ABSTRACT
Shift work is a common occupational exposure, however, few studies have examined aspects of shift work beyond night work and long hours, such as rotational patterns or weekend work, which may contribute to poor health through disruption of the body’s circadian rhythms. In this manuscript, we calculated the prevalence of working hour characteristics using algorithms for type (e.g., day), duration, intensity, rotational direction, and social aspects (e.g., weekend work) in a nationwide cohort of American manufacturing workers (N = 23,044) between 2003 and 2014. Distributions of working hour characteristics were examined by schedules (e.g., permanent day, day/night) and demographics, and were cross-classified in a matrix to examine co-occurrence. Approximately 55% of shifts may cause circadian rhythm disruption as they were non-day shifts or day shifts with a quick return or rotation, or were 13 h or longer. Older workers, female workers, and White workers worked permanent day shifts most often, while workers of color worked more day/night schedules. Night and evening shifts had more frequent shift rotations, quick returns, and longer hours than day shifts. Yet, day shifts, which are presumed to have little negative circadian impact, may cause circadian rhythm disruption as long hours, quick returns and rotations also occurred within day shifts.
Introduction
Shift work continues to be an important occupational exposure with a prevalence of near 20% of the US workforce (Demetriades and Pedersini Citation2008; Straif et al. Citation2007; United States Bureau of Labor Citation2004). Shift work has been associated with increased risk of cardiovascular disease (Akerstedt et al. Citation1984; Hublin et al. Citation2010; Lunde et al. Citation2020; Mosendane et al. Citation2008; Skogstad et al. Citation2019), injury (Mustard et al. Citation2013; Nielsen et al. Citation2011), hypertension (Manohar et al. Citation2017; Ferguson et al. Citation2019), breast cancer (Hansen Citation2017), psychological and mental health disorders (Costa Citation2010; Lee et al. Citation2017), diabetes (Gan et al. Citation2015; Knutsson and Kempe Citation2014; Morikawa et al. Citation2005; Pan et al. Citation2011), metabolic syndrome (Lin et al. Citation2009; Lu et al. Citation2017; Wang et al. Citation2014) and fatigue (Cunningham et al. Citation2022). However, the term ‘shift work’ is often vaguely defined and generically used to describe any non-standard work schedule that has one or more characteristics such as occurring at night, changing start times (rotational work), or long hours (Straif et al. Citation2007). Yet, definitions of these characteristics of shift work are not standardized or well described across working populations. Moreover, few studies have examined aspects of shift work beyond night work and long hours, such as rotational pattern, limited time off, or weekend work, which may also contribute to increased risks of adverse health outcomes.
To clarify adverse aspects of shift work and improve exposure classification, more specific definitions of shift work have recently been proposed – “working hour characteristics” (Stevens et al. Citation2011). Working hour characteristics classify specific components of work, such as night work or long hours, which may be relevant for worker health. Working hour characteristics were categorized into large ‘domains’ of interest defined by the International Agency for Research on Cancer Working Group in 2009 following their 2007 classification of “shift work that involves circadian rhythm disruption as a probable human carcinogen” (Stevens et al. Citation2011; Straif et al. Citation2007). While many domains were identified in the IARC report, five key domains of interest that can be calculated from time registry data are 1) Shift type, 2) Duration, 3) Intensity, 4) Rotational Pattern, and 5) Social aspects of working time; with each containing several working hour characteristics (Stevens et al. Citation2011; Straif et al. Citation2007). These five domains are described as follows:
The shift-type domain contains working hour characteristics of morning, day, evening, and night shifts. These working hour characteristics are defined by starting time and duration, to estimate displacement from solar day and the resulting circadian phase shift. While the impact of night shifts on human health have been studied extensively (Hansen Citation2017; Manohar et al. Citation2017), shifts with start times in early morning or evening may be equally disruptive to circadian rhythms, while day shifts are presumed to have a no impact (Härmä et al. Citation2015).
The duration domain characterizes the length of shift, workday, or work weeks in order to capture the displacement of normal sleeping times. Long working hours are risk factors for diabetes and cardiovascular disease (Hannerz et al. Citation2018a,Citationb; Kivimäki et al. Citation2015a,Citationb). Longer shifts may indirectly cause circadian rhythm disruption by reducing recovery time between working periods which impacts the speed at which a worker entrains their circadian rhythm to solar day (Stevens et al. Citation2011). Long working hours may also independently impact worker health through sleep disruption and resulting fatigue (Cunningham et al. Citation2022).
The intensity domain contains working hour characteristics such as quick returns, which are designed to capture reduced recovery time between working periods, while the rotational direction domain contains working hour characteristics like the frequency and direction of rotation. By capturing rotation frequency, we hope to estimate the effect of repeat circadian rhythm phase shifts (Stevens et al. Citation2011; Straif et al. Citation2007).
The rotational direction domain distinguishes clockwise/forward rotating (morning to afternoon to night shift) from counter clockwise/backward rotating (night to afternoon to morning shift). The last domain, social aspects of working time, includes working hour characteristics such as weekend work which are critical for maintaining regularity of household and family tasks (Straif et al. Citation2007).
Previous studies suggest working hours that combine multiple characteristics, such as night work and rotating shifts, may confer multiplicative or more than additive increases in risk of adverse health outcomes (Ferguson et al. Citation2019; Straif et al. Citation2007). Prior work has also emphasized the need to conceptualize working hour characteristics as a ‘ecosystem’ rather than evaluate risks in one dimension at a time (Ferguson and Dawson Citation2012). However, evidence is limited as the co-occurrence of the different characteristics among a worker population have not been examined; as cohort studies to date are not well suited to classify more than one domain due to lower resolution data sources such as surveys (Härmä et al. Citation2015). A major limitation of the existing literature is the lack of detail regarding shift work exposure. Most studies classify shift work into dichotomous metrics of night work vs. day work, rotational work vs. non-rotational work, or long hours vs. regular hours due to self-reported and low-definition data sources such as questionnaires. These general and individual classifications limit our understanding of which components of shift work are responsible for increased risk of poor health through circadian rhythm disruption. In order to understand the etiological effect of shift work on human health, it is critical to examine the joint impact of working hour characteristics.
The goal of this study was to operationalize these domains in a US manufacturing cohort using daily administrative time clock data. In this report, we identify the co-occurrence of different working hour characteristics classified by domains of shift type, duration, intensity, rotation pattern, and social aspects of working time in a cohort of light metal manufacturing workers. We also identify potential social disparities in exposures potentially related to circadian rhythm disruption by describing the distribution of working hour characteristics by demographics and annual shift schedules.
Methods
Study population
The subset of the American Manufacturing Cohort (AMC) population eligible for this analysis includes 28,331 active hourly workers with time-registry data (Elser et al. Citation2019). Workers were employed between 2003 and 2014 and performed blue-collar work (i.e. jobs requiring manual labor) in smelters, refineries and fabrication in jobs such as anode assembly operator, sheet finishing, pack/ship operator, casting, autoclaving, and electrical or mechanical maintenance (Noth et al. Citation2013). Information on employee demographics and employment histories were obtained from deidentified company personnel files, employment records, and insurance claims. Workers were employed in 54 plants; 51 of which operated 24 h a day, 7 d a week. To evaluate the distribution of work time patterns among full-time employees, the study population was limited to employees working more than 150 work days a year (N = 23,095) and excluded 5,236 part-time workers. Plants with fewer than 50 employees were excluded (N = 3 plants, 51 workers). The final study population included 23,044 workers with over 22.4 million shifts at 51 plants.
Sources of working time & data quality
Daily working hour data retrieved from two time-registry systems spanning 2003–2009 and 2009–2014 were used to calculate hours worked from January 1st, 2003 through December 31, 2014. The data included details on start and end times of a shift to every billable second (i.e., the smallest time unit) and their associated pay codes (e.g., surcharges due to night shifts, overtime hours, call-in work). Data also included what each unit of time corresponded to in terms of active work time and compensation (e.g., grace time to put on personal protective equipment and walk to station, sick time/paid time off, vacation, or unexcused absence).
Time data were cleaned following previously described time-oriented data cleaning taxonomies (Gschwandtner et al. Citation2012). Consecutive working hours with less than one hour between them were considered one continuous shift as short gaps between billable hours were overwhelmingly associated with and coded as meal breaks. Data were compressed into one row keeping the earliest starting time and/or the latest ending time. Shifts over 18 h (<1%) were excluded as they likely represented paid time during which employees were allowed to sleep (e.g., manager on-call hours). Shifts less than 3 h were excluded (<1%) similar to prior work as shifts smaller than 3 h cannot be defined as a night or day shift (Härmä et al. Citation2015). Shifts that represented paid and non-paid time off work (e.g., sick leave, vacation, etc.) were excluded (N = 2.5 million)
Definitions of annual shift schedule
Person-years were classified into annual shift schedules by combinations of permanent and rotating day, evening, and night. Shift schedules were defined using definitions developed by Garde et al. (Citation2018), where schedules are intended to capture the predominant pattern of work. For example, a “permanent day schedule” was a person-year with ≥6.7% day shifts (10 or fewer shifts a year) and <6.7% evening and <6.7% night shifts. In comparison, a “day/night schedule” had ≥6.7% day and ≥6.7% night shifts but <6.7% evening shifts (Garde et al. Citation2018). Morning shifts were considered day shifts when defining yearly shift schedules in this cohort due to their rarity and similarity in start times and duration similar to work by Garde et al. (Citation2018).
Definitions of working time characteristics
The definitions for each working hour characteristic are presented in Appendix Table A1. Shifts were classified using binary definitions for working time data developed by Härmä et al. (Citation2015) and Garde et al. (Citation2018) for shift type, quick returns, and long shifts. These definitions were first implemented in a Scandinavian healthcare work force (Garde et al. Citation2018). Therefore, to accommodate the norm of longer shifts and more hours worked during the week in this sector of the American manufacturing work force, supplemental definitions were added by modifying binary variable cut-points. Modifications included a secondary definition for long shifts (from ≥12 h to ≥13 h) and two alternative definitions for long work weeks (from ≥40 h to ≥48 and ≥60 h). The definition for a morning shift was also slightly adapted to fit this population as workers for the 6:00am shift often clocked in 10–15 min prior to their shift start to prepare for their shift (e.g., unpaid time where workers put on protective equipment and walk to work station). Therefore, we defined morning shifts as a shift that starts after 03:00 and not later than 05:30 (Härmä definition: 06:00). Day shifts were defined as a shift that starts after 05:30 (Härmä definition: 06:00) and ends no later than 21:00. Novel definitions for the direction of rotations were developed from previously applied definitions for classifying rotations in this cohort (Ferguson et al. Citation2019).
Statistical analysis
We calculated the frequency of working hour characteristics of shift type, quick returns, long shifts, rotation direction, and weekend work over 12 years of data. We then examined four joint distributions. First, the distribution of annual shift schedule by age, race and gender was examined (i.e., what proportion of Black workers worked the permanent night shift schedule). Second, the prevalence of each working hour characteristic by person-year and annual shift schedules was examined (i.e., what proportion of shifts worked by permanent day workers were night shifts). Third, the prevalence of each working hour characteristic by age, race and gender (i.e., what proportion of shifts worked by Black workers were night shifts). Fourth, each working hour characteristic was cross-classified in a matrix to examine its co-occurrence with all other working hour characteristics (i.e., what proportion of night shifts were also long shifts).
Non-parametric tests of trend across ranks of ordered groups (an extension of the Wilcoxon rank sum test) (Cuzick Citation1985) and chi-squared tests were used to identify trends and the statistical significance of differences (α = 0.05) between categories of annual shift schedules, race, age categories, and gender. All data cleaning and statistical analyses were conducted on de-identified data and performed in Stata version 15, 2017 (StataCorp LLC, College Station TX). Clock plot graphics were produced using R software (version 3.5.2) with code adapted from Zoonekynd et al. (Zoonekynd and Gama Citation2012). The Institutional Review Board at the University of California, Berkeley (Protocol ID: 2010–07-1823) and at Stanford University (Protocol ID: 55306) approved this study.
Results
This study population includes 23,044 eligible workers () with over 22.4 million shifts worked. While the cohort comprised mostly White men between ages 30–60 y old, 25% of the cohort was non-White and the most common racial group after White was Black/African American (14% of workers). Of the 51 plants in the cohort, only seven were followed for the full 12 years. The mean length of follow-up across locations was 4 years. Due to the change in time-registry systems in 2009 and the acquisition of new plants, the distribution of plants and employees changed over the course of the study period. On average, for each year between 2003 and 2008, roughly 7,000 workers were employed in 30 plants. In 2009, the year of transition between the two time-registry systems, only 4,183 were employed in 17 plants, but between 2010 and 2014, on average 10,500 workers were employed across 31 plants, representing new plant acquisitions.
Table 1. Demographic characteristics by annual shift schedule in the American Manufacturing Cohort (AMC) 51 plant shift work cohort 2003–2014 (N = 98,771 person-years).
All plants operated with either two 12-h shifts or three 8-h shifts. The majority of the plants operated 24 h, 7 d a week; however, three plants had a day shift of either 8 or 12 h but no night shift. As seen in plot titled “All Shifts,” plants staffed fewer workers at night compared to during the day. However, the presence of night workers varied by location from a skeleton staff to a modest 10% reduction in workforce at night (data not shown).
Figure 1. Proportion of worker-hours by hour in a 24-h clock: stratified by shift type in the American Manufacturing Cohort (AMC) 51 plant cohort 2003–2014, USA (N = 98,771 person-years).
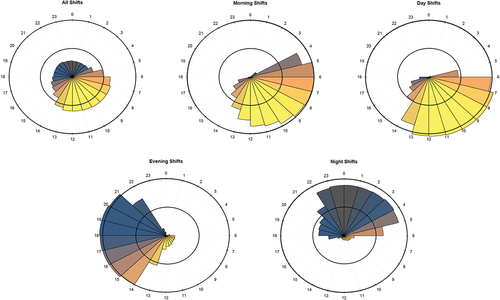
Distribution of demographics by annual shift schedule
Approximately half of the workers were on a rotational schedule, while the other half worked a permanent schedule each year (). The most common annual schedules were permanent day and rotating day/night. Differences in the distribution of annual schedules were identified by gender, race and age. Men were more likely to work rotational schedules than women (54.7% vs. 42.7% of person-years, chi-square p < .01). Yet, more women worked permanent night schedules than men (15% vs. 11%). White workers worked permanent day shifts most often, while minorities including Black, Hispanic, or American Indian workers were more likely to work day/night schedules. A strong age trend was detected, as older workers were more likely to work permanent day shifts (p < .01) and less likely to work day/evening/night schedules (p < .01) and day/night schedules compared with younger workers ( and Appendix Figure A1, Test for trend: p < .01). The largest proportion of permanent night workers were 60 years of age or older.
Descriptive statistics of working hour characteristics in a year
On average, each subject worked 227 shifts in each year; however, about 10% of the population worked between 280 and 455 shifts per year (). The number of shifts can exceed the number of days due to the presence of split shifts (multiple shifts <6 h per day with a break of more than an hour in between). In this population, it was normal to work one to two weeks without a day off and to work 40% of the Saturdays and Sundays in a year (45 of the total 105 weekend days per year). The average work week was 42 h long. While a worker on average rotated 19 times in a year, there was large variation, with some workers never rotating (e.g., permanent schedules) while others rotated almost every other shift.
Table 2. Descriptive statistics of yearly counts of working hour characteristics across all person-years ≥150 shifts/year in the American Manufacturing Cohort (AMC) 51 plant shift work cohort 2003–2014, USA (N = 98,771 person-years).
Distribution of working hour characteristics by demographics
The majority of the shifts worked by men occurred in daylight hours. However, compared with women, men worked over twice the number of shifts with quick returns (2.5% vs 5.8%), shifts with very quick returns (0.9% vs. 1.9%), shifts that were longer than 13 h (1.8 vs. 6.3%), work weeks longer than 60 h (1.0% vs. 2.2%) and near twice the number of shifts with a rotation (4.7% vs 9.3%) (). Women had higher proportions of shifts that were evening shifts or morning shifts, lower proportions of shifts that occurred on the weekend, and similar proportions of night work compared to men.
Table 3. Percentage of shifts with each working hour characteristic stratified by gender, race, and age in the American Manufacturing Cohort (AMC) 51 plant cohort 2003–2014, USA (N shifts = 22,443,533).
Over 50% of the shifts worked by White workers were day shifts compared to 39.7% to 48% among persons of color (). American Indian/Alaska Native persons and Hispanic/Latino persons had twice the proportion of morning shifts than all other race and ethnicity groups. American Indian/Alaska Native workers also had the highest proportion of shifts with extended hours (40.6% of shifts >12 h, 7.3% of weeks >48 h and 2.6% of weeks over 60 h). Black/African American workers and Multi-racial workers had the highest proportion of shifts with a rotation, but American Indian/Alaska Native workers had the highest proportion of shifts with a flipped rotation.
Shifts worked by older workers had higher proportions of morning shifts, day shifts and shifts with a quick return or a very quick return (). Shifts worked by younger workers had higher proportions of evenings shifts, night shifts, weekend shifts, and shifts with rotations. While younger workers had higher proportions of shifts that were longer than 12 h, older workers had higher proportions of shifts that were longer than 13 h. There were only marginal differences between the proportion of work weeks with extended hours by age.
Distribution of working hour characteristics by annual shift schedule
Distributions of quick returns, shift length, rotations, and amount of time off-work varied by annual shift schedule(). Permanent day workers had the lowest percentage of quick returns, rotations and weekend work, as well as long shifts (≥13 h) and long work (≥40 h/week) despite the inclusion of morning shifts in the definition of permanent day work. Notably, day/evening and day/evening/night schedules had higher percentages of quick and very quick returns to work, as well as shifts 13 h or longer. Additionally, day/evening/night shifts had the highest percentages of rotations (19%) followed by day/night schedules (16.9%). Shift duration, measured by length of hours per shift or week, fluctuated only slightly across annual shift schedules, with the exception of day/night schedules that had more 12-h shifts than any other schedule
Table 4. Percentage of shifts in a year by each working hour characteristic stratified by person-year shift schedules in the American Manufacturing Cohort (AMC) 51 plant cohort 2003–2014, USA (N = 98,771 person-years).
Co-occurrence of working hour characteristics
Shifts were classified into morning (5.5%), day (50.2%), evening (16.2%), and night (28.1%) (). Approximately 54.6% shifts occurred at non-day hours, included a quick return, included long hours (≥13 h), or included a rotation, thus fall into categories that are hypothesized to cause circadian rhythm disruption.
Table 5. Joint distributions (%) of working hour characteristics in American Manufacturing Cohort (AMC), 51 plant shift work cohort 2003–2014, USA (N shifts = 22,443,533).
Overall, the probability of co-occurrence between pairs of working hour characteristics varied substantially. Among day shifts, 23.9% of shifts were 12 h or longer, 6.2% included a rotation, and 3.6% were quick returns. Compared with day shifts, night shifts were more likely to be 12 h or longer (48.2%), however, evening shifts were most often 13 h or longer (13.6%). Night and evening shifts had the highest joint probability with any type of rotation, but nights were twice as likely to include a flipped rotation when compared with day (6.1% vs. 3.2%). Forward and flipped rotations rarely occurred with a quick return (<1%), while 62.1% of backward rotations coincided with a quick return (<11 h between shifts), and 25.1% with a very quick return (<8 h between shifts).
Nearly all instances of quick returns co-occurred with the following conditions: a backwards rotation (11.6%), a shift longer than 12 h (0.76%), the previous shift was longer than 12 h (31.8%), or a combination of all three (50.2%). The remaining 6% of quick returns were attributable to a worker coming in earlier or staying later than normal but not with a large enough difference to cause a rotation or qualify as a long shift (i.e., a shift <12 h long and starting <6 h earlier).
Working shifts longer than 12 h was fairly common in this cohort (30%) and working shifts longer than 13 h was relatively rare (5.6%). Compared with shifts shorter than 12 h, shifts longer than 13 h had a tenfold higher prevalence of co-occurring with a backward rotation (16.3% vs. 1.7%) or co-occurring with a very quick return (12.2% vs. 1.4%). Weekend and weekday shifts had roughly the same joint probability with all working hour characteristics with the exception of shift length; weekend shifts were more often 12 h or longer (40.5% vs. 27.7%). However, there was a negligible difference in the frequency of shifts 13 h or longer (5.8% vs. 5.5%) among weekend and weekday shifts.
Discussion
Our results provide evidence that working hour characteristics hypothesized to cause circadian rhythm disruption have a varying distribution across shift schedules and demographic characteristics. While the literature has primarily focused on the health impacts of night work, rotations, or long work, little attention has been paid to the co-occurrence of working hour characteristics. In this cohort, night and evening shifts were more commonly associated with longer work hours, rotations, and quick returns compared to morning shifts or day shifts. Assuming an additive effect, night and evening shifts with longer work hours, rotations, or quick returns may confer more disruption to workers’ circadian rhythm which may contribute to the diverse adverse health effects seen among night workers.
Furthermore, these working hour characteristics that may cause circadian rhythm disruption were not exclusively found in night or evening shifts. As expected, the day shift had the lowest co-occurrence with quick returns, long work hours or weeks, and rotations. However, working the day shift did not provide absolute protection from potentially disruptive characteristics of working hours since long work hours, as well as quick returns and rotations occurred when workers switched from morning to afternoon shifts (both considered day work). Our work suggests that day workers are exposed to potentially circadian rhythm disruptive shifts despite not working during biological night. This calls into question to de-facto assumption that day workers are an appropriate ‘unexposed’ group for shift work as circadian rhythm disruption may be caused in part by long work hours, rotations, or quick returns.
Of note, circadian rhythm disruption can be caused both directly through a phase shift (such as those caused by a shift rotation) or indirectly through the disruption of the sleep/wake cycle which are important for phase shift, phase resetting, and phase maintenance. Both quick returns to work and long working hours may cause a disruption of the sleep/wake cycle by restricting the number of non-work hours and hours available for sleep. Furthermore, the association between long working hours and adverse human health may be modified by the interaction of long working hours with non-day shift work as long shifts may extend the hours working in a bright area and therefore increase the resulting phase delay for workers on non-day shifts.
We also identified differences in the occurrence of specific patterns of rotation direction (forward, backward, or flipped) and shift type. Flipped rotations more often co-occurred with the 12-h schedule of night/days whereas backward rotations were seen primarily with night shifts on an 8-h schedule. Forward rotations occurred equally among day, night, and evening shifts. The direction of a rotation is important to consider because forward rotations may be less disruptive to the circadian system than backwards or flipped rotations (Bambra et al. Citation2008; Knauth Citation1996; Stevens et al. Citation2011). Anecdotal evidence indicates that workers prefer forward rotation schedules over backwards rotation schedules, likely because forward rotation schedules allow a worker to ‘sleep-in’ rather than wake earlier (Bambra et al. Citation2008; Knauth Citation1996; Stevens et al. Citation2011). Yet, little is known about the impact of forward versus backward rotations on health outcomes. The majority of quick returns and very quick returns are due to long shifts and rotations. However, a small fraction of quick returns is due to working double shifts. While working a double or long shift may be due to an unanticipated workforce shortage, some quick returns may be built into the shift system and represent a possible area of intervention.
An unexpected finding was that the percentage of shift type, quick returns, and rotations varied minimally between weekday or weekend shifts in this population. This suggests that assessing the impact of weekend shifts may not be influenced by shift type, quick returns, or rotations in this population. As expected, working hour characteristics varied by annual shift schedule as well. Most notably, day/night and day/evening/night schedules had more rotations on average than the day/evening or evening/night schedules which might indicate a slower pattern of rotation among the later.
We assessed the average yearly percentage of rotations rather than the speed of rotations (i.e., number of shifts between rotations). We note that regular fast shift rotations which occur every one to three days would correspond to an annual average of >33% rotations in a year. Intermediate shift rotations which occur one a week would correspond to an annual average of roughly 20%. Slow shift rotations occur every 15–30 days or longer would correspond to an annual average between 5% and 10% (Stevens et al. Citation2011). Our results show that, on average, workers rotate about every two weeks, and workers on the day/night or day/evening/night schedules are rotating about every week. Slower patterns of rotations are hypothesized to have a smaller impact on the circadian system since slower speed corresponds with fewer rotations, allowing a worker to acclimatize their circadian system to the new schedule before the next rotation. However, there might be some benefit to a faster rotating system in which the worker switches start times too frequently for the circadian system to entrain itself to the new schedule (Bambra et al. Citation2008). Currently, it is still unclear if a constant state of circadian misalignment associated with fast rotations is better than disrupting it periodically with a slower rotation schedule (Moreno et al. Citation2019). Future work should more directly examine the frequency of rotations (e.g., times of high frequency of rotations followed by low frequency) and their co-occurrence with the characteristics of working hours.
When assessing the differences in yearly shift schedules and working hour characteristics by age, our results indicated a strong age trend. Older workers are more likely to work a permanent day schedule than younger workers. Older workers also had lower proportions of shifts with more than 12 h, but higher proportions of shifts longer than 12 h and similar proportions of long work weeks compared to younger workers. This suggests that older workers may be less likely to work the 12-hr shifts, but when they do, they are more likely to work daily overtime (≥13 h). These patterns may be due to older workers with seniority expressing shift schedule preferences. However, we have no information on the proportion of preferred shifts granted in this population.
We also identified differences in the proportion of workers in permanent or rotational schedules by race and gender. Black/African American workers were the most likely to perform work with rotations, similar to the survey done by the Bureau of Labor Statistics in 2004 (United States Bureau of Labor Citation2004). We also found that American Indian/Alaska Native workers had higher proportions of shifts that were morning shifts, longer than 13 h, and weeks that were longer than 40/48/60 h. These difference by race and ethnicity likely reflects differences in racial composition by job type, due to underlying social factors such as institutionalized racism and higher rates of poverty among racial minorities in America.
Similar to other studies, we found that more women worked permanent night, permanent evening, and permanent day shifts compared to men; with over 37% of women working the permanent day shift (Ghaziri et al. Citation2019; Straif et al. Citation2007; United States Bureau of Labor Citation2004). This pattern might be because women tend to select the day shift more often due to child rearing and meal preparation duties during the evening and night (Ghaziri et al. Citation2019; Mustard et al. Citation2013). Alternatively, a permanent schedule, even permanent nights, affords a predictability that makes arranging child-care easier (Ghaziri et al. Citation2019; Mustard et al. Citation2013). While men worked higher proportions of day shifts than women, they may be at an increased of circadian rhythm disruption due to the higher (nearly double) proportion of their shifts that have a quick return, shifts longer than 13 h, shifts with rotations, and long work weeks.
Identifying differences in working hour exposures is the first step in addressing the causes and consequences of circadian rhythm disruption in vulnerable populations such as young or new workers, aging workers, female workers and minority workers (Cunningham et al. Citation2022). While the field is moving towards refining exposure assessment in studies of shift work, large-scale quantitative studies and in-depth qualitative research are needed to further contextualize and understand the complex and competing motivations for selecting into shift work such as monetary compensation, work–life balance, or schedule autonomy. Furthermore, we encourage researchers to consider the intersectionality of shift systems, sociodemographic characteristics and organizational systems; and the impact they may have on worker health. Future studies should also consider the multiple and overlapping sociodemographic characteristics (e.g., being young and female, being old and identifying as a racial minority) in tandem with shift work exposures; rather than independently evaluating each as a single dimension.
Our study has a few limitations. First, while this study benefits from data captured in an administrative time-registry used to document worker’s hours for payroll calculation, our data is limited to that captured by human resources. We have no information regarding an individual’s chronotype or preference for working days or nights. Individual chronotype is just one of the many unmeasured confounders or effect measure modifiers that may affect both working hours and worker health (Costa Citation2003; Straif et al. Citation2007). Second, the results from this study may have limited generalizability as they describe employees at a single American firm in a single industry (Elser et al. Citation2019). However, there remains considerable diversity in this population. Of the 51 plants in this subset of the AMC only six were unionized under the same union contract, 27 were unionized under local union contracts, and 18 were not unionized and were therefore governed under location-specific shift schedules.
This study employs a novel methodology of assessing the joint probabilities of working hour characteristics. Furthermore, it is the only description of working hour characteristics derived from objective time-registry data in the US and thus represents actual work time in an American manufacturing cohort (Elser et al. Citation2019). This study also updates our understanding of disparities in annual shift schedules by race, gender, and age among American workers, in a rapidly changing workforce.
Conclusion
This research identified patterns in the joint distributions of working hour characteristics that may impact circadian rhythms by shift schedules, race, gender, and age. Night and evening shifts had more frequent shift rotations, quick returns, and longer work hours than day shifts which may confer higher levels of circadian rhythm disruption. Yet, day shifts, traditionally considered to have little negative circadian impact, may still be associated with circadian rhythm disruption as long hours, quick returns and rotations also occurred within day shifts. Younger, male, Hispanic or Black workers were disproportionately working rotational schedules, suggesting potential health disparities may exist. These patterns highlight the need to account for multiple working hour characteristics when assessing health outcomes in relation to working hour exposures.
Author contributions
J.M.F conceived of the study, implemented all analysis, and wrote the manuscript with support from all authors.
E.A.E and S.C provided critical support and mentorship during study design, methods implementation, and analysis of results.
P.T.B aided in data analysis, interpreting the results, and edited the final manuscript.
M.R.C was instrumental in the acquisition of the data and framing of the research question, and reviewed and edited the final manuscript
D.R was instrumental in data preparation, and reviewed and edited the final manuscript.
All authors discussed results and contributed to the final manuscript.
Supplemental Material
Download PDF (112.8 KB)Disclosure statement
Dr. Mark Cullen has received salary support from Alcoa, Inc (Pittsburg, Pennsylvania) through contracts with Stanford University (Stanford, California). The other authors report no conflicts of interest or competing interests to declare.
Data availability statement
naDue to the nature of this research, participants of this study did not agree for their data to be shared publicly, so supporting data is not available.
Supplementary material
Supplemental data for this article can be accessed online at https://doi.org/10.1080/07420528.2023.2168200
Correction Statement
This article has been republished with minor changes. These changes do not impact the academic content of the article.
Additional information
Funding
References
- Akerstedt T, Knutsson A, Alfredsson L, Theorell T. 1984. Shift work and cardiovascular disease. Scand J Work Environ Health. 10:409–14. doi:10.5271/sjweh.2302
- Bambra CL, Whitehead MM, Sowden AJ, Akers J, Petticrew MP. 2008. Shifting schedules. The health effects of reorganizing shift work. Am J Prev Med. 34:5. doi:10.1016/j.amepre.2007.12.023
- Costa G. 2003. Factors influencing health of workers and tolerance to shift work. Theor Issues Ergon Sci. 4:4–263. doi:10.1080/14639220210158880
- Costa G. 2010. Shift work and health: Current problems and preventive actions. Saf Health Work. 1:112–23. doi:10.5491/SHAW.2010.1.2.112
- Cunningham TR, Guerin RJ, Ferguson J, Cavallari J. 2022. Work-related fatigue: A hazard for workers experiencing disproportionate occupational risks. Am J Ind Med. 2021 Aug:1–13. doi:10.1002/ajim.23325
- Cuzick J. 1985. A Wilcoxon-type test for trend. Stat Med. 4:543–47. doi:10.1002/sim.4780040416
- Demetriades S, Pedersini R. 2008. Working time in the EU and other global economie – Industrial relations in the EU and other global economies 2006-2007. Dublin, Ireland: European Foundation for the Improvement of Living and Working Conditions. https://www.eurofound.europa.eu/sites/default/files/ef_files/docs/eiro/tn0804058s/tn0804058s.pdf
- Elser H, Neophytou AM, Tribett E, Galusha D, Modrek S, Noth EM, Meausoone V, Eisen EA, Cantley LF, Cullen MR. 2019. Cohort profile: The American Manufacturing Cohort (AMC) study. Int J Epidemiol. 48:1412–1422J. doi:10.1093/ije/dyz059
- Ferguson JM, Costello S, Neophytou AM, Balmes JR, Bradshaw PT, Cullen MR, Eisen EA. 2019. Night and rotational work exposure within the last 12 months and risk of incident hypertension. Scand J Work Environ Health. 45:256–66. doi:10.5271/sjweh.3788
- Ferguson SA, Dawson D. 2012. 12-h or 8-h shifts? It depends. Sleep Med Rev. 16:519–28. doi:10.1016/j.smrv.2011.11.001
- Gan Y, Yang C, Tong X, Sun H, Cong Y, Yin X, Liqing L, Cao S, Dong X, Gong Y, et al. 2015. Shift work and diabetes mellitus: A meta-analysis of observational studies. Occup Environ Med. 72:72–78. doi:10.1136/oemed-2014-102150
- Garde AH, Hansen J, Kolstad HA, Larsen AD, Pedersen J, Petersen JD, Hansen ÅM. 2018. Payroll data based description of working hours in the Danish regions. Chronobiol Int. 35:795–800. doi:10.1080/07420528.2018.1466797
- Ghaziri ME, Dugan AG, Zhang Y, Gore R, Castro ME. 2019. Sex and gender role differences in occupational exposures and work outcomes among registered nurses in correctional settings. Ann Work Exposures Health. 63:568–82. doi:10.1093/annweh/wxz018
- Gschwandtner T, Gartner J, Aigner W, Miksch S. 2012. A taxonomy of dirty time-oriented data. Int Fed Inf Process. 58–72. doi:10.1007/978-3-642-32498-7
- Hannerz H, Albertsen K, Burr H, Nielsen ML, Garde AH, Larsen AD, Pejtersen JH. 2018a. Long working hours and stroke among employees in the general workforce of Denmark. Scand J Public Health. 46:368–74. doi:10.1177/1403494817748264
- Hannerz H, Larsen AD, Garde AH. 2018b. Long weekly working hours and ischaemic heart disease: A follow-up study among 145 861 randomly selected workers in Denmark. BMJ Open. 8:6. doi:10.1136/bmjopen-2017-019807
- Hansen J. 2017. Night shift work and risk of breast cancer. Curr Environ Health Rep. 4:325–39. doi:10.1007/s40572-017-0155-y
- Härmä M, Ropponen A, Hakola T, Koskinen A, Vanttola P, Puttonen S, Sallinen M, Salo P, Oksanen T, and Pentti J, et al. 2015. Developing register-based measures for assessment of working time patterns for epidemiologic studies. Scandinavian Journal of Work, Environment and Health. 41(3):268–79. doi:10.5271/sjweh.3492
- Hublin C, Partinen M, Koskenvuo K, Silventoinen K, Koskenvuo M, Kaprio J. 2010. Shift-work and cardiovascular disease: A population-based 22-year follow-up study. Eur J Epidemiol. 25:315–23. doi:10.1007/s10654-010-9439-3
- Kivimäki M, Jokela M, Nyberg ST, Singh-Manoux A, Fransson EI, Alfredsson L, Bjorner JB, Borritz M, Burr H, Casini A, et al. 2015a. “Long working hours and risk of coronary heart disease and stroke: A systematic review and meta-analysis of published and unpublished data for 603 838 individuals.” The Lancet. 386:1739–46. doi:10.1016/S0140-6736(15)60295-1
- Kivimäki M, Virtanen M, Kawachi I, Nyberg ST, Alfredsson L, Batty GD, Bjorner JB, Brunner EJ, Burr H, Dragano N, et al. 2015b. “Long working hours, socioeconomic status, and the risk of incident type 2 diabetes: A meta-analysis of published and unpublished data from 222120 individuals.” Lancet Diabetes Endocrinol. 3:27–34. doi:10.1016/S2213-8587(14)70178-0
- Knauth P. 1996. Designing better shift systems. Appl Ergon. 27:39–44. doi:10.1016/0003-6870(95)00044-5
- Knutsson A, Kempe A. 2014. Shift work and diabetes - A systematic review. Chronobiol Int. 31:1146–51. doi:10.3109/07420528.2014.957308
- Lee A, Myung SK, Cho JJ, Jung YJ, Yoon JL, Kim MY. 2017. Night shift work and risk of depression: Meta-analysis of observational studies. J Korean Med Sci. 32:1091–96. doi:10.3346/jkms.2017.32.7.1091
- Lin Y-C, Hsiao T-J, Chen P-C. 2009. Persistent rotating shift-work exposure accelerates development of metabolic syndrome among middle-aged female employees: A five- year follow-up. 0528 (2016 Nov). doi:10.1080/07420520902929029
- Lunde LK, Skare Ø, Mamen A, Sirnes PA, Hans CD, Aass RØ, Goffeng E, Matre D, Nielsen P, Heglum HSA, et al. 2020. Cardiovascular health effects of shift work with long working hours and night shifts: Study protocol for a three-year prospective follow- up study on industrial workers. Int J Environ Res Public Health. 17:2. doi:10.3390/ijerph17020589
- Lu YC, Wang CP, Yu TH, Tsai IT, Hung WC, Lu IC, Hsu CC, Tang WH, Houng JY, Chung FM, et al. 2017. Shift work is associated with metabolic syndrome in male steel workers ‑ the role of resistin and WBC count ‑ related metabolic derangements. Diabetol Metab Syndr. 1–7. doi:10.1186/s13098-017-0283-4
- Manohar S, Thongprayoon C, Cheungpasitporn W, Mao MA, Herrmann SM. 2017. Associations of rotational shift work and night shift status with hypertension: A systematic review and meta-analysis. J Hypertens. 35:1929–37. doi:10.1097/HJH.0000000000001442
- Moreno CRC, Marqueze EC, Sargent C, Wright KP Jr, Ferguson SA, Tucker P, Moreno C. 2019. Working time society consensus statements: Evidence-based effects of shift work on physical and mental health. Ind Health. 57:1–51. doi:10.2486/indhealth.SW-1
- Morikawa Y, Nakagawa H, Miura K, Ishizaki M, Soyama Y, Kido T, Naruse Y, Suwazono Y, Nogawa K. 2005. Shift work and the risk of diabetes mellitus among Japanese male factory workers. Scand J Work Environ Health. 31:179–83. doi:10.5271/sjweh.867
- Mosendane T, Mosendane T, Raal FJ. 2008. Shift work and its effects on the cardiovascular system. Cardiovasc J Afr. 19:210–15.
- Mustard CA, Chambers A, McLeod C, Bielecky A, Smith PM. 2013. Work injury risk by time of day in two population-based data sources. Occup Environ Med. 70:49–56. doi:10.1136/oemed-2012-100920
- Nielsen H, Larsen AD, Dyreborg J, Hansen AM, Pompeii LA, Conway SH, Hansen J, Kolstad HA, Nabe-Nielsen K, Garde AH. 2011. Risk of injury after evening and night work-findings from the Danish working hour database. no. c: 631–39. doi:10.1111/j.1365-2699.2010.02440.x
- Noth EM, Dixon-Ernst C, Liu S, Cantley L, Tessier-Sherman B, Eisen EA, Cullen MR, Hammond SK. 2013. Development of a job-exposure matrix for exposure to total and fine particulate matter in the aluminum industry. J Expo Sci Environ Epidemiol. 24:89–99. doi:10.1038/jes.2013.53
- Pan A, Schernhammer ES, Sun Q, Hu FB, Groop L. 2011. Rotating night shift work and risk of type 2 diabetes: Two prospective cohort studies in women. PLoS Med. 8:12. doi:10.1371/journal.pmed.1001141
- Skogstad M, Mamen A, Lunde LK, Ulvestad B, Matre D, Aass HCD, Øvstebø R, Nielsen P, Samuelsen KN, Skare Ø, et al. 2019. Shift work including night work and long working hours in industrial plants increases the risk of atherosclerosis. Int J Environ Res Public Health. 16:3. doi:10.3390/ijerph16030521
- Stevens RG, Hansen J, Costa G, Rüdiger HW, Kauppinen T, Aronson KJ, Castano-Vinyals G, Davis S, Frings-Dresen MHW, Fritschi L. 2011. Considerations of circadian impact for defining ‘shift work’ in cancer studies: IARC working group report. Occup Environ Med. 68:154–62. doi:10.1136/oem.2009.053512
- Straif K, Baan R, Grosse Y, Secretan BE, Ghissassi FE, Bouvard V. 2007. IARC monographs on the evaluation of carcinogenic risks to humans: Volume 98 painting, firefighting, and shiftwork. Vol. 98. Lyon, France: International Agency for Research on Cancer.
- United States Bureau of Labor. 2004. Occupational outlook handbook: 2004-2005. United States: Jist Publishing.
- Wang F, Zhang L, Zhang Y, Zhang B, He Y, Xie S, Li M, Miao X, Chan EYY, Tang JL, et al. 2014. Meta-analysis on night shift work and risk of metabolic syndrome. Obesity Rev. 15:709–20. doi:10.1111/obr.12194
- Zoonekynd V, Gama J. 2012. Statistics with R. Vincent Zoonekynd and Abo Akademi University.