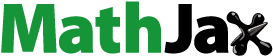
Abstract
Noncustodial sanctions may present an attractive way to reduce the prison population rate, but only when noncustodial sanctions meet custodial ones in terms of deterring recidivism. Using administrative criminal records data of all individuals convicted in the Netherlands in 2012, this study examines the effects of short-term imprisonment versus noncustodial sanctions on crime. We employ an instrumental variables approach to account for selection processes and to produce consistent estimates of the effects of imprisonment. Findings indicate that being sentenced to prison rather than a noncustodial sanction increases the prevalence of recidivism by 10 percentage points and increases recidivism rates by 1.07 registered crimes during a follow-up period of three years. Treatment effect heterogeneity analyses show that the detrimental impact of imprisonment is most pronounced for first-time prisoners, and adult offenders, compared to repeat prisoners and young adult offenders.
Introduction
Reducing the prison population is one of the biggest challenges faced in the criminal justice system across countries worldwide. There are many good reasons to exercise restraint when it comes to imprisonment. For one, imprisoning people is an expensive enterprise, and the costs of imprisonment typically weigh heavy on the criminal justice budget (e.g. Phelps & Pager, Citation2016). To the extent that imprisonment maintains or even increases marginalization of the imprisoned population following their release, these direct costs may be dwarfed by imprisonment’s indirect societal costs (Kirk & Wakefield, Citation2018; Kopf & Mowen, Citation2020). Given that there are serious doubts about both the crime preventive and rehabilitative effects of imprisonment, humanitarian considerations also suggest that imprisonment is best used only as a last resort.
Strategies to effectively reduce the prison population depend on the sentencing practices and inmate profile of the particular country. A country’s prison population is affected by the number of individuals sentenced to prison, as well as the average length of these prison sentences. Countries in which a large part of the prison population is serving long sentences would benefit most from initiating or expanding early release programs. For countries in which most prisoners serve short sentences, seeking noncustodial alternatives to imprisonment would be a more effective approach (Dünkel, Citation2017). In fact, such noncustodial alternatives were first developed in Western countries specifically on the assumption that even short prison spells may be “damaging” and therefore should be avoided wherever possible (Killias et al., Citation2000).
Whether noncustodial alternatives to short-term imprisonment are a viable option, depends in large part on the extent to which they are as successful as short-term prison sentences in meeting the goals of punishment. Given their limited duration, both the incapacitation and rehabilitation effects of short-term prison sentences appear ancillary to their potential deterrent and retributive effects (Killias et al., Citation2000; Wermink et al., Citation2013). A key question to be answered when contemplating the increased deployment of noncustodial sentences therefore relates to the extent these sentences match their custodial counterparts in terms of deterring future crimes.
The present study will examine specific deterrence, as measured by levels of repeat offending following either a short-term prison sentence (i.e. with a maximum of 6 months) or a noncustodial equivalent. While abundant research has compared repeat offending rates after custodial and noncustodial sentences, few of these studies meet the mark of scientific rigor that would justify drawing substantive conclusions regarding possibly differential deterrent effects of existing alternatives to imprisonment. The present study uses longitudinal administrative conviction data on all individuals convicted by single sitting judges in the Netherlands in 2012 to estimate the effects of noncustodial versus custodial sanctions on recidivism. It takes advantage of the random assignment of cases to judges, and exploits judges’ different sentencing tendencies as a source of exogenous variation in receipt of a custodial versus noncustodial sanction. Random assignment ensures that defendants’ characteristics, criminal histories, and offenses are evenly distributed (in expectation) across judges, which means that any differences across defendants in the types of sanction they receive are strictly a function of the judges who sentence them, rather than the particular characteristics of their cases. Known as an instrumental variables design, it recovers the causal effect of a sentence to a custodial sanction following conviction among otherwise identical individuals who could have instead been sentenced to a noncustodial sanction, but for their random assignment to a “punitive” rather than “lenient” judge to handle their case.
Theories of Imprisonment Effects
While reducing the prison population is a goal worthy of emulation, this must not be at the expense of rising crime levels. Increased reoffending rates among those sentenced to noncustodial alternatives to short-term imprisonment would make a reductionist policy less desirable, not to mention less politically palatable.
Theoretically, custodial sentences are typically perceived as one of the “harshest” punishments available in the sentencing arsenal of the modern state (Bagaric, Citation2020). It follows that in terms of specific deterrence much is expected from custodial sentences, though the typically high observed recidivism rates following imprisonment readily caution against unrealistic expectations in this respect (Nagin et al., Citation2009). To the extent that prison time is used for educational, vocational or treatment purposes, a rehabilitative effect of custodial sentences might add to their crime preventive effect. Custodial sentences however, may also have several collateral effects that may offset some, if not all, of its beneficial effects. First and foremost, prisons are offender convergence settings par excellence, providing a moral climate conducive to crime, as well as access to the skills, accomplishments and opportunities needed to actually engage in future criminal behavior (Damm & Gorinas, Citation2020). In addition, spending time in custody may interfere with the individual’s conventional social bonds in ways that enhance the likelihood of reoffending upon release (Kirk & Wakefield, Citation2018; Kopf & Mowen, Citation2020). Having to spend time in prison may lead individuals to lose their home, source of income and social support network. As such, custodial sentences may increase the financial motivation to engage in crime, while at the same time reduce the social barriers to do so. Finally, the stigma associated with being an ex-prisoner may detrimentally impact the individual’s conventional opportunities (Pager et al., Citation2009), rendering a life of crime an increasingly attractive and viable option.
The above arguments require nuance when it comes to short-term imprisonment. For one, some noncustodial sentences can be rather punitive in nature, and those undergoing noncustodial sentences report experiencing them as equally harsh or even harsher than imprisonment (e.g. Petersilia & Deschenes, Citation1994). The deterrent effects of both sentence types thus may differ less than commonly assumed. In addition, those serving short-term prison sentences are typically exempt from educational or vocational programs that apply to those serving longer prison sentences (Pompoco et al., Citation2017; RSJ, Citation2021). To the extent that noncustodial sentences pertain to community service, the rehabilitative effect of noncustodial sentences—if anything—likely trumps that of short-term imprisonment. In terms of collateral effects, noncustodial sentences may suffer less from the unintended detrimental effects than custodial sentences. Noncustodial sentences avoid the criminal contagion effects that plague imprisonment. Noncustodial sentences also do not interfere with existing conventional bonds, as these sentences can be accomplished alongside working a regular job and meeting other conventional social obligations. More so than imprisonment, noncustodial sentences can be kept from the public eye (e.g. fines, suspended sentences), but even when involving a public component (e.g. community service), the social mark of imprisonment might be worse than that of noncustodial sentences. To the extent that continued social bonds and conventional opportunities stop the individual from reoffending, noncustodial sentences might outperform custodial sentences in this regard.
As the net effect of both custodial and noncustodial sentences represents an amalgam of both intended and unintended, and rehabilitative and criminogenic consequences of the particular sanction, it is difficult to predict which of the two is to be preferred in terms of preventing repeat offending. A substantive number of studies have therefore aimed to empirically address this question.
Prior Empirical Research
A substantial amount of previous research has empirically compared reoffending following custodial versus noncustodial sentences (Jonson, Citation2010; Smith et al., Citation2002; Villettaz et al., Citation2006, Citation2015). In fact, a recent systematic review on the topic included a total of 116 studies, from 16 countries (Petrich et al., Citation2021). Still, only a minority of these studies adopted an experimental or quasi-experimental design to control for potential selection effects. The vast remainder of studies used regression-based designs or by-variable matching to control for known confounders that might influence both the probability of receiving a particular sentence type as well as the likelihood of reoffending. Typically, these latter studies were only able to control for the most obvious demographic characteristics such as age and sex, and basic features of the individual’s criminal history. Quasi-experimental studies using propensity score matching summarize individual profiles on many variables into a single summary statistic (i.e. the propensity score). Consequently, these studies are able to include many more individual and background characteristics. Yet, like regression and by-variable matching, propensity scores are based on the limited range of observed features typically available in large-scale datasets. As potentially many more characteristics may influence the sentencing decision as well as the individual’s subsequent criminal career development, these studies still potentially suffer from omitted variables bias.
Though smaller in magnitude than the effect found in less methodologically rigorous studies, pooled evidence from experimental and quasi-experimental studies still suggests that custodial sanctions perform significantly worse than noncustodial sanctions when it comes to preventing repeat offending. Looking only at studies employing experimental designs, Villettaz et al. (Citation2015) find no statistically significant differences in reoffending between custodial and noncustodial sentences, which they interpret as the differences found in matching studies most likely still reflecting the assignment of individuals with different personal and criminal profiles to different sanction modalities. Relevant to efforts aimed at reducing the prison population, the overarching conclusion to be drawn from previous empirical work is that noncustodial sentences seem to result in reoffending rates that are equal to, or even slightly lower than, custodial sentences.
Recently there is a small but growing number of studies that addresses selection bias resulting from unobserved confounders by using random assignment of cases to judges that differ in their levels of leniency, which provides a source of exogenous variation in the type of sentence imposed following conviction. The review by Loeffler and Nagin (Citation2022) includes 13 studies using such instrumental variable (IV) designs, while new IV-studies continue to be published (Eren & Mocran, Citation2021; Rahman & Weatherburn, 2021), suggesting it has growing utility as a solution to the selection bias problem.Footnote1 The instrumental variable design used in this way resembles use of an assignment lottery to determine a person’s eligibility for some form of treatment. For example, it has been used to estimate the effect of Vietnam-era military service on long-term earnings (Angrist, Citation1990), the impact of income on political attitudes (Doherty et al., Citation2006), and the effect of prison sentence length on earnings (Kling, Citation2006), among many others.
Whereas matching relies on observed variables that are assumed to affect both the likelihood of receiving a custodial sanction and the likelihood of recidivism upon release, the influence of an instrumental variable on recidivism is assumed to be fully mediated via its effect on selection into a particular sanction type. Propensity score matching will control for hidden selection bias only to the extent that unmeasured confounders are correlated with observed variables included in calculating the propensity score. In contrast, instrumental variable (IV) analysis compares groups of offenders that differ in their likelihood of receiving a custodial or noncustodial sanction only because their cases are dealt with by a different judge. Estimates of the effect of custodial versus noncustodial sanctions are based on those offenders who would have received a custodial sentence in the event their case had been dealt with by a more punitive judge.
provides a list of all studies known to the authors at the time of writing that used (quasi-)random judge assignment to estimate the effect of custodial versus noncustodial sanctions and some of their core features.Footnote2 Most previous studies compare imprisonment to probation in samples of adult offenders. Few studies compared custodial sentences to some noncustodial sentence other than probation, or use juvenile instead of adult samples. The results of these studies to some extent reflect differences in sample selection, historical time and geographic place, and different custodial and noncustodial regimes. Yet, the overall message from these studies is that the majority finds imprisonment to have either no effect or an enhancing effect on future criminal behavior compared to noncustodial alternatives. Only two prior studies find imprisonment to reduce crime; one is on juveniles—a group not under scrutiny here and only for property crime, nor for drug crime—the other is situated in Norway, a country known for its focus on offender rehabilitation. Findings from the latter study suggest that rather than deterrence, previously unemployed prisoners in Norway benefit from the vocational training programs that Norwegian prisons offer.
Table 1. Prior studies using random judge assignment to estimate effect of custodial versus noncustodial sanctions on recidivism.
Contribution of the Current Study
The present study adds to the extant literature in a number of ways. First, given widespread use of short-term imprisonment in various sentencing contexts, there is a surprising lack of analysis as to what the effects of short-term incarceration are on recidivism. In various Western-European countries, such as the Netherlands, Germany, Norway, Switzerland, and Denmark, a substantial share of those incarcerated serve prison sentences up to six months (Aebi & Delgrande, Citation2014). While in the US, prison sentences tend to be considerably longer than in Western Europe, the number of US citizens admitted to local jails greatly exceeds the number of those admitted to prisons and the average length of stay in US jails is likewise brief (Western et al., Citation2021). Short-term incarceration, whether experienced in prison or in jail, is thereby a common judicial sanction of which the effects on recidivism are presently far from clear.
Second, we use data from the Netherlands where single sitting judges may impose prison sentences up to one year (Art. 369 Dutch Code of Criminal Procedure). Within this boundary, judges enjoy high levels of autonomy in determining both the length and type of sanction. In the Netherlands, judges’ sanctioning discretion is not constrained by sentencing grids or mandatory minimum sentences, thus observed differences in punitivity can be taken to reflect personal preferences. Third, administrative regulations prescribe the procedure of allocation of cases to judges within the district court. Cases to be dealt with by single sitting judges are randomly assigned via an automated process based on the court’s work schedule. Though true random assignment is essential for judges to serve as an instrumental variable, prior work sometimes relies on quasi-random assignment and therefore remains vulnerable to biased estimates (also see Bhuller et al., Citation2020; Thorley, Citation2020).Footnote3 Fourth, although a limited number of prior studies have used a similar strategy to isolate imprisonment effects, our study is distinctive in that it compares imprisonment effects using multiple instrumental variable estimators, including a judge leniency/punitivity measure (Dahl et al., Citation2014; Dobbie et al., Citation2018).
Fifth, our data are from administrative records at the national level and thus applicable to the full population and not an idiosyncratic subpopulation. The data also include enough convicted offenders to study meaningful variation in imprisonment effects, which we take advantage of to explore potentially heterogeneous effects among different types of offenders. Addressing such variation is critical considering that “incarceration likely exerts no single, uniform effect but rather different effects depending on the counterfactual and the specific populations subject to incarceration or to other types of sanctions” (Mears et al., Citation2015, p. 693). Yet, few studies systematically investigate such variable effects of imprisonment. Sixth, our study covers a five-year follow-up period and uses both recidivism prevalence and recidivism incidence as outcomes measures, and includes analyses in which the severity of recidivism is taken into account.
Finally, pulling together all the features described above, our study provides causal estimates of the impact of custodial versus noncustodial sanctions on recidivism in a population of 16.75 million people in 2012—roughly the midpoint of the populations of Illinois and Florida in 2012 (the Netherlands would be the 5th most populous state), but in 1/4 the area of those states and thus of considerably higher population density than New Jersey, with a similar percentage white population as Pennsylvania and a homicide rate like New Hampshire. It has long been an objective of sentencing and corrections scholars to provide robust causal estimates of custodial punishments relative to alternatives, and across many different contexts (Nagin et al., Citation2009; Petrich et al., Citation2021; Villettaz et al., Citation2015). Hints from prior research that certain custodial sanctions might backfire and paradoxically worsen public safety adds urgency to the need to gather high-quality evidence on what the leading punishment alternatives might entail.
Data and Method
To investigate our research questions, we analyze data from the “Life after Release Study” (LRS), a full population national study of all offenders convicted by a judge in the Netherlands in 2012 (Wermink & Blokland, Citation2019). This study was approved by the Data and Ethics Committee from Leiden University, the Council for the Judiciary, the Netherlands Ministry of Justice and Security, and the Public Prosecutor’s Office.
The data were made available by the Research and Documentation Centre of the Netherlands Ministry of Justice and Security (WODC), and include defendant information (ethnicity, sex, age), offense information (type of crime, number of crimes committed), case information (imposed sentence), and criminal history information. Recorded arrest histories were reconstructed for all convicted offenders using information on every criminal case registered by the police at the Public Prosecutor’s Office starting at age 12 (the minimum age of criminal responsibility in the Netherlands) and ending in calendar year 2019.
This starting sample of convicted individuals was then merged to judge identifier information by the Public Prosecutor’s Office as data files from the WODC do not typically contain this information. We were granted access to this restricted judge information specifically for the purpose of this study. The Public Prosecutor’s Office was able to match data using common individual identifiers to no less than 99.8 percent of all cases, and 99.99 percent thereof included judge information resulting in a sample that consists of 61,129 individuals. We further made various selections to our dataset that invariably reduce the sample size eligible for further analysis. First, cases with unknown or inconsistent sentencing outcomes were removed (N = 1,119). Second, all cases not disposed of by a single sitting judge in criminal courts were removed (N = 13,303) because they are either governed by special provisions in the criminal code or they lack complete judge information. These cases primarily involved cases disposed of by a panel of judges or by judges in juvenile court. Third, juveniles younger than 18 and elderly older than 50 were removed (N = 5,287). Fourth, prison sentences longer than six months were excluded (N = 162).Footnote4 Fifth, measures and acquittals were removed (N = 3) as well as cases with data quality problems due to missing information (N = 116). After applying our restrictions, our baseline analysis sample consists of 735 judges and 41,139 unique defendants, of whom 6,564 (16%) were sentenced to prison.
Measuring Recidivism
The outcome variable in our study is recidivism after custodial or noncustodial sanction. All registered offenses that resulted in a conviction (excluding acquittals and technical waivers) after the index case are measured as recidivism, even when these subsequent registrations took place in 2012. Our analysis measures the prevalence and incidence of recidivism at 1, 3, and 5 years after conviction and after prisoners are released from prison.Footnote5 We also compare the prevalence and incidence of recidivism pre-sanction to prevalence and incidence of recidivism post-sanction to measure levels of improvement as noncustodial and custodial sanctions may both deter recidivism, but differ in the extent to which they are successful in doing so. In constructing our outcome variable, we account for time spend incarcerated by multiplying the observed number of registered crimes by the inverse of the proportion of the follow-up period offenders were actually free to offend. This implies that we assume that individuals would have been committing crimes at the same rate for the entire period had they been on the street instead of in prison. For instance, if an individual commits two crimes in a one-year follow-up period but was incarcerated for six months during this time, we count 4 crimes (2/((360-180)/360)). Because we are faced with skewed incidence outcome variables we transformed them by winsorizing at the 99th percentile. We further used date of death to account for mortality. Supplemental analyses revealed that death is largely uncorrelated with other relevant observables, and that imprisonment does not influence mortality for any of the follow-up times. Offenders who pass away are therefore omitted for each follow-up period.
Focal Independent Variable
The IV-analysis compares sentencing outcomes for individuals assigned to judges with different preferences to impose a prison sanction versus a noncustodial sanction. Imprisonment includes all unconditional custodial sanctions that imply offenders’ reduced freedom of movement resulting from their placement in a residential setting for a period of six months maximum. Noncustodial sanctions in turn are those sanctions not involving placement in a residential setting, which in the Dutch context predominantly refer to suspended sentences, fines and community service orders.
Using Judges as Instruments
The setting of this study includes the criminal divisions of all district courts in the Netherlands, which are governed by a uniform criminal procedure and national criminal law. All cases are handled by professional career judges and public prosecutors and the Dutch legal system is not jury based. This amounts to a caseload of 88,000 cases per year of which the vast majority is tried by single sitting judges (82%) (Choenni et al., Citation2019). The instruments in the current study are single sitting judges in the Netherlands who settled a case in 2012 in courts of first instance. Cases typically range from simple theft and minor drug possession to severe abuse and armed robbery. Single sitting judges can impose penalties up to one year imprisonment. Because the minimum term of a prison sentence in the Netherlands is one day and presumptive guidelines are absent, single sitting judges in the Netherlands enjoy broad discretionary powers in deciding over both the type and length of the sentence. Our empirical strategy exploits variation between judges in the propensity to impose a prison sentence to statistically isolate the influence of imprisonment on criminal behavior after release (Angrist, Citation2006; Angrist & Krueger, Citation2001; Angrist & Pischke, Citation2009).
Administrative regulations in each court lay down the procedure of allocation of cases to judges. Two types of cases are distinguished, namely those that need tailor-made allocation and cases that do not. Tailor-made cases pertain to specific complex categories of offending, such as human trafficking and large-scale fraud, and are typically handled by teams of specialized judges. In contrast, allocation of judges to cases handled by single sitting judges is unrelated to offense type.
Among single-sitting judges, new cases are randomly distributed within courts based on the courts’ work schedule, or zittingsrooster. Because cases are randomly allocated the chance to receive a certain sanction has an element of randomness. This randomization ensures that imprisoned offenders and non-imprisoned offenders are indistinguishable on all (un)observed characteristics that might be predictive of behavioral outcomes after release. Using variation in sentencing across judges, therefore, allows controlling for both observed and unobserved differences between imprisoned and non-imprisoned offenders. A balance test in which each observed covariate was regressed on the set of dummy variables for the assigned judges confirmed that the punitivity of a judge is indeed largely uncorrelated with defendant and case characteristics that could affect recidivism (see Appendix, ). This means that our instrument complies with the “independence” assumption. Nevertheless, we control for these observables in all instrumental variable models. Relatedly, the exclusion restriction in our application assumes that judges only influence recidivism outcomes via the imposed sanction. The exclusion restriction assumption is not empirically testable. For instance, it could be violated if, irrespective of the sanction imposed, the manner in which the judge treats the defendant strongly determines recidivism outcomes. However, research on effects of perceived procedural justice on recidivism suggests that such a scenario is unlikely (e.g. Yasrebi-De Kom et al., Citation2022). Moreover, in order to threaten the validity of the current instrument, possible differences in the way defendants are treated by judges would also have to be strongly correlated with their preference for a particular sentence. Based on prior work the exclusion-restriction assumption is plausible in the case of judge IVs.
Because Dutch judges have considerable discretion in sentencing it is to be expected that the severity of the imposed sentence varies systematically between different judges. This variation is important, because the intuition behind our design is to estimate the causal effect of interest (i.e. custodial vs. noncustodial sanction) using the variation in the “treatment” produced by the instruments. To test this, judges are ranked by punitivity estimated by the probability of imprisonment. As can be observed in , there is a wide spread in a judge’s tendency to incarcerate.
Figure 1. First stage—The probability of imprisonment by judge. Notes: The horizontal dashed line is the sample mean imprisonment probability. The size of the circle is proportional to the number of cases sentenced by each judge.
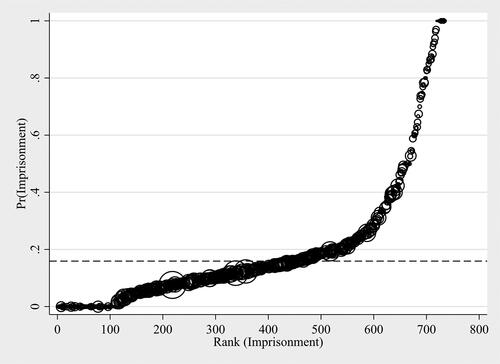
Also, we test whether the instruments are relevant by performing an F-test on the coefficients of the instruments in the first-stage regression, also referred to as the “relevance” assumption. The idea is that the instrument is relevant if it is strongly correlated with sanction (i.e. imprisonment rather than a noncustodial sanction). Typically, it is assumed that an instrument is ideal when the F-statistic exceeds 10 (Staiger & Stock, Citation1997). The F-statistic in our study is 5.50, but this is not necessarily problematic (Angrist & Pischke, Citation2009). F-statistics lower than 10 are common in the presence of many instruments, as is the case in our study and in prior criminological work (see for instance Harding et al., Citation2017), as well as in econometrics in general (e.g. Davies et al., Citation2014; Hansen & Kozbur, Citation2014). Given the potential problem of many weak instruments, we present estimates of two other instrumental variable estimators that are more robust to potentially weak instruments to check the robustness of our results.
In line with prior work, we use the two-stage least-squares (2SLS) estimator for the baseline model in which instruments are included as dummy variables (Harding et al., Citation2017; Loeffler, Citation2013). In addition to 2SLS, we present estimates from two other estimators that are available. The leading alternative estimator is the so-called limited information maximum likelihood (LIML) estimator which is less sensitive to potentially weak instruments. When there are many instruments, LIML tends to exhibit less bias than 2SLS. Additionally, we present judge stringency as the instrumental variable. This approach aligns with Dahl et al. (Citation2014), Dobbie et al. (Citation2018), and Bhuller et al. (Citation2020) and does not directly include dummies for each judge, but uses a more aggregated judge punitivity measure. We define judge punitivity as the share of imprisonment sanctions allowed by a judge on all other cases apart from the one being considered (i.e. a “leave-one-out” mean), and use this as an alternative instrument (first stage F-statistic is 924.77). All models are estimated using ivregress in Stata version 15, and condition on the effects of pre-sentence defendant, offense, case, and criminal history characteristics, and district court fixed effects (district court dummy variables).Footnote6 This latter is necessary given that cases are randomly distributed within courts. Measurement properties for all variables are shown in Appendix, .
Empirical Model
In order to estimate causal effects of imprisonment on recidivism, we estimate the following equations via 2SLS:
(1)
(1)
(2)
(2)
Here, depending on the outcome under scrutiny, y yields either recidivism prevalence or incidence and Prison a binary variable indicating whether person i received imprisonment (1) or a noncustodial sanctions (0) at time t. X’ is a vector of control variables including age, gender, country of birth, offense type, and court district. To estimate our effect of interest (
) causally we use D’ which is a vector of dummies indicating the judge. We provided empirical evidence for the validity and relevance of the instrument in the previous section. The error terms ε and ν are iid ∼ N(0,σ) and allowed to be correlated.Footnote7 The empirical model estimates a local treatment effect (LATE), which is the average treatment effect (ATE) for the sub-population that is treated only because they were sanctioned by a more punitive judge (Angrist & Pischke, Citation2009). The LATE approximates the ATE when the percentage of compliers in the population is high. All individuals who were sentenced to prison in 2012, according to the available data, actually served their prison sentence (this is referred to as compliance). This also applies to the vast majority of community service orders, as 86 percent of community service orders were successfully completed in 2012 (Kalidien, Citation2013). Importantly, failure to successfully complete community service does not always result in imprisonment. Furthermore, when estimating the probability of short-term imprisonment—i.e. the propensity score—the region of common support for those sentenced to imprisonment and those sentenced to a noncustodial sentence appears to be almost 100 percent. This means that for almost every value of the propensity score there are individuals in our data who actually received a short-term prison sentence, but also individuals who actually received a noncustodial sentence. This suggests that there are no (combinations of) observed personal and criminal characteristics that inevitably lead to a certain type of punishment—regardless of the judge one meets (always takers and never takers). Because of the large proportion of compliers in our study, LATE approximates ATE.
Besides that judge assignment is independent of the unobserved confounding variables, and that judge punitivity is only indirectly associated with the outcome variable through the imprisonment decision, to identify the local average treatment effect monotonicity has to be assumed. Monotonicity implies there are no individuals sentenced to imprisonment by judges that prefer noncustodial sanctions that would not have been sentenced to imprisonment by judges that prefer custodial sanctions, and likewise, that no individuals are sentenced to a noncustodial sanction by a harsher judge that would not have been so be a more lenient judge. Mueller-Smith (Citation2015) argues that monotonicity can be tested by estimating the following equation as the first-stage
(3)
(3)
Here, vector
measures the fact that offender’s characteristics may affect judges’ verdicts for imprisonment differently. As such, monotonicity no longer needs to be assumed across the entire sample, but only among groups of individuals defined by the observed variables. In the case of non-monotonicity, Mueller-Smith (Citation2015) shows that the bias from this approach is smaller than the bias from regular IV. Additional analyses suggest that monotonicity issues are likely to be small in our study, as the results of this approach align with our regular IV results in and .
Table 2. Estimated effects of imprisonment versus noncustodial sanctions on recidivism prevalence by time since release and IV estimator.
Table 3. Estimated effects of imprisonment versus noncustodial sanctions on recidivism incidence by time since release and IV estimator.
Unadjusted Estimates
For comparative purposes, we first show the unadjusted relationship between imprisonment and recidivism (i.e. the naïve comparison) and compare unadjusted pre- to post-sanction differences in criminal behavior without using an IV design. We then present the effects of imprisonment using instrumental variable estimators to adjust for pre-existing differences between individuals who have been imprisoned and those who have not.
shows the relationship between each judge’s use of imprisonment and recidivism rates, and shows the so called “reduced form” relationship between the instruments and recidivism using a follow-up period of 1, 3, and 5 years (see Angrist & Krueger, Citation2001). These reduced form estimates provide simple insight into the presence and direction of a correlation between sanction and recidivism. For each of the follow-up times it is clear that recidivism rates increase as a judge becomes more punitive. In practice this means that being sentenced by judges who more often impose a prison sanction is associated with higher levels of recidivism than being sentenced by more lenient judges. Appendix shows that this conclusion holds when different minimum caseload thresholds are used.
Figure 2. Mean recidivism rate during 1 yr, 3 yr, and 5 yr follow-up period, by judge’s tendency to incarcerate. Notes: The horizontal dashed lines are sample mean recidivism probabilities, while the angled solid lines are linear fits. The size of the circle is proportional to the number of cases sentenced by each judge.
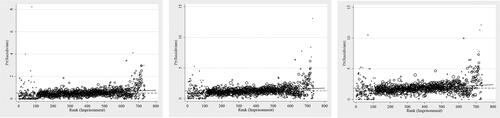
Does this correlation indicate that imprisonment is criminogenic? There are several interpretations for these findings. One suggests that while noncustodial sanctions deter crime, imprisonment does not; it could also be that imprisonment is criminogenic while noncustodial sanctions have no such effect. Alternatively, it could be the case that imprisonment and noncustodial sanctions both deter crime, but that noncustodial sanctions are more successful in doing so. Finally, imprisonment and noncustodial sanctions may both be criminogenic, but the crime increase after imprisonment is more pronounced. We attempt to adjudicate between these interpretations by modeling the level of unadjusted pre- to post-sanction differences in criminal behavior. graphs differences in recidivism for those sentenced to imprisonment and those sentenced to a noncustodial sanction for each of the follow-up times, comparing the incidence of criminal behavior one year prior to the sanction with one year after the sanction as well as for three and five years pre- to post. For each of the incidence variables we accounted for street time, mortality, and extreme outliers. Note that the results presented here are unadjusted estimates without using an IV design. This means that these results should not be interpreted as a test of the pre-to post sanction offending relationship but rather as a description of observed recidivism patterns.
Two findings are germane. First, comparing pre-to post-sanction levels of criminal behavior within a one-year window, there is an increase in criminal behavior after being released from prison and after having received a noncustodial sanction. T-tests confirm that these pre- to post-sanction differences are significant (p < .05). Because the incidence of criminal behavior in this naïve comparison is higher after punishment than before it seems that both sanctions induce some criminogenic effect. The relative increase in incidence of criminal behavior is substantially stronger after imprisonment (+19%) than after noncustodial sanctions (+10%). Second, we observe a decrease in the level of criminal behavior after punishment for both the three- and five-year period compared with the pre-sanction periods (t-3 and t-5). However, the longer the follow-up, the older individuals become, and individuals aging out of crime likely become increasingly important in explaining decreasing levels of criminal behavior across the three- and five-year period. Given the pre-existing differences between the imprisoned and noncustodial group it remains difficult to make meaningful comparisons between them. Therefore, the variation in the “treatment” produced by the instruments is used to make causal interpretations of the results regarding sanction effectiveness.
Results
Effects of Imprisonment on Recidivism
Next, the effect of imprisonment on recidivism measured at 1, 3, and 5 years after release was estimated using judge identifiers as instruments for sentence type. All models of our instrumental variable analysis also include other pre-sentence characteristics that are commonly used in the literature on sentencing disparity and effects of sentencing outcomes.
The results of this analysis for recidivism prevalence are reported in model 1 of . Model 1 shows that the effect of imprisonment is positive and highly significant. The positive sign suggests that imprisonment leads to more recidivism than noncustodial sanctions. Results show that being sentenced to prison rather than a noncustodial sanction increases the probability of criminal behavior in the first year after release by 17 percentage points. The difference in prevalence of recidivism between groups diminishes as the time since release becomes longer. After three years the difference is 10 percentage points, and after five years a 7 percentage point difference remains. Additional analyses show that approximately two thirds of the ex-prisoners who recidivate do so within the first year after release, whereas individuals with a noncustodial sanction take longer (the majority recidivates after the first year). Because ex-prisoners recidivate relatively quickly upon release, the magnitude of the difference in recidivism prevalence will by definition be less pronounced as the follow-up period increases. That said, we still observe significant positive differences in recidivism prevalence at any of the time periods after release, even in the long run.
To test the robustness of these findings, we reproduce these results using different instrumental variable estimators as alternative estimation frameworks. In model 2 and model 3, we report the results of the limited information maximum likelihood (LIML) estimator and the judge stringency instrument. Regardless of the chosen estimator, the results align with our findings in model 1. There is a significant positive effect of imprisonment on recidivism prevalence for each follow-up period (1, 3, and 5) when LIML and judge stringency are employed, and the magnitude of the effect is overall comparable. The large sample size, small standard errors, and LIML point estimates and standard errors that align with our 2SLS estimates indicate that the instruments included as dummy variables yield reliable estimates. Whether the quantity of offending is different after imprisonment than after noncustodial sanctions, however, remains opaque. Is it that only recidivism prevalence is higher for imprisoned individuals? Or do they also commit crimes more frequently in various follow-up times? Or is it both?
The results in model 1 of , where we model offending frequency using instrumental variable estimators, provide a clear answer: being sentenced to prison rather than a noncustodial sanction increases the incidence of recidivism at any of the time periods after release from prison.Footnote8 Not only are the effects of imprisonment versus noncustodial sanctions highly significant their magnitude is large. In the first year after release, being sentenced to imprisonment rather than a noncustodial sanction increases recidivism rates by 0.72 registered crimes. These effects grow considerable to 1.07 crimes within three years after release, and to 1.15 in the long run. In relative terms, imprisonment leads to an increase in recidivism of 76% compared to noncustodial sanctions within five years after release.Footnote9
In model 2 and model 3, we again report the results of the other instrumental variable estimators. When LIML and judge stringency are used, the results again align with our findings in model 1 in terms of significance level and direction of the treatment effect for each follow-up period. In addition, the effect sizes are substantively meaningful, ranging from a 1.23 to 1.18 absolute increase in recidivism rates after being sentenced to imprisonment rather than a noncustodial sanction in the five years after release. These estimates equal relative treatment effects of 81 percent and 78 percent, respectively.Footnote10
Combined, the results in and align with the theoretical arguments that, relative to noncustodial sanctions, being imprisoned increases offending behavior both in terms of prevalence as well as incidence. Moreover, the recidivism prevalence and incidence estimates of all instrumental variable estimators (2SLS, LIML, and judge stringency) are reassuringly close for each time period after release, providing greater confidence in our study’s findings.
Additional analyses in which we include all prison sentences imposed by single sitting judges, show that our results are largely generalizable to prison sentences up to one year. shows that significant criminogenic effects of imprisonment compared to noncustodial sanctions are found for recidivism prevalence and recidivism incidence for virtually all follow-up periods and IV estimators. Note that our main findings do not necessarily imply that imprisonment is criminogenic relative to noncustodial sanctions for all. Perhaps the “prison as crime preventative” and “prison as criminogenic” perspective are both correct because effects of imprisonment may vary by population. We examine the extent to which these effects are heterogeneous in .
Table 4. Estimated effects of prison sentences up to one year versus noncustodial sanctions on recidivism prevalence and incidence by time since release and IV estimator.
Table 5. 2SLS estimates of effects of imprisonment versus noncustodial sanctions on recidivism prevalence and incidence.
Effects of Imprisonment by Population and Severity of Repeat Offending
To investigate whether imprisonment effects vary by population, each set of 2SLS regression equations was re-estimated first on the subsample with first-time prisoners and then on the subsample with one or more prior prison spells. Additionally, we compare treatment effects for young adults aged 18 to 25 versus adults.
First, shows positive effects of imprisonment on recidivism prevalence and incidence for first-timers and repeated prisoners at any of the time periods after release, and, except for recidivism prevalence at five years for repeated prisoners, this effect is always significant. The effect of imprisonment on recidivism prevalence is most pronounced in the first year after release for both groups and becomes smaller as the time since release becomes longer. The criminogenic effect of imprisonment versus noncustodial sanctions is stronger for repeated prisoners than for first-timers. For instance, within a follow-up period of five years the difference in registered crimes is 1.52 and .33, respectively. The difference between these coefficients is significant (T-stat > 1.96). Additional analyses in which we take into account that the base rate of the control groups is different for the two subpopulations show that also the relative treatment effect is more pronounced for repeated prisoners than first-timers, with increased levels of recidivism of 48 and 33 percent respectively. Second, we observe some differences between young adults and adults. shows that the effect of imprisonment on recidivism prevalence is significant for adults at any time period after release, but not for young adults. In terms of recidivism incidence, we observe significantly higher levels of criminal behavior for both subpopulations at any time period after release. The absolute difference in the long run is 1.24 registered crimes for adults and .69 for young adults, and in relative terms the difference is 84 and 43 percent respectively.
Finally, also shows the effects of short-term imprisonment across different severity levels of repeat offending. Rather than counting crimes as equal, these analyses take into account that crime event harm varies between offenses. To do so, and following the categorization made by the Dutch ministry of Justice and Safety, we repeated the analysis distinguishing “serious crimes”—all crimes with a maximum penalty of 4 years or more—and “very serious crimes”—all crimes with a maximum penalty of 8 years or more. Given that Dutch judge’s discretionary power is not bound by mandatory minimum sentences, the maximum penalty can be argued to best reflect the legislator’s opinion with regard to the severity of the crime in question. shows that short-term imprisonment significantly increases recidivism prevalence and incidence of serious crimes at any time point after release, but it does not affect the prevalence and incidence of very serious crimes.
Combined, the results of imprisonment by population in align broadly with the sample-wide estimates reported in and . For all subpopulations, being sentenced to prison rather than a noncustodial sanction increases either the prevalence or incidence of criminal behavior, or both. In contrast, clear differences are observed across different severity levels of repeat offending as short-term imprisonment increases serious repeat offending behaviors, but has no effect on very serious repeat offending behaviors.
Conclusion and Discussion
Imprisonment is often perceived as society’s last resort in reaction to crime. The widespread use of short-term custodial sanctions however seriously questions the validity of this perception and provides room to consider noncustodial alternatives to imprisonment. To reduce criminal justice expenditures, noncustodial sanctions may be preferred over custodial ones provided that the former match the latter in their ability to prevent future crime. Causally valid estimates of the effects of custodial versus noncustodial sanctions are therefore pivotal for shaping penal policy.
In the Netherlands, as in many other jurisdictions, judges have extensive discretion, leaving room for different judges to act on different preferences when it comes to deciding the type of sanction to impose. As long as judges are not allowed to choose the cases they sentence, their preferences have a direct impact on the sentence a defendant receives, but only an indirect impact on recidivism through that sentence. The instrumental variables estimator, therefore, circumvents bias from potentially unobserved variables that plague many prior studies employing regression or matching designs. Given the frequent use of short-term imprisonment in many European jurisdictions, estimating these effects in a non-US setting is especially relevant. As a point of comparison, the (total) incarceration rate in the Netherlands in 2012 was 68 per 100,000 (as of 2021, it stands at 54 per 100,000), which makes the country a uniquely low-incarceration regime by US state standards, by an order of magnitude (Aebi et al., Citation2022).
Compared to noncustodial sanctions, we find short-term imprisonment to promote rather than deter recidivism, the latter increasing the incidence of future criminal behavior by 76 to 81 percent over a 5-year follow-up depending on model specification. It is important to emphasize that, in light of the random assignment of defendants to judges and the use of several instrumental variable estimators (2SLS, LIML, and judge stringency), this represents the causal effect of custodial punishment on recidivism. These criminogenic effects are not only found for all types of recidivism, but also for serious repeat offending behaviors specifically. The robustness of these findings strongly suggests that when it comes to preventing future crime and reducing criminal justice expenditures, noncustodial sanctions are the better option over short-term imprisonment. This was found for prison sentences up to six months as well as for prison sentences up to one year. Given that repeat offending is likely to result in harsher penalties, the benefits of noncustodial sanctions exceed current sanctioning and future victimization costs also by preventing escalation of punishment.
Criminogenic effects of custodial sanctions are typically found in contexts that fail to emphasize rehabilitative programming (Loeffler & Nagin, Citation2022). When short-term prison sanctions are concerned, this applies to the Netherlands as well. In contrast to those serving longer prison sanctions, prisoners serving short term sentences are not able to participate in Penitentiary Programs to support successful reintegration into society after release in the Netherlands. Moreover, reintegration efforts in terms of extramural employment activities are only possible when the prison sanction is longer than six months, and guidance and supervision from probation officers are largely absent after being released from short-term imprisonment (RSJ, Citation2021). The fact that we find substantial criminogenic effects may, in part, result from a lack of such rehabilitative programming. The remarkable consistency when comparing our IV-results with previous work based on regression and/or matching techniques (Loeffler & Nagin, Citation2022), also in the Netherlands (e.g. Wermink et al., Citation2010), seems to imply that, even though greater confidence can be placed in the accuracy of IV-estimates, issues of omitted variable bias in regression and/or matching based studies may have been overstated.
We find the criminogenic effects of short-term imprisonment to be more salient for repeat compared to first-time prisoners and adult versus young adult offenders. At present, based on the available data, we can only speculate why this is the case. It might be that young people and those sentenced to prison for the first time show more resilience in the face of the collateral consequences of imprisonment. For many, young adulthood is a period of insecurity in which ties to conventional institutions like work and marriage are highly flexible (Arnett, Citation2007). Besides insecurity, this flexibility may offer young adults the opportunity to begin with a clean slate more so than older adults who are less socially maneuverable. Likewise, romantic partners and employers might be more willing to give first-time prisoners the proverbial second chance, whereas repeat prisoners may face a social network that is less forgiving. To examine the differential effects of imprisonment by age and imprisonment history, and more general, the causal mechanisms that might underlie the observed difference in recidivism for those that served custodial versus noncustodial sanctions, in our future work we will seek to augment the current data with data on household composition, employment history and other relevant social ties. Adding these data to our analysis will provide for the opportunity to truly examine the effects different types of formal interventions from a life-course perspective.
Besides a lack of information on other life course domains, another obvious draw back of the present study is its reliance on registered crime, which is well-known to yield an image of individuals’ actual criminal behavior that is both selective and incomplete. To the extent that ex-prisoners, more so than those that served a noncustodial sanction, attract more attention from the police or are faced with more aggressive prosecution, changes in system behavior rather than offender behavior may explain the current findings. However, given the short-term nature of the sanctions examined, and the exchangeability of custodial and noncustodial sanctions under scrutiny, selective police attention directed to ex-prisoners does not seem an obvious alternative explanation of the current findings. Alternatively, to the extent that prison serves as an offender convergence setting and imprisoned offenders leave prison with the skills, opportunities and contacts to better hide their crimes from law enforcement, our results would underestimate rather than overestimate the criminogenic effects of imprisonment compared to noncustodial alternatives.
Finally, the purposes of punishment encompass more than preventing those sanctioned from committing future crime. To the extent that custodial sanctions are generally perceived as the “harsher” option, imprisonment might more strongly deter others beyond the current offender from committing future crime. Prior empirical research however concludes that, at least in the Netherlands, such general deterrent effects of short-term imprisonment over noncustodial alternatives appear negligible (Research & Documentation Centre, Citation2008). Furthermore, it could be argued that imprisonment more incontrovertibly conveys that moral boundaries have been crossed than do noncustodial sanctions. More so than fines or community service orders, imprisonment may quench victims’ thirst for personal avengement and enhance the faith in the criminal justice system in society at large. Dutch legislative history seems to corroborate this line of reasoning proscribing community service orders for those convicted of certain sexual offenses involving child victims since 2012.
While the current analyses do not speak directly to retribution, the observed differences in recidivism following custodial versus noncustodial sanctions are nevertheless relevant in this respect. To the extent that short-term imprisonment results in more future crimes than do noncustodial alternatives, the societal costs involved in the detection, prosecution, and sanctioning of the perpetrators of these future crimes, as well as the personal costs incurred by its victims, will need to be reckoned with when aspiring the retribution of present wrongs. Results from the present analyses may provide the basis for meticulously evaluating these costs, and as such contribute to social debate on the favored governmental reaction to crime.
Acknowledgements
The authors gratefully acknowledge funding from the Gratama-foundation and The Netherlands Organisation for Scientific Research (451-17-018). The authors further thank the Research and Documentation Centre of the Dutch Ministry of Justice and the Dutch Public Prosecutors Office for facilitating access to the data and for providing advice on their use. An earlier version of this article was presented at the 2019 annual meeting of the American Society of Criminology in San Francisco.
Data Availability Statement
Restrictions apply to the access of the data that support the findings of this study as these data can only be reused if permission has been granted by all relevant authorities, i.e. Statistics Netherlands (CBS), Public Prosecutor’s Office, and the Research and Documentation Centre of the Netherlands Ministry of Justice and Security (WODC). The CBS and WODC only grant data access to institutions, services and organizations that are located in countries that fall under the scope of the GDPR (the European Economic Area) or to which an adequacy decision of the European Commission applies.
Disclosure Statement
No potential conflict of interest was reported by the authors.
Notes
</sup> Among the studies reviewed by Loeffler and Nagin (Citation2022) are 8 that estimate the effect of a custodial versus noncustodial sanction on recidivism, and an additional 5 that estimate the impact of pretrial detention on recidivism. Given our interest is in the use of prison as a punishment, the former group of studies is more directly relevant.
2 While many jurisdictions use computerized “randomizers” in allocating cases to calendars or judges, judge assignment is sometimes quasi-random, based on rotation systems or allowing some degree of discretion to administrative personnel in overruling true random assignment to accommodate balancing of the workflow (e.g. Green & Winik, Citation2010; Dobbie et al., Citation2018). Important for judge assignment to be valid as an instrumental variable in these cases, is that despite not being truly random in a statistical sense, the assignment of judges does not depend in any way on the characteristics of the case or the accused.
3 Thorley (Citation2020) proposes speaking with individuals who are in charge of the case assignment process in the court system. In our case, prior to data collection we consulted Prof. Pauline Schuyt, who has years of personal experience serving as a judge, and hence has inside knowledge of the court. Also, attrition is viewed as a potential driver of biased outcomes, in particular when missingness is correlated with the recidivism outcomes. We use administrative data from the Netherlands which are known to be rich, of high quality, and complete, and include common identifiers that can be used to link data from various sources. Indeed, missing data is virtually non-existent in our dataset. We only excluded individuals to account for mortality which appeared to be unrelated to other relevant observables including being imprisoned.
4 As of 1989, criminal courts in the Netherlands may impose community service orders of maximally 240 hours only to replace an unconditional prison sanction of six months or less, as stated in the Penal Code. Since the introduction of community sanctions as a primary penalty option in 2001, conversion keys in the Netherlands also do not allow community services to replace prison terms exceeding six months. Prisoners serving longer prison sentences (i.e. > than 6 months) may therefore not be truly “at risk” for at least some noncustodial sentencing options and we exclude them from our main analyses. However, to be better able to compare our estimated effects of imprisonment with findings of prior empirical studies, and to indicate whether our findings seem to be generalizable to longer prison sentences, in addition to the main analyses, we present IV results for all prison sentences up to one year.
5 Even though some studies measure the effects of imprisonment from the time of disposition (Harding et al., Citation2019), we focus on the effects after release because most prison sentences imposed are so short that incapacitation does not seem to be a plausible aim of punishment among single sitting judges in the Netherlands. Additional analyses in which the recidivism clock starts at the time of disposition show that the effects of short-term imprisonment are the same up to one decimal place for all 2SLS recidivism prevalence and incidence outcomes. This implies that incapacitation effects of short-term prison sentences are indeed negligible in the Netherlands, and that short-term imprisonment is criminogenic even when incapacitation effects are taken into account. These results of this analysis are available upon request.
6 Code/syntax is available upon request from the corresponding author.
7 The error terms are independent and identically distributed (iid) and follow a normal distribution approximately with mean zero and variance sigma. Additionally, the 2SLS assumes that the error terms of the first-stage and second-stage are arbitrarily correlated. In our specific case, this means that recidivism covaries with the sanction. Estimation results are robust to using a probit instead of a linear probability model in the first-stage (not reported here).
8 Each set of regression equations was re-estimated first using incidence outcomes without outlier adjustment and second using incidence outcomes winsorized at the 5% tail (not reported here). The results align with the results reported in table 2 in terms of sign and significance of the treatment effects at each follow-up period. Therefore, these additional analyses seem to strengthen the reliability of the positive effect of imprisonment versus noncustodial sanctions in our main results.
9 The relative treatment effect equals the absolute treatment effect divided by the average recidivism rate in the control condition, that is after being sentenced to a noncustodial sanction, times 100. Consequently, the relative treatment effect depicts the deviation of the base rate (mean recidivism of the control group) in percentages. The mean five-year recidivism incidence for individuals sentenced to noncustodial sanctions is 1.52, and after imprisonment 1.15 more crimes are registered. The relative increase in criminal behavior equals 76 percent ((1.15/1.52)*100).
10 Our IV-models include pre-sentence characteristics, because this increases precision and reduces effect size bias (Lee, Citation2016). Yet, in Appendix Table 3 we present the regression results without these covariates. Again the recidivism prevalence and incidence results align with our main findings in Table 2 and 3 in terms of significance level and direction of the treatment effect for each follow-up period and for all instrumental variable estimators. Also, the size of the standard errors is reassuringly close to those reported in the main text. In Appendix Table 4 we also present the IV-regression models with different caseload thresholds to address the issue of unmeasured defendant characteristics, and diminish concerns regarding noise in the construction of our judge punitivity measure. We first present the results excluding all judges who handled fewer than 10 cases as it is relatively common in the Netherlands that judges handle very few cases per year (i.e. 20% of all judges handled fewer than 10 cases). When using this threshold, we find positive and significant effects of short-term imprisonment compared to noncustodial sanctions on repeat offending for all outcome measures at each follow-up period. In line with Eren and Mocan (Citation2021), we additionally exclude individuals whose disposition judge has handled fewer than 25 criminal cases. The results show that only the effects on recidivism prevalence during the five-year follow-up period become non-significant, but all other effects on recidivism prevalence (i.e. one- and three-year follow-up) and recidivism incidence remain positive and significant.
References
- Aebi, M. F., & Delgrande, N. (2014). Council of Europe annual penal statistics, SPACE I – Prison populations – Survey 2012. Council of Europe.
- Aebi, M. F., Cocco, E., Molnar, L., & Tiago, M. M. (2022). Prison populations, SPACE 1 – 2021. Council of Europe.
- Aizer, A., & Doyle, J. J. Jr. (2015). Juvenile incarceration, human capital, and future crime: Evidence from randomly assigned judges. The Quarterly Journal of Economics, 130, 759–803.
- Angrist, J. D. (1990). Lifetime earnings and the Vietnam era draft lottery: Evidence from social security administrative records. American Economic Review, 80, 313–336.
- Angrist, J. D. (2006). Instrumental variables methods in experimental criminological research: What, why and how. Journal of Experimental Criminology, 2, 23–44.
- Angrist, J. D., & Krueger, A. B. (2001). Instrumental variables and the search for identification: From supply and demand to natural experiments. Journal of Economic Perspectives, 15, 69–85.
- Angrist, J. D., & Pischke, J.-S. (2009). Mostly harmless econometrics: An empiricist’s companion. Princeton University Press.
- Arnett, J. J. (2007). Emerging adulthood: What is it, and what is it good for? Child Development Perspectives, 1, 68–73.
- Bagaric, M. (2020). The contours of a utilitarian theory of punishment in light of contemporary empirical knowledge about the attainment of traditional sentencing objectives. In F. Focquaert, E. Shaw, & B. N. Waller (Eds.), The Routledge handbook of the philosophy and science of punishment (pp. 62–74). Routledge.
- Bhuller, M., Dahl, G. B., Løken, K. V., & Mogstad, M. (2020). Incarceration, recidivism, and employment. Journal of Political Economy, 128, 1269–1324.
- Choenni, R., Van den Baak, S. W., & Platenburg, P. F. M. (2019). Criminaliteit en rechtshandhaving. Ontwikkelingen en samenhangen [Crime and law enforcement. Trends and correlations]. Research and Documentation Centre/Statistics Netherlands.
- Dahl, G. B., Kostol, A. R., & Mogstad, M. (2014). Family welfare cultures. Quarterly Journal of Economics, 129, 1711–1752.
- Damm, A. P., & Gorinas, C. (2020). Prison as a criminal school: Peer effects and criminal learning behind bars. The Journal of Law and Economics, 63, 149–180.
- Davies, N. M., Von Hinke Kessler Scholder, S., Farbmacher, H., Burgess, S., Windmeijer, F., Davey, & Smith, G. (2014). The many weak instruments problem and Mendelian Randomization. Statistics in Medicine, 34, 454–468.
- Dobbie, W., Goldin, J., & Yang, C. (2018). The effects of pre-trial detention on conviction, future crime, and employment: Evidence from randomly assigned judges. American Economic Review, 108, 201–240.
- Doherty, D., Gerber, A. S., & Green, D. P. (2006). Personal income and attitudes toward redistribution: A study of lottery winners. Political Psychology, 27, 441–458.
- Dünkel, F. (2017). European penology: The rise and fall of prison population rates in Europe in times of migrant crises and terrorism. European Journal of Criminology, 14, 629–653.
- Eren, O., & Mocan, N. (2021). Juvenile punishment, high school graduation, and adult crime: Evidence from idiosyncratic judge harshness. The Review of Economics and Statistics, 103, 34–47.
- Green, D. P., & Winik, D. (2010). Using random judge assignments to estimate the effects of incarceration and probation on recidivism on drug offenders. Criminology, 48, 357–387.
- Hansen, C., & Kozbur, D. (2014). Instrumental variables estimation with many weak instruments using regularized JIVE. Journal of Econometrics, 182, 290–308.
- Harding, D. J., Morenoff, J. D., Nguyen, A. P., & Bushway, S. D. (2017). Short- and long term effects of imprisonment on future felony convictions and prison admissions. Proceedings of the National Academy of Sciences of the United States of America, 114, 11103–11108.
- Harding, D. J., Morenoff, J. D., Nguyen, A. P., Bushway, S. D., & Binswanger, I. A. (2019). A natural experiment study of the effects of imprisonment on violence in the community. Nature Human Behavior, 3, 671–677.
- Jonson, C. L. (2010). The impact of imprisonment on reoffending: A meta-analysis Unpublished doctoral dissertation, University of Cincinnati.
- Kalidien, S. N. (2013). Tenuitvoerlegging van sancties. In S. N. Kalidien & N. E. de Heer-de Lange (Eds.), Criminaliteit en rechtshandhaving 2012 (pp. 153–178). Boom Uitgevers.
- Killias, M., Aebi, M., & Ribeaud, D. (2000). Does community service rehabilitate better than short-term imprisonmen?: Results of a controlled experiment. The Howard Journal, 39, 40–57.
- Kirk, D. S., & Wakefield, S. (2018). Collateral consequences of punishment: A critical review and path forward. Annual Review of Criminology, 1, 171–194.
- Kling, J. R. (2006). Incarceration length, employment, and earnings. American Economic Review, 96, 863–876.
- Kopf, S., & Mowen, T. J. (2020). Collateral consequences of incarceration. In L. A. Gould & J. J. Brent (Eds.), Routledge handbook on American prisons (pp. 271–279). Routledge.
- Lee, P. H. (2016). Covariate adjustments in randomized controlled trials increased study power and reduced biasedness of effect size estimation. Journal of Clinical Epidemiology, 76, 137–146.
- Loeffler, C. E. (2013). Does imprisonment alter the life course? Evidence on crime and employment from a natural experiment. Criminology, 51, 137–166.
- Loeffler, C. E., & Nagin, D. S. (2022). The Impact of incarceration on recidivism. Annual Review of Criminology, 5, 133–153.
- Mears, D. P., Cochran, J. C., & Cullen, F. T. (2015). Incarceration heterogeneity and its implications for assessing the effectiveness of imprisonment on recidivism. Criminal Justice Policy Review, 26, 691–712.
- Mueller-Smith, M. (2015). The criminal and labor market impacts of incarceration. Working paper. 1–59. https://sites.lsa.umich.edu/mgms/wp-content/uploads/sites/283/2015/09/incar.pdf
- Nagin, D., Cullen, F. T., & Jonson, C. L. (2009). Imprisonment and reoffending. In M. Tonry (Ed.), Crime and justice (Vol. 38, pp. 115–200). University of Chicago Press.
- Nagin, D. S., & Snodgrass, G. M. (2013). The effect of incarceration on re-offending: Evidence from a natural experiment in Pennsylvania. Journal of Quantitative Criminology, 29, 601–642.
- Pager, D., Western, B., & Sugie, N. (2009). Sequencing disadvantage: Barriers to employment facing young Black and White men with criminal records. The Annals of the American Academy of Political and Social Science, 623(1), 195–213. https://doi.org/10.1177/0002716208330793
- Petersilia, J., & Deschenes, E. P. (1994). Perceptions of punishment: Inmates and staff rank the severity of prison versus intermediate sanctions. The Prison Journal, 74, 306–328.
- Petrich, D. M., Pratt, T. C., Jonson, C. L., & Cullen, F. T. (2021). Custodial sanctions and reoffending: A meta-analytic review. Crime and Justice, 50, 353–424.
- Phelps, M. S., & Pager, D. (2016). Inequality and punishment: A turning point for mass incarceration? The Annals of the American Academy of Political and Social Science, 663, 185–203.
- Pompoco, A., Wooldredge, J., Lugo, M., Sullivan, C., & Latessa, E. J. (2017). Reducing inmate misconduct and prison returns with facility education programs. Criminology & Public Policy, 16, 515–547.
- Rahman, S., & Weahterburn, D. (2021). Does prison deter drunk-drivers? Journal of Quantitative Criminology, 37, 979–1001.
- Research and Documentation Centre. (2008). Afschrikking en generale preventie [Deterrence and general prevention]. Justitiële Verkenningen, 34, 1–140.
- RSJ – The council for the administration of criminal justice and protection of juveniles. (2021). Korte detenties nader bekeken [A closer look on short-term imprisonment]. RSJ.
- Smith, P., Gendreau, C., & Goggin, C. (2002). The effects of prison sentences and intermediate sanctions on recidivism: General effects and individual differences. User Report 2002-01, Public Safety Canada, Ottawa, ON.
- Staiger, D., & Stock, J. H. (1997). Instrumental variables regression with weak instruments. Econometrica, 65, 557–586.
- Thorley, D. (2020). Randomness pre‐considered: Recognizing and accounting for “de‐randomizing” events when utilizing random judicial assignment. Journal of Empirical Legal Studies, 17(2), 342–382.
- Villettaz, P., Killias, M., & Zoder, I. (2006). The effects of custodial vs. non-custodial sentences on re-offending: A systematic review of the state of knowledge. Campbell Systematic Reviews, 2, 1–69.
- Villettaz, P., Gillieron, G., & Killias, M. (2015). The effects on re-offending of custodial vs. non-custodial sanctions: An updated systematic review of the state of knowledge. Campbell Systematic Reviews, 11, 1–92.
- Western, B., Davis, J., Ganter, F., & Smith, N. (2021). The cumulative risk of jail incarceration. Proceedings of the National Academy of Sciences of the United States of America, 118, 1–6.
- Wermink, H. T., Blokland, A. A. J., Nieuwbeerta, P., Nagin, D., & Tollenaar, N. (2010). Comparing the effects of community service and short-term imprisonment on recidivism: A matched samples approach. Journal of Experimental Criminology, 6(3), 325–349.
- Wermink, H. T., Apel, R., Nieuwbeerta, P., & Blokland, A. A. J. (2013). The incapacitation effect of first-time imprisonment: A matched samples comparison. Journal of Quantitative Criminology, 29, 579–600.
- Wermink, H. T., & Blokland, A. A. J. (2019). Criminal careers and life-course circumstances of Dutch sentenced offenders (Codebook and Documentation). Leiden University, Leiden.
- Yasrebi-De Kom, F. M., Dirkzwager, A. J., Van Der Laan, P. H., & Nieuwbeerta, P. (2022). The effect of sanction severity and its interaction with procedural justice. Criminal Justice and Behavior, 49(2), 200–219.
Appendix
Figure A1. Mean recidivism rate during 1 yr, 3 yr, and 5 yr follow-up period by judge’s tendency to incarcerate using different minimum caseload thresholds. (A) Minimum caseload ≥ 10. (B) Minimum caseload ≥ 25. Notes: The horizontal dashed lines are sample mean recidivism probabilities, while the angled solid lines are linear fits. The size of the circle is proportional to the number of cases sentenced by each judge.
Table A1. Randomization checks—global F-tests
Table A2. Measurement properties for all variables
Table A3. Estimated effects of imprisonment versus noncustodial sanctions on recidivism prevalence and incidence without covariates (other than fixed effects).
Table A4. Estimated effects of imprisonment versus noncustodial sanctions on recidivism prevalence and incidence using different caseload thresholds