ABSTRACT
The cognitive ability to self-monitor one’s current performance is important for hospital nurses to maintain safety and health. However, studies on the effects of rotating shift work on self-monitoring ability are insufficient. We examined the differences in self-monitoring accuracy across shifts in a rotating three-shift system among 30 female ward nurses (mean age 28.2 years). Their self-monitoring ability was calculated by subtracting the predicted reaction times of the psychomotor vigilance task performed just before exiting the workplace from the actual reaction times. A mixed-effect model was employed to assess the effects of shift, awake hours, and prior sleep duration on self-monitoring ability. We observed impaired self-monitoring ability in nurses, particularly after the night shift. Although actual performance remained high across all shifts, their self-predictions on reaction times became pessimistic in the night shift, resulting in a difference of approximately−100 msec. The effect of the shift on self-monitoring was obvious even after adjusting for sleep duration and hours awake. Our findings indicate that the misalignment between their working hours and circadian rhythms may affect even professional nurses. Occupational management that emphasizes maintaining circadian rhythms will improve the safety and health of nurses.
Introduction
As health problems follow no set schedule, healthcare services need to be provided 24 hours a day, 7 days a week. Thus, healthcare institutions depend heavily on nurses, particularly those who work the night shift. However, problems linked to work performance and health are more prevalent in night shift workers than in day shift workers owing to the deviation between their work schedule and circadian rhythm (Vedaa et al. Citation2016; Zion and Shochat Citation2018). In nurses, impaired ability to perform tasks and a high risk of accidents are obvious risk factors for safety and health (Caldwell et al. Citation2019; Dorrian et al. Citation2000; Härmä Citation2006). Ensuring the safety and health of nurses and patients also depends on their capacity to accurately self-monitor their performance (Barbe et al. Citation2018). For instance, to utilize compensatory behaviors for subpar performances, we must be aware of our status (Boardman et al. Citation2021). Reportedly, risk-coping and protective behaviors are closely correlated with accurate self-awareness of one’s performance (Slovic Citation1978; Stanton et al. Citation2001).
In general, self-monitoring ability refers to the discrepancies between the actual measured task performance and predicted performance prior to the task or self-rating after the task (Baranski and Pigeau Citation1997; Boardman et al. Citation2021). The majority of earlier research on self-monitoring has centered on the impact of sleep deprivation or restriction on self-monitoring ability (Aidman et al. Citation2019; Baranski and Pigeau Citation1997; Boardman et al. Citation2018; Dorrian et al. Citation2000), and these studies have discovered that sleep deprivation causes a synchronized decline in alertness and performance perception (Boardman et al. Citation2021). The relationship between rotating shifts and self-monitoring ability in actual shift workers has not been investigated, although a few self-monitoring studies exist in which simulated night shifts were performed (Dorrian et al. Citation2003; Morris et al. Citation2017).
Most Japanese ward nurses work rotating shifts, i.e., their reporting times to the workplace change daily (Kida and Takemura Citation2022). Additionally, workers encounter accidents and injuries more often during late night hours (Folkard et al. Citation2005). To maintain the safety and health of shift workers and patients, it is crucial to comprehend the decision-making process and behaviors that underlie self-monitoring.
The subjective cognition among nurses is linked to improved sleep in terms of both amount and quality (Veal et al. Citation2021). Nevertheless, although sleep and fatigue are significant elements that may affect self-monitoring ability, these issues have not been investigated because the majority of earlier studies have focused on the impact of sleep loss in lab settings. We intended to compensate for these variables and assess their effect on self-monitoring ability by incorporating the times spent sleeping and awake in the statistical model and enlisting the actual nurses as participants. Moreover, investigating workers in their actual workplace may yield valuable data that more closely reflect actual conditions than laboratory experiments performed on university students.
We selected the psychomotor vigilance task (PVT) for the performance measurement. The PVT is an extensively used vigilance measurement method and has high reliability, task simplicity, and robustness to the practice effect (Basner and Dinges Citation2011; Roach et al. Citation2006; Wilkinson and Houghton Citation1982). Furthermore, it was anticipated that the reaction time feedback (displayed for each button press during PVT) would provide valuable data for the forecast to be made in the actual millisecond format.
In this study, we examined the connection between circadian rhythm and the self-monitoring ability of hospital ward nurses working under a rotating three-shift system. Circadian misalignment issues have been documented in nurses who work rotating shifts (Chellappa et al. Citation2019; Smith and Eastman Citation2012). Moreover, one’s self-monitoring ability has been reported to be related to the circadian rhythm in a laboratory study that also recorded the body temperature and heart rate data (Morris et al. Citation2017). Each nurse works more day shifts than night or evening shifts in hospitals that use a rotating shift system (Kida and Takemura Citation2022), including the studied hospital. Moreover, nurses may be required to stay awake during the day for various reasons, such as social and family events (Berkman et al. Citation2015; Winwood et al. Citation2006). Hence, we considered a high adaptive load to evening, particularly for night shifts rather than day shifts, based on the assumption that their circadian rhythm might be aligned similarly to those without the night shifts, as stated in an earlier study (Costa et al. Citation1994).
Given the divergence from the circadian cycle, we hypothesized that self-monitoring ability would be most impaired after the night shift. We used shift (i.e., the timing for task operation), awake hours (including working hours), and sleep duration as proxies for indication of deviations from circadian rhythm, fatigue, and recovery, respectively.
Materials and methods
Participants
Thirty female nurses aged 22–46 years (M = 28.2, SD = 5.9) from a single ward (mainly in charge of cardiology and cardiac surgery) of a general hospital in Kanagawa Prefecture, Japan, participated in the study. The participants were oblivious to the research hypothesis, and we assured them that the collected data would be solely used for research and no other purposes, such as personnel evaluation. Their average work experience was 6.4 ± 5.6 years. Nurses in this hospital work under a backward rotating, three-shift system, comprising the day (scheduled work time: 8:15–17:00), evening (15:45–0:30), and night (0:00–8:45) shifts. We asked the participants to specify their chronotype by answering the following question: “Please indicate how active you are in the morning or evening” (1: obviously active in the morning, 2: somewhat active in the morning, 3: somewhat active in the evening, or 4: obviously active in the evening). The targeted ward did not receive any patients with COVID-19, and no infections were noted throughout the study period.
Research ethics
Before beginning the study, we received each participant’s signed, informed consent. Additionally, all participants received a debriefing after the study was completed. All procedures undertaken in this work were in accordance with the ethical standards of the institutional committees on human experimentation and the Helsinki Declaration of 1975, as revised in 2008. The ethics review committee of the National Institute of Occupational Safety and Health in Japan approved all procedures (2020N07).
Data collection
PVT task and performance prediction
From 2020/10/16 to 2020/11/06 (22 days), the participants were asked to perform a PVT after every workday (just before leaving the ward) using a PVT testing device (PVT-192, A.M.I., USA) that was positioned in the break room throughout the study period. The PVT is a widely employed psychomotor task designed to objectively measure one’s state of alertness (Basner and Dinges Citation2011; Wilkinson and Houghton Citation1982). To reduce the task load on the participants, we applied a shorter (5 min) version of the PVT (Roach et al. Citation2006). The lapse threshold was>500 ms, and the PVT’s interstimulus intervals ranged from 2000 to 10 000 ms (Cvirn et al. Citation2019). Before beginning the PVT, the participants were asked to use their smartphones to report their shift type (day, evening, or night shifts), subjective sleepiness, and performance prediction. Subjective sleepiness was conveyed on a 10-point Likert scale that ranged from 1 representing “not sleepy” to 10 representing “sleepy.” (Riegel et al. Citation2013). In the performance prediction section, the participants predicted their overall reaction time (RT, range: 200–1000 ms) of the PVT, which was conducted immediately after answering the questionnaire item “What do you estimate the average PVT reaction time (milliseconds indicated by the red number in the small window) will be? (0.1 seconds = 100 milliseconds).” We provided thorough instructions on the PVT and performance prediction before the commencement of the study via face-to-face orientation and video instructions. The first and second days of data collection for each participant were not included in the analysis. We asked the participants to predict the “average RT” to make predictions simpler, and then, we used the median reaction time (mRT; ms) to calculate the actual PVT performance to optimize the sensitivity while handling numbers in millisecond units (Basner and Dinges Citation2011). To assess the accuracy of performance prediction, we calculated the correctness of the prediction (Δ mRT) by subtracting the predicted RT from the actual performance (mRT) (Boardman et al. Citation2021). A Δ mRT close to 0 indicates a correct prediction, whereas a negative value denotes impaired self-monitoring by a prediction that is too pessimistic.
Sleep length and awake hours
The participants documented their sleep using a graphical self-written sleep daily with a time resolution of 30 minutes. They were asked to keep track of all periods of sleep, including naps. By adding all the sleep times initiated in the previous 24 hours leading up to check-in at work, entries in this sleep diary were used to calculate the sleep duration before each duty. Based on the diary, the awake hours from the nearest wake-up time until each PVT was calculated. These values were employed as a covariate in later analysis.
Statistical analysis
We used a linear mixed model (LMM, or multilevel analysis) with three fixed effects (shift, prior sleep, and prior awake hours) and one random effect (subject) to analyze the effects of shift, sleep, and awake hours on PVT measurements (except the number of lapses) and self-monitoring ability. We selected LMM to consider the unbalanced data structure from a different number of shift assignments. To test the model’s multicollinearity, the shift’s df-adjusted generalized variance inflation factor (GVIF) and GVIF for other variables were below 1.8. On the fitted models, the effect of the shift was tested using Wald’s F tests with Kenward – Roger’s degree of freedom (Luke Citation2017). As a post-hoc test, we performed pairwise comparisons of the estimated marginal means between shifts (Searle et al. Citation1980) using Holm’s method for multiple comparisons if the effect of the shift was significant (Holm Citation1979). Additionally, the impacts of sleep and awake hours on independent variables were assessed using t-tests on the estimated coefficients using Kenward – Roger’s degree of freedom.
Assuming the Poisson distribution of the errors, we utilized an extended linear mixed model with the same design as the LMM to examine the number of lapses. Lastly, as a supplementary study, Pearson’s correlation coefficients computed for pooled data of each shift were used to examine the link between anticipated RT and actual median RT. All data processing and statistical tests were performed in R version 4.1.3 (R Core Team Citation2021), running under Ubuntu 20.04.4. The tests were conducted using functions in the car (Fox and Weisberg Citation2019), effects (Fox Citation2003; Fox and Weisberg Citation2019), emmeans (Lenth et al. Citation2022), lmerTest (Kuznetsova et al. Citation2017), and multcomp (Hothorn et al. Citation2008) packages of R in addition to the basic R functions. A 5% level of significance was set for all tests.
Results
We examined 305 measurements from 30 participants during the study period. Of the participants, 19 (63.3%) identified their chronotype as “3: somewhat active in the evening.” Moreover, six (20%) participants were “4: obviously active in the evening,” four (13.3%) participants were “2: somewhat active in the morning,” and only one (3.3%) participant was “1: obviously active in the morning.” The descriptive data for the lengths of work and sleep for each shift are shown in . During the 22-day trial, the participants had, on average, 10.2 ± 2.0 days of duty, and they were most often assigned to the day shift. Consecutive two-night shifts happened 0–2 times during the study period (M = 1.03, SD = 0.72). There were no three or more consecutive night shifts during the study period. The increased need for overtime work resulted in more working hours on day shifts. A preparative sleep period is required before working in the night shift, as evidenced by the fact that the amount of sleep before the day shift was shorter than that before other shifts.
Table 1. Number of assignments, number of working hours, and preceding sleep durations for the day, evening, and night shifts.
Median RT
compares the median RT values of the different nursing shifts and illustrates no significant effect of shift (F 2, 189.7 = 0.25, p = 0.781). Awake hours and sleep duration displayed no effect on median RT (coefficient = −0.73, 95% CI [−2.39, 0.94], p = 0.397; coefficient = 3.08, 95% CI [−1.15, 7.26], p = 0.155).
Figure 1. Differences in psychomotor vigilance task measures and subjective sleepiness of day, evening, and night shifts. The fitted values for the day, evening, and night shifts are shown as filled circles, triangles, squares, and vertical lines along with their associated 95% confidence intervals. To determine the Δ reaction time, the anticipated RT was subtracted from the median RT.
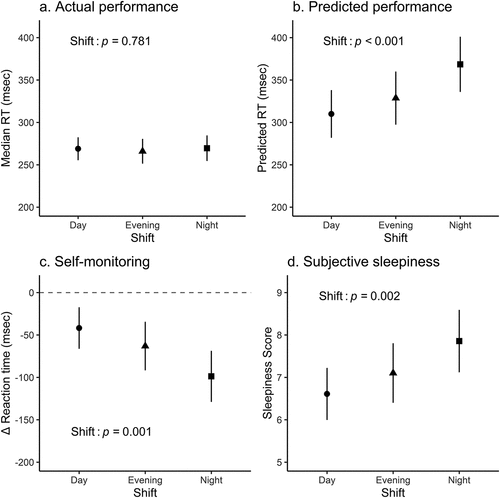
Predicted RT
The shift had a substantial impact on predicted RT (F 2, 191.8 = 8.97, p < 0.001), as shown in . Following a post-hoc analysis, it was determined that there were significant differences between the day and night shifts and the evening and night shifts (t 195.1 = −4.11, p < 0.001; t 188.3 = −2.97, p = 0.007, respectively). In contrast, there was no discernible difference between the predicted RTs for the day and evening shifts (t 192.4 = −1.36, p = 0.176). The most pessimistic predictions were made in the night shift condition. The predicted RT was not affected by the number of awake hours (coefficient = −1, 95% CI [−5.3, 2.93], p = 0.565). However, the sleep duration exerted a significant effect on the predicted RT (coefficient = 12.39, 95% CI [2, 22.64], p = 0.02), i.e., the prediction of RT became 12.4 ms slower for every one hour of prior sleep.
Self-monitoring (Δ mRT)
Self-monitoring ability, as determined by Δ mRT, differed significantly between shifts (F 2, 195.6 = 7.29, p = 0.001, ). Post-hoc analysis indicated significant differences between day and night shifts and between evening and night shifts (t 200.6 = 3.76, p = 0.001; and t 190.3 = 2.49, p = 0.027, respectively). The Δ mRT values between the day and night shift nurses were approximately−50 and−100 ms, respectively. There was no difference between the self-monitoring scores of the day and evening shifts (t 196.7 = 1.45, p = 0.149). Awake hours and sleep duration did not affect the self-monitoring ability (coefficient = 0.01, 95% CI [−4.43, 4.37], p = 0.995 and coefficient = −9.21, 95% CI [−20.02, 1.66], p = 0.101, respectively).
Subjective sleepiness
illustrates that the shift significantly affected subjective sleepiness (F 2, 193.8 = 6.47, p = 0.002). Post-hoc analysis showed significant differences between day and night shifts and between evening and night shifts (198.1 = −3.55, p = 0.001; and t 189.4 = −2.28, p = 0.048, respectively). Conversely, the difference between the day and evening shifts was insignificant (t 194.7 = −1.45, p = 0.148). The nurses in the night shift were the most sleepy (7.9 points), followed by those working in the evening and day shifts (7.0 and 6.7 points, respectively). The duration of awake hours and sleep did not affect subjective sleepiness (coefficient = 0.05, 95% CI [−0.06, 0.15], p = 0.382; coefficient = 0.03, 95% CI [−0.22, 0.28], p = 0.82, respectively).
Number of lapses
The number of lapses (RT > 500 ms) differed significantly between shifts (Chisq 2 = 14.4, p < 0.001; see Supplemental Figure S1). Post-hoc analysis indicated significantly more lapses during the day shift (Z = 3.57, p = 0.001; and Z = 2.86, p = 0.009 for the day – evening and day – night pairs, respectively). The number of lapses did not differ significantly between evening and night shifts (Z = −0.540, p = 0.5894). Although the effect of the shift attained significance, the numbers of lapses in all shifts were less than two (number of lapses: 1.39 [95% confidence interval (95% CI): 0.87, 2.24], 0.65 [0.4, 1.1], and 0.63 [0.36, 1.12] times in the day, evening, and night shifts, respectively). Hence, the difference in raw lapse times between shifts was slight, suggesting that shifts had little effect on lapses. Both awake hours and sleep duration did not exert a significant effect on the number of lapses (coefficient = −0.005, 95% CI [−0.072, 0.058], p = 0.89; and coefficient = 0.03, 95% CI [−0.1, 0.16], p = 0.679, respectively).
Supplemental correlation analysis
displays the outcomes of the supplemental correlation analysis between predicted and actual performance. The predicted RT and actual median RT were positively correlated in the day and evening shifts, whereas no correlation was observed in the night shift data.
Table 2. Relationships between predicted reaction time and actual median reaction time in each shift.
Discussion
In this study, we investigated how rotating shifts affected the self-monitoring capacity of ward nurses. In line with our theory, the ability was affected by rotating shifts and was most impaired in the night shift. Nevertheless, the nurses’ actual performance levels were not affected by shifts and remained high. This is the first study to test the capacity for self-monitoring using RT (ms) of PVT rather than the commonly used Likert scale or visual analogue scale (which requires z-transformations). It was feasible to immediately measure the impact of the shift on one’s capacity for self-monitoring by obtaining forecasts in raw RT (Boardman et al. Citation2021).
Shift work and self-monitoring ability
The nurses’ ability to accurately self-monitor decreased considerably when they worked in the night shift. This finding somewhat supports a previous finding that sleep loss resulted in an increased discrepancy between objective and (pretask) subjective PVT performance (Smith et al. Citation2016). However, another study has reported that sleep loss may lead to an overestimation of PVT performance (Baranski et al. Citation2002). The underestimation detected in this study was not due to impaired PVT performance (mRT) but because of pessimistic predictions of performance, which have rarely been documented in earlier sleep deprivation studies. Most earlier investigations on sleep deprivation have reported relatively accurate self-monitoring (Åkerstedt et al. Citation2014; Dorrian et al. Citation2000, Citation2003; Lee et al. Citation2016; Morris et al. Citation2017). Contrarily, we found that even while their actual performance was unaffected, their self-monitoring was impaired, which could be explained by gloomy forecasts. Thus, sleep deprivation and deviation from one’s circadian rhythm may affect self-monitoring capacity differently.
The nurses in the present study might have made negative forecasts as a result of their work. They are officially mandated to minimize the risk of accidents during work (Winwood et al. Citation2006), and healthcare workers tend to typically steer clear of risk in medical decision-making (Arrieta et al. Citation2017). These tendencies may lead nurses to underestimate their PVT performance even when their actual performance is not impaired. Such pessimistic self-monitoring may even be advantageous, but underestimating is problematic from a production standpoint. For instance, underestimated pretask predictions caused by lack of sleep have been stated in physical activity (Daviaux et al. Citation2014) and on an academic exam (Terlizzese et al. Citation2019). One’s work performance and overall productivity may suffer if these underestimates lead to task avoidance. On the contrary, negative forecasts made during the night shift may not be related to the circadian rhythm, but some differences exist among shifts, which we could not control for.
The homeostatic sleep – wake process (Process S) and the circadian process (Process C) are the two widely used process models of sleep regulation (Borbély Citation1980, Citation1982; Borbély et al. Citation2016). The night shift had the longest awake hours in the current investigation, but the measurements were unaffected. However, shift as a proxy of deviation from circadian rhythms significantly affected the self-monitoring capability. The current outcomes indicate a more potent effect of process C than process S on the self-monitoring ability of shift-working nurses. Nonetheless, in the work setting of the current study, a majority of these factors are standardized and have slight variations; thus, they should be examined further on a broader group of shift workers.
Strengths and limitations
To the best of our knowledge, this is the first study to investigate how shift work affects a person’s self-monitoring ability in an actual work setting. By conducting a field study, we gathered information from actual nurses who worked shift rotations and analyzed its impact. Our statistical model, which considered sleep length and awake hours, provided an in-depth understanding of the self-monitoring ability of professional healthcare workers. Additionally, we collected performance estimates in a manner that can be directly compared with the measured values and avoided the subjective rating scales extensively used previously (Boardman et al. Citation2021).
However, there are several limitations in this study. First, we used shift type as a proxy of deviation for one’s circadian rhythm; however, there is enormous ambiguity in this assumption. As cognitive performance and circadian rhythm are strongly linked (Blatter and Cajochen Citation2007), future research should objectively determine the participants’ circadian rhythm via physiological means, such as melatonin (Benloucif et al. Citation2008). Second, we did not consider the qualitative aspects of sleep, which may be equally crucial as the quantities. Many quality indicators can be used to evaluate sleep (Cudney et al. Citation2022). In addition, the sleep data were only acquired from the participants’ sleep diaries. Objective means to record sleep, such as an actimeter, should be considered in the future, although we assume high compliance of the participants based on their PVT results and interview at the debriefing session. Third, PVT is restricted to the evaluation of vigilance levels. Future research should specifically examine more complicated activities, such as those used in prior studies, even though vigilance is an important underlying aspect for more complex brain functions (Baranski and Pigeau Citation1997; Baranski et al. Citation2002; Sallinen et al. Citation2013). Finally, we did not perform a post-test evaluation of the PVT performance and solely considered the pretask forecasts. Further research on the capacity to self-perceive the performance of a task that has just been completed is warranted.
Future implications
As mentioned in the limitation section, objective measurement of the circadian phase is needed in the future. The subjective sleepiness of the nurses was highest during the night shift (answered at approximately 9:30 in the morning), which is in line with earlier studies on shift work (Folkard et al. Citation2005; Wilson et al. Citation2019). Subjective sleepiness/alertness is greatly impacted by circadian rhythms, particularly those relying on melatonin secretion (Arendt Citation2005; Cajochen et al. Citation2003). An earlier report also indicated the involvement of circadian rhythms in sleepiness and self-monitoring (Dorrian et al. Citation2003). Additionally, considering the effect of consecutive night shifts (Haidarimoghadam et al. Citation2017) may offer more profound insights.
Owing to the fact that shift work was related to impaired self-monitoring ability, occupational management aimed at maintaining circadian rhythms will be advantageous for the safety and health of the nurses. Implementing a forward-rotating shift system can alleviate the adverse effects of shift work (Garde et al. Citation2020; Sallinen and Kecklund Citation2010). Countermeasures premeditated to lessen sleepiness and fatigue during the night will also aid in managing the self-monitoring ability. For instance, taking a nap (Lee et al. Citation2021) or installing workplace lighting with a highly correlated color temperature (Cajochen Citation2007) may be beneficial. Nevertheless, its effect on circadian rhythm should be cautiously considered (Higuchi et al. Citation2021).
The current study’s outcomes may apply to workplaces employing rotating shift systems because they exhibited the negative effects of deviating from biological rhythms. However, our findings were obtained from a single ward in a Japanese hospital, which may necessitate caution in generalizing. For instance, diverse shift systems (van Amelsvoort et al. Citation2004) between hospitals and countries, various responsibilities in the hospital (Oyama et al. Citation2015), napping opportunity (Lee et al. Citation2021), and workers’ age structure (Philip et al. Citation2004) may pose difficulties in generalizing the current results.
Conclusions
We examined the self-monitoring capability of hospital ward nurses working under a rotating three-shift system by performing PVTs and requesting performance predictions. Consequently, we discovered an impaired self-monitoring ability, particularly after night shift duties. An overly pessimistic self-assessment influenced the result, although the actual performance remained high. The outcomes also suggested a more substantial impact of the circadian phase than homeostatic pressure on the self-monitoring ability. Thus, occupational management concentrating on maintaining circadian rhythms will be beneficial for the safety and health of the nurses.
Supplemental Material
Download PDF (64.8 KB)Acknowledgments
The authors would like to thank Atsuko Tamaoki for her help in collecting and analyzing the data. We also wish to express our gratitude to all the personnel and participants for their unwavering commitment to the research during the COVID-19 pandemic. This work was supported by the Industrial Disease Clinical Research Grants from the Ministry of Health, Labour and Welfare, Government of Japan (grant numbers 150903-01, 180902-01) and Grant-in-Aid for Young Scientists (start-up) from the Japan Society for the Promotion of Science (grant number 19K24187).
Disclosure statement
No potential conflict of interest was reported by the authors.
Data availability statement
The data supporting this study’s findings are available from the corresponding author upon reasonable request.
Supplementary material
Supplemental data for this article can be accessed online at https://doi.org/10.1080/07420528.2023.2193270
Additional information
Funding
References
- Aidman E, Jackson SA, Kleitman S. 2019. Effects of sleep deprivation on executive functioning, cognitive abilities, metacognitive confidence, and decision making. Appl Cogn Psychol. 33:188–200. doi:10.1002/acp.3463.
- Åkerstedt T, Anund A, Axelsson J, Kecklund G. 2014. Subjective sleepiness is a sensitive indicator of insufficient sleep and impaired waking function. J Sleep Res. 23:242–254. doi:10.1111/jsr.12158.
- Arendt J. 2005. Melatonin: characteristics, concerns, and prospects. J Biol Rhythms. 20:291–303. doi:10.1177/0748730405277492.
- Arrieta A, García-Prado A, González P, Pinto-Prades JL. 2017. Risk attitudes in medical decisions for others: an experimental approach. Health Econ. 26:97–113. doi:10.1002/hec.3628.
- Baranski JV, Gil V, McLellan TM, Moroz D, Buguet A, Radomski M. 2002. Effects of modafinil on cognitive performance during 40 hr of sleep deprivation in a warm environment. Mil Psychol. 14:23–47. doi:10.1207/S15327876MP1401_02.
- Baranski JV, Pigeau RA. 1997. Self-monitoring cognitive performance during sleep deprivation: effects of modafinil, d-amphetamine and placebo. J Sleep Res. 6:84–91. doi:10.1111/j.1365-2869.1997.00032.x.
- Barbe T, Kimble LP, Rubenstein C. 2018. Subjective cognitive complaints, psychosocial factors and nursing work function in nurses providing direct patient care. J Adv Nurs. 74:914–925. doi:10.1111/jan.13505.
- Basner M, Dinges DF. 2011. Maximizing sensitivity of the psychomotor vigilance test (PVT) to sleep loss. Sleep. 34:581–591. doi:10.1093/sleep/34.5.581.
- Benloucif S, Burgess HJ, Klerman EB, Lewy AJ, Middleton B, Murphy PJ, Parry BL, Revell VL. 2008. Measuring Melatonin in humans. J Clin Sleep Med. 4:66–69. doi:10.5664/jcsm.27083.
- Berkman LF, Liu SY, Hammer L, Moen P, Klein LC, Kelly E, Fay M, Davis K, Durham M, Karuntzos G, et al. 2015. Work–family conflict, cardiometabolic risk, and sleep duration in nursing employees. J Occup Health Psychol. 20:420–433. doi:10.1037/a0039143.
- Blatter K, Cajochen C. 2007. Circadian rhythms in cognitive performance: methodological constraints, protocols, theoretical underpinnings. Physiol Behav. 90:196–208. doi:10.1016/j.physbeh.2006.09.009.
- Boardman JM, Bei B, Mellor A, Anderson C, Sletten TL, Drummond SPA. 2018. The ability to self-monitor cognitive performance during 60 h total sleep deprivation and following 2 nights recovery sleep. J Sleep Res. 27:e12633. doi:10.1111/jsr.12633.
- Boardman JM, Porcheret K, Clark JW, Andrillon T, Cai AWT, Anderson C, Drummond SPA. 2021. The impact of sleep loss on performance monitoring and error-monitoring: a systematic review and meta-analysis. Sleep Med Rev. 58:101490. doi:10.1016/j.smrv.2021.101490.
- Borbély AA. 1980. Sleep: circadian rhythm versus recovery process. In: Lehmann MK, Angst J, editors. Funct states brain their determinants. Amsterdam: Elsevier. p. 151–161.
- Borbély AA. 1982. A two process model of sleep regulation. Hum Neurobiol. 1:195–204.
- Borbély AA, Daan S, Wirz-Justice A, Deboer T. 2016. The two-process model of sleep regulation: a reappraisal. J Sleep Res. 25:131–143. doi:10.1111/jsr.12371.
- Cajochen C. 2007. Alerting effects of light. Sleep Med Rev. 11:453–464. doi:10.1016/j.smrv.2007.07.009.
- Cajochen C, Kräuchi K, Wirz-Justice A. 2003. Role of melatonin in the regulation of human circadian rhythms and sleep. J Neuroendocrinol. 15:432–437. doi:10.1046/j.1365-2826.2003.00989.x.
- Caldwell JA, Caldwell JL, Thompson LA, Lieberman HR. 2019. Fatigue and its management in the workplace. Neurosci Biobehav Rev. 96:272–289. doi:10.1016/j.neubiorev.2018.10.024.
- Chellappa SL, Morris CJ, Fajl S. 2019. Effects of circadian misalignment on cognition in chronic shift workers. Sci Rep. 9:699. doi:10.1038/s41598-018-36762-w.
- Costa G, Ghirlanda G, Tarondi G, Minors D, Waterhouse J. 1994. Evaluation of a rapidly rotating shift system for tolerance of nurses to nightwork. Int Arch Occup Environ Health. 65:305–311. doi:10.1007/BF00405694.
- Cudney LE, Frey BN, McCabe RE, Green SM. 2022. Investigating the relationship between objective measures of sleep and self-report sleep quality in healthy adults: a review. J Clin Sleep Med. 18:927–936. doi:10.5664/jcsm.9708.
- Cvirn MA, Dorrian J, Smith BP, Vincent GE, Jay SM, Roach GD, Sargent C, Larsen B, Aisbett B, Ferguson SA. 2019. The effects of hydration on cognitive performance during a simulated wildfire suppression shift in temperate and hot conditions. Appl Ergon. 77:9–15. doi:10.1016/j.apergo.2018.12.018.
- Daviaux Y, Mignardot J-B, Cornu C, Deschamps T. 2014. Effects of total sleep deprivation on the perception of action capabilities. Exp Brain Res. 232:2243–2253. doi:10.1007/s00221-014-3915-z.
- Dorrian J, Lamond N, Dawson D. 2000. The ability to self-monitor performance when fatigued. J Sleep Res. 9:137–144. doi:10.1046/j.1365-2869.2000.00195.x.
- Dorrian J, Lamond N, Holmes AL, Burgess HJ, Roach GD, Fletcher A, Dawson D. 2003. The ability to self-monitor performance during a week of simulated night shifts. Sleep. 26:871–877. doi:10.1093/sleep/26.7.871.
- Folkard S, Lombardi DA, Tucker PT. 2005. Shiftwork: safety, sleepiness and sleep. Ind Health. 43:20–23. doi:10.2486/indhealth.43.20.
- Fox J. 2003. Effect displays in R for generalised linear models. J Stat Softw. 8:1–27. doi:10.18637/jss.v008.i15.
- Fox J, Weisberg S. 2019. An R companion to applied regression [Internet]. 3rd ed. Thousand Oaks (CA): Sage. https://socialsciences.mcmaster.ca/jfox/Books/Companion/.
- Garde AH, Begtrup L, Bjorvatn B, Bonde JP, Hansen J, Hansen ÅM, Härmä M, Jensen MA, Kecklund G, Kolstad HA, et al. 2020. How to schedule night shift work in order to reduce health and safety risks. Scand J Work Environ Health. 46:557–569.
- Haidarimoghadam R, Kazemi R, Motamedzadeh M, Golmohamadi R, Soltanian A, Zoghipaydar MR. 2017. The effects of consecutive night shifts and shift length on cognitive performance and sleepiness: A field study. International Journal of Occupational Safety and Ergonomics. 23:251–258. doi:10.1080/10803548.2016.1244422.
- Härmä M. 2006. Workhours in relation to work stress, recovery and health. Scand J Work Environ Health. 32:502–514. doi:10.5271/sjweh.1055.
- Higuchi S, Lin Y, Qiu J, Zhang Y, Ohashi M, Lee S, Kitamura S, Yasukouchi A. 2021. Is the use of high correlated color temperature light at night related to delay of sleep timing in university students? A cross-country study in Japan and China. J Physiol Anthropol. 40:7. doi:10.1186/s40101-021-00257-x.
- Holm S. 1979. A simple sequentially rejective multiple test procedure. Scand J Stat. 6:65–70.
- Hothorn T, Bretz F, Westfall P. 2008. Simultaneous inference in general parametric models. Biom J. 50:346–363. doi:10.1002/bimj.200810425.
- Kida R, Takemura Y. 2022. Working conditions and fatigue in Japanese shift work nurses: a cross-sectional survey. Asian Nurs Res. 16:80–86. doi:10.1016/j.anr.2022.03.001.
- Kuznetsova A, Brockhoff PB, Christensen RHB. 2017. lmerTest package: tests in linear mixed effects models. J Stat Softw [Internet]. 82. doi:10.18637/jss.v082.i13.
- Lee ML, Howard ME, Horrey WJ, Liang Y, Anderson C, Shreeve MS, O’brien CS, Czeisler CA. 2016. High risk of near-crash driving events following night-shift work. Proc Natl Acad Sci USA. 113:176–181. doi:10.1073/pnas.1510383112.
- Lee S, Nishi T, Takahashi M, Higuchi S. 2021. Effects of 2-hour nighttime nap on melatonin concentration and alertness during 12-hour simulated night work. Ind Health. 59:393–402. doi:10.2486/indhealth.2020-0245.
- Lenth RV, Buerkner P, Herve M, Love J, Miguez F, Riebl H, Singmann H. 2022. Emmeans: estimated marginal means, aka least-squares means [Internet]. [accessed 2022 Apr 6]. https://CRAN.R-project.org/package=emmeans.
- Luke SG. 2017. Evaluating significance in linear mixed-effects models in R. Behav Res Methods. 49:1494–1502. doi:10.3758/s13428-016-0809-y.
- Morris DM, Pilcher JJ, Mulvihill JB, Vander Wood MA. 2017. Performance awareness: predicting cognitive performance during simulated shiftwork using chronobiological measures. Appl Ergon. 63:9–16. doi:10.1016/j.apergo.2017.03.009.
- Oyama Y, Yonekura Y, Fukahori H. 2015. Nurse health-related quality of life: associations with patient and ward characteristics in Japanese general acute care wards. J Nurs Manag. 23:775–783. doi:10.1111/jonm.12207.
- Philip P, Taillard J, Sagaspe P, Valtat C, Sanchez-Ortuno M, Moore N, Charles A, Bioulac B. 2004. Age, performance and sleep deprivation. J Sleep Res. 13:105–110. doi:10.1111/j.1365-2869.2004.00399.x.
- R Core Team. 2021. R: a language and environment for statistical computing. [Internet]. https://www.r-project.org/.
- Riegel B, Hanlon AL, Zhang X, Fleck D, Sayers SL, Goldberg LR, Weintraub WS. 2013. What is the best measure of daytime sleepiness in adults with heart failure? J Am Assoc Nurse Pract. 25:272–279. doi:10.1111/j.1745-7599.2012.00784.x.
- Roach GD, Dawson D, Lamond N. 2006. Can a shorter psychomotor vigilance task be used as a reasonable substitute for the ten‐minute psychomotor vigilance task? Chronobiol Int. 23:1379–1387. doi:10.1080/07420520601067931.
- Sallinen M, Kecklund G. 2010. Shift work, sleep, and sleepiness — differences between shift schedules and systems. Scand J Work Environ Health. 36:121–133. doi:10.5271/sjweh.2900.
- Sallinen M, Onninen J, Tirkkonen K, Haavisto M-L, Härmä M, Kubo T, Mutanen P, Virkkala J, Tolvanen A, Porkka-Heiskanen T. 2013. Effects of cumulative sleep restriction on self-perceptions while multitasking. J Sleep Res. 22:273–281. doi:10.1111/jsr.12013.
- Searle SR, Speed FM, Milliken GA. 1980. Population marginal means in the linear model: an alternative to least squares means. Am Stat. 34:216–221. doi:10.1080/00031305.1980.10483031.
- Slovic P. 1978. The psychology of protective behavior. J Safety Res. 10:58–68.
- Smith BP, Browne M, Armstrong TA, Ferguson SA. 2016. The accuracy of subjective measures for assessing fatigue related decrements in multi-stressor environments. Saf Sci. 86:238–244. doi:10.1016/j.ssci.2016.03.006.
- Smith MR, Eastman CI. 2012. Shift work: Health, performance and safety problems, traditional countermeasures, and innovative management strategies to reduce circadian misalignment. Nat Sci Sleep. 4:111–132. doi:10.2147/NSS.S10372.
- Stanton NA, Chambers PRG, Piggott J. 2001. Situational awareness and safety. Saf Sci. 39:189–204. doi:10.1016/S0925-7535(01)00010-8.
- Terlizzese T, Hsiou D, Zeter D, Chapagain N, Gao C, Ginty A, Scullin M. 2019. 0101 Experimental sleep restriction, anticipatory stress, and learning organic chemistry. In: Sleep [Internet]. Vol. 42. San Antonio (USA): Oxford academic. p. A41–42. doi:10.1093/sleep/zsz067.100.
- van Amelsvoort LG, Jansen NW, Swaen GM, van den Brandt PA, Kant I, van Amelsvoort LG, van den Brandt PA. 2004. Direction of shift rotation among three-shift workers in relation to psychological health and work-family conflict. Scand J Work Environ Health. 30:149–156. doi:10.5271/sjweh.772.
- Veal BM, Mu CX, Small BJ, Lee S. 2021. Subjective cognitive abilities correlate with poor sleep among day‐shift and night‐shift nurses. J Sleep Res. 30:e13359. doi:10.1111/jsr.13359.
- Vedaa Ø, Harris A, Bjorvatn B, Waage S, Sivertsen B, Tucker P, Pallesen S. 2016. Systematic review of the relationship between quick returns in rotating shift work and health-related outcomes. Ergonomics. 59:1–14. doi:10.1080/00140139.2015.1052020.
- Wilkinson RT, Houghton D. 1982. Field test of arousal: a portable reaction timer with data storage. Hum Factors. 24:487–493. doi:10.1177/001872088202400409.
- Wilson M, Permito R, English A, Albritton S, Coogle C, Van Dongen HPA. 2019. Performance and sleepiness in nurses working 12-h day shifts or night shifts in a community hospital. Accid Anal Prev. 126:43–46. doi:10.1016/j.aap.2017.09.023.
- Winwood PC, Winefield AH, Lushington K. 2006. Work-related fatigue and recovery: the contribution of age, domestic responsibilities and shiftwork. J Adv Nurs. 56:438–449. doi:10.1111/j.1365-2648.2006.04011.x.
- Zion N, Shochat T. 2018. Cognitive functioning of female nurses during the night shift: the impact of age, clock time, time awake and subjective sleepiness. Chronobiol Int. 35:1595–1607. doi:10.1080/07420528.2018.1497642.