chimera–a visualization system for exploratory research and analysis. Journal of Computational Chemistry, 25(13), 1605–1612. https://doi.org/10.1002/jcc.20084
- Rebollar-Ramos, D., Ovalle-Magallanes, B., Palacios-Espinosa, J. F., Macías-Rubalcava, M. L., Raja, H. A., González-Andrade, M., & Mata, R. (2021). α-Glucosidase and PTP-1B inhibitors from malbranchea dendritica. ACS Omega, 6(35), 22969–22981. https://doi.org/10.1021/acsomega.1c03708
- Roe, D. R., & Cheatham, T. E. (2018). Parallelization of CPPTRAJ enables large scale analysis of molecular dynamics trajectory data. Journal of Computational Chemistry, 39(25), 2110–2117. https://doi.org/10.1002/jcc.25382
- Ross, G. A., Rustenburg, A. S., Grinaway, P. B., Fass, J., & Chodera, J. D. (2018). Biomolecular simulations under realistic macroscopic salt conditions. The Journal of Physical Chemistry B, 122(21), 5466–5486. https://doi.org/10.1021/acs.jpcb.7b11734
- Salomon-Ferrer, R., Götz, A. W., Poole, D., Le Grand, S., & Walker, R. C. (2013). Routine microsecond molecular dynamics simulations with AMBER on GPUs. 2. Explicit solvent particle mesh Ewald. Journal of Chemical Theory and Computation, 9(9), 3878–3888. https://doi.org/10.1021/ct400314y
- Sawaya, M. R., & Kraut, J. (1997). Loop and subdomain movements in the mechanism of Escherichia Coli dihydrofolate reductase: Crystallographic evidence. Biochemistry, 36(3), 586–603. https://doi.org/10.1021/bi962337c
- Schneider, J., Korshunova, K., Si Chaib, Z., Giorgetti, A., Alfonso-Prieto, M., & Carloni, P. (2020). Ligand pose predictions for human G protein-coupled receptors: Insights from the amber-based hybrid molecular mechanics/coarse-grained approach. Journal of Chemical Information and Modeling, 60(10), 5103–5116. https://doi.org/10.1021/acs.jcim.0c00661
- Shao, Q., & Zhu, W. (2018). Assessing AMBER force fields for protein folding in an implicit solvent. Physical Chemistry Chemical Physics, 20(10), 7206–7216. https://doi.org/10.1039/c7cp08010g
- Song, K., Stewart, J. M., Fesinmeyer, R. M., Andersen, N. H., & Simmerling, C. (2008). Structural insights for designed alanine-rich helices: Comparing NMR helicity measures and conformational ensembles from molecular dynamics simulation. Biopolymers, 89(9), 747–760. https://doi.org/10.1002/bip.21004
- Steuber, H., Zentgraf, M., Gerlach, C., Sotriffer, C. A., Heine, A., & Klebe, G. (2006). Expect the unexpected or caveat for drug designers: Multiple structure determinations using aldose reductase crystals treated under varying soaking and co-crystallisation conditions. Journal of Molecular Biology, 363(1), 174–187. https://doi.org/10.1016/j.jmb.2006.08.011
- Tian, C., Kasavajhala, K., Belfon, K. A. A., Raguette, L., Huang, H., Migues, A. N., Bickel, J., Wang, Y., Pincay, J., Wu, Q., & Simmerling, C. (2020). Ff19SB: Amino-acid-specific protein backbone parameters trained against quantum mechanics energy surfaces in solution. Journal of Chemical Theory and Computation, 16(1), 528–552. https://doi.org/10.1021/acs.jctc.9b00591
- Tosso, R. D., Andujar, S. A., Gutierrez, L., Angelina, E., Rodríguez, R., Nogueras, M., Baldoni, H., Suvire, F. D., Cobo, J., & Enriz, R. D. (2013). Molecular modeling study of dihydrofolate reductase inhibitors. Molecular dynamics simulations, quantum mechanical calculations, and experimental corroboration. Journal of Chemical Information and Modeling, 53(8), 2018–2032. https://doi.org/10.1021/ci400178h
- Trbovic, N., Kim, B., Friesner, R. A., & Palmer, A. G. (2008). Structural analysis of protein dynamics by MD simulations and NMR spin-relaxation. Proteins, 71(2), 684–694. https://doi.org/10.1002/prot.21750
- Venkitakrishnan, R. P., Zaborowski, E., McElheny, D., Benkovic, S. J., Dyson, H. J., & Wright, P. E. (2004). Conformational changes in the active site loops of dihydrofolate reductase during the catalytic cycle. Biochemistry, 43(51), 16046–16055. https://doi.org/10.1021/bi048119y
- Wang, J., Wolf, R. M., Caldwell, J. W., Kollman, P. A., & Case, D. A. (2004). Development and testing of a general amber force field. Journal of Computational Chemistry, 25(9), 1157–1174. https://doi.org/10.1002/jcc.20035
- Zou, J., Tian, C., & Simmerling, C. (2019). Blinded prediction of protein–ligand binding affinity using amber thermodynamic integration for the 2018 D3R grand challenge 4. Journal of Computer-Aided Molecular Design, 33(12), 1021–1029. https://doi.org/10.1007/s10822-019-00223-x
- Zouridakis, M., Papakyriakou, A., Ivanov, I. A., Kasheverov, I. E., Tsetlin, V., Tzartos, S., & Giastas, P. (2019). Crystal structure of the monomeric extracellular domain of Α9 nicotinic receptor subunit in complex with α-conotoxin RgIA: Molecular dynamics insights into RgIA binding to Α9α10 nicotinic receptors. Frontiers in Pharmacology, 10, 474. https://doi.org/10.3389/fphar.2019.00474