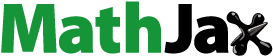
ABSTRACT
Markets in which similar goods of different qualities are sold suffer from information asymmetries and their negative consequences. Dealers have established themselves, and mediate these markets through their use of quality signals. While these signals help to mitigate information asymmetries, these markets still function well below their optimum: a large share of goods sold are overpriced, and most of the benefits are reaped by intermediaries. In this paper we build on prior research that proposes the use of blockchain as an enabler for trusted, decentralized asset documentation. Applying a socio-technical lens, we describe how blockchain-enabled multi-party certification affords dealers the action potential to send signals that are more closely correlated to the unobservable quality of the underlying good (i.e., signals with a higher fit) than the signals they send today. We then both theorize and experimentally explore the market effects of the two types of signals. Using data from a laboratory market experiment with 210 participants, we find empirical evidence that multi-party certification affords dealers the action potential to send signals of significantly higher fit than those sent by intermediaries alone, leading to a reduction in information asymmetries, a more efficient allocation of goods, and an increase in market fairness.
Introduction
Information asymmetries emerge in markets in which one party has more information about the quality of the offered goods [Citation58]. The party with this information advantage is able to discern the quality of the goods; the party with the information disadvantage is not [Citation36]. Classical examples of markets that suffer from information asymmetry include the insurance market, the employee-recruitment market, and the used-car market. The last of these is perhaps the best-known illustrative example of this issue. In such markets, signaling methods have emerged as one of the most effective mechanisms for mitigating the effects of information asymmetry [Citation57]. Signals are pieces of information sent by one [Citation17] party—the better-informed party—to the less-informed party [Citation19]. To be trustworthy and effective, signals need to be difficult to fake [Citation57]. The extent to which a signal correlates with the unobservable quality of the underlying element it refers to is called the signal’s fit [Citation17]. In the case of the used-car market, the most common type of signal is that sent by intermediating dealers. Dealers have the skills necessary to better identify product quality and to profit from buying peaches (goods of relatively high quality) at—or slightly above—the average price. And to then sell them back to buyers at higher prices, using their established brand name or guarantees as signals [Citation13].
Yet despite the positive effects of intermediary signaling sent by dealers—the fact that it forestalls market collapse and enables a large share of market transactions—the used-car market still functions well below its optimum [Citation60]. Overall, according to the European Parliament [Citation21], odometer fraud alone entails losses for European Union citizens of 8.9 billion euros each year. Furthermore, the overpayment made by buyers for used lemons (cars of relatively low quality) sold by dealers after their first year of ownership constitutes on average 18 percent of the price of such cars [Citation13]. Finally, while dealer signaling allows more transactions to take place, most of the additional revenue generated is reaped by the intermediary dealers themselves [Citation35]. Consequently, many sellers of peaches refrain from entering the market and a large number of potential trades do not even take place.
The digitalization of the automotive ecosystem is about to change all this: Car manufacturers aim to monetize their access to clients by offering clients services and generating value from the very data that clients, and their vehicles, collect. Such data can much better describe and predict the value of a used car, and thus resolve the market-for-lemons problem. Centralized control of car usage data (by vehicle manufacturers, or technology firms such as Google) may, however, result in digital platforms of ever greater power reaping the lion’s share of the benefits of any efficiency gains these changes lead to. This would not be in the interests of other players in this market, including insurers, or national and supranational regulators [Citation49, Citation55].
Lately, blockchain information systems have been proposed as an enabler of a novel approach to allowing real-world assets, such as used cars, to be digitized in a trusted and decentralized manner [Citation25, Citation43]. Using blockchain technology, it is possible to ensure data integrity and compatibilities that foster data sharing [Citation54] while keeping transaction costs under control [Citation68]. This enables multiple parties to collaborate and create trusted, decentralized product history certificates that draw on the data sources of many agents [Citation45, Citation69]. Such blockchain-based multi-party product history certificates—as we will argue in this paper—afford dealers the action potential to send signals of a higher fit than those they send today. However, given that blockchain-enabled multi-party certificates are only a resource in the context of information asymmetries and not a solution per se, it remains to be seen whether this will indeed lead to significantly different market outcomes. Contrary to the hype surrounding the technology, it has yet to prove itself to several challenges and open questions. Building on the behavioral assumptions and theories that govern the market for lemons, we therefore formulate the following research question (RQ):
Research Question: How does multi-party certification impact the market for lemons?
This question responds to widespread calls for in-depth analytical and empirical analysis of the affordances of blockchain applications for their users and how these affect market outcomes [Citation22, Citation51, Citation59]. The information systems (IS) discipline is methodologically the best suited to the study of the capabilities of blockchain and its economic impacts, an endeavor that essentially can help to avoid unnecessary costs and at the same time foster the advancement of blockchain-based systems by guiding better-founded and targeted development [Citation70]. We will apply a socio-technical artifact perspective [Citation15] to structure the benefits of blockchain more precisely and to theorize and explicate “the axis of cohesion” of blockchain-enabled multi-party certification in the market for lemons [Citation53].
The remainder of this paper is organized as follows. First, we review the economics that govern the market for lemons today and the shortcomings of current mitigants. Next, we introduce the socio-technical artifact framework [Citation15], which enables us to work out. On the one hand, how blockchain affords multiple businesses the action potential to generate multi-party certificates and, on the other hand, blockchain’s affordance for the users of its application in the used-car market. In the subsequent section, we theorize the impact of dealers’ use of multi-party certification in the market for lemons in a formal model and derive a set of hypotheses. We then empirically test these hypotheses using data from an experiment. Next, we present the results of that experiment, which indicate that buyers and dealers react to multi-party certification as theoretically proposed in the assertions derived from the model. In light of prior work, we continue discussing the observed effects of blockchain-enabled multi-party certification in the market for lemons and its practical implications. Finally, we conclude, pointing out the limitations of this paper and possible lines of future work.
Related Work
Market for Lemons and Current Mitigants
The market for lemons is usually modelled as though only two types of goods—peaches and lemons—are available to trade. While peaches have no observable defects, lemons do. Since the characteristics that differentiate the two types of goods, in our case used cars, are mostly hidden or hard to ascertain, buyers cannot tell the difference between a peach and a lemon, so, between a good and a bad car. As a result, both types of car will sell at a market price (resulting from an equilibrium of supply and demand) that reflects the average quality of the cars on the market [Citation62]. This market outcome is caused by buyers’ inability to evaluate product quality as well as by sellers’ inability to prove the quality of their cars [Citation47]. This market equilibrium leaves the sellers of good cars with no choice but to sell their peaches for less than their actual value—obtaining negative rents—or to leave the market [Citation62]. Consequently, the classical adverse-selection phenomenon comes into effect: lemons tend to drive out peaches, which theoretically might even lead to a market collapse [Citation3]. Hence, markets with such an asymmetric distribution of information suffer from losses in social welfare [Citation17]. These losses include overpayments by buyers for lesser quality goods (in our case, poorer quality used cars), the negative rents of the sellers of peaches, and the losses of both buyers and sellers due to a reduced number of trades [Citation3].
A collapse of this market is, however, unknown. One main reason for this in the case of the used-car market is the existence of used-car dealers [Citation3, Citation34]. Dealers have the skills to identify product quality and consequently profit from buying peaches at, or slightly above, the average price and selling them at a higher price by using signaling methods such as warranties or licensing models, or simply thanks to buyers’ greater trust in their established brand names [Citation13]. Such methods intermediate the market by providing a signal to the buyer, and hence reduce product uncertainty [Citation63]. From a seller’s perspective, such measures lead to more high-quality cars entering the market thanks to the promise of higher prices [Citation50] for peaches. Signals can be understood by the less-informed party, and help that party to mitigate information asymmetry [Citation19]. While signals can reduce information asymmetry, their effectiveness is affected by their fit (i.e., the extent to which a signal is correlated with unobservable quality), their frequency (the number of times a receiver receives a signal), and their observability (the extent to which outsiders are able to notice them) [Citation18, Citation29]. Signals provided by intermediaries, such as dealers, have been shown to somewhat ameliorate the lemons problem. Yet, the great share of the benefits in such markets are reaped by these very intermediaries [Citation12]. Due to their information advantage and the market setting, they apply negotiation tactics such as working with the anchoring effect [Citation1, Citation4]. The anchoring effect is a well-known cognitive bias in negotiation. It is the tendency to assign too much weight to the first number put on the table and then to inadequately adjust from that starting point [Citation11].
Product history certification provides an alternative to signaling by intermediating agents. Product history certification can be provided either by individual sellers who create individual documentation of their products’ quality or by independent certifiers. Product history certification provided by individual sellers has thus far been inefficient and costly from a seller’s perspective. Moreover, this type of signal also fails to create the necessary trust on the buyers’ side: document falsification is easy, which makes the faking of the signal easy too [Citation47]. Product history certification generated by single independent certifiers is more established than that provided by individual sellers. Carfax and Eurotax are examples of this type of third-party certification in the car market, and each also enables signaling [Citation58]. However, despite their established role, they have shortcomings. First, single providers often lack the ability to obtain extensive information that is dispersed among different actors [Citation43]. Second, even if an intermediary certifier collects data from multiple parties, it is often not known where this data comes from and whether it is correct or can be trusted [Citation64]. There is, therefore, no traceability and no data provenance, both of which have been deemed crucial for the efficient transmission of valuable information [Citation32, Citation34]. This lack of traceability and data provenance negatively affects signal fit [Citation17]. Third, single third-party intermediary certifiers have no incentive to provide more information than the minimum required. According to prior studies [Citation39], in a monopolistic market the best strategic choice for such intermediaries is to maximize profits by designing certificates that reveal only minimal information. Thus, in today’s markets we often observe such single third-party certifiers employing monopolistic strategies, including providing a quality label concerning specific vehicle characteristics inspected. These shortcomings—the lack of access to relevant information dispersed among multiple parties, the lack of trust in data quality, and the lack of incentives for single third-party certifiers to provide extensive information—all limit signals’ fit, and hence their effectiveness [Citation18].
From prior work it emerges that the signals provided by used-car dealers have thus far been the most effective mechanism for mitigating the negative consequences of information asymmetry in this particular instance of the market for lemons. Yet such intermediaries also reap a large share of the benefits that they themselves generate in the market. Markets with information asymmetry thus continue to function below a socially optimal level, and there is clear evidence that the classical market-for-lemons problem still persists today [Citation21, Citation60].
Blockchain-Based Product History Certification
“Blockchain” is regularly used as an umbrella term for a combination of technologies. The key characteristics of blockchains [Citation10, Citation23, Citation24, Citation33, Citation37, Citation41] are their distributed database architecture, which enables them to store and access transactions dispersed among multiple parties; their network-based consensus mechanism, which allows agreement to be reached regarding transaction validity, based upon predefined rules in a decentralized manner without the need for a central authority; and their inherent cryptographic logic, which defines their immutable and transparent nature. Blockchain-based systems make it possible to manage unique digital assets in a distributed and decentralized setting [Citation46, Citation54]. Bitcoin strove to create and distribute digital money without a central bank [Citation42]. Thus, decentralization is not just a feature of blockchains; rather it is their defining element, and one that has been eagerly adopted by practitioners. The Cardossier consortium, for example, strives to collect all data from a car’s life cycle, creating unique and definitive records describing the history and the state of each car on its platform. The consortium chose to go with a distributed ledger to avoid the concentration of economic power that comes with a central platform [Citation67]. In recent years more sophisticated architectures have become popular (e.g., Ethereum, Corda). They encapsulate the traditional blockchain components in hardware and protocol layers. On top, they establish platform mechanisms to allow for distributed apps (DApps). They share typical features of app stores (such as secure and authenticated access and quality control) but in addition can access data shared via a blockchain [Citation23, Citation28] and ensure its integrity [Citation16].
For the purposes of this paper, a blockchain is best regarded as a socio-technical system [Citation53]. As the information stored in the system is key to an understanding of the potential and impact of blockchain systems, the recently published conception of an IS artifact [Citation15] is well suited to structuring prior work. It also serves as a theoretical basis that helps us to deduce the affordances of the technology for a variety of social actors working with it or using an application of it. Finally, we will use, in particular, insights about the affordance of the technology for sellers of used cars to dive deeper, and to model the effects of the use of the technology’s application in the market for lemons. The relationship between the framework [Citation15] and our model emerges through incorporating their language and some of their assertions and meta-principles.
The core of the reference IS artifact consists of the technical and the social subsystems, which are in an affording/constraining relationship. While the technical subsystem describes the techniques and mechanisms that enable organizational transformation, the social subsystem describes the individual actors and their knowledge and skills, as well as incentives and these actors’ relationships. The notion of affordances–constraints connects these two subsystems. Another essential part of the reference IS artifact is the information element, which determines how the social and technical subsystems interact [Citation15]. The subsystems are open and interact with the surrounding environment. The dynamic nature of the IS artifact is highlighted by a feedback loop, which feeds information from the output of the system back into the system, leading to changes in the interactions between the subsystems promoting a dynamic equilibrium. The feedback loop also helps to manage the system’s information entropy (or entropy as we will hereafter refer to it), which is the average level of uncertainty inherent in a variable’s possible outcomes [Citation56]. As such, the entropy describes the degree to which a system is disorganized, with higher entropy implying a higher level of uncertainty in a (sub)system [Citation15].
An analysis of prior research reveals three distinct but interrelated socio-technical subsystems working with or using blockchain in the automotive ecosystem (): the infrastructure provider subsystem, the car-related business subsystem, and the used-car buyer and seller subsystem. We will now briefly introduce the first two subsystems in the context of our research, and clarify the role of blockchain technology in the development of multi-party certificates. We will also detail the used-car seller (i.e., dealers) and buyer subsystem, which allows us to derive the affordance of multi-party certificates provided by blockchain for the participants of the used-car market.
Figure 1. A socio-technical artifact perspective [Citation15] on prior works on blockchain-enabled certification in the car ecosystem.
![Figure 1. A socio-technical artifact perspective [Citation15] on prior works on blockchain-enabled certification in the car ecosystem.](/cms/asset/2dd401f5-b7b9-4fc8-a7e9-8200f4329059/mmis_a_2063555_f0001_oc.jpg)
Infrastructure Provider Subsystem (Marked in Dark Grey in )
Participants in automotive ecosystems, including car manufacturers, importers, used-car dealers, car insurers, and mobility service providers, are experiencing rising competitive pressures from “digital giants” (input). At the same time regulatory pressure (for example, from the European Union) [Citation67] is forcing car manufacturers to explore new ways of collecting, managing, and using their ever increasing amounts of car-related data (input). While digital giants try to establish monopolistic platforms for collecting and maintaining the increasing amounts of data generated by car usage, the goal of blockchain infrastructure providers is to build cross-organizational decentralized platforms [Citation5, Citation59] (output). Thus, the distributed, decentralized, and immutable system architecture of blockchain (technical subsystem) becomes a necessary enabler to addressing the social needs (i.e., decentralized interorganizational collaboration [Citation69]) of the developers and providers of a blockchain infrastructure (social subsystem). Put differently, through the affordance of decentralizing systems’ power, blockchain enables the developers and providers of a decentralized car ledger to collaborate in a decentralized manner [Citation20]. As a result of the distribution of “information,” and consequentially a reduction of extreme outcomes, the system experiences a reduction in entropy. While other technologies (e.g., distributed databases) could in principle provide similar solutions, they tend to be either too centralized or less technologically mature. The chief architect of Cardossier summarizes: “Of course we could solve these collaboration challenges we previously had differently but if we would do so, and evaluate the resulting technology neutrally, we would end up with exactly a solution as blockchain” [7, p. 7)].
Car-Related Businesses (Marked in Light Grey in )
The individual business actors of the automotive ecosystem see opportunities to exchange data and increase efficiencies, create novel, data-driven products, and better customize their products and services to suit users’ needs [Citation7, Citation46] (output). Today, however, they fear losing control over advantageous resources if they engage in exchanging data [Citation6, Citation32] (input). Building on its decentralized infrastructure and utilizing blockchain’s property of tokenization (technical subsystem) [Citation10, Citation54], businesses can create novel data exchanges that address their need to leverage but at the same time protect their resources [Citation68] (social subsystem). More specifically, besides the decentralization of system power for infrastructure providers, blockchain affords providers of car data the action potential to create ownership over digital resources [Citation40, Citation45]. By enabling the explicit assignment of property rights over digital goods such as data [Citation69], it incentivizes car-related businesses to provide and trade their proprietary data, as they can now control its use and, consequently, monetize it fairly [Citation66]. This consequently enables car-related businesses to create joint product innovations such as multi-party car history certificates [Citation6, Citation7]. While research in this area is, to date, rather limited, it is fair to say that by integrating previously fragmented data, such joint data products enable a reduction in uncertainty (e.g., concerning the characteristics of a car being registered, insured, or sold)—and, therefore, a decrease in information entropy.
While the idea of providing certification of a car’s history is not new, based on the above insights we can conclude that blockchain-based approaches to product history certification (e.g., the initial prototype [Citation43]) differ from previous approaches—such as a Carfax or Eurotax reports, both provided by a single third party—in that they are joint, multi-party efforts via which tokenized, digital representations of real-world assets are created. Building on these insights, we coin the term “multi-party certification,” defining it as follows: “Multi-party certification is the documentation of the history of an asset by multiple independent parties in a trusted manner.” We acknowledge that multi-party certification could be delivered by any (existing or future) technology similar to blockchain that would address the needs of its providers in terms of power decentralization and unique digital ownership.
Buyers and Sellers of Used Cars Subsystem (Marked in White in )
While the first two subsystems concern the development of multi-party certificates, the last subsystem, the Buyers and Sellers of Used Cars Subsystem (marked in white in ) concerns the use of multi-party certificates: The social actors that are intended to use multi-party certificates are the sellers and buyers of used cars [Citation9, Citation64, Citation65]. These are confronted with problems of information asymmetry during used-car sales (input) [Citation3]. The desired outputs of the use of blockchain applications are a reduction of information asymmetries and a consequent increase in market fairness [Citation8, Citation43] (output). The use of multi-party certificates—which are provided by blockchain DApps that draw on the resources of many and consequently provide more comprehensive information, and come with the ability to provide proof-of provenance of the record history (technical subsystem)—affords sellers the action potential to better signal the quality of their cars [Citation8]. This ability of sellers to better signal product quality is due to both the increased amount of data collected and combined from multiple sources and the increased trust in data quality due to the difficulty of faking data. Thus, we posit that blockchain-enabled multi-party certificates afford dealers (i.e., sellers) the action potential to send signals of higher fit than they would without such certificates.
While the present review of prior work in this field has allowed us to coin the term multi-party certification and to suggest how it affords dealers the action potential to send signals of higher fit, multi-party certification’s impact on agent behavior and on market outcomes (including the dynamic equilibrium of the system and of the subsystems, induced by changes in information entropy) remains an unknown. A first approach to addressing this gap has been proposed for the credit market [Citation44]. Using modelling techniques, the authors show that disclosed blockchain-based information allows participants in the credit market to learn about quality differences between peaches and lemons, to deceive their counterparties, and to move to a new equilibrium with increased utility. The researchers forecast a market collapse despite a welfare gain [Citation44]. While this research provides important insights into the credit market, its findings cannot simply be transferred to the used-car market, as market dynamics might differ due to the presence of different participants and interrelationships. Additionally, it lacks an empirical analysis of the proposed market dynamics and the changes in entropy. This lack, and uncertainty regarding market outcomes, motivates our research study. In the following we derive a model to theorize the market effects of dealers’ use of multi-party certificates.
Model
This paper strives to understand how (blockchain-enabled) multi-party certification impacts the market for lemons. This goal requires a more explicit modelling of the buyers’ and sellers’ subsystem to understand its current dynamics and how these may be affected by the availability of multi-party certificates.
In this subsystem potential sellers (i.e., dealers) and buyers meet and negotiate potential trades for used cars. They may use information provided by the blockchain application. This results in lower entropy due to the higher fit of the signals afforded by the dealers’ use of multi-party certification. We now present a simple economic model that describes this particular social subsystem in more detail. The objective of this model is to analyze how the existence of multi-party certification might affect agent behavior and market outcomes. The model is a slightly altered version of that introduced by Levin [Citation36]. To represent a signal’s fit, we use different parameterizations for signals sent by dealers where multi-party certification is available and for those signals sent by dealers where multi-party certification is not available. Furthermore, the model formalizes the concept of a good’s quality over a continuum of possible values. This differs from the traditional, dichotomous approach, which only accounts for high- and low-quality goods [Citation18].
Market Definition
Following Levin [Citation36], we consider an indivisible good, which can be traded between a used-car dealer and a buyer. The exact quality of the good, and the resulting value, v, are unknown to both agents. Each agent receives a signal about the good. After receiving their respective signals, dealer and buyer attempt to negotiate a price, p, for the good. If they agree on a price, they trade the good at that price. Thus, the respective relative revenues for dealer and buyer are (p-v)/v and (v-p)/v. If no trade occurs, each agent receives a zero payoff. We employ relative revenues (both in the model and in the experiment) to account for the fact that cars of different prices are sold. In order to demonstrate the generalizability of our findings, we also develop a model using absolute price differences for risk-averse dealers and buyers, and a standard CARA utility function. This function changes neither the resulting propositions nor the outcomes of their testing. The results are available upon request. In the setup where multi-party certification is not available, both buyer and dealer receive a general description of the good. As a result, both dealer and buyer believe that the good’s value, v, lies in some compact interval [L,U] ⊂ .
In addition, the buyer believes that a distribution of possible values is given by the density function fB: [L,U] → with L > 0. In line with the instructions provided to participants in the experiment, the buyer wants to maximize the expected value of his relative revenue, (v-p)/v, if he pays the price, p. This allows us to model the quality of the cars to be traded over a continuum space, and not only in a dichotomous manner. Since he receives a payoff of zero in the absence of a trade, the buyer will only buy at price p if his expected relative revenue at that price is nonnegative,
In addition to the general description of the good, the dealer also receives the average value, m, of the good, satisfying the general description that dealers have more information than buyers about any particular car’s quality. The used-car dealer now believes that a distribution of possible values is given by the density function fS: [L,U] → . The dealer wants to maximize her relative revenue, (p-v)/v, if she receives the price, p. Since she receives a payoff of zero in the absence of a trade, she will only accept price p if the expected relative revenue at p is nonnegative. This conditional expected value is
with the density satisfying
In the setup with multi-party certification, both dealer and buyer not only receive the general information about the car (and the dealer also the mean price, m) but also verified and detailed information about the specific instance of the car. Both used-car dealers and buyers need, however, to derive their own price expectations with regard to the car based on this information. To simplify the analysis, we assume that the two agents form their expectations independently of one another. Thus, we avoid the analysis of a common value problem, and instead assume independent values. As before, the buyer forms his beliefs about the support of a density function of values of the good. However, due to the additional detailed information, fB,M: [LM,UM] → with L ≤ LM < UM ≤ U, the buyer’s conditional expected surplus at a trade of price p is now
Similarly, the dealer’s conditional expected surplus at a trade of price p is now
with her density being fS,M: [LM,UM] → . Depending on the detailed information, the dealer’s density may or may not satisfy the mean condition. The detailed information on the specific instance of the good may convince the dealer that the average price of such an instance cannot be m.
Parametrizations
To obtain more specific predictions, we make further assumptions. Without loss of generality, we impose the normalization L = 1. Furthermore, we assume that fB is the uniform density; therefore:
Hence, in the setting where multi-party certification is not available the buyer will only accept a price of at most (U-1)/ln(U). In this setup, the dealer only receives the additional information that the mean value of this type of good is m. The value of m now affects her beliefs. For Case Single-Low (SL), she believes that 1 < m < (U + 1)/2, in a uniform distribution on [1, 2m-1], and for Case Single-High (SH) that U > m > (U + 1)/2, in a uniform distribution on [2m-U,U]. In Case SL, the dealer’s expected payoff, conditional on a trade at price p, is
and in Case SH the payoff is given by
This simple model reproduces the classical result of Akerlof [Citation3]. In Case SL, when the dealer receives a signal indicating a low value for m she will trade and agree on a price within
On the contrary, in Case SH, when the dealer receives a signal of higher fit the agents will not trade. For m > (U + 1)/2, the dealer will demand a price of at least 2(U-m)/ln(U/(2m-U)), which exceeds the upper bound on the price, (U-1)/ln(U), that the buyer is willing to pay. We summarize the results in the following proposition.
Proposition 1: In the parameterized model where multi-party certification is not available, a trade is impossible if and only if the dealer receives a signal, m, larger than (U + 1)/2. If a trade occurs, then the price satisfies .
Next, we consider the parameterized setup where dealers are expected to send signals of higher fit through the use of multi-party certificates. In this case, the buyer’s conditional expected surplus at a price p is
assuming again a uniform distribution. Hence, with signals of higher fit the buyer will only accept a price of at most ()/
.
Recall that in the parametrized model where multi-party certification is not available—and hence only signals sent by dealers based on their own knowledge advantage and reputation are available—the buyer has a uniform distribution on [1,U]. This probability distribution has the maximal entropy of all continuous distributions with the support [1,U]. Therefore, whenever or
, the entropy for the buyer’s belief distribution is smaller in the parametrized model with signals of higher fit, which dealers send by means of multi-party certification. Put differently, the difference in belief distributions between the parametrized models reflects how the technical subsystems in affect the level of entropy of information in the social subsystem of dealer and buyer. The signals sent when multi-party certification is available provide more information and thus lower entropy than those sent when multi-party certification is not available.
The dealer now has access to the more detailed multi-party information (in addition to the mean price, m). In order to combine this detailed multi-party information on the specific good with the average m of typical instances of the good, we need to distinguish three cases.
Case Multi-Low (ML)
The signal sent when multi-party certification is available indicates a very-low-quality car, such that UM ≤ m. While the dealer realizes that the value of the good cannot be m, she believes that the density is increasing in [LM,UM]. Specifically, she believes that the density is linear with
for v and 0 otherwise. The dealer’s conditional expected surplus at price p is then
There will be no trade, since the dealer’s and buyer’s surpluses are never both nonnegative for a price p . (Aside: here, the signals sent thanks to the dealer’s use of multi-party certification and the signal on the average, m, are so diffuse that the resulting beliefs of the dealer prohibit a trade.) The entropy of the information from the signal afforded by the dealer’s use of multi-party certification is smaller than that for the signal sent by the dealer alone where multi-party certification is not available since UM ≤ m and so
. Recall that the uniform distribution on
in Case SL is the distribution with maximal entropy on
.
Case Multi-High (MH)
The signal sent when multi-party certification is available indicates a very-high-quality car, such that LM ≥ m. While the dealer realizes that the value of the good cannot be m, she believes that the density is decreasing in [LM,UM]. Specifically, she believes that the density is linear with
for v and 0 otherwise. The dealer’s conditional expected surplus at a price p is then
There will always be a trade, since now the dealer’s and buyer’s surpluses are both nonnegative for all prices in a nonempty, compact subinterval of [LM,UM]. In particular, contrary to the intermediary signaling only setup, there will now be a trade even for high-value cars, when
Comparing Case SH with Case MH, we notice that once again the signal sent when multi-party certification is available leads to a lower entropy distribution for the dealer than does the signal sent without the availability of multi-party certification. Since LM ≥ m,
, with the latter interval being the support of the uniform distribution in Case SH.
Case Multi-Middle (MM)
The signal sent when multi-party certification is available satisfies [LM,UM] ϶ m. If 3m > LM + 2UM—such that the signal indicates a rather-low-quality car—then no triangular distribution on [LM,UM] has the mean m. In this case the dealer chooses the same distribution as in Case ML. There is no trade. Moreover, , and, therefore, the entropy is lower than in the system with the signal of the dealer only.
If 3m < 2 LM + UM—as a result, the signal sent when multi-party certification is available indicates a rather-high-quality car—then no triangular distribution on [LM,UM] has the mean m. In this case the dealer chooses the same distribution as in Case MH. There is a trade. Moreover, and so the entropy is again smaller with the signal sent when multi-party certification is available.
Finally, for
the dealer assumes a triangular density on [LM,UM] with mean m. The mode, D , of the distribution satisfies (LM + D + UM)/3 = m. The resulting density is
, and
and 0 otherwise. The dealer’s conditional expected value given a trade at price p is then
For a sufficiently small D near LM there is a trade. For larger values of D (and m) there is no trade. We summarize the results in the following proposition.
Proposition 2: In the parameterized model with signals sent where multi-party certification is available, there is a threshold T such that there is no trade if the dealer’s signal, m, satisfies 3m > T. On the contrary, for 3m ≤ T there is always a trade. If a trade occurs, then the price satisfies
.
Based on these two propositions, we can draw additional conclusions for an important special case—namely, when the signals sent when multi-party certification is available lead to identical relative changes of the lower and upper bounds of possible values.
Corollary 1: Suppose for some
such that
; that is, the signal afforded by the dealer’s use of multi-party certification increases the lower bound and decreases the upper bound of the good’s value by the factor
. Then,
that is, the model with signals afforded by the dealer’s use of multi-party certification predicts trades occurring within a smaller price range than the model with signals sent by the dealer only.
Alternatively, suppose and
for
such that
; that is, the signal sent when multi-party certification is available increases the lower bound and decreases the upper bound of the good’s value by the portion . Then, again,
.
positions the models with dealer-only signaling and with signaling through the dealers’ use of multi-party certification, respectively, in the context of the social subsystem of the used-car buyer and seller system. The left (right) diagram in the center right of the figure shows the knowledge of the individual agents (buyer/seller) under dealer-only (with the use of multi-party certification) signals. Based on the available knowledge, the buyer derives a maximal price he is willing to pay and the dealer derives a minimal price at which she is willing to sell. If the dealer’s minimal price exceeds the buyer’s maximal price, then there will be no trade. On the contrary, if the order of the two price limits is reversed, then the two agents can find a trade price in the arrangement zone. As a consequence of changes in the availability of knowledge, we expect to see changes in the asking price, sale price, the revenues for buyer and seller, and the allocation of goods in the market, which we will formulate as hypotheses in the following. And, all in all, as indicates we expect an increase in market fairness at the overall output.
Hypotheses Development
A comparison between the model with signals sent by the dealer alone and the model with additional signals sent by the dealer where multi-party certification is available—in conjunction with insights from the academic literature—leads us to several testable hypotheses regarding the effects of the introduction of multi-party certification. First, due to the fact that dealers—by their very nature—have an information advantage in the market today, and in line with Akerlof [Citation3], we expect our experiment to mirror the natural behavior of dealers. As a result, dealers will utilize their information advantage and anchor buyers by demanding well-above-average prices [Citation1, Citation44]. However, since we expect the signals afforded by the dealer’s use of multi-party certification—enabled by blockchain technology [Citation43]—to have greater fit and therefore to be more effective, we also expect the dealer’s initial asking price to decrease. Thus, we derive Hypothesis 1 regarding the dealer’s initial asking price:
Hypothesis 1 (Dealer’s Initial Asking Price) (H1): Where multi-party certification is available, the dealer realizes that her relative information advantage will be reduced by the ability to send signals of higher fit. Consequently, the dealer will open negotiations with lower initial asking prices compared to those in the setup where multi-party certification is not available.
While our model does not consider the agents’ negotiation strategies, it does predict—under the assumptions of Corollary 1—that the set of possible prices for trades in the model with signaling afforded by the dealer’s use of multi-party certification will be a subset of the corresponding set for the model with signaling stemming from dealers alone; see also the price ranges in . We therefore expect lower average sale prices with signals sent when multi-party certification is available than with signals sent by intermediary dealers only. We would expect the same outcome as a consequence of the reduction in opportunities for dealers to take advantage of the anchoring effect. The confluence of the model’s implications and our knowledge, from the behavioral literature, of the workings of the anchoring effect suggests the following outcome for sale prices:
Hypothesis 2 (Sale Prices) (H2): Given the reduced anchoring effect with signals where multi-party certification is available, the lower opening asking prices of the dealer lead to lower average sale prices than is the case with signals sent by the dealer only.
Consequently, as the dealer’s use of multi-party certification provides the buyer with signals of higher fit, reducing the relative information disadvantage if not eliminating it, hypothesis 2 appears to suggest that the dealer’s (buyer’s) relative revenue is lower (higher) when multi-party certification is available than when it is not. Thus, to specify H2, we test two additional hypotheses, specifically concerning the agents’ relative revenues:
Hypothesis 2.1 (Impact for the Dealer) (H2.1): The dealer’s average relative revenue is lower with signaling afforded by her use of multi-party certification than with dealer signaling alone.
Hypothesis 2.2 (Impact for the Buyer) (H2.2): The buyer’s average relative revenue is higher with signals afforded by the dealer’s use of multi-party certification than with dealer signaling alone.
The model predicts, somewhat starkly, that in the setup where only dealer signaling is available no peaches will be sold (see also Proposition 1). In the model (see Cases ML and MH in the derivation of Proposition 2) with signals of higher fit meanwhile, both lemons and peaches will be sold. This leads to a hypothesis with respect to the quality of the cars sold:
Hypothesis 3 (Market Impact) (H3): The proportion of lemons sold decreases with signaling afforded by the dealer’s use of multi-party certification, whereas the proportion of peaches sold increases.
Experimental Methods
In the following, we first explain how our laboratory market game was designed and then detail the experiment setup for the data collection processes.
Market Game Design
With the ultimate aim of testing our hypotheses regarding the effects of multi-party certification on market outcomes, we developed the experimental market game CarMarket. An experimental approach is beneficial in studies that, like the present one, aim to understand market dynamics under changing conditions [Citation14]. The laboratory environment provides a suitable vessel within which to study how the introduction of a novel application—in this case, multi-party certification—affects the market and its participants [Citation2, Citation27, Citation52, Citation61].
Our CarMarket game involves a web-based application designed in the form of an online car marketplace in which the participants have the task of buying and, respectively, selling a used car. The overall design was drawn from, and discussed with experts from, an existing, widely used online used-car market platform in Europe, AutoScout24. As is the case for today’s used-car market platforms, participants in our experiment had the opportunity to sign up to the platform and view or advertise cars. Unlike in much of today’s market, they were additionally able to negotiate and engage in binding sales transactions and to close deals online. A used-car deal is considered closed once both buyer and dealer agree and confirm the transaction. In order to resemble the real-world market setting, in which dealers have an information advantage, as mentioned in the model description dealers also receive the average market price of their car.
Furthermore, CarMarket was designed to allow the installation of two different information structures. In the first setup, Instance A, participants receive no more information than that we describe in the Modeling Section (general information about the car, provided by the dealer), such that buyers have to form their beliefs based solely on the advertisement and the negotiation process with dealers. In the second setup, Instance B, ceteris paribus, both buyers and dealers have access to information that emerges from a blockchain-based IS artifact—CarCerti. CarCerti simulates a blockchain-based information system that enables a variety of stakeholders to collect and maintain all relevant events that occur during the life cycle of a car. CarCerti therefore offers trusted car history certificates created jointly by multiple parties. CarCerti was designed and developed in collaboration with representatives of the real-world blockchain project Cardossier that actually builds such car history certificates using Corda technology. As mentioned in the model description, CarCerti was used to verify the general information about the cars (e.g., mileage wear, model, year of manufacture, engine type) and to provide additional detailed information about the specific instance of each car, which helps users to narrow the expected value range. The two additional information categories provided were: repair and service history (e.g., accidents, maintenance, service recalls), and driver dynamics (e.g., previous handling, driving characteristics and distances). The information provided by CarCerti was visually highlighted. illustrates how this was experienced by experiment participants.
Figure 3. Information representation with and without CarCerti (Left: Instance A; Right: Instance B).
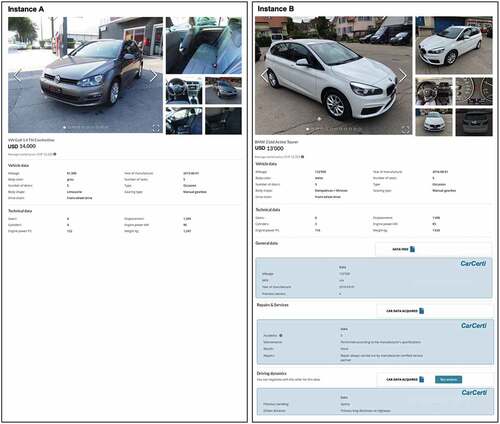
To ensure external validity, the design of the marketplace was iteratively discussed and developed in collaboration with experts from the field. Furthermore, the cars used in CarMarket were real cars that were available on the real-world used-car market at the time the experiment was conducted. To reduce complexity and ease comparability, the value of the cars that were available on CarMarket varied within a fixed range of USD 8,000‒12,000. Similarly, the number of models and brands were kept to a minimum. We received all our information about the cars thanks to our collaboration with the aforementioned online used-car market platform. Furthermore, in order to evaluate the cars and approximate their true values, we used a standard-assessment table provided to us by a leading Swiss used-car retailer. We acknowledge that no universal true actual value exists; thus, additionally, two expert valuations were conducted, each by a project partner who are also major players in Switzerland. The mean of these valuations constituted the actual value of the cars for the purposes of our analysis. This value is the value in the model from the Modeling Section. As is the case with today’s used-car market, a certain proportion of the cars on CarMarket were of below average quality, just as a certain proportion of the cars were of above average quality. Over the course of a year, we conducted several small-scale tests of our market game to validate its design.
Experimental Design
In order to test our hypotheses, a larger test group was selected and a between-subjects design was applied [Citation48]. Overall, 105 participants played both roles (buyer and dealer), giving us 210 users. The participants in the market game were students from a Master’s program in Business Administration at the University of Zürich, who were strongly incentivized to act realistically by the opportunity to earn bonus points for their final assessment. The bonus points that they received at the end of the game were dependent on the results they achieved during the game. The objective of each player was to maximize his or her relative revenue from both roles. We choose relative revenue as a measurement of success in order to account for the fact that the cars involved had values that differed slightly from one another. In other words, it did not matter whether a participant bought a rather expensive or a rather cheap car. This approach also validates our choice of having a continuum of qualities rather than a dichotomous view that merely differentiates peaches from lemons. All relevant information (i.e., regarding the game’s objective and the criteria governing the apportioning of bonus points, the quality of the cars on the market, and CarCerti, including the underlying blockchain technology) was clearly communicated and explained to the participants in advance, in written form and through a screencast. Additionally, at the beginning of the experiment, the game, the objectives, and the application’s key features, as well as the CarCerti and the concept of blockchain technology, which it is built upon, were again explained, this time verbally.
Approximately 70 of the 130 students enrolled in this class normally attend sessions. For this particular session, 105 students signed up and participated, thus indicating that the incentive of earning bonus points for the final assessment did indeed work. The results achieved (i.e., bonus points) were automatically calculated and communicated to the students either after they finished playing both roles or once the allotted time was up. If a participant did not conclude a deal within the given time, the final relative revenue was zero. From prior studies, we know that using students as study objects can yield just as reliable results as using actual customers if the students have sufficient requisite knowledge, and we tested for this before the game using a questionnaire [Citation30]. Furthermore, implementing an economic experiment in the context of IS can bridge the gap between rational economic models like the one in the Modeling Section and the actual process of human decision-making [Citation26].
To allocate the participants between the two instances (Instance A and Instance B) and apply a between-subjects design, we randomly divided the participants into two groups and only controlled for an equal distribution of study subjects between the groups [Citation38]. Also, in order to account for internal validity, we randomized the allocation of cars to the participants. We ensured, however, that in both instances the same cars were put on sale. Since all participants had the same objective, the budget each received was also the same. Finally, all participants started the CarMarket game at the same time and in both instances the duration was set equally for all participants, at one hour.
Results
In the following we present the results of our experimental market game in relation to the hypotheses formulated in the Modeling Section.
Starting with the impact of the availability of multi-party certification on dealers’ initial asking prices, presents descriptive statistics with regard to the average initial asking price proposed by dealers in each instance. We analyzed the very first offer prices set by dealers for the cars that had been randomly allocated to them on the platform.
Table 1. Dealers’ Initial Asking Prices
While we distributed the participants equally between the instances, we deleted one transaction in Instance A due to a participant having sold a car at an unrealistic price.Footnote1 Thus, we report on 52 transactions for Instance A and 53 transactions for Instance B. The results show that the average initial asking price proposed by dealers in the instance with dealer signaling only, Instance A, is USD 1.832 higher than that in the instance with signaling afforded by the use of multi-party certification, Instance B. In order to test if the average initial asking price is significantly different between the instances, we conduct a two-population t-test for the difference between the two means. The resulting t-statistics show a standard deviation of 3.162 for the average initial asking price under dealer signaling only and one of 2.568 for the average initial asking price under signals sent when multi-party certification was available, which leads to a two-sided p-value of 0.00174. Hence, there is support for H1.
Next, we analyzed how the anchoring effect of the initial asking price influenced the final average sale price of the cars sold in each instance ().
Table 2. Sale prices
We find that the average sale price for cars decreased between the instances, from USD 11.567 in the instance with no multi-party certification, Instance A, to USD 10.011 in the instance where multi-party certification was available, Instance B. In other words, we observe a USD 1.556 reduction in the average sale price between the instances. The difference between the two means is strongly significant, with a p-value for the two-population t-test of 0.00256. Therefore, we find support for H2.
Next, we examined more closely the impact of signaling afforded by the dealers’ use of multi-party certification on dealers’ relative revenues (). Recall that dealers’ relative revenues are given by the relative difference between the car’s selling price and the car’s actual value: (p-v)/v.
Table 3. Dealers’ relative revenues
The results show an average relative revenue of 0.1866 in the instance with dealer signaling only and a lower average relative revenue of only 0.0429 in the instance with multi-party certification. In other words, the large positive average relative revenue achieved by dealers under dealer signaling only was reduced by 0.1437 under signaling with multi-party certification. The results of the single-population t-test yield a p-value of 0. Hence, we accept H2.1.
The buyers’ relative revenue, meanwhile, significantly increases in the instance where multi-party certification was available, as shown in . Recall that the buyers’ relative revenue is given by (v-p)/v.
Table 4. Buyers’ relative revenues
While under dealer signaling only, buyers’ average relative revenue is -0.1866, under signals sent when multi-party certification was available these negative rents are reduced to -0.04290. The results of the two-population t-test yield a p-value of 0.00213. Thus, we cannot reject H2.2.
Finally, we compare the proportion of peaches sold under dealer signaling only and under signaling where multi-party certification was available, and make the equivalent comparison for lemons. The results are presented in .
Table 5. The proportions of peaches and lemons sold
In Instance A, only 4 of the 52 cars offered on the market were purchased at a price below or equivalent to their real value, which classifies these cars as peaches. Moreover, 29 cars were sold at a price above their actual value, which classifies these cars as lemons. The remaining 17 cars were not sold at all, meaning no deal was reached. In Instance B, meanwhile, out of the 53 cars on offer 18 were sold as peaches whereas only 16 were sold as lemons. Regarding the proportion of lemons sold in each instance, there was a significant decrease in the proportion of lemons sold in Instance B compared to Instance A. Finally, the p-values (0.0011 for peaches and 0.0071 for lemons) that result from testing whether the proportions of cars sold are different between the instances are strongly significant. This leads us to accept H3.
Discussion
Many scholars have begun to investigate the use of blockchain technology as a way of collecting and maintaining data throughout a car’s life cycle [Citation43, Citation67] in order to provide trusted car history certificates to buyers and sellers [Citation7, Citation8].That research provides us with valuable insights into the technical foundations of how blockchain can be applied to the used-car market. While it is essential to have an in-depth understanding of the opportunities of the technical subsystem, it is just as important to understand how it unfolds in and impacts the users in the social subsystem and how this interaction shapes and is shaped by information [Citation53]. Viewing the central artifact as an interconnected system with its elements and subsystems allowed us to investigate what is at the core of IS research. Namely, how technology unfolds in a specific application context, and how it affects that context [Citation15]. This is also the crux of our research question, “How does multi-party certification impact the market for lemons?” Thus, within the framework of blockchain research [Citation31], our study can be positioned as follows: the research theme that we analyze is how a blockchain application unfolds in an application domain and how it impacts market outcomes; our specific application domain is that of the used-car market but is generalizable to other markets that suffer from asymmetric distribution of information; the key construct we analyze is the effectiveness of blockchain-enabled asset documentation for signaling; the outcome we are interested in is changes in market dynamics and social welfare; for this we use and add to the underlying theories of signaling, information asymmetry, and socio-technical information systems; and the applied research methods are economic modelling and experimental techniques.
In the following section, we discuss whether, and if so why, blockchain-based certification is required if dealers are to send signals of higher fit. Then, we discuss what the availability of multi-party certificates means for the market’s agents and for market outcomes. Based on these insights, at the end of each sub-section, we also present recommendations for practitioners working on the development of systems of this kind.
Blockchain-Based Multi-party Certification for Signals of Higher Fit
Our analysis shows us that, all else being equal, signaling afforded by a dealer’s use of multi-party certification is more informative and consequently provides a greater fit than signals sent by dealers only. The reason why signals sent when multi-party certification is available are more informative is threefold. First, due to the way in which multi-party certification systems are built, they are able to collect extensive, dispersed information from several stakeholders—information that is difficult for dealers to obtain today [Citation43]. Second, the use of blockchain’s tokenization feature ensures that the data collected from dispersed stakeholders can be trusted and traced, which ensures the provenance of this data without disclosing business-relevant specifics [Citation68], a feature deemed crucial for the efficient transmission of valuable information [Citation32, Citation34]. Third, multi-party certification counteracts the problem of single-party intermediary certifiers having no incentive to provide more information than the minimum required, which is the case in monopolistic market structures [Citation33]. The necessary incentives are generated by giving the different participants in the system control over their original tokenized data, which consequently allows them to monetize that data accordingly. While data could potentially be copied and resold outside the blockchain-based system, it would not hold the same value for users. In sum, these effects can be attributed to blockchain’s distributed database architecture, decentralized consensus mechanism, and immutable cryptographic logic, all of which allows multiple stakeholders to collect and maintain data from multiple sources and to create a novel, shared certification offering [Citation5, Citation59]. These stakeholders can include car-related businesses such as importers, road-traffic authorities, and insurers, but also private owners of vehicles that generate data during their use [Citation66, Citation67].
The three described effects illustrate how blockchain is a technology that enables the development of multi-party certification. It is important to note that the technology (blockchain in this particular case) can be used to generate incentives that trigger behaviors in the social system that reduce entropy and therefore increase the harmony of the system as a whole. The extent to which individual stakeholders will contribute to a shared car ledger—and consequently the level of the signal’s informativeness vis-à-vis the market—largely depends on the incentives set for them [Citation67]. However, even a minimal number of stakeholders providing only minimal information would result in dealers being able to send signals of higher fit.
Another reason why multi-party certification affords dealers the action potential to send signals of higher fit, is the difficulty of faking the information provided by multi-party certification. Since signals need to be difficult to fake in order to be effective [Citation57], information provided by multi-party certification is more trustworthy than that provided by a single dealer alone, who could also—potentially—lie. This increase in trustworthiness is, again, due to the technical design of blockchain-based certification (and therefore to an element of the technical subsystem), which makes it almost impossible to corrupt transactions [Citation54] and consequently to corrupt multi-party certificates. While false entries could—potentially—harm trust in such certificates, the adoption of mechanisms that incentivize truthful event reporting [Citation66] has been shown to counteract this risk. Further, blockchain’s transparency paradigm [Citation44] makes each piece of information that is part of the signal traceable, and therefore objectively verifiable [Citation32], a quality that is crucial for the efficient transmission of valuable information. This is yet another example of an interaction between subsystems: only those systems that provide adequate incentives for agents in the social subsystem to record their available information accurately will be able to generate useful signals.
To harness the promised benefits of blockchain-based systems at a large scale, signals need to be effective. Hence, it is fundamental that the agents recording the information to be stored in the blockchain (car owners, merchants, etc.) only record correct information. Thus, designers of multi-party certification need to apply proper incentive schemes to mitigate the risk of false event reporting, and offer traceability options to those receiving the signal.
In this study, and in line with [Citation67], we argue that blockchain is an enabler if applications are to achieve the goals of multiple stakeholders in the generation multi-party certificates. At this stage, one could, however, ask whether other technologies could do the same. The answer to that question is that blockchain is currently the only technology that—by its nature—makes possible decentralized collaboration between parties that do not trust each other [Citation69], and creates unique ownership over digital goods for data providers [Citation40], by doing so countering the current information strategies of intermediaries, who maximize their profits by providing minimal information [Citation39]. Any technology or mechanism that achieves the same goals would also be adequate for the generation of multi-party certificates.
The Economic Impacts of Multi-party Certification in the Market for Lemons
In order to analyze the market effects of multi-party certification we developed a simple economic model and tested the derived propositions by using a laboratory market game. Overall, our results show that the availability of multi-party certification and consequently the ability of dealers to send signals of higher fit leads to a significant reduction in information asymmetries and an increase in market fairness.
In our empirical analysis we observed the changing behavior of dealers, who demand lower initial asking prices when multi-party certification is available than those they demand in a market where multi-party certification is not available (H1). This behavior reduces the anchoring effect, which, today, leads to overpayment for cars purchased [Citation2]. Therefore, as theoretically proposed and empirically proven, the resulting sale prices are significantly lower in the market where multi-party certification is available compared to in the market where it is not (H2). From a market perspective, we can confirm the proposed benefit of trusted car data [Citation8]—that it results in average sale prices that are much closer to the actual values of the used cars concerned, also in the wholesale market. Further, we advance these insights by pointing out how this is achieved—namely, through decreasing the information gap (entropy) between buyers and sellers. As shown and calculated by our analytical model, a reduction of the entropy in the buyers’ and sellers’ subsystem occurs as a consequence of the increase in information availability. Sellers are thus prohibited from taking advantage of the anchoring effect. In our study, we observed an average deviation of sale prices from actual values of more than 18 percent where multi-party certification is not available; a figure that was reduced to only around 4 percent when multi-party certification was available. While our results indicate a slightly higher lemons penalty than that proposed by Blundell et al. [Citation13], one should note that we did not differentiate between the age of the cars available, and that for our experiment only a certain class of car was used.
Furthermore, analyzing in particular the situational changes for buyers and dealers we observe an increase in market fairness. This is explained, on the one hand, by a significant reduction in the profits that better-informed dealers make today (H2.1) and, on the other, by a significant reduction in buyers’ losses (H2.2). If we disregard information costs, this is a simple zero-sum game: the losses of one group of actors being the profits of the other. Additionally, we observe that significantly more peaches and significantly fewer lemons are sold in the market where multi-party certification is available. This, the outcome described by H3, can be explained by increased incentives for dealers of peaches to strike a deal given the promise of higher returns for their good-quality cars. In line with prior research [Citation3, Citation8], we argue that this observed result can be explained by an increased ability to signal a product’s quality.
One implication that we can derive from these market changes is that as information advantage is reduced, dealers will no longer have the incentive to intermediate the market, given reduced transaction prices and the consequently smaller profits that they can achieve. This reduction in information asymmetry reflects how the relationship between the technical and the social subsystems reaches a new equilibrium, which is induced by a new level of entropy. In a world where multi-party certification is not available, dealers are valuable for private sellers of rather good quality cars as they mediate the market [Citation3]. They enable sellers of good quality cars to achieve slightly larger margins than they would alone. If, however, we assume that multi-party certification is accessible also to private car sellers, following [Citation8] we argue that the incentive for private sellers of good quality cars to work through intermediating dealers will be reduced. Thus, it is only a matter of time before dealers raise for themselves the question of whether their current business model is still worthwhile.Footnote2
Another implication of the higher fit of signals afforded by the dealers’ use of multi-party certification is a reduction in the system’s entropy (explicitly modelled in the Model Section). This reduction in entropy occurs due to a reduction of the probability of extreme outcomes. Recall that when entropy is high, subsystems find innovative ways to reduce entropy such that creative outcomes happen [560, p. 11], which explains the currently observable surge in innovative proposals for mitigating the information asymmetries inherent in this type of market. Our analytical results show how the entropy of the system is reduced by the dealers’ use of multi-party certification. Our empirical results, meanwhile, depict how the technical subsystem affects the behavior of the agents in the social subsystem. Ultimately, the interaction of the technical subsystem and the social subsystem increases the harmony in the entire system due to the reduction in entropy induced by the ability of dealers to send signals of higher fit. Note that when social and technical subsystems are jointly optimized and in harmony, the entropy level is low [Citation15]. This higher degree of harmony (i.e., lower level of entropy) is reflected in the empirical results of this paper, which show why the (actually desirable) outcomes stated in our hypotheses cannot be rejected.
Finally, practitioners should note that signals of higher fit increase the social welfare of the economy and reduce the number of transactions resulting in highly unprofitable outcomes for some agents. As a result, agents who are concerned about significant (downside) risks from unprofitable outcomes (e.g., sellers of peaches, buyers) will benefit from the availability of such signals and enjoy higher relative revenue. The increased relative revenue leaves space for requiring a fee in exchange for accessing the signal. Such fees can help finance the initial development cost of new peer-to-peer systems that reduce information asymmetries inherent to certain markets. Put differently, the increased relative revenues generated by the signals of higher fit offer new opportunities for forward-looking practitioners who want to use information technology to establish and finance (decentralized) solutions that help solve problems associated with information asymmetries.
Conclusion
While the concepts of information asymmetries [Citation3] and signaling [Citation36,Citation57] have been widely discussed, their intrinsic problems remain. The latest developments in the field of information systems include a novel solution mitigant based on blockchain. In this paper, we take prior work a step further and show how the use of blockchain-based asset certification unfolds in practice, and empirically evaluate its impact on the market and its agents. Our results confirm that blockchain-based certificates enable dealers to send signals of higher fit than the signals they send today, leading to a reduction in information asymmetries by reducing the entropy of the overall system. This leads to a more efficient allocation of goods and increased market fairness. These results are relevant for scholars, practitioners, and policymakers.
For scholars, we advance the growing body of research into the economics of blockchain by shedding light on the phenomena that blockchain technology induces at the market and the agent level, all in the context of well-established IS and economics theories. This allows blockchain research to advance further, and to go beyond a mere technology or application potential standpoint. Further, our results establish the initial ground for studying blockchain in markets with asymmetric information. This may result in fruitful discussions that help scholars to broaden the theoretical basis of blockchain-based systems. Moreover, we show how to apply the analytical computation of a system’s entropy, something that might ease the path toward the broader use of this concept in IS research.
For practitioners, our results provide valuable insights and guidance concerning their blockchain development work. These can be especially relevant for designers of blockchain-based systems, as they allow them to consider and anticipate market participants’ strategic behavior and consequently sharpen future business models.
For policymakers, our results show how novel technology might provide solutions to some of the problems they are called to tackle. Fostering innovation, research, and regulation in the realm of blockchain technology (e.g., by providing subsidies [Citation16]) might help policymakers acquire new tools to better achieve their objectives of market fairness and transparency. And more broadly, as researchers and practitioners alike move beyond mere blockchain prototyping, society itself might benefit from the adoption of the technology.
Our research is not without its limitations, but these limitations themselves open avenues for further research. First, the economic model is fairly simple. We deliberately choose a parameterization of the model that allows for closed-form solutions. We represent the idea of a signal’s fit simply, by using different parameterizations for signals sent by dealers when multi-party certification is available and signals sent by dealers alone. Also, we make deliberately elementary choices for the utility and density functions. Yet to counteract these shortcomings and demonstrate generalizability, we additionally develop a different model, using a CARA utility function, which yields the same hypotheses. Further research could advance our work by adopting more complex modelling approaches.
Second, the sample size of our experiment, while not small by the standards of experimental economics, is not large enough to enable more in-depth analysis. In further research it would be interesting to study further the changes in the behavioral patterns of different agents caused by the adoption and use of the technology and by the phenomena it induces in the market. We also acknowledge that an experimental negotiation in a classroom setting is not the same as a real-life negotiation with (tens of) thousands of dollars at stake. However, the use of experiments in economics has been proven useful by many scholars before us [Citation26, Citation30, Citation52]. Our close relationship with industry experts ensured a design and setup that were as close as possible to reality.
Third, in future research it would be worthwhile exploring end users’ perceptions of the role of blockchain in product history certification.
Finally, since the realization of systems of this kind largely depends on whether or not enough money is available to finance their development and maintenance, we emphasize the need for future research that investigates how multi-party certification could be funded. Also, since the cost for the infrastructure would ultimately translate into transaction costs, a careful investigation of pricing options and their effects is vital.
Summarizing, we consider that our study can help design and implement multi-party certification that will enable sellers to send signals of higher fit. Eventually, such signals could result in a reduction of information asymmetry in a manner that would end up solving the market for lemons problem. The fact that this might be the case justifies the current study and future studies of this type of certification.
Supplemental Material
Download MS Word (42.3 KB)Acknowledgment
Cardossier is a collaborative effort of multiple partners from the automotive sector in Switzerland. We thank all Cardossier project members for their feedback and involvement during the design of our market game. Especially, we would like to thank AutoScout24 (one of Europe’s largest online platform providers for used-cars) for the right to use its layouts, and for access to data and expert car evaluations and its participation in and feedback on the pretests. We would also like to thank Auto-I dat (a leading supplier of car evaluations and studies) for its expert valuation of the used cars that were used during our experiment as well as for its participation in and feedback during the pretests. Last but not least, we would also like to thank AMAG, one of the largest car importers and retailers in Switzerland, for providing a standard assessment table that we used for car evaluation as, well as for valuable feedback on and input to the pretests.
Disclosure Statement
No potential conflict of interest was reported by the authors.
Supplementary Information
Supplemental data for this article can be accessed on the publisher’s website
Additional information
Funding
Notes on contributors
Ingrid Bauer
Ingrid Bauer ([email protected]; corresponding author) is a Ph.D. candidate and research assistant at the Information Management Research Group of the University of Zurich, Switzerland. Her research focuses on blockchain platforms and data-markets, and token systems. She holds a BSc in Information Engineering and Management from the Karlsruhe Institute of Technology and a MSc in Information Systems from the Copenhagen Business School. She led the industry collaboration aspect of this project.
José Parra-Moyano
José Parra-Moyano ([email protected]) is an assistant professor at the Department of Digitalization of the Copenhagen Business School. He holds a Ph.D. in Management and Economics from the University of Zurich. His research focuses on the management and economics of data and privacy, where blockchain technology plays a pivotal role.
Karl Schmedders
Karl Schmedders ([email protected]) is Professor of Finance at IMD, Lausanne, Switzerland. He holds a Ph.D. in Operations Research from Stanford University. His research focuses on quantitative methods in economics and finance. Dr. Schmedders applies numerical solution techniques to complex economic and financial models shedding light on relevant market issues and industry problems. He has published numerous research articles in international academic journals.
Gerhard Schwabe
Gerhard Schwabe ([email protected]) is a Professor in the Department of Informatics at the University of Zurich, Switzerland, where he leads the Information Management research group. He received his doctoral and postdoctoral education at the University of Hohenheim, Germany. He researches the intersection of collaborative technologies and information management. Dr. Schwabe has studied collaboration in commercial and government organizations at the granularity of dyads, small teams, large teams, organizations, communities, and social networks, frequently in collaboration with companies and public organizations. He has published numerous papers in major journals and conference proceedings, in Information Systems as well as in Computer Science.
Notes
1 The car was sold for USD 10 when the participant wrongly placed a decimal separator.
2 We acknowledge that there might be other reasons for private sellers to work through intermediating dealers, including market liquidity or efficiency. In the current study, however, we only focus on relative revenues.
References
- Adomavicius, G.; Bockstedt, J.C.; Curley, S.P.; and Zhang, J. Do recommender systems manipulate consumer preferences? A study of anchoring effects. Information Systems Research, 24, 4 (December 2013), 956–975.
- Adomavicius, G.; Gupta, A.; and Sanyal, P. Effect of information feedback on the outcomes and dynamics of multisourcing multiattribute procurement auctions. Journal of Management Information Systems, 28, 4 (April 2012), 199–230.
- Akerlof, G. The market for “lemons”: Quality uncertainty and the market mechanism. The Quarterly Journal of Economics, 84 (1970), 488–500.
- Allen, G.; and Parsons, J. Is query reuse potentially harmful? Anchoring and adjustment in adapting existing database queries. Information Systems Research, 21, 1 (March 2010), 56–77.
- Avital, M.; Beck, R.; King, J.; Rossi, M., and Teigland, R. Jumping on the blockchain bandwagon: Lessons of the past and outlook to the future. In International Conference on Information Systems, ICIS 2016. Dublin: Association for Information Systems. AIS Electronic Library (AISeL), 2016.
- Bauer, I.; Zavolokina, L.; Leisibach, F.; and Schwabe, G. Exploring blockchain value creation: The. case of the car ecosystem In Proceedings of the 52nd Annual Hawaii International Conference on System Sciences. Maui, Hawaii, 2019, p. 10.
- Bauer, I.; Zavolokina, L.; Leisibach, F.; and Schwabe, G. Value creation from a decentralized car ledger. Frontiers in Blockchain, 2, (January 2020).
- Bauer, I.; Zavolokina, L., and Schwabe, G. Is there a market for trusted car data? Electronic Markets, 30, 2 (September 2019), 211–225.
- Baumann, J.; Zavolokina, L., and Schwabe, G. Dealers of peaches and lemons: How can used car dealers use trusted car data to improve their value proposition? In 54th Hawaii International Conference on System Sciences,(HICSS) 2021. Kauai, Hawaii, USA, January 5: HICSS, 2021. doi:10.125/71278.
- Beck, R.; Avital, M.; Rossi, M.; and Thatcher, J.B. Blockchain technology in business and information systems research. Business & Information Systems Engineering, 59, 6 (December 2017), 381–384.
- Beggs, A.; and Graddy, K. Anchoring effects: Evidence from art auctions. American Economic Review, 99, 3 (May 2009), 1027–1039.
- Biglaiser, G. Middlemen as experts. The RAND Journal of Economics, 24, 2 (1993), 212–223.
- Blundell, R.; Gu, R.; Leth-Petersen, S.; Low, H.; and Meghir, C. Durables and lemons: Private information and the market for cars. US National Bureau of Economic Research,Working Paper Series, 26281 (2019).
- Bolton, G.; Loebbecke, C.; and Ockenfels, A. Does competition promote trust and trustworthiness in online trading? An experimental study. Journal of Management Information Systems, 25, 2 (September 2008), 145–170.
- Chatterjee, S.; Sarker, S.; Lee, M.J.; Xiao, X., and Elbanna, A. Apossible conceptualization of the information systems artifact: Ageneral systems theory perspective. Information Systems Journal, 31 4 (2020 December), 550–578.
- Cho, S.; Cheong, A.; No, W.G., and Vasarhelyi, M.A. Chain of values: Examining the economic impacts of blockchain on the value-added tax system. Journal of Management Information Systems, 38, 2 (2021), 282–287.
- Clemons, E.K.; and Thatcher, M.E. Evaluating alternative information regimes in the private health insurance industry: Managing the social cost of private information. Journal of Management Information Systems, 14, 2 (September 1997), 9–31.
- Connelly, B.L.; Certo, S.T.; Ireland, R.D.; and Reutzel, C.R. Signaling theory: A review and assessment. Journal of Management, 37, 1 (January 2011), 39–67.
- Dawson, G.S.; Watson, R.T.; and Boudreau, M.-C. Information asymmetry in information systems consulting: Toward a theory of relationship constraints. Journal of Management Information Systems, 27, 3 (December 2010), 143–178.
- Du, W. (Derek); Pan, S.L.; Leidner, D.E.; and Ying, W. Affordances, experimentation and actualization of FinTech: A blockchain implementation study. The Journal of Strategic Information Systems, 28, 1 (November 2018), 50–65.
- European Parliament. European Parliament vote is a significant step towards restoring consumer trust in the used car market and helping citizens save billions. 2018. https://citainsp.org/wp-content/uploads/2018/05/20180523-joint-press-release-Ertug-report-final-corr.pdf (accessed on December 06, 2019).
- Fridgen, G.; Lockl, J.; Radszuwill, S., and Rieger, A. A solution in search of a problem: A method for the development of blockchain use cases. In Proceedings of the 24th Americas Conference on Information Systems (AMCIS), 2018, p. 10.
- Glaser, F. Pervasive decentralisation of digital infrastructures: A framework for blockchain enabled system and use case analysis. In Proceedings of the 50th Hawaii International Conference on System Sciences. Waikoloa Village, Hawaii, January 4 - 7, January 2017.
- Glaser, F.; and Bezzenberger, L. Beyond cryptocurrencies - Ataxonomy of decentralized consensus systems. In Münster, Germany 23rd European conference on information systems (ECIS). Münster, Germany, 2015. https://ssrn.com/abstract=2605803
- Gomber, P.; Kauffman, R.J.; Parker, C.; and Weber, B.W. On the Fintech revolution: Interpreting the forces of innovation, disruption, and transformation in financial services. Journal of Management Information Systems, 35, 1 (January 2018), 220–265.
- Gupta, A.; Kannan, K.; and Sanyal, P. Economic experiments in information systems. MIS Quarterly: Management Information Systems, 42, 2 (2018), 595–606.
- Hashim, M.J.; Kannan, K.N.; Maximiano, S.; and Ulmer, J.R. Digital piracy, teens, and the source of advice: An experimental study. Journal of Management Information Systems, 31, 2 (October 2014), 211–244.
- Hawlitschek, F.; Notheisen, B.; and Teubner, T. The limits of trust-free systems: A literature review on blockchain technology and trust in the sharing economy. Electronic Commerce Research and Applications, 29, (May 2018), 50–63.
- Hoang, A.-P.; and Kauffman, R.J. Content sampling, household informedness, and the consumption of digital information goods. Journal of Management Information Systems, 35, 2 (April 2018), 575–609.
- Höst, M.; Regnell, B.; and Wholin, C. Using students as subjects - A comparative study of students and professionals in lead-time impact assessment. Empirical Software Engineering: An International Journal, 5, 3 (2000), 201–214.
- Kohli, R.; and Liang, T.-P. Special section: Strategic integration of blockchain technology into organizations. Journal of Management Information Systems, 38, 2 (2021), 8.
- Koutroumpis, P.; Leiponen, A.; and Thomas, L.D.W. Markets for data. Industrial and Corporate Change, 29, 3 (June 2020), 645–660.
- Lacity, M.C. Addressing key challenges to making enterprise blockchain applications a reality. MIS Quarterly Executive, 17, 3 (2018), 201–222.
- Lee, H.G. Aucnet: Electronic intermediary for used-car transactions. Electronic Markets, 7, 4 (1997), 24–28.
- Lee, H.G.; Westland, J.C.; and Hong, S. The impact of electronic marketplaces on product prices: An empirical study of AUCNET. International Journal of Electronic Commerce, 4, 2 (December 1999), 45–60.
- Levin, J. Information and the market for lemons. The RAND Journal of Economics, 32, 4 (2001), 657.
- Lindman, J.; Rossi, M.; and Virpi, K.T. Opportunities and risks of blockchain technologies in payments – A research agenda. In Proceedings of the 50th Hawaii International Conference on System Sciences, 2017. doi:10.24251/HICSS.2017.185.
- List, J.; Sadoff, S., and Wagner, M. So you want to run an experiment, now what? Some simple rules of thumb for optimal experimental design. VOX, CEPR Policy Portal, 2010. https://voxeu.org/article/simple-rules-designing-economic-experiments (accessed on December 02, 2019).
- Lizzeri, A. Information revelation and certification intermediaries. The RAND Journal of Economics, 30, 2 (1999), 214.
- Miscione, G.; Richter, C.; and Ziolkowski, R. Authenticating deeds/organizing society: Considerations for Blockchain-based Land Registries. In W. De Vries, ed., Responsible and Smart Land Management Interventions: An African Context. CRC Press: Taylor & Francis, 2020, 133–147.
- Mougayar, W. The Business Blockchain: Promise, Practice, and Application of the Next Internet Technology. Hoboken, NJ: Wiley, 2016.
- Nakamoto, S. Bitcoin: A peer-to-peer electronic cash system. (2008); 9. https://bitcoin.org/bitcoin.pdf
- Notheisen, B.; Cholewa, J.B.; and Shanmugam, A.P. Trading real-world assets on blockchain: An application of trust-free transaction systems in the market for lemons. Business & Information Systems Engineering, 59, 6 (December 2017), 425–440.
- Notheisen, B., and Weinhardt, C. The blockchain, plums, and lemons. SSRN Electronic Journal , Karlsruhe, KIT Working Paper in Economics No. 130, (2019). https://ssrn.com/abstract=3202667
- Ostern, N.K.; Rosemann, M., and Moormann, J. Determining the idiosyncrasy of blockchain: An affordances perspective. Forty-First International Conference on Information Systems. AIS eLibrary, 2020, pp. 18. https://aisel.aisnet.org/icis2020/blockchain_fintech/blockchain_fintech/4
- Parra-Moyano, J.; Schmedders, K.; and Pentland, A. What managers need to know about data exchanges. MIT Sloan Management Review, 61, 4 (2020), 39–44.
- Pavlou, P., and Dimoka, A. Understanding and mitigating product uncertainty in online auction marketplaces. In Industry Studies Conference Paper. 2008. https://ssrn.com/abstract=1135006
- Qureshi, I.; and Compeau, D. Assessing between-group differences in information systems research: A comparison of covariance- and component-based SEM. MIS Quarterly, 33, 1 (2009), 197–214.
- Rieger, A.; Guggenmos, F.; Lockl, J.; Fridgen, G.; and Urbach, N. Building a blockchain application that complies with the EU general data protection regulation. MIS Quarterly Executive, 18, 4 (December 2019), 263–279.
- Rosenman, R.E.; and Wilson, W.W. Quality differentials and prices: Are cherries lemons? The Journal of Industrial Economics, 39, 6 (December 1991), 649.
- Rossi, M.; Mueller-Bloch, C.; Thatcher, C.; Bennett, J.; and Beck, R. Blockchain research in information systems: Current trends and an inclusive future research agenda. Journal of the Association for Information Systems, 20, 9 (2019), 1388–1403.
- Roth, A.E. The economist as engineer: Game theory, experimentation, and computation as tools for design economics. Econometrica, 70, 4 (July 2002), 1341–1378.
- Sarker, S.; Chatterjee, S.; Xiao, X.; and Elbanna, A. The sociotechnical axis of cohesion for the IS discipline: Its historical legacy and its continued relevance. MIS Quarterly, 43, 3 (2019), 695–719.
- Sarker, S.; Henningsson, S.; Jensen, T., and Hedman, J. Blockchain as a strategy for combating corruption in global shipping: An interpretive case study. Journal of Management Information Systems, 38, 2 (2021), 338–373.
- Schallbruch, M.; Schweitzer, H.; and Wambach, A. Europa stutzt die Digitalkonzerne. Frankfurter Allgemeine Zeitung (FAZ), (2021). https://www.faz.net/aktuell/wirtschaft/europa-stutzt-die-digitalkonzerne-kampf-gegen-monopolstellungen-17158280.html
- Shannon, C.E. A Mathematical theory of communication. ACM SIGMOBILE Mobile Computing and Communications Review, 5, 1 (2001), 3–55.
- Spence, M. Job market signaling. The Quarterly Journal of Economics, 87, 3 (August 1973), 355.
- Stahl, K.; and Strausz, R. Certification and market transparency. Review of Economic Studies, 84, (2017), 1842–1868.
- Sun Yin, H.H.; Langenheldt, K.; Harlev, M.; Mukkamala, R.R.; and Vatrapu, R. Regulating cryptocurrencies: A supervised machine learning approach to de-anonymizing the bitcoin blockchain. Journal of Management Information Systems, 36, 1 (January 2019), 37–73.
- The Economist. The juicy market for lemons. Can you buy a good second-hand car? The Economist, (September 2019). https://www.economist.com/finance-and-economics/2019/09/26/can-you-buy-a-good-second-hand-car (accessed on December 22, 2019).
- Tung, Y.A.; and Marsden, J.R. Trading volumes with and without private information: A study using computerized market experiments. Journal of Management Information Systems, 17, 1 (June 2000), 31–57.
- Wilson, C. The nature of equilibrium in markets with adverse selection. The Bell Journal of Economics, 11, 1 (1980), 108.
- Wolinsky, A. Prices as signals of product quality. The Review of Economic Studies, 50, 4 (October 1983), 647.
- Zavolokina, L.; Miscione, G., and Schwabe, G. Buyers of “lemons”: How can a blockchain platform address buyers’ needs in the market for “lemons”? Electronic Markets, 30, 2 (December 2019), 227–239.
- Zavolokina, L.; Schlegel, M., and Schwabe, G. How can we reduce information asymmetries and enhance trust in “The Market for Lemons”? Information Systems and e-Business Management, 19, 3 (February 2020), 883–908.
- Zavolokina, L.; Tessone, C.J.; Spychiger, F., and Schwabe, G. Incentivizing data quality in blockchains for inter-organizational networks – Learning from the digital car dossier. In Thirty ninth International Conference on Information Systems. San Francisco, CA: AIS eLibrary, 2018, pp. 17.
- Zavolokina, L.; Ziolkowski, R.; Bauer, I.; and Schwabe, G. Management, governance, and value creation in a blockchain consortium. MIS Quarterly Executive, 19, 1 (March 2020), 1–17.
- Zhang, W.; Wei, C.P.; Jiang, Q.; Peng, C.H., and Zhao, J.L. Beyond the block: A novel blockchain-based technical model for long-term care insurance. Journal of Management Information Systems, 38, 2 (2021), 374–400.
- Ziolkowski, R.; Miscione, G.; and Schwabe, G. Decision Problems in blockchain governance: Old wine in new bottles or walking in someone else’s shoes? Journal of Management Information Systems, 37, 2 (April 2020), 316–348.
- Zwass, V. Editorial introduction. Journal of Management Information Systems, 38, 2 (2021), 277–281.