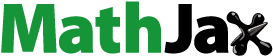
ABSTRACT
Emerging literature has begun to investigate the role of technology in public health. Yet, a minimal amount is understood about whether, how, and why digital games, notably mobile games, might affect mental health, particularly depression. In this work, we examine the effect of location-based mobile gaming on local depression trends. We measure population-level depression using a well-established mechanism from the medical and public health literature, internet search of depression-related terms. We argue that the introduction of Pokémon Go, a mobile game that encourages outdoor physical activity, face-to-face socialization, and exposure to nature, may alleviate non-clinical forms of mild depression for users playing the game. To identify the effect, we employ a difference-in-differences approach to exploit the staggered release of Pokémon Go into 166 regions in 12 English-speaking countries. We empirically document a disproportionate decrease in depression-related search in those regions where users are able to play Pokémon Go. This finding lends credence to anecdotal claims that location-based mobile games may alleviate symptoms of depression of their users, underscoring the mental health opportunities of location-based mobile gaming and creating new opportunities for information systems research.
Introduction
Emerging literature at the intersection of information systems and public health has begun to intensely discuss the effects of digital technologies on public welfare and population health. In such studies, researchers examine a wide variety of questions, such as how search engine query data can be used to detect and monitor influenza epidemics [Citation47], how online health communities provide support for users with chronic diseases [Citation49], and how digital platforms attenuate drunk driving [Citation50]. Research has also begun to examine if digital technologies have had a deleterious effect on public health, including the spread of sexually transmitted diseases [Citation24], drug abuse [Citation67], and obsessive-compulsive disorder [Citation58]. In this study, we focus on an emerging digital artifact, location-based mobile games, and their potential to affect a notorious public health issue, depression, one of the most common mental and emotional disorders characterized by “persistent sadness and a lack of interest or pleasure in previously rewarding or enjoyable activities” [Citation110].
Defined as games played on a mobile device, such that the gaming experience is based on the player’s location [Citation63], location-based mobile games have received significant attention in the academic and practitioner literature [Citation104]. Accounts from scholars and the popular press have identified several effects wrought by such games, some of which would seem to ameliorate the widely-acknowledged adverse health effects of traditional gaming (e.g., self-isolation, addiction [Citation100]). These include promoting outdoor physical activity, face-to-face socialization, and interaction with nature (e.g., [Citation2,Citation21,Citation51]), all of which alleviate obesityFootnote1 and ease social isolation.Footnote2 Recent accounts have even raised the prospect that location-based mobile games (e.g., Geocaching, BotFighters, Ingress) may influence their users’ moods [Citation69]. Grohol [Citation51], for example, performed a content analysis of users’ tweets, showing that users were less anxious when playing location-based mobile games, a finding corroborated by anecdotal reports also made by McCartney [Citation73] and Tateno et al. [Citation107]. Although these studies show suggestive evidence of the benefits of location-based mobile gaming for their users’ well-being, it is crucial to subject such anecdotal claims to rigorous empirical scrutiny. In this study, we explore the prospect of mobile games to alleviate depression by asking: Do location-based mobile games affect local depression trends?
The importance of investigating depression at the population level should also be emphasized given its non-trivial economic and human toll. According to the World Health Organization [Citation110], depression affected 264 million people worldwide in 2020—3.4 percent of the global population. In the United States, 51.5 million adults (nearly one in five of U.S. adults) suffered from mental illness in 2019 [Citation78], and 17.3 million U.S. adults had at least one major depressive episode (63 percent of which experienced severe impairment [Citation77]). Economically, depressive disorders are the sixth-most-costly health condition overall, with U.S. spending about $71 billion annually as of 2016 [Citation35]. It should thus come as no surprise that depression poses significant challenges for individuals, organizations, policymakers, and society at large.
In this study, we examine the effects that location-based mobile games may have on the prevalence of the most common form of depression, that is, emotional distress or non-clinical depression [Citation25]. It is important to separate these types of depression from each other, and our focus on acute non-major depression is deliberate. While it is plausible that the symptoms of more moderate depressive disorders like anhedonia and melancholia might be alleviated through positive behavioral changes such as exercise and socialization, it is implausible that chronic or severe depressive disorders (such as those that lead to self-harm) might be; these disorders often require pharmacological or psychotherapeutic intervention.
To identify any effect of location-based mobile gaming on local depression trends, we exploit the phased rollout of Pokémon Go into 166 regions of 12 English-speaking countries in 2016. In doing so, we take advantage of the game’s staggered release patternFootnote3 using a difference-in-differences approach. This provides several benefits, the primary one being the creation of a natural control group, i.e., those locations where Pokémon Go has not yet been released. Following extensive research in the public and population health literature [Citation6, Citation17, Citation22, Citation47, Citation80, Citation108], we capture local trends in depression using Google Trends data of the Google Misery Index, that is, the prevalence of common depression-related terms (i.e., “depression,” “stress,” “anxiety,” “fatigue”).Footnote4 We then validate the coverage and accuracy of our measures by expanding the set of search terms using Brynjolfsson et al. [Citation18]’s “Crowd-Squared” approach and correlate our measures with administrative data from the U.S. Centers for Disease Control and Prevention (CDC) and the Global Health Data Exchange (GHDx). A battery of falsification tests yields consistent results.
Our empirical analyses indicate that the release of Pokémon Go is associated with a significant short-term decrease in depression-related internet search, suggesting that location-based mobile games may decrease the prevalence of local rates of depression. This finding lends credence to anecdotal reports that the game may alter the mood of location-based mobile gamers [Citation51, Citation107]. Importantly, while this effect exists with depression-related search, we observe no evidence for the correlation between the game release and suicide-related search (i.e., “suicide,” painless suicide,” “how to suicide,” “how to kill yourself”), suggesting that more severe forms of depression are less likely to be alleviated by mobile games.
We further delve into the theoretical mechanisms by which the effect might manifest. Extant research suggests three plausible mechanisms through which depression might be reduced by location-based mobile gaming. First, these games encourage players to be physically active [Citation2]. Medical research has widely documented the mental health benefits stemming from physical activity (e.g., [Citation86]). Intuitively, the physical activity enabled by location-based mobile games should yield similar benefits. Second, location-based mobile games create opportunities to socialize offline through gameplay [Citation104]. Research indicates that when people interact in person and share experiences (e.g., about gaming) more actively, their symptoms of stress and anxiety are subsequently diminished [Citation60]. Third, such games motivate people to go outdoors and interact with nature [Citation69]. Research shows that even innocuous exposure to nature in parks and public greenspaces generally alleviates depression by reducing common risk factors associated with depression, most notably rumination [Citation14], that is, repetitive thought on negative aspects of oneself. Our results show supportive empirical evidence of all three mechanisms. We also observe an opposite effect (i.e., an increase in depression-related search) when a digital game without the aforementioned mechanisms is introduced.
Several contributions stem from this work. First, we study an under-researched digital artifact—location-based mobile gaming—and its potential role in an important public health problem, that is, depression. A growing Information Systems (IS) literature has documented the potentially deleterious effects of digital technologies on public health (e.g., the spread of sexually transmitted diseases [Citation24], drug abuse [Citation67], and addiction [Citation100]). Kyung et al. [Citation59] even find that the proliferation of mobile internet is associated with an increase in suicide rates (as a function of suicide-related search). While much attention has focused on negative externalities of IT, we examine a potential public health benefit of IT (e.g., [Citation47, Citation50, Citation84]), and we provide evidence that location-based mobile games may alleviate depression by facilitating outdoor physical activity, face-to-face socialization, and exposure to nature.
Relatedly, we contribute to the IS literature on gaming [Citation52, Citation55, Citation64, Citation66, Citation83, Citation96, Citation102, Citation109]. While this literature has examined ways to mitigate the adverse effects of digital games [Citation52] and incorporate gaming into the workplace [Citation102], a minimal amount of consideration has been given to the public health implications of gaming and location-based mobile games. This study extends the discussion of the positive effects of gaming from online to offline socialization [Citation39, Citation42]. This work also contributes to the burgeoning IS literature (e.g., [Citation1, Citation24, Citation28, Citation44, Citation49, Citation50, Citation67, Citation68, Citation84]) on the broader societal impacts of IT, by suggesting that search query data may be able to improve the monitoring of population-level depression and estimating its prevalence [Citation47, Citation80].
Second, we contribute to the literature on population health [Citation11, Citation32, Citation70, Citation71, Citation81, Citation95] by proposing the prospect of location-based mobile gaming to help cope with public concerns over depression, at least in its mild forms, by encouraging a healthy lifestyle. Although this finding must be corroborated by medical professionals to identify robust individual effects in the long term, our work highlights a potential, and hitherto unexplored, option for public health officials in the form of complementary approaches to alleviating depression. Notably, location-based mobile games might be leveraged to encourage positive behavioral changes, specifically outdoor physical activity, offline socialization, and nature-connected lifestyles.
Finally, practical implications stem from this work. For policymakers, we raise the potential for location-based mobile games to help ease depression and highlight a real-time and inexpensive means by which population levels of depression might be monitored (i.e., internet search data). Due to the ease of use, relatively low cost, and high accessibility, location-based mobile games may be attractive subsidy targets for policymakers. This is important given the ever-increasing economic burden of healthcare for depression. For game developers, our work shows the benefit of game features which encourage physical activity, offline social interaction, and exposure to nature. With the benefits from these activities, such features may help people pursue healthy lifestyle in a proactive way to cope with depression.
Background and Theory Development
In what follows, we provide an overview of various streams of literature that inform our question. First, we review the IS literature on digital gaming, including an extensive discussion of research on location-based mobile games. Second, we zoom out and discuss the epidemiological effects of IT, with a focus on mobile technology and public health. We then briefly review research on depression, including its causes, symptoms, and treatments. Finally, we integrate the IS and public health literature on non-medical treatments for depression and theorize the relationship between location-based mobile gaming and local depression trends.
Digital Gaming and Location-Based Mobile Games
This line of research has documented the positive and negative effects of digital gaming across contexts and investigated different gamification elements for meaningful engagement of users. Several streams exist.
In the first stream, IS scholars have held the assumption that online and mobile games may have adverse effects on well-being and investigated factors that mitigate or exacerbate harm. Chen et al. [Citation26], for example, discuss the consequences of problematic smartphone game use—including a decrease in work/study performance and an increase in social isolation—as well as mitigation strategies via game design. Similarly, Xu et al. [Citation111] stress the concern of addiction to online gaming among adolescents. They find that mental health issues, like social isolation and a need for escapism, can intensify such addiction, while education and attention-switching activities can mitigate addiction. In contrast, work exists on the positive social effects of digital gaming. Fayard and DeSanctis [Citation39] show that language games allow for constructing collective identity, leading to a sense of “we-ness.” Furthermore, Franceschi et al. [Citation42] show that virtual worlds enable university students to meet and interact with each other, facilitating a sense of group and high engagement in group learning.
Beyond investigations of digital gaming and well-being, scholars have sought to understand how to manage user engagement either in a gaming context or in the workplace. Game developers face an inherent tension: more gaming leads to increased revenues but can also lead to issues of addiction for gamers. Research explores this tension. Huang et al. [Citation55], for instance, show that curtailing player experience to a player’s engagement level can increase gameplay, and therefore revenues. Still, other scholars have shown ways of increasing revenues without excessive gameplay (e.g., out-game markets for virtual currency [Citation52]). Unsurprisingly, managers have sought to bring the benefits of gamification into the workplace as well [Citation66, Citation102]; exploring the effects of game complexity, competition, and collaboration on engagement with, and efficacy of, various workplace activities, for example, employee training [Citation64, Citation93, Citation96]. Essential in this research stream is that gamification extends well beyond hedonic activities, and can drive meaningful engagement in the workplace [Citation102].
Despite the richness of this literature, digital gaming research has devoted conspicuously little attention to either the location-based elements of mobile gaming or how their unique design features might influence the psychological state of their users. Similar to traditional games, location-based mobile games might facilitate social interactions and promote health outcomes [Citation4]. Yet, mobile games, be they location-based or not, are also known to be highly addictive, which leads to health issues. This dilemma brings a natural tension in the literature to bear. Beyond IS, the literature on digital gaming, and specifically location-based games, from other fields has shown mixed effects on health (broadly defined).
On the one hand, Ayers et al. [Citation8] and Faccio and McConnell [Citation38], raise concerns of addiction. There are also concerns of game-led distraction for drivers and pedestrians, and the subsequent risk of injuries and fatalities [Citation38]. On the other hand, Howe et al. [Citation54] and Nigg et al. [Citation79] have identified a significant increase in physical activity (and a decrease in sedentary behaviors) after individuals start to play Pokémon Go. Indeed, Pamuru et al. [Citation83] even find that the footprint made by players of location-based games can revitalize local businesses and restaurants. Yet, despite these differences, the mechanisms by which a change in behavior might manifest remain unclear, as do the technological features of games that might play a role in mental well-being. In what follows, we zoom out of the digital gaming literature and review the broader literature on the relationship between technologyFootnote5—in particular, mobile technologies—and public health.
Mobile Technology and Public Health
Scholars have mostly examined the potential of mobile technologies (e.g., telehealth, self-help/fitness apps) to improve health [Citation4]. Ghose and colleagues [Citation46], for example, conducted an experiment on diabetes patients; finding that the adoption of a mobile health (mHealth) app can reduce blood glucose levels, hospital visits, and medical costs. They also find that the mHealth technology helps track, educate, and make diagnoses, thereby allowing patients to self-regulate their health behavior with less intervention. Yet, the implications of mHealth technology do not only stem from individual use. Instead, they can come from positive externalities because of a network of individuals who use technology together. For example, Aral and Nicolaides [Citation4] find contagion patterns from the use of mobile fitness apps, in which users can view the exercise activities of their peers. This suggests that the social features of mobile apps bring about peer influence and create contagious health benefits.
However, while a large literature has focused on the potential of mobile technologies to improve population physical health, limited research has examined their mental health benefits. Nevertheless, extant research suggests several means by which such technologies may help to alleviate depression. These include, but are not limited to, lower treatment costs [Citation33], personalized care [Citation40], increased continuity and quality of care [Citation87], and creating an environment that yields diminished stigma for patients [Citation91]. The recent investments in smartphone apps by the U.S. Department of Veterans Affairs and the Department of Defense are perfect examples. Both agencies recently invested in apps to help caretakers monitor and treat veterans with post-traumatic stress disorder.Footnote6 In both cases, mobile health interventions helped caretakers reach patients more quickly and the latter to reduce the stigma of visiting a psychiatrist’s office. More broadly, such interventions can be leveraged to enact medical treatments, such as telephone-delivered cognitive behavioral therapy [Citation15], and treatment for depression [Citation95]; as well as non-medical interventions, such as social support forums [Citation10,Citation27], consumer health wearables [Citation85, Citation88], and exercise and fitness apps [Citation4, Citation89, Citation95] (for a review of exemplary studies on technology and health, see Table A-1 in the Online Supplemental Appendix A). These interventions document the health benefits of technology, implying not only the prospect of location-based mobile games in easing depression, but also the need for a deeper understanding of depression—and its causes, symptoms, and treatments—among IS scholars.
Depression: Causes, Symptoms, and Treatments
According to the World Health Organization [Citation110], depression is the most common mental disorder worldwide and a leading cause of disability. Depression results in feelings of sadness and a loss of interest in activities once enjoyed, leading to various emotional and physical problems.Footnote7 Broad consensus indicates depression is a mental disorder that can be influenced by genetic characteristics, changes in hormone levels, certain illnesses, stress, grief, and substance abuse [Citation16, Citation34]. Degrees and symptoms of the depressive condition can vary widely, ranging from no impairment, to mild and moderate symptoms, to severe depression which results in persistent sadness, anxiety, feelings of emptiness, pessimism, irritability, and even self-harm [Citation31]. Medical treatments for depression are diverse, ranging from traditional therapeutic interventions, for example, pharmacological interventionFootnote8 and psychotherapy,Footnote9 to more aggressive treatments, for example, electroconvulsive therapy [Citation65].Footnote10
In addition to medical treatments, non-medical approaches to easing depression exist. Research to date has highlighted multiple behavioral changes which can yield positive impacts. These include: promoting physical activity, encouraging face-to-face socialization, and interacting with nature [Citation14, Citation32, Citation60]. Physical activity has been shown to have many benefits for patients, inasmuch as regular exercise brings positive changes to the brain and promotes feelings of well-being. Exercise also helps release endorphins, chemicals in the brain that inhibit the transmission of pain signals and produce feelings of euphoria [Citation32].Footnote11 Face-to-face socialization reduces feelings of isolation and loneliness, key catalysts of depression [Citation60]. Keeping in regular contact and interacting with friends and family, joining social groups, and volunteering are all practical ways to keep others around oneself [Citation10]. Finally, exposure to nature has been found effective to ease depression. Walking in nature, even parks and green spaces, has been shown to reduce risk factors such as rumination [Citation14]. We next elaborate on the relationship between location-based mobile games and depression.
Location-based Mobile Gaming and Depression
Why might location-based mobile games ease the effects of depression? By integrating public health literature with research on how digital technology affects depression, we posit three means by which such games may impact depression: i) promoting outdoor physical activity, ii) facilitating face-to-face social interaction, and iii) increasing exposure to nature.
First, location-based mobile games encourage people to be physically active through gameplay, as players must physically move to play the game. Medical research indicates a strong relationship between physical activity (or exercise) and depression alleviation (e.g., [Citation82, Citation86]). There are several reasons for this relationship. First, exercise promotes changes in the brain, including neural growth and reduced inflammation, while new activity patterns promote a feeling of calm and well-being [Citation41]. Second, outdoor physical activity energizes people by releasing endorphins, which reduce perceptions of pain and stress [Citation70]. Third, outdoor exercise can distract from daily routines, which helps break the negative thought cycles that feed depression [Citation97]. This is beneficial because negative thoughts and feelings are less likely to manifest during physical activity. Applied to location-based mobile games like Pokémon Go, players who cover greater distances have opportunities to catch more Pokémon creatures, the key objective of the game, thus exercising more. Indeed, Althoff et al. [Citation2] find that Pokémon Go players increased their physical activity by 1,473 steps a day (notably for sedentary populations), showing that location-based games do promote physical activity.
Second, in terms of face-to-face socialization, location-based mobile games create opportunities for people to socialize, build relationships, and enhance their sense of social belonging within the gaming community. Sung et al. [Citation104] argue that games with offline social features encourage face-to-face interaction, communication, and self-disclosure, thereby limiting feelings of social isolation. Furthermore, research notes that the more people actively interact in person (i.e., communicating and sharing experiences), the less stress and anxiety they suffer [Citation60]. Tateno et al. [Citation107] support this by showing that young people who suffer from ineffectively treated social withdrawal leave their houses to play Pokémon Go. Finally, Cao [Citation21] documents the benefits of socialization and finds that children with Asperger syndrome who played Pokémon Go become more sociable.
Third, location-based mobile games motivate people to go outdoors and be exposed to nature. Exposure to nature need not be deep in the wilderness and has generally been shown to have beneficial effects on depression alleviation. For instance, Bratman et al. [Citation14] show that exposure to nature via a walk in public green spaces decreases rumination, a known risk factor for depression. Shanahan et al. [Citation94] show that 30 minutes of walking in an urban green space can reduce the prevalence of depression by up to 7 percent. These findings imply that access to nature in parks and green spaces may be vital to people’s mental well-being, particularly in an increasingly urbanized world.Footnote12 As Pokémon Go players must walk outdoors and explore their surroundings to capture Pokémon creatures, their exposure to nature intuitively increases, notably as the main game features (e.g., PokéStopsFootnote13 and PokéGymsFootnote14) are often located at natural places (e.g., parks).
Importantly, these three mechanisms are not mutually exclusive and may even be mutually enhancing. If a large group explores a natural area with many Pokémon creatures, players in the same area are likely to join them. Such positive externalities increase physical activity, exposure to nature with the neighboring residents, and opportunities to socialize in person. Taken together, we argue that location-based mobile games may help to ease depression by encouraging healthy behavioral changes, specifically physical activity, face-to-face social interaction, and exposure to nature. While unlikely that non-medical interventions, such as exercise and socialization, would yield a material impact on severe forms of depression (e.g., major depressive disorder, bipolar disorder), the public health literature has made explicit reference to their positive effects in the case of lesser conditions of depression.
Data and Methodology
Data
To examine the effect of location-based mobile gaming on local depression trends, we leverage a unique empirical setup, the phased release of Pokémon Go into 166 regions,Footnote15 across 12 English-speaking countries, over 50 weeks between January 1, 2016, and December 12, 2016. This approach offers notable benefits. Pokémon Go is the most popular location-based mobile game in history, covering multiple countries and reaching 2.1 billion people. Furthermore, the game release is temporally and geographically staggered (), which allows us to execute a difference-in-differences estimation.
Table 1. Release of Pokémon Go into 12 English-speaking countries/areas in 2016
To measure the local depression trends, we leverage an increasingly popular approach from the medical and public health literature: internet search of depression-related terms [Citation6, Citation7, Citation23, Citation56, Citation80, Citation108]. This well-established methodology gives us notable benefits, primarily that depression trends can be observed in real time across varying geographies.
For example, Tefft [Citation108] shows a strong relationship between unemployment claims and search trends for “depression” and “anxiety.” Similarly, Ayers et al. [Citation6] show how depression-related search can be used to track economic and psychological distress (PD) in the population. Findings indicate that “PD queries [form] a framework and toolkit for real-time surveillance,” giving clinicians and policymakers an impressive tool to monitor public health. In a systematic review, Nuti et al. [Citation80] surveyed 70 studies between 2009-2013 that use Google Trends data in health care research, concluding that “Google Trends holds potential as a free and easily accessible means to access large population search data to derive meaningful insights about population behavior and its link to health and health care.” A summary of mental health research using search data from the medicine, psychiatry, health economics, and public health literature is in Table A-2 of the Online Supplemental Appendix A.
Considering this information, it is worth noting that while conventional measurements for depression remain popular [Citation11, Citation53, Citation76], they are concerning in our context. Namely, psychometric measurements are likely biased because they rely on self-reporting, which is problematic given the stigmatized nature of depression. Furthermore, health data from public administrations are limited in coverage and timeliness. For example, data from the CDC on mental health are collected and compiled on an annual basis. In contrast, the emerging availability of search query data offers solutions to the challenges posed by low frequent data entry [Citation22, Citation29, Citation47], and has been embraced by information systems, medical, and population health researchers as a method to monitor and measure population-level trends of interest in a timely and unobtrusive fashion [Citation6, Citation18, Citation45, Citation80].Footnote16
To capture the local depression trends, we extract internet search associated with depression at the region-week level from Google Trends. States outside the United States, hereafter also called regions, are defined as provinces or small countries (e.g., Wales in the United Kingdom). Weekly data are more stable than daily data, and using weekly data avoids the problem of missing values [Citation101], as Google Trends does not provide data if there are insufficient searches at a location to break their internally defined privacy threshold. In line with extant work using Google Trends (e.g., [Citation72]), we retrieve the search data following the approach described by Stephens-Davidowitz and Varian [Citation99]. For each region, we pull a time series of depression-related search for the 50 weeks from January 1, 2016, to December 12, 2016. For each search term, we retrieve its time series at one time in the same request, instead of repetitive ones once a week.Footnote17 This ensures that search popularity values are scaled in the same way and are comparable within time series.Footnote18 In addition, we execute the data retrieval for all identified search terms (i.e., “stress,” “anxiety,” “depression,” “fatigue”) on the same day.Footnote19 We standardize (i.e., rescale to a mean of 0 and standard deviation of 1) each time series to ensure meaningful comparisons of relative changes across regions, which allows us to interpret changes in search, that is, flows, as opposed to levels of search, i.e., stocks.
We capture a full 50-week term in 2016 for two reasons. First, as Pokémon Go was initially released on July 6, 2016, we captured a full 6-month period before the release. This allows us to observe pre-treatment depression-related search trends for each region, which is critical for validating the parallel trends assumption of the difference-in-differences estimates [Citation3, Citation12]. Varying the length of the pre-treatment period leads to similar results. Second, Pokémon Go was released to India and Pakistan on December 13, 2016, immediately after the conclusion of the sample.Footnote20 Casting these two countries as untreated (i.e., Pokémon Go was not available) throughout the sample allows us to compare the depression-related search across treated and untreated regions over the entire period [Citation3].
Before discussing our empirical approach, we note several potential limitations which might undermine our ability to capture the effect of Pokémon Go on local depression trends. First, depression-related search words from the Google Misery Index could be used by people who express sentiments versus those who suffer from clinically identified depression. While this is possible, we do not study and measure depression in a clinical context. Instead, we capture the widely accepted symptoms of depression that people discuss in their everyday lives, and that can be inferred from depression-related search trends. Second, it is difficult to tie the search of specific terms to depressed people, i.e., differentiating the general organic search of such terms by the public from searches by the depressed population. Yet, changes in organic search should not be affected by the introduction of Pokémon Go. Within-location change that is correlated with the release of the game is what our estimate captures, that is, change in behavior by people after Pokémon Go is released. Put simply, our empirical strategy does not intend to predict and explain all changes in depression-related search; only the difference in the differences in treated versus non-treated locations after the release of Pokémon Go. Third, it is worth considering whether our estimates capture search from Pokémon players versus from non-Pokémon players. This is a common limitation of studies using macro-level secondary data. Yet, for depressed persons who are not Pokémon players, there should be no change in their conditions of or trends in depression due to the release of Pokémon Go. The reason is that they do not play the game, and they are thus untreated by the game. In other words, there is no a priori reason to believe the changes of their overall search patterns would be different after the game release.
Measurement
Dependent Variable
Following extant work in public and population health (Table A-2 in the Online Supplemental Appendix A), the dependent variable for the main analysis is the Google Trends search popularity of depression-related terms for a region-week (
Table 2. Main variables and data sources
One concern with this approach is that using a single term in any given regression analysis as a proxy for overall depression may introduce measurement error. For example, someone may search “fatigue” immediately after exercising. To mitigate this concern, we construct a composite index to measure depression using a Principal Component Analysis (PCA). PCA is an unsupervised machine learning method used to generate a set of representative components (or factors) that capture most of the variability in a set of variables with an orthogonal transformation [Citation57]. We employ a PCA so that the common variance of all depression-related terms is summarized by the principal component, from which we can approximate the aggregate level of depression-related search more effectively than using the variance of any single search term. For a detailed discussion of the PCA, see the Online Supplemental Appendix B.
Independent Variables
Our main independent variable is a dichotomous indicator PokémonGo. This variable is set to one if Pokémon Go had been introduced to region j at week t; zero otherwise. This indicator captures the change in depression-related search in regions that have received the PokémonGo treatment, as compared to those regions that have yet to receive the PokémonGo treatment as of week t.
In falsification tests, we use Pokémon-related search intensity, as well as local Pokémon Go game features to proxy gameplay, as opposed to the mere availability of the game. In these estimations, we use both a set of search terms (viz. “Pokémon near me,” “where to catch Pokémon,” “PokéStop,” and “PokéGym,” Column 2 in Table C-8 in Online Supplemental Appendix C) as well as the number of PokéStops and PokéGyms (i.e., critical game elements) in U.S. cities (Table C-10A). Using the number of PokéStops and PokéGyms as independent variables enables the validation and replication of the main analysis by varying the measure for Pokémon Go availability. Results remain consistent.
Control Variables
We first account for time-invariant region-level heterogeneity using location fixed effects. For locations outside the United States, this is done using “province” or “region” fixed effects. Inside the United States, they are state fixed effects. These fixed effects capture time-invariant region-level factors (e.g., public health service conditions, mobile internet development) throughout the course of the sample that may be correlated with both the release of Pokémon Go and local search of depression-related terms. For example, demographic (e.g., age or gender composition) or social-economic conditions of a region are relatively stable across weeks and are thus captured by the region fixed effect.Footnote22 Second, we include time fixed effects at the week level to control seasonal or temporal changes in depression-related search. Finally, we include country-specific linear and quadratic time trends to account for time-varying confounders that follow different trajectories within each location.Footnote23 For example, the release of Pokémon Go might be correlated with depression-related seasonal confounds, for example, seasonal affective disorder, stemming from the summertime release of the game in the United States.Footnote24 Using the location-specific time trends as well as time fixed effects should capture any systemic issues stemming from this launch timing.Footnote25 provide data sources, measures, and summary statistics.
Table 3. Variables, measurement, and summary statistics
Empirical Strategy
Our empirical strategy relies on the phased rollout of Pokémon Go into 166 regions across 12 English-speaking countries in 2016 using a difference-in-differences approach. This is performed by comparing the change in depression-related search, before and after the release of the game, to change in depression-related search in regions where the game has yet to be released. This approach has two benefits. First, the phased release creates a natural control group, i.e., those regions yet to receive the PokémonGo treatment. Second, it eliminates concerns of aggregation biases in the analysis of regions, rather than individuals [Citation74]. We model depression-related search (yjt) in region j at week t using the following specification:
indicates whether the game has been activated in region j as of week t (1 if yes, 0 otherwise).
represents the vector of region fixed effects, and
represents the vector of week fixed effects.
is the coefficient of interest
A negative
indicates that the introduction of Pokémon Go is associated with a decrease in depression-related search. The estimator is an OLS with robust standard errors clustered at the country level.
Results
presents the results using searches for “depression,” “stress,” “anxiety,” and “fatigue” as dependent variables. Conditional on region and week fixed effects, we find that the release of Pokémon Go is associated with a decrease in search for “depression,” “stress,” and “fatigue.” However, only the depression term is significant at conventional levels (Row (i)). Row (ii) adds country-specific linear time trends (), and Row (iii) incorporates quadratic time trends (
) to account for the idiosyncratic trend for each country. Results remain consistent, indicating that, on the margin, the release of Pokémon Go decreases the search interest in depression and stress, conditional on fixed effects, as well as location-specific time trends. We observe no evidence of an effect on search intensity related to anxiety or fatigue. However, this may be due to the commonality of the terms. We, therefore, take the described PCA approach to construct a composite depression index using the first principal component for the variance of [depression, stress, anxiety, fatigue]. We then replicate the estimation of EquationEq. 1
(1)
(1) using the composite depression index as the dependent variable.Footnote26 Results in Column 5 of indicate a significant decrease in the aggregate depression-related search after the release of Pokémon Go across all specifications [Citation56].Footnote27
Table 4. Effects of Pokémon Go release on depression-related search
Testing the Parallel Trend Assumption
While the results of the baseline difference-in-differences estimations are compelling, it is important to note that they are subject to several assumptions. The most important one is the absence of heterogeneity in the pre-treatment trends, that is, the difference-in-differences assumes depression-related search before the release of Pokémon Go is parallel across both the treated and untreated regions [Citation3, Citation12]. This assumption may not be satisfied if unobservable environmental factors, which are native to individual regions, result in pre-treatment heterogeneity. For example, if Pokémon Go was released in countries that happened to launch a program aimed at reducing the cost of mental healthcare, there might be additional search and treatment seeking prior to the game release. To rule out this possibility, and further substantiate the claim that the release of Pokémon Go can be treated as an exogenous event conditional upon controls (i.e., fixed effects and location-specific time trends), we execute a variant of Autor’s [Citation5] leads and lags model [Citation19, Citation24]. In doing so, we include pre- and post-treatment dummies to capture the inter-temporal effects of the game release. Formally:
is an indicator equal to 1 if the temporal distance between the game release into region j and the pre-treatment week t is k weeks, while
is an indicator equal to 1 if the temporal distance between the game release into region j and the post-treatment week t is m weeks. Intuitively, this model allows us to capture trends semi-parametrically, and to observe the effects week-by-week before and after the PokémonGo treatment. Consistent with extant literature, we use the week prior to the release of Pokémon Go as the baseline by normalizing the coefficient of
to zero [Citation19]. Weeks later (or earlier) than eight weeks after (or before) Pokémon Go entry are collapsed into a single dummy to increase interpretability. Results are in and .
Table 5. Relative time model of Pokémon Go release on depression-related search
Figure 1. Effects of Pokémon Go release on depression-related search, over time. Note: Figure 1 visualizes the estimates in Column 1 of . Solid line depicts the difference in depression-related search between treated and untreated regions over time, while dash lines the upper and lower bounds of 95 confidence intervals.
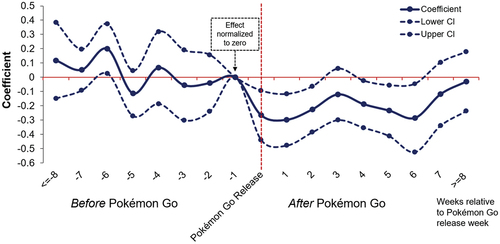
shows small differences in the pre-treatment period, except for a single point estimate six weeks prior to the game release, between treated and untreated regions (i.e., coefficients of , for k < -8 to k < -1, are not significant). Point estimates vacillate intermittently above and below zero, suggesting no systemic heterogeneity in the pre-treatment period. This lends credence to the notion that the estimations do not violate the parallel trends assumption [Citation3]. Moreover, when examining the post-treatment coefficients, we observe a significant decrease (p < 0.05) in depression-related search immediately after the introduction of Pokémon Go, and the negative effect holds for several weeks. Interestingly, the effect appears to dissipate after about six weeks or so, suggesting that it is not permanent. This is not surprising. A short-term reduction in search is consistent with the herd behavior of playing the game [Citation103], which had peak popularity lasting just under two months. While long-term players did persist in playing, most players stopped their play in the months following initiation, thereby resulting in a diminished herding effect. Furthermore, it is consistent with evidence from medicine, inasmuch as the treatment’s benefits will fade when the treatment is removed. Thus, like any short-term treatment, the symptoms of depression returned when the treatment was abandoned. further shows the effect and corroborates the absence of a pre-treatment trend. In sum, these results provide evidence that the introduction of Pokémon Go decreases depression-related search in the short term.
Enhancing Depression Measurement
One empirical challenge lies in the fact that depression-related search may not fully capture the local incidence rate of depression. Prior studies using search query data acknowledge this challenge and propose several solutions to assess the comprehensiveness of the selected terms and the accuracy of predicting offline trends using Google Trends data [Citation18, Citation22, Citation29, Citation45, Citation48, Citation99, Citation101]. Inherent in this challenge are two core issues: proper selection of search terms to capture changes in offline depression (recall that the terms we leverage are based on the widely used Google Misery Index) and a strong correlation between depression-related search and objective, and previously corroborated, measures of local depression.
To ensure that the pool of selected search terms is appropriate, we examine their validity using Brynjolfsson et al. [Citation18]’s “Crowd-Squared” approach. In doing so, we conduct a word association task on Amazon Mechanical Turk wherein we ask participants to “Write 5 terms that come to mind when seeing the word depression (a mental disorder).” Demographic information of the participants is in Table D-1 in the Online Supplemental Appendix D. For any term frequently reported by more than 1 percent of respondents, we collect weekly search data from Google Trends. We then replicate our estimations using these newly identified terms (viz., “unhappy,” “tired,” “sad,” “lonely,” “hopeless,” “dark,” “crying,” “blue”). Results in show that the Pokémon Go release is negatively associated with the newly identified depression-related search (Columns 1-8), corroborating the main effect in . Results are consistent when the PCA-generated composite depression measure is used (Column 9).
Table 6. Effects of Pokémon Go release on depression-related search (search terms generated using “crowd-squared” approach)
Next, we examine if there is a significant difference between the point estimates of the original search terms (i.e., Google Misery Index) and the terms obtained using the Brynjolfsson et al. [Citation18] method. In doing so, we regress different composite depression measures on the introduction of Pokémon Go (Columns 1-3 in ) and compare the coefficients directly. These measures include those from the PCA of the original four terms, the eight newly identified terms, and the comprehensive set of twelve terms. Results show that adding search terms does not significantly affect the estimates, notably when location-specific time trends are considered (Columns 4-6). This suggests that the observed effect of Pokémon Go on local depression using Google Misery terms is robust to different data selection methods.
Table 7. Effects of the Pokémon Go release on depression-related search using different composite depression measures
To resolve the second concern, i.e., the face validity of using internet search trends to measure depression, we correlate depression-related search from Google Trends and objective mental health measures from two well-established administrative datasets: the GHDx and the CDC’s Behavioral Risk Factors Surveillance Systems (BRFSS) survey. We avoid using these data to measure the dependent variable in our main analysis for structural reasons. In short, because these data are collected at the country-year and state-month level, they lack the granularity and sensitivity to capture short-term changes in depression once Pokémon Go was released, as Google Trends can. GHDx captures the number of new mental disorder cases per 100,000 people between 2004 and 2016 for a given country. The BRFSS, which is the United States only, captures each survey response on mental health status, that is, the percentage of days in the past 30 days with poor mental health conditions, and it aggregates the microdata to each month (2004-2016) for each of the 50 States and Washington DC. Although these measures do not exclusively capture depression, they should provide a reasonable facsimile of offline trends in population mental health, of which depression is the most prevalent, over time. To determine the correlation between depression-related search trends and offline mental health trends, we regress the latter on the PCA-generated composite depression index that integrates multiple depression-related search trends.
Results are in Column 1 of and indicate a positive and statistically significant correlation at both the country-year and state-month levels. In addition, we consider the newly-identified search terms using the “Crowd-Squared” approach [Citation18], and the estimates remain consistent (Column 2). In sum, the positive and significant correlation suggests that the depression-related search well captures the local trends in mental health conditions.Footnote28
Table 8. Correlation between depression-related search using Google trends data and depression trends using health administrative data
Robustness and Heterogeneity
While the results hold across several specifications and consistently support the notion that the introduction of Pokémon Go is associated with a decrease in depression-related search, alternative explanations of this relationship exist. We thus extend our empirical analysis to ensure the robustness of the observed effect (see the full list of tests in ). Among these falsification analyses, we consider and rule out the influences of seasonality in Google search trends (Table C-1 in the Online Supplemental Appendix C), organic changes in the general Google search (Table C-2), and different language use preferences across various English-speaking countries (Tables C-3A and C-3B). We add population weighting to account for heteroskedasticity, heterogeneity, and potential sampling issues (Table C-4). We check the possibility of serial correlation using randomly-generated hypothetical release dates of Pokémon Go as placebo treatments (Table C-5). We conduct falsification tests using non-communicable diseases (Table C-6), test the potential reverse causality in the game release (Table C-7), and compare the effect of game availability with that of gameplay (Table C-8). In addition, we explore heterogeneity in the observed effect across countries (Table C-9) and alter our sampling strategy by zooming in and examining the Pokémon Go effect across U.S. cities and states (Tables C-10A and C-10B). These results remain consistent with the main estimators (Column 5 of ).
Table 9. Summary of empirical challenges, tests, and results
Table 10. Effects of Pokémon Go release on searches related to physical activity, face-to-face socialization, and exposure to nature
Table 11. Moderating roles of physical activity, face-to-face socialization, and exposure to nature on the effects of Pokémon Go release on depression-related search
Table 12. Falsification test for alternative mechanisms: Effects of Pokémon sun and moon release on depression-related search
Exploring Underlying Mechanisms
Having established compelling evidence for the decline in depression-related search after the release of Pokémon Go, we explore the underlying mechanisms that might drive the effect. As discussed, location-based mobile games encourage outdoor physical activity, face-to-face socialization, and interaction with nature, all of which may help alleviate the symptoms of depression [Citation14, Citation32, Citation60]. To empirically test these mechanisms, we take three approaches. First, we use search data to capture behavioral changes after the introduction of Pokémon Go. As the relationship between positive behavioral changes (e.g., physical activity) and depression has been well established in the public health literature, it is intuitive that we might be able to explain the changes in behavior by examining changes in the related search (much as we did with depression-related search). Next, as the first approach does not allow us to test each mechanism simultaneously, we proxy each to capture the extent of change in the mechanism (i.e., physical activity, socialization, and exposure to nature). In doing so, we examine how these factors moderate the effect. Note that this is impossible with the first approach due to multicollinearity. Finally, to disentangle confounding mechanisms (e.g., distraction), we use a digital game with similar gameplay as Pokémon Go, albeit without the aforementioned mechanisms.
To execute the first approach, our objective is to find search trends that reflect different behavioral mechanisms by which Pokémon Go might affect depression-related search. In doing so, we use Brynjolfsson et al. [Citation18]’s “Crowd-Squared” approach again to obtain a representative set for each of these behavioral mechanisms. We recruited 500 participants on Amazon Mechanical Turk to associate words with physical activity, offline socialization, and exposure to nature, and list their preferred search terms. After crowdsourcing the terms, we use those which appear in more than 1 percent of responses.Footnote29 We then replicate EquationEquation 1(1)
(1) with the PCA-generated composite indexes of these search terms as the dependent variables. Results are in and indicate a strong relationship between these searches and the release of Pokémon Go. This finding lends credence to the anecdotal claims that location-based mobile games encourage positive behavioral changes.
Second, we examine the channeling impact of behavioral changes on depression-related trends (i.e., whether the behavioral changes moderate the effect of Pokémon Go). In doing so, we use the average obesity rate, population density, and search interest in parks one year before the release of Pokémon Go as proxies for the extent of physical inactivity, offline socialization, and willingness to interact with nature at the country level.Footnote30 We then replicate our estimations. Results are in , indicating that the identified effect of Pokémon Go on depression search is larger at locations where people remain at a relatively low level of physical activity (e.g., high obesity rate), have less opportunity to socialize offline (e.g., low population density), and they are more willing to interact with parks or green spaces. In other words, the game influences depression-related search to a greater extent where behavioral changes are most needed. This further corroborates the channeling from location-based mobile gaming to local depression trends through behavioral mechanisms.
Finally, while the aforementioned approaches provide suggestive evidence of the behavioral mechanisms, it is possible that alternative mechanisms may be at play. For example, Pokémon Go may simply be a short-term distraction like any other game. We, therefore, conduct a falsification test using a video game, Pokémon Sun and Moon, which has gameplay similar to Pokémon Go. The properties of Pokémon Sun and Moon allow us to check whether distraction confounds our explanation and to examine the effects of different game features (Pokémon Sun and Moon not being location-based). Put simply, while Pokémon Sun and Moon has similar gameplay to attract players, it does not encourage outdoor exercise or exposure to nature because there is no location-based component to the game. However, it does retain the game’s social features (e.g., battling with friends, trading Pokémon objects), albeit not face to face. Importantly, Pokémon Sun and Moon had a similar phased release as Pokémon Go did because it was gradually released to North America, Australia, and Europe in later 2016 at different weeks. Results are in and, interestingly, show a significant increase in depression-related search following the release of Pokémon Sun and Moon. In contrast to the Pokémon Go effect, this result provides suggestive evidence that digital games without location-based features encouraging outdoor activities and offline socialization may not yield similar effects on local depression trends.
Discussion
Key Findings
In this study, we investigate the relationship between location-based mobile gaming and local depression trends. Building on burgeoning work in information systems and public health [Citation17, Citation18, Citation29, Citation45, Citation47, Citation48, Citation80, Citation108], we exploit the phased rollout of Pokémon Go into 166 regions of 12 English-speaking countries using internet search data. Results indicate a material decline in the search associated with depression, following the release of Pokémon Go. These findings suggest that location-based mobile gaming may yield short-term relief of an acute and mild form of depression. Empirical evidence also suggests the behavioral mechanisms underpinning this observed effect, notably that Pokémon Go has its observed effects by encouraging outdoor physical activity, face-to-face social interaction, and exposure to nature.
Before discussing implications for theory and practice, we make one important caveat. It would be inappropriate to conclude from this study that location-based mobile games like Pokémon Go can immediately be deployed on a large scale as a quick remedy to depression, particularly severe or clinical depression, or serve as a substitute for medical treatments. Indeed, our study of self-harm related search (Table B-6 in Appendix B) indicates a clear boundary to the effect. Instead, we hope this work serves as a call for future research in three key directions. First, we believe it is important for medical practitioners to assess individual-level effects of location-based mobile gaming, as opposed to the population-level effects we measure. Second, we encourage future research to explore factors that might push the identified short-term effect into a long-term effect. While the planned obsolescence of video games makes the identification of “critical success factors” appealing, ensuring positive behavioral changes that persist across successive generations of games will be critical. Third, we hope this work serves as a call for more in-depth and rigorous research on the complementarities between location-based mobile gaming, as a non-medical approach to depression treatment, and medical interventions alleviating the symptoms of depression in clinical settings. As the medical system is already overloaded, and the coupling of proactive behavioral interventions is often beneficial, we believe that location-based mobile gaming can improve patient welfare by complementing clinical remedies.
Contributions and Implications for Theory and Research
This study makes several contributions with implications for theory and research. First, we examine an emerging technological artifact, location-based mobile games, and their potential effects on public health. While IS literature has identified numerous negative effects of technology on public health (e.g., technostress in the workplace [Citation9], increased local rates of suicide [Citation59]), we observe a positive effect of location-based mobile games on depression-related search. This suggests that this technological artifact may facilitate positive behavioral changes, namely physical activity, face-to-face socialization, and interaction with nature, which provides a nuanced contribution to the nascent IS literature on digital gaming [Citation66]. Indeed, while research in this space has examined both means by which gaming can be less addictive [Citation52] and incorporated into the workplace [Citation102], it has yet to consider the potential of location-based gaming in the context of alleviating depression (at least in its mild forms). Furthermore, our findings enrich the discussion of the social effects of digital gaming, especially for location-based mobile games that facilitate online to offline socialization [Citation39, Citation42]. Finally, this work contributes to the literature on the broader societal impacts of IT on public health (e.g., [Citation24, Citation44, Citation50, Citation67, Citation84]) by exploring the potential mental health benefits from digital gaming.
Second, this work contributes to the emerging literature on public and population health by illustrating the potential for location-based mobile games to combat local depression. While existing research has extensively discussed medical interventions [Citation30, Citation65, Citation71, Citation81], we believe a similar focus on non-medical and behavioral approaches is warranted, as they are often less costly and may be effective in coping with public health problems, such as outdoor activity for obesity. While our findings require corroboration from medical professionals to identify individual-level effects in clinical settings, we believe it is a first step in drawing the attention of scholars and practitioners to technology-based behavioral interventions. The critical takeaway is that location-based mobile gaming may be effective in encouraging people toward positive behavioral changes. Thus, our study responds to the calls of incorporating “Serious Games [Citation75],” that is, video games with an applied positive purpose, for better clinical practices of mental health care.
Finally, this study makes an empirical contribution, particularly by measuring local depression trends using non-traditional data, namely, depression-related internet search using Google Trends. While this approach has been taken by dozens of scholars in the medical field (See Appendix A in the Online Supplemental Material), it has yet to be fully embraced by the IS community. We employ this approach due to a major challenge in mental and public health research, how to assess local depression trends among a population that cannot be reached with surveys [Citation7]. Internet search is a near costless venue in which information seeking carries a dramatically lower stigma than self-reported surveys or in-person interviews. Furthermore, as the most common digital footprint [Citation29], internet search logs offer a solution to the challenge of measurement in depression research and practice, as they allow for a nearly costless general assessment of the public health trends. Indeed, the depression-related search can capture both comprehensive and timely data regarding depression over a wide geographic area, and do so for a protracted period, a notion aligned with extant IS and public health research using search query data [Citation17, Citation18, Citation22, Citation29, Citation45, Citation47, Citation80, Citation108]. In this study, we are further able to examine the coverage and accuracy of our proposed depression-related measures by expanding the set of search terms using the “Crowd-Squared” approach [Citation18], and by correlating depression-related search trends with the regional and national depression data monitored, collected, and compiled by the health administrations (CDC and GHDx). Cross-validations corroborate the fact that depression-related internet search is strongly correlated with objective, and previously corroborated, measures of the local incidence rate of depression.
Contributions and Implications for Practice and Public Policy
Practical and public policy implications stem from this work. First, for mobile game or app developers, we show that the game design encouraging physical activity, offline socialization, and exposure to nature may bring a windfall of public health benefits and potential publicity. This suggestion indicates promising directions for game developers to incorporate these behavioral mechanisms into the design of mobile games or apps. In addition, to the extent that such positive health benefits for users may serve as valuable selling points, notably among parents who have expressed concerns about the potential adverse effects of video games on their children, mobile game and app developers may be able to reap non-trivial economic benefits from the inclusion of above-mentioned features in their games and apps. Last, due to the attractiveness and cost of incorporating these design features, such changes may allow gaming companies to cater to a wider customer base, thereby expanding the accessibility and utilization of their games.
Second, for public health officials, we highlight an attractive option in efforts to track and curtail population-level depression. As discussed, mobile apps are favorable because of their ease of use, low cost, and ubiquitous accessibility, as opposed to more expensive traditional efforts by governments, communities, and physicians. While further corroboration is essential to assess any effect in the long-term, location-based mobile gaming could be a viable means to nudge positive behavioral changes. Furthermore, its effects not only yield benefits for easing depression but also encourage a healthy lifestyle, that is, being physically active, socializing in person in real life, and connecting to nature. Policymakers should consider paying much more attention to technological approaches like location-based mobile games or apps that have the prospects of coping with depression at a modest cost.
Limitations and Suggestions for Future Work
It is important to note that this research is subject to several limitations that create fruitful opportunities for future research. First, we are unable to observe Pokémon Go usage at the individual level. To reduce the effect of this concern, in Table C-8 in Appendix C, we integrate a large set of search trends for Pokémon Go-related terms (e.g., “pokémon near me,” “where to catch pokémon”) to approximate the overall Pokémon Go usage level. However, additional work is needed to ensure the robustness of this individual-level finding.
Second, although we theorize as to how location-based mobile gaming might alleviate depression, we can only observe an aggregate (population level) prevalence of the condition (as opposed to the incidence of the disease at the individual level) through the lens of internet search, and we cannot assess the effect on the intensive or extensive margin (i.e., a general reduction among all people or certain people no longer searching). Additional analyses are essential in a clinical setting, both to corroborate the presence of the observed effect, and to better capture the underlying behavioral mechanisms. For example, future research could consider conducting randomized controlled trials to evaluate the changes in physical activity, face-to-face socialization, and exposure to nature for individual participants who are assigned to play a location-based mobile game. However, this cannot be achieved in our empirical setup (and unit of analysis) due to the practical limitations of macro-level secondary data.
Third, while the depression indicators based on search query data have merits, including timeliness and a greater reach to a broader population who may be suffering from depression, at least compared to surveys, they may be subject to measurement issues. It is possible that the online information search for depression may not fully capture local trends in depression [Citation6, Citation7]. The epidemiology literature acknowledges that construct validity is a common issue in internet data studies [Citation36, Citation62]. However, key validity work (e.g., [Citation17, Citation48]) shows that, in the absence of alternative data sources, search trend data can capture useful and informative changes in the population interest of certain subjects. In our case, alternative fine-grained secondary data sources for contemporary depression are not available. Nevertheless, we mitigate the concern of representation bias by adopting a Principal Component Analysis to improve the measurement and by accounting for temporal and geographical differences in our econometric analysis. Further, we find a strong correlation between depression-related internet search and offline trends monitored by the United States and the global health institutes. Even so, there exists considerable room for improvement.
Finally, it is worth discussing the reliability of Google location data: Google cannot track the location of searches if they are anonymized or obfuscated (e.g., through a VPN). However, this possibility should not materially bias the estimations for three reasons. First, a hidden search occurs but is rather minimal.Footnote31 Second, the regional usage of VPNs should be stable week by week for the duration of the sample and will thus be captured by location fixed effects. Therefore, the difference-in-differences estimates will not be biased unless the change in VPN use over time has been correlated with the phased rollout of Pokémon Go. Finally, even if this occurs, the net effect would be to make our estimates more conservative. In other words, the true effect of Pokémon Go would be larger if the regional usage of VPNs has had been captured because untreated locations (without PokémonGo treatment) will act as a better counterfactual to the treated locations (with PokémonGo treatment).
Conclusion
This work theoretically develops and empirically explores the complex relationship between location-based mobile gaming and local depression trends. Depression is a critical public health and societal problem that is gaining increased attention in medical and population health research. However, these issues have remained largely absent from the IS literature (with some notable exceptions [Citation25, Citation59]). Whereas in earlier research the social and health effects of IT have been relegated to the spread of public health problems [Citation24, Citation67] and the contagious effect of lifestyle decisions [Citation4], we hope that this study ushers in a novel and important area of IS research: the potential of technology toward population mental health. Our study highlights mental health benefits that may be facilitated by technology, and how certain technology, such as location-based mobile games, may encourage healthy behavioral changes, while simultaneously coping with costly societal issues. Future research may include additional phenomena pertaining to digital technology and depression, the underlying behavioral mechanisms, and the design of technological and non-medical approaches to, and the coupling with medical interventions on, other mental health problems besides depression.
We hope this research sparks a new intellectual discourse around technology and mental health in the IS field and hopefully in other literatures. In this way, we hope to gain a deeper understanding of whether, how, and why the design, implementation, and use of digital technologies can gain positive effects on population mental health and bring about other broader societal benefits by helping to promote and facilitate a healthy lifestyle.
Supplemental Material
Download MS Word (374.5 KB)Disclosure statement
No potential conflict of interest was reported by the author(s).
Supplementary Material
Supplemental data for this article can be accessed on the publisher’s website.
Additional information
Notes on contributors
Zhi (Aaron) Cheng
Zhi (Aaron) Cheng ([email protected]; corresponding author) is an Assistant Professor of Information Systems in the Department of Management at the London School of Economics and Political Science (LSE). He joined the faculty at the LSE from Temple University’s Fox School of Business where he received his Ph.D. in Business Administration, with a concentration on Management Information Systems. Dr. Cheng’s research examines the economics of digitization, ICT for social good, digital strategy, and data-informed management. His methodological expertise lies in causal inference and big data analytics, notably on leveraging econometrics, experimentation, and machine learning techniques to understand the causes of digital innovation for the betterment of society. Dr. Cheng’s research appeared in Information Systems Research and Journal of Information Technology, among others, and won several awards.
Brad N. Greenwood
Brad N. Greenwood ([email protected]) is an Associate Professor of Information Systems at George Mason University. He received his Ph.D. in Decision, Operations, and Information Technology, with a minor in Strategic Management, from the University of Maryland, College Park. Previously, he served on the faculty of the University of Minnesota’s Carlson School of Management, Temple University’s Fox School of Business, and the University of Maryland’s Smith School of Business. Dr. Greenwood’s research examines the intended and unintended consequence of innovation, and how access to the resulting information affects welfare at the interface between business, technology, and social issues; notably in the contexts of healthcare and entrepreneurship.
Paul A. Pavlou
Paul A. Pavlou ([email protected]) is the Dean of the C.T. Bauer College of Business at the University of Houston. He is also the Cullen Distinguished Chair of Information Sciences. Dr. Pavlou received his Ph.D. from the University of Southern California. His research has been cited over 75,000 times according to Google Scholar, and he was recognized among the World’s Most Influential Scientific Minds by Thomson Reuters based on an analysis of Highly Cited authors in Economics & Business for 2002-2012. He received about $2,000,000 in grants from funding agencies, such as the National Science Foundation (NSF). His research appeared in MIS Quarterly (MISQ), Information Systems Research (ISR), Journal of Management Information Systems, Journal of Marketing, Journal of Marketing Research, Journal of the Academy of Marketing Science, and Journal of the Association of Information Systems (JAIS), among others. Dr. Pavlou is a Senior Editor at ISR, and earlier at MISQ and JAIS. Dr. Pavlou has won several Best Paper recognitions for his research.
Notes
1. http://www.theguardian.com/commentisfree/2016/jul/13/is-pokemon-go-the-answer-to-obesity-america.
5. There is literature on the negative relationship between general-purpose technology (e.g., email, instant messaging, and social media) and public mental health. Specifically, research has shown that technology may induce feelings of depression in the workplace and people’s personal lives [Citation98]. In the workplace, pervasive technology use may cause technostress, that is, stress or psychosomatic illness caused by working with technology [Citation20]. The reasons for technostress include a digital invasion of one’s work routine, information overload, the uncertainty and complexity of technology, and insecurity due to rapid technological advances [Citation9, Citation92, Citation105, Citation106]. Research indicates such stressors can reduce job satisfaction, innovation, and productivity [Citation90]. Other negatives that come from technology, such as online social networks [Citation95], have been linked to low self-esteem and jealousy [Citation37]. Steers et al. [Citation98] find that Facebook and depressive symptoms go hand-in-hand, mainly driven by social comparison, which may induce a sense of inferiority and fuel depression. Hence, it is unsurprising that scholars have observed a distinct connection between intense technology use and depression, even suicide [Citation59].
8. Pharmacological treatments usually come in the form of antidepressants (e.g., SSRIs), which are designed to regulate levels of dopamine, serotonin, and monoamine oxidase in the body.
9. Psychotherapy (i.e., counseling) can help patients who suffer from depression by resolving problematic behaviors, compulsions, or negative beliefs.
10. While depression is generally treatable, challenges persist in the efficacy of medical treatments for several reasons [Citation30]. First, complex and compounding depression symptoms increase the uncertainty of a treatment’s effect, which may demotivate patients from seeking treatment [Citation43]. Second, treatment may yield adverse side effects. Last, but not least, depression is often attached to a significant social stigma, posing non-trivial challenges to expanding access to necessary mental health care [Citation61].
11. Frequent exercise can stimulate the release of endorphins, which contributes to phenomena like the “runner’s high [Citation13].”
13. PokéStops provide players with items, such as Poké balls, eggs, berries, and potions. These PokéStops can be equipped with items called “lure modules”, which attract wild, and occasionally rare, Pokémon creatures.
14. PokéGyms serve as battle locations for the team-based king of the hill matches.
15. States/regions are the most granular location units designated by Google Trends.
16. We discuss the contentious issues of using search trends and validate our search-based measure using administrative datasets later. The scope of our findings is discussed in the subsection, “Enhancing Depression Measurement.”
17. We thank the anonymous reviewer for the valuable feedback, which allows us to better clarify the details of data collection.
18. For example, in the Google Trends data, an index value of 50 for “depression” on July 5, 2016, and an index value of 30 for “depression” on August 5, 2016, show a relative decrease of 20 because 50 and 30 are the values (that indicate the level of search volume) relative to the same baseline (the peak value), 100 on May 5, 2016.
19. As Google Trends only allows to retrieve no more than five queries in tandem, we retrieve each query one request at a time (but all queries retrieved within one day) and standardize them all together to increase comparability across queries.
20. India is the world’s second largest English-speaking country (See www.bbc.com/news/magazine-20500312), and over 58 percent of the Pakistani population speaks English (the state lingua franca) (See www.worldatlas.com/articles/english-speakers-by-country.html).
21. We drop the search term “pain” because it is more likely a symptom of physical health issues.
22. Note that population migration should also not bias the estimate unless populations are moving internationally in a way that is correlated with the release of Pokémon Go (at the country level; see ), which is unlikely.
23. In unreported analyses, we also use region-specific time trends to allow idiosyncratic trends for each location (e.g., cost changes in mental healthcare over time). Results remain consistent.
24. Note that not all countries activated the game during their summer (); for example, countries in the southern hemisphere (e.g., Australia, New Zealand, South Africa). This is thus a benefit of the global difference-in-differences design.
25. We also consider the impact of seasonality by conducting a falsification test in Table C-1 in the Online Supplemental Appendix C wherein we shift treatment forward a year. No evidence of seasonality bias is found.
26. Tables B-1 to B-4 in the Online Supplemental Appendix B presents the correlation/covariance among search terms and the PCA results. As seen in Table B-4 of the Online Supplemental Appendix, the common variance of depression-related terms (i.e., stress, anxiety, depression, fatigue) are well summarized by the first principal component (A_Comp1) with a cumulative explained variance of 66.51 percent. Figure B-1 of the Online Supplemental material presents a Scree Plot and suggests that the eigenvalue of A_Comp1 is above the widely-used threshold of 1 for the best count of components ([Citation57], pp. 374). This suggests that the A_Comp1 is qualified to serve as a composite index of the depression-related search terms.
27. It is also worth considering other depression-related information seeking behaviors by examining trends in self-harm related search before and after the game release. In doing so, we replicate the PCA using [suicide, painless suicide, how to commit suicide, how to kill yourself], but we find no evidence for this relationship (Table B-6 in the Online Supplemental Appendix B). Although the absence of evidence is not necessarily evidence of absence, this does suggest that the game has not yielded changes to more severe forms of depression. This is an important test. While it is plausible that a local-based mobile game might alleviate less severe forms of depression, the suggestion that playing Pokémon Go might help stave off self-harm is untenable.
28. To further validate the measure of depression-related search trends, we use them to predict local depression (measured by the statistics from CDC and GHDx) and evaluate their out-of-sample predictive performance. Two prediction methods are used. First, we select 50 percent random subsamples as training and testing sets, respectively. We then use the 2004–2014 sample as the training set and the 2015-2016 sample as the testing set. Results are shown in Table B-5 of the Online Supplemental Appendix B. We find the prediction performs well across different datasets, out-of-sample prediction methods, and different sets of predictors (Columns 4-7). In addition to results from , this further corroborates that the depression-related search terms are reliable to model the objective offline depression trends that are well captured within administrative mental health datasets.
29. The new terms for physical activities are “running,” “hiking,” “jogging,” “biking,” “swimming,” “trail,” “yoga,” “outside.” The new terms for offline socialization are “fun,” “restaurant,” “party,” “laughing,” “dinner,” “eating,” “conversation,” “places”. The new terms for exposure to nature are “hiking,”, “trail,” “park,” “animal,” “places,” “forest,” “meditation,” “green,” “environment.” See the Online Supplemental Appendix D for more details.
30. We thank an anonymous reviewer for suggesting this analysis and potential proxies. Obesity rate (percent) is the proportion of the population that is obese. The data are from The World Factbook by Central Intelligence Agency in 2016. Population density (people per sq. kilometers) is from World Bank data in 2016. Park & Green Space is the average search interest of “park” on Google in 2015 (one year before the release of Pokémon Go), which represents the willingness to access public green space.
31. In the year of 2016 which we focus on for the empirical analysis, fewer than 4 percent of Americans use VPNs to access online content (https://bigdata-madesimple.com/vpn-use-and-data-privacy-stats-for-2016/).
References
- Agarwal, R.; Gao, G.; DesRoches, C.; and Jha, A.K. Research commentary—The digital transformation of healthcare: Current status and the road ahead. Information Systems Research, 21, 4 (2010), 796–809.
- Althoff, T.; White, R.W., and Horvitz, E. Influence of Pokémon Go on physical activity: Study and implications. Journal of Medical Internet Research, 18, 12 (2016), e315.
- Angrist, J.D.; and Pischke, J.-S. Mostly Harmless Econometrics: An Empiricist’s Companion. Princeton, NH: Princeton University Press, 2008.
- Aral, S.; and Nicolaides, C. Exercise contagion in a global social network. Nature Communications, 8, (2017), 14753.
- Autor, D.H. Outsourcing at will: The contribution of unjust dismissal doctrine to the growth of employment outsourcing. Journal of Labor Economics, 21, 1 (2003), 1–42.
- Ayers, J.W.; Althouse, B.M.; Allem, J.-P., Childers, M.A.; Zafar, W.; Latkin, C.; Ribisl, K.M.; and Brownstein, J.S. Novel surveillance of psychological distress during the Great Recession. Journal of Affective Disorders, 142, 1 (2012), 323–330.
- Ayers, J.W.; Althouse, B.M.; Allem, J.-P.; Rosenquist, J.N.; and Ford, D.E. Seasonality in seeking mental health information on Google. American Journal of Preventive Medicine, 44, 5 (2013), 520–525.
- Ayers, J.W.; Althouse, B.M.; Leas, E.C.; Dredze, M.; and Allem, J.-P. Internet searches for suicide following the release of 13 reasons why. JAMA Internal Medicine, 177, 10 (2017), 1527–1529.
- Ayyagari, R.; Grover, V.; and Purvis, R. Technostress: Technological antecedents and implications. MIS Quarterly, 35, 4 (2011), 831–858.
- Barney, L.J.; Griffiths, K.M.; and Banfield, M.A. Explicit and implicit information needs of people with depression: A qualitative investigation of problems reported on an online depression support forum. BMC Psychiatry, 11, 1 (2011), 88.
- Beck, A.T.; Ward, C.H.; Mendelson, M.; Mock, J.; and Erbaugh, J. An inventory for measuring depression. Archives of General Psychiatry, 4, 6 (1961), 561–571.
- Bertrand, M.; Duflo, E.; and Mullainathan, S. How much should we trust differences-in-differences estimates? The Quarterly Journal of Economics, 119, 1 (2004), 249–275.
- Boecker, H.; Sprenger, T.; Spilker, M.E., Henriksen, G; Koppenhoefer, M.; Wagner, K.J.; Valet, M.; Berthele, A.; and Tolle, T.R. The runner’s high: Opioidergic mechanisms in the human brain. Cerebral Cortex, 18, 11 (2008), 2523–2531.
- Bratman, G.N.; Hamilton, J.P.; Hahn, K.S.; Daily, G.C.; and Gross, J.J. Nature experience reduces rumination and subgenual prefrontal cortex activation. Proceedings of the National Academy of Sciences, 112, 28 (2015), 8567–8572.
- Brenes, G.A.; Danhauer, S.C.; Lyles, M.F.; Hogan, P.E.; and Miller, M.E. Telephone-delivered cognitive behavioral therapy and telephone-delivered nondirective supportive therapy for rural older adults with generalized anxiety disorder: A randomized clinical trial. JAMA Psychiatry, 72, 10 (2015), 1012–1020.
- Brent, D.; Melhem, N.; Donohoe, M.B.; and Walker, M. The incidence and course of depression in bereaved youth 21 months after the loss of a parent to suicide, accident, or sudden natural death. American Journal of Psychiatry, 166, 7 (2009), 786–794.
- Brownstein, J.S.; Freifeld, C.C.; and Madoff, L.C. Digital disease detection—harnessing the Web for public health surveillance. New England Journal of Medicine, 360, 21 (2009), 2153–2157.
- Brynjolfsson, E.; Geva, T.; and Reichman, S. Crowd-squared: Amplifying the predictive power of search trend data. MIS Quarterly, 40, 4 (2016), 941–962.
- Burtch, G.; Carnahan, S.; and Greenwood, B.N. Can you gig it? An empirical examination of the gig economy and entrepreneurial activity. Management Science, 64, 12 (2018), 5497–5520.
- Califf, C.B.; Sarker, S.; and Sarker, S. The bright and dark sides of technostress: A mixed-methods study involving healthcare IT. MIS Quarterly, 44, 2 (2020), 809–856.
- Cao, R. How “Pokemon Go” Helps Kids with Autism and Asperger’s. CNN, 2016. https://www.cnn.com/2016/08/05/health/pokemon-go-autism-aspergers/index.html. Accessed, November 17, 2021).
- Carneiro, H.A.; and Mylonakis, E. Google trends: A web-based tool for real-time surveillance of disease outbreaks. Clinical Infectious Diseases, 49, 10 (2009), 1557–1564.
- Carr, L.J.; and Dunsiger, S.I. Search query data to monitor interest in behavior change: Application for public health. PLOS ONE, 7, 10 (2012), e48158.
- Chan, J.; and Ghose, A. Internet’s dirty secret: Assessing the impact of online intermediaries on HIV transmission. MIS Quarterly, 38, 4 (2014), 955–976.
- Chau, M.; Li, T.M.H.; Wong, P.W.C.; Xu, J.J.; Yip, P.S.F.; and Chen, H. Finding people with emotional distress in online social media: A design combining machine learning and rule-based classification. MIS Quarterly, 44, 2 (2020), 933–955.
- Chen, C.; Zhang, K.Z.K.; Gong, X.; Lee, M.K.O.; and Wang, Y. Decreasing the problematic use of an information system: An empirical investigation of smartphone game players. Information Systems Journal, 30, 3 (2020), 492–534.
- Chen, L.; Baird, A.; and Straub, D. Fostering participant health knowledge and attitudes: An econometric study of a chronic disease-focused online health community. Journal of Management Information Systems, 36, 1 (2019), 194–229.
- Cheng, Z.; Pang, M.-S.; and Pavlou, P.A. Mitigating traffic congestion: The role of intelligent transportation systems. Information Systems Research, 31, 3 (2020), 653–674.
- Choi, H.; and Varian, H. Predicting the present with Google trends. Economic Record, 88, s1 (2012), 2–9.
- Collins, P.Y.; Patel, V.; Joestl, S.S., March, D.; Insel, T.R.; Daar, A.S.; Bordin, I.A.; Costello, E.J.; Durkin, M.; Fairburn, C.; Glass, R.I.; Hall, W.; Huang, Y.; Hyman, S.E.; Jamison, K; Kaay, S.; Kapur, S., Kleinman, A.; Ogunniyi, A.; Otero-Ojeda, A.; Poo, M.-M.; et al. Grand challenges in global mental health. Nature, 475, 7354 (2011), 27–30.
- Comstock, G.W.; and Helsing, K.J. Symptoms of depression in two communities. Psychological Medicine, 6, 4 (1977), 551–563.
- Cooney, G.; Dwan, K.; and Mead, G. Exercise for depression. JAMA, 311, 23 (2014), 2432–2433.
- Devaraj, S.; and Kohli, R. Information technology payoff in the health-care industry: A longitudinal study. Journal of Management Information Systems, 16, 4 (2000), 41–67.
- Deykin, E.Y.; Levy, J.C.; and Wells, V. Adolescent depression, alcohol and drug abuse. American Journal of Public Health, 77, 2 (1987), 178–182.
- Dieleman, J.L.; Baral, R.; Birger, M., Bui, A.L.; Bulchis, A.; Chapin, A.; Hamavid, H.; Horst, C.; Johnson, E.K.; Joseph, J.; Lavado, R.; Lomsadze, L.; Reynolds, A.; Squires, E.; Campbell, M.; DeCenso, B.; Dicker, D.; Flaxman, A.D.; Gabert, R.; Highfill, T.; Naghavi, M.; et al. US spending on personal health care and public health, 1996-2013. JAMA, 316, 24 (2016), 2627.
- Einav, L.; and Levin, J. Economics in the age of big data. Science, 346, 6210 (2014), 1243089.
- Ellison, N.B.; Steinfield, C.; and Lampe, C. The benefits of Facebook “friends:” Social capital and college students’ use of online social network sites. Journal of Computer-Mediated Communication, 12, 4 (2007), 1143–1168.
- Faccio, M.; and McConnell, J.J. Death by Pokémon Go: The economic and human cost of using apps while driving. Journal of Risk and Insurance, 87, 3 (2020), 815–849.
- Fayard, A.-L.; and DeSanctis, G. Enacting language games: The development of a sense of “we-ness” in online forums. Information Systems Journal, 20, 4 (2010), 383–416.
- Fichman, R.G.; Kohli, R.; and Krishnan, R. The role of information systems in healthcare: Current research and future trends. Information Systems Research, 22, 3 (2011), 419–428.
- Fletcher G.; Balady G.; Blair S.N., Blumenthal, J.; Caspersen, C.; Chaitman, S.; Epstein, E.S.S.; Froelicher, V.F.; Pina, I.L.; Pollock, M.L. Statement on exercise: Benefits and recommendations for physical activity programs for all Americans. Circulation, 94, 4 (1996), 857–862.
- Franceschi, K.; Lee, R.M.; Zanakis, S.H.; and Hinds, D. Engaging group E-learning in virtual worlds. Journal of Management Information Systems, 26, 1 (2009), 73–100.
- Frank, R.G., and McGuire, T.G. Chapter 16 Economics and mental health. In Anthony J. Culyer, and Joseph P. Newhouse. (eds.), Handbook of Health Economics. Elsevier, 2000, pp. 893–954.
- Ganju, K.K.; Pavlou, P.A.; and Banker, R. Does information and communication technology lead to the well-being of nations? A country-level empirical investigation. MIS Quarterly, 40, 2 (2016), 417–430.
- Geva, T.; Oestreicher-Singer, G.; Efron, N.; and Shimshoni, Y. Using forum and search data for sales prediction of high-involvement projects. MIS Quarterly, 41, 1 (2017), 65–82.
- Ghose, A.; Guo, X.; Li, B., and Dang, Y. Empowering patients using smart mobile health 1080 platforms: Evidence from a randomized field experiment. MIS Quarterly, 46 1(2022), 151–192.
- Ginsberg, J.; Mohebbi, M.H.; Patel, R.S.; Brammer, L.; Smolinski, M.S.; and Brilliant, L. Detecting influenza epidemics using search engine query data. Nature, 457, 7232 (2009), 1012.
- Goel, S.; Hofman, J.M.; Lahaie, S.; Pennock, D.M.; and Watts, D.J. Predicting consumer behavior with web search. Proceedings of the National Academy of Sciences, 107, 41 (2010), 17486–17490.
- Goh, J.M.; Gao, G.; and Agarwal, R. The creation of social value: Can an online health community reduce rural-urban health disparities? MIS Quarterly, 40, 1 (2016), 247–263.
- Greenwood, B.N.; and Wattal, S. Show me the way to go home: An empirical investigation of ride-sharing and alcohol related motor vehicle fatalities. MIS Quarterly, 41, 1 (2017), 163–187.
- Grohol, J.M. Pokemon Go Reportedly Helping People’s Mental Health, Depression. Psych Central, 2016. https://psychcentral.com/blog/pokemon-go-reportedly-helping-peoples-mental-health-depression . Accessed, November17, 2021.
- Guo, H.; Hao, L.; Mukhopadhyay, T.; and Sun, D. Selling virtual currency in digital games: Implications for gameplay and social welfare. Information Systems Research, 30, 2 (2019), 430.
- Hamilton, M. A rating scale for depression. Journal of Neurology, Neurosurgery, and Psychiatry, 23, 1 (1960), 56–62.
- Howe, K.B.; Suharlim, C.; Ueda, P.; Howe, D.; Kawachi, I.; and Rimm, E.B. Gotta Catch’em All! Pokémon Go and physical activity among young adults: Difference in differences study. BMJ, 355, (2016), i6270.
- Huang, Y.; Jasin, S.; and Manchanda, P. “Level up”: Leveraging skill and engagement to maximize player game-play in online video games. Information Systems Research, 30, 3 (2019), 927–947.
- Ingraham, C. The Google Misery Index: The times of year we’re most depressed, anxious and stressed. Washington Post, 3, (2014).
- James, G.; Witten, D.; Hastie, T.; and Tibshirani, R. An Introduction to Statistical Learning. Springer, 2013.
- James, T.L.; Lowry, P.B.; Wallace, L.; and Warkentin, M. The effect of belongingness on obsessive-compulsive disorder in the use of online social networks. Journal of Management Information Systems, 34, 2 (2017), 560–596.
- Kyung, N.; Lim, S.; and Lee, B. A Depressing internet tale: Empirical analysis of the internet’s impact on suicide. ICIS 2017 Proceedings, (2017).
- Lakey, B.; and Orehek, E. Relational regulation theory: A new approach to explain the link between perceived social support and mental health. Psychological Review, 118, 3 (2011), 482.
- Lasalvia, A.; Zoppei, S., Van Bortel, T.; Bonetto, C.; Cristofalo, D.; Wahlbeck, K.; Bacle, S.V.; van Audenhove, C.; van Weeghe, J.; Reneses, B.; Germanavicius, A.; Economou, M.; Lanfredi, M.; Ando, S.; Sartorius, N.; Lopez-Ibor, J.J.; Thornicroft, G. Global pattern of experienced and anticipated discrimination reported by people with major depressive disorder: A cross-sectional survey. The Lancet, 381, 9860 (2013), 55–62. New York, U.S.A.
- Lazer, D.; Kennedy, R.; King, G.; and Vespignani, A. The parable of Google flu: Traps in big data analysis. Science, 343, 6176 (2014), 1203–1205.
- Lehmann, L.A. Location-based Mobile Games. Munich, Germany: GRIN Verlag, 2012.
- Li, M.; Jiang, Q.; Tan, C.-H.; and Wei, K.-K. Enhancing user-game engagement through software gaming elements. Journal of Management Information Systems, 30, 4 (2014), 115–149.
- Lisanby, S.H. Electroconvulsive therapy for depression. New England Journal of Medicine, 357, 19 (2007), 1939–1945.
- Liu, D.; Santhanam, R.; and Webster, J. Toward meaningful engagement: A framework for design and research of gamified information systems. MIS Quarterly, 41, 4 (2017), 1011-+.
- Liu, J.; and Bharadwaj, A. Drug abuse and the internet: Evidence from Craigslist. Management Science, 66, 5 (2020), 2040–2049.
- Lucas Jr, H.C.; Agarwal, R.; Clemons, E.K.; and Sawy, O.A.E. Impactful research on transformational information technology: An opportunity to inform new audiences. MIS Quarterly, 37, 2 (2013), 371.
- MacDonald, F. Pokémon Go is reportedly helping people with their depression. ScienceAlert, 2016. http://www.sciencealert.com/pokemon-go-is-reportedly-helping-people-with-their-depression. Accessed, November 17, 2021.
- Mammen, G.; and Faulkner, G. Physical activity and the prevention of depression: A systematic review of prospective studies. American Journal of Preventive Medicine, 45, 5 (2013), 649–657.
- Mann, J.J. The medical management of depression. New England Journal of Medicine, 353, 17 (2005), 1819–1834.
- Marthews, A.; and Tucker, C.E. Government Surveillance and Internet Search Behavior. (2017). Available at SSRN: https://ssrn.com/abstract=2412564
- McCartney, M. Game on for Pokémon Go. BMJ, 354, (2016), i4306.
- Moffitt, R. Causal analysis in population research: An economist’s perspective. Population and Development Review, 29, 3 (2003), 448–458.
- Mohan, D.; Schell, J.; and Angus, D.C. Not thinking clearly? Play a game, seriously! JAMA, 316, 18 (2016), 1867–1868.
- Montgomery, S.A.; and Åsberg, M. A new depression scale designed to be sensitive to change. The British Journal of Psychiatry, 134, 4 (1979), 382–389.
- National Institute of Mental Health. Major Depression. 2017. https://www.nimh.nih.gov/health/statistics/major-depression. Accessed, November 17, 2021.
- National Institute of Mental Health. Mental Illness. 2019. https://www.nimh.nih.gov/health/statistics/mental-illness.
- Nigg, C.R.; Mateo, D.J.; and An, J. Pokémon GO may increase physical activity and decrease sedentary behaviors. American Journal of Public Health, 107, 1 (2016), 37–38.
- Nuti, S.V.; Wayda, B.; Ranasinghe, I.; Wang, S.; Dreyer, R.P.; Chen, S.I.; Murugiah, K. The use of Google trends in health care research: A systematic review. PLOS ONE, 9, 10 (2014), e109583.
- Olfson, M.; Marcus, S.C.; Druss, B.; Elinson, L.; Tanielian, T.; and Pincus, H.A. National trends in the outpatient treatment of depression. JAMA, 287, 2 (2002), 203–209.
- Paluska, S.A.; and Schwenk, T.L. Physical activity and mental health. Sports Medicine, 29, 3 (2000), 167–180.
- Pamuru, V.; Khern-am-nuai, W.; and Kannan, K. The impact of an augmented-reality game on local businesses: A study of Pokémon Go on restaurants. Information Systems Research, 32, 3 (2021), 950–966.
- Park, J.; Pang, M.-S.; Kim, J.; and Lee, B. The deterrent effect of ride-sharing on sexual assault and investigation of situational contingencies. Information Systems Research, 32, 2 (2021), 497–516.
- Patel, M.S.; Asch, D.A.; and Volpp, K.G. Wearable devices as facilitators, not drivers, of health behavior change. JAMA, 313, 5 (2015), 459–460.
- Penedo, F.J.; and Dahn, J.R. Exercise and well-being: A review of mental and physical health benefits associated with physical activity. Current Opinion in Psychiatry, 18, 2 (2005), 189–193.
- Pinsonneault, A.; Addas, S.; Qian, C.; Dakshinamoorthy, V.; and Tamblyn, R. Integrated health information technology and the quality of patient care: A natural experiment. Journal of Management Information Systems, 34, 2 (2017), 457–486.
- Piwek, L.; Ellis, D.A.; Andrews, S.; and Joinson, A. The rise of consumer health wearables: Promises and barriers. PLOS Medicine, 13, 2 (February 2016), e1001953.
- Powell, A.C.; Landman, A.B.; and Bates, D.W. In search of a few good apps. JAMA, 311, 18 (2014), 1851–1852.
- Ragu-Nathan, T.S.; Tarafdar, M.; Ragu-Nathan, B.S.; and Tu, Q. The consequences of technostress for end users in organizations: Conceptual development and empirical validation. Information Systems Research, 19, 4 (2008), 417–433.
- Richards, D.; and Richardson, T. Computer-based psychological treatments for depression: A systematic review and meta-analysis. Clinical Psychology Review, 32, 4 (June 2012), 329–342.
- Salo, M.; Pirkkalainen, H.; Chua, C.E.H.; and Koskelainen, T. Formation and mitigation of technostress in the personal use of IT. MIS Quarterly, 46, (2022).
- Santhanam, R.; Liu, D.; and Shen, W.-C.M. Gamification of technology-mediated training: Not all competitions are the same. Information Systems Research, 27, 2 (2016), 453–465.
- Shanahan, D.F.; Bush, R.; Gaston, K.J.; Lin, B.B.; Dean, J.; Barber, E.; Fuller, R.; et al. Health benefits from nature experiences depend on dose. Scientific Reports, 6, 1 (2016), 1–10.
- Shen, N.; Levitan, M.-J.; Johnson, A.; Bender, J.L.; Hamilton-Page, M.; Jadad, A.R.; Wiljer, D. Finding a depression app: A review and content analysis of the depression app marketplace. JMIR mHealth and uHealth, 3, 1 (2015), e16.
- Silic, M.; and Lowry, P.B. Using design-science based gamification to improve organizational security training and compliance. Journal of Management Information Systems, 37, 1 (2020), 129–161.
- Stathopoulou, G.; Powers, M.B.; Berry, A.C.; Smits, J.A.J.; and Otto, M.W. Exercise interventions for mental health: A quantitative and qualitative review. Clinical Psychology: Science and Practice, 13, 2 (2006), 179–193.
- Steers, M.-L.N.; Wickham, R.E.; and Acitelli, L.K. Seeing everyone else’s highlight reels: How Facebook usage is linked to depressive symptoms. Journal of Social and Clinical Psychology, 33, 8 (2014), 701–731.
- Stephens-Davidowitz, S.; and Varian, H. A Hands-on guide to Google data. Mountain View, CA, 2014.
- Stetina, B.U.; Kothgassner, O.D.; Lehenbauer, M.; and Kryspin-Exner, I. Beyond the fascination of online-games: Probing addictive behavior and depression in the world of online-gaming. Computers in Human Behavior, 27, 1 (2011), 473–479.
- Stocking, G., and Matsa, K.E. Using Google Trends Data for Research? Here Are 6 Questions to Ask. Pew Research Center, 2017. https://medium.com/@pewresearch/using-google-trendsdata-for-research-here-are-6-questions-to-ask-a7097f5fb526. Accessed, March 01, 2022.
- Suh, A.; Cheung, C.M.K.; Ahuja, M.; and Wagner, C. Gamification in the workplace: The central role of the aesthetic experience. Journal of Management Information Systems, 34, 1 (2017), 268–305.
- Sun, H. A Longitudinal study of herd behavior in the adoption and continued use of technology. MIS Quarterly, (2013), 1013–1041.
- Sung, H.; Sigerson, L.; and Cheng, C. Social capital accumulation in location-based mobile game playing: A multiple-process approach. Cyberpsychology, Behavior, and Social Networking, 20, 8 (2017), 486–493.
- Tarafdar, M.; Tu, Q.; Ragu-Nathan, B.S.; and Ragu-Nathan, T.S. The impact of technostress on role stress and productivity. Journal of Management Information Systems, 24, 1 (2007), 301–328.
- Tarafdar, M.; Tu, Q.; Ragu-Nathan, T.S.; and Ragu-Nathan, B.S. Crossing to the dark side: examining creators, outcomes, and inhibitors of technostress. Communications of the ACM, 54, 9 (2011), 113–120.
- Tateno, M.; Skokauskas, N.; Kato, T.A.; Teo, A.R.; and Guerrero, A.P. New game software (Pokémon Go) may help youth with severe social withdrawal, Hikikomori. Psychiatry Research, 246, (2016), 848.
- Tefft, N. Insights on unemployment, unemployment insurance, and mental health. Journal of Health Economics, 30, 2 (2011), 258–264.
- Wang, L.; Gunasti, K.; Shankar, R.; Pancras, J.; and Gopal, R. Impact of gamification on perceptions of word-of-mouth contributors and actions of word-of-mouth consumers. MIS Quarterly, 44, 4 (2020), 1987–2011.
- World Health Organization. Depression. 2020. https://www.who.int/news-room/fact-sheets/detail/depression . Accessed, November 17, 2021.
- Xu, Z.; Turel, O.; and Yuan, Y. Online game addiction among adolescents—Motivation and prevention factors. European Journal of Information Systems, 21, 3 (2012), 321–340.