Abstract
We outline and test an aquatic plant sampling methodology designed to track changes in and make comparisons among lake plant communities over time. The method employs a systematic grid-based point-intercept sampling design with sampling resolution adjusted based on littoral area and lake shape. We applied this method in 72 Wisconsin lakes ranging from 6.5–245 ha in size, recording species presence–absence and depth at approximately 20,000 unique sample points. To assess how reductions in sampling effort might affect data quality, we used Monte Carlo simulations (100 iterations at each of 9 levels of sampling intensity) to reduce total lake sample points by 10% through 90% using a stratified random selection approach. Species accumulation curves were fit using the Michaelis-Menten 2-parameter formula for a hyperbola, and the predicted asymptote was similar to observed species richness. In a subset of lakes, oversampling (200% effort) did not yield significant increases in species richness. However, even a modest reduction (10–20%) in sampling effort affected species richness, while frequencies of occurrence of dominant species and estimations of percent littoral area and maximum depth of plant growth were less sensitive to sampling effort. In addition, we provide results of a power analysis for detecting changes in plant communities over time. Future applications of this protocol will provide information suitable for in-lake management and for assessing patterns in aquatic plant communities state-wide related to geographic region, hydrological characteristics, land use, invasive species and climate.
Human activity affects aquatic ecosystems both directly (e.g., habitat removal, species introductions) and indirectly (e.g., nutrient runoff, climate change). Recognition of these impacts and efforts to ameliorate their effects has resulted in an increased focus on monitoring, and now many institutions and organizations monitor the state and health of natural communities. To ensure that trends revealed by monitoring efforts are due to actual ecological changes rather than to differences in measurement or survey methods, protocols should be rigorously tested before implementation (CitationOakley et al. 2003) and then consistently applied. We present a critical evaluation of one method of sampling aquatic macrophytes in inland lakes. Specifically, we assessed the influence of sampling intensity on data quality to provide guidance for planning an efficient macrophyte monitoring program.
Aquatic macrophytes affect the structure, function and composition of entire aquatic communities (CitationScheffer 1998, CitationWetzel 2001). Macrophyte communities can be monitored to assess the effect of external and internal disturbances to lakes, track ecosystem change over time and determine appropriate usage guidelines and management goals. Thus, organizations acknowledge the utility of monitoring aquatic plant populations over the long term (CitationSteinbeck et al. 2005). Applications of monitoring data range widely, and so do the sampling protocols employed in data collection. Each method has advantages and disadvantages (CitationMadsen and Bloomfield 1993), and selections should be made carefully considering cost, resources and objectives. Noting the presence of a conspicuous invasive species forming a surface canopy, for instance, may be accomplished through simple search surveys designed considering the species' morphology, life cycle and habitat (CitationHayes et al. 2005); whereas monitoring the status and distribution of a rare species may require the use of more intensive multi-scale methods (CitationStohlgren et al. 1995, Citation1998).
Sampling in aquatic ecosystems presents a unique set of challenges to surveyors. Selecting an appropriate sampling method often involves a trade-off between data quality and cost. For example, in-water methods like quadrat-based diver-assisted biomass sampling produce data that are less variable and more accurate than sampling the same variables from a boat (CitationCapers 2000), but they are often too time- and effort-intensive to implement on a large scale (CitationMadsen and Bloomfield 1993, CitationBartodziej and Ludlow 1997). Terrestrial ecologists have been using point and line-intercept methods to perform rapid and cost-effective surveys for years (CitationLevy and Madden 1933, CitationCanfield 1941, CitationPoissonet et al. 1972), and because submersed plants are seldom visible at the water's surface, limnologists have adapted terrestrial point-intercept methods by employing a rake sampler to observe species under water (e.g., CitationMadsen 1999, CitationParsons et al. 2001, CitationCheruvelil et al. 2002, CitationWersal et al. 2007). These surveys are relatively inexpensive, but they cannot be used to estimate plant abundance (e.g., biomass, plant volume, cover; CitationDeppe and Lathrop 1992), which varies seasonally and interannually more than species presence–absence. However, incidence-based methods do tolerate more flexible survey timing, allowing surveys to be conducted and compared over the length of the growing season. Incidence data are also more sensitive to species diversity, and point-intercept methods are more readily adapted to large survey areas (CitationMadsen 1999, CitationDodd-Williams et al. 2008). We acknowledge the utility of point- and line-intercept methods for large-scale sampling of aquatic ecosystems and present a comprehensive investigation that we hope will guide implementation in the field.
We evaluated the application of a point-intercept survey design for monitoring aquatic plants. Grid-based point-intercept methods have been employed in recent studies (e.g., CitationParsons et al. 2001, CitationCheruvelil et al. 2002, CitationWersal et al. 2007, CitationDodd-Williams et al. 2008, CitationMadsen et al. 2008), but little information is available on sampling effort requirements. The level of sampling intensity significantly influences bias, precision and accuracy of species richness estimates (CitationBrose et al. 2003) and is one of the most important aspects to consider when designing a sampling protocol. Species richness sampled in one community over time can act as an effective barometer for local ecosystem change, but comparisons among populations or communities based on raw richness counts are specious at best unless their accumulation curves have reached a clear asymptote (CitationGotelli and Colwell 2001) or comparisons are made at the same point on the curve. Overall, to achieve objective interpretation of biological community data, estimates must be accompanied by known limits of accuracy and precision (CitationMarshall 1988). After sampling 72 Wisconsin lakes at relatively high sampling intensities (1.3–2 times that of other published studies; e.g., CitationMadsen 1999, CitationCheruvelil et al. 2002, CitationMadsen et al. 2008), we used a Monte Carlo statistical approach to develop a post-hoc simulation that allowed us to mimic in-field sampling of each lake at a series of reduced sampling intensities (e.g., CitationAndrew and Chen 1997, CitationGibbs and Melvin 1997, CitationStatzner et al. 1998, CitationBrose et al. 2003, CitationGraves 2004). We discuss the impact of sample size on estimated species richness, percent littoral area and maximum depth of colonization and report the variation in results that can be expected given varying effort. In addition, we present results of a power analysis for Pearson's chi-square statistical test to determine the number of sampling points needed to detect differences in plant community variables over time. We hope these analyses will help managers effectively plan monitoring programs given the resources at their disposal.
Materials and methods
Distribution of study lakes
As part of a statewide aquatic plant monitoring program, we randomly selected 72 Wisconsin lakes within a study design that balanced different lake types (seepage, headwater drainage and lowland drainage lakes, following CitationRiera et al. 2000) within the 3 lake-rich ecoregions of Wisconsin (CitationOmernik et al. 2000). Means and ranges of lake chemical and morphological parameters were recorded ().
Table 1 Chemical and morphological characteristics of 72 lakes in 3 lake-rich ecoregions in northern, central and southeastern Wisconsin.
Point-intercept sampling
Creating the sampling grid
Lakes were sampled from a boat using a point-intercept methodology (CitationHauxwell et al. 2010) from 25 May to 14 September 2005. The point-intercept method requires collecting species presence–absence data at each point on a predetermined grid. We know empirically that the number of species increases as more area is sampled (CitationMacArthur and Wilson 1967) and that more complex shorelines allow increased development of the littoral community (CitationWetzel 2001); therefore, we determined grid resolution based on the estimated littoral area and shape of the shoreline. The Wisconsin Department of Natural Resources maintains a lake database that includes an estimation of the amount of area deeper than 6 m, and because we could not calculate littoral area a priori, we used this percentage as a proxy for littoral area. We also used the standard limnological shoreline development factor (SDF, the ratio of the length of the shoreline to the circumference of a circle equal in area to that of the lake; Wetzel 2001) to scale point resolutions so that more complex lakes would be sampled more intensively. We made an arbitrary but pragmatic decision to set grid resolutions to allow an 80-ha lake of moderate shoreline complexity to be surveyed in 8 h. Using that as our starting point, we then scaled grids to produce more points on increasingly large and complex lakes. We scaled down the number of points used to represent very large systems by generating curvilinear functions to relate estimated littoral area (x, in ha) and number of sample points (y) for lakes of low (< 1.5), medium (1.5–2.99), and high (≥ 3) shoreline development factors. The equations were, respectively,
Survey methods
We used a Global Positioning System receiver to navigate to each point on the survey grid, where we used a double-headed rake on a 4.5-m pole to rake plants from a ∼ 0.75-m swath. We employed a similar rake attached to a rope to collect plants from sites deeper than 4.5 m. Plants were identified to species following Crow and Hellquist (Citation2000a, Citation2000b); species presence was recorded. From this dataset, we determined for each lake (1) species richness (S 100% ) as the total number of species found; (2) maximum depth of colonization (MDC) as the greatest depth at which vegetation was encountered; and (3) percent littoral area as the percentage of the total lake area represented by points occurring at depths equal to or more shallow than the maximum depth of colonization.
Evaluating sampling intensity
Doubling sampling effort
Because we can only accurately simulate reductions in sampling intensity, it was necessary to evaluate the adequacy of our starting level of effort. Therefore, we sampled one species-rich lake (S 100% above the 85th percentile of all lakes surveyed) in the Northern Lakes and Forests ecoregion and one species-poor lake (S 100% below the 15th percentile of all lakes surveyed) in the Southeastern Wisconsin Till Plains ecoregion on consecutive days using both the original sampling grid as well as a grid scaled to double the original intensity.
Estimating the asymptote of species accumulation curves
We constructed species accumulation curves for each lake based on the number of unique species observed as sample points accumulate using the vegan package in R (CitationOksanen et al. 2008, R Development Core Team 2008). Curves were averaged over 500 iterations of random-order point accumulation, and a 2-parameter function for a hyperbola based on the Michaelis-Menten equation for enzyme kinetics was used to fit the resulting mean species numbers. Parameters controlling the curve shape were determined using the Eadie-Hofstee transformation of the Michaelis-Menten equation and Raaijmakers' maximum likelihood estimators (CitationRaaijmakers 1987, CitationColwell and Coddington 1994, CitationLongino et al. 2002). One of the parameters of the resulting curve is the value of the horizontal asymptote (S max ), which serves as an estimate of the total species richness (S) for the sampled community (de Caprariis et al. 1976, CitationClench 1979, CitationColwell and Coddington 1994).
Simulated effort reduction
We designed a Monte Carlo simulation program to produce 100 simulated datasets per lake sampled for each of 9 levels of intensity ranging from 10 to 90% of the total effort invested (SAS v9.1). To best replicate field conditions and to avoid random elimination of large areas of a sampling grid, we used a stratified-random approach for point selection by directing the program to randomly select points to eliminate out of contiguous blocks of 10 points each. A statistical evaluation of the effect of the number of iterations revealed no benefit to increasing the number above 100 per level of intensity per lake. Overall, the simulation resulted in the creation of 900 simulated datasets for each of the 72 study lakes and 65,700 total sets of data for cross-region and cross-lake type analyses. For each group of 100 iterations, we determined median species richness, maximum depth of colonization and percent littoral area.
Detecting interannual change within a lake
To assist managers in selecting a sampling intensity most appropriate for detecting differences in plant community metrics in a lake from year to year, we evaluated the power of Pearson's chi-square test to detect change in repeated surveys. Presence-absence data are binomially distributed and can be compared using chi-square methods (CitationMadsen 1999) that allow one to state with a known level of probability whether the frequencies of occurrence observed on 2 sampling events (at time t 1 and t 2) are different. The magnitude of change that can be reliably detected depends largely on sample size. Thus, to calculate the minimum sample size (n) necessary to detect a statistically significant difference of a certain magnitude, we completed a power analysis for this test using
Results
Implementation of the point-intercept survey methodology resulted in the production of sampling grids with resolution ranging 25–70 m; approximately 20,000 total sites were sampled at a rate of 37 (+1.8 SE) points per hour with a range of 1.5–27.5 worker-hours spent per lake. Depending on shoreline complexity, our sampling regime resulted in the population of approximately 250–400 sampling points for a 50-ha shallow lake. We found a significant increase in species richness with log-transformed littoral area (F = 8.19,R 2 = 0.10,P = 0.005) but not with log-transformed shoreline complexity (F = 3.53,R 2 = 0.05,P = 0.06; ) with a large amount of variation unexplained by either predictor variable. Mean MDC in this study was 5 m (+0.82 SE), and we revealed concordance between estimated littoral area using the 6 m proxy and littoral area determined by field-sampled MDC (F = 234.4,R 2 = 0.88, P ≪ 0.001).
Figure 1 Species richness as estimated using the point-intercept method and 100% effort versus shoreline development factor (SDF) and littoral area for 72 lakes spanning 3 ecoregions in northern, central and southeastern Wisconsin.
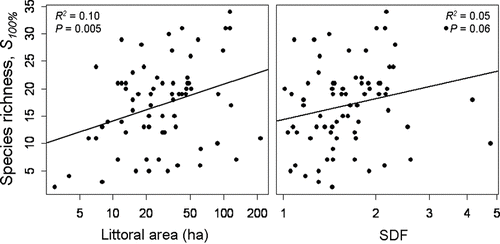
Species accumulation curves leveled in many of the systems sampled (); decreasing sampling intensity from 100 to 90% produced no change in species richness estimation in 94% of lakes. Doubling the sampling intensity had a negligible effect on observed species richness (one additional species and one fewer species discovered in the northern and southern lakes, respectively). When we used the Michaelis-Menten equation to predict the total number of species in the system (S max ) as a function of the number of sites considered, we found strong concurrence with field observations (R 2 = 0.96; P < 0.001, ); S max predictions for the 72 lakes deviated on average only 0.89 species from S 100%. Applying accumulation methods to the S max estimator revealed that predictions tended to stabilize as more sites were considered.
Figure 2 Species accumulation curves based on the mean number of unique species observed as sites are accumulated in random order. Means were calculated from 500 randomizations for each of 72 lakes in Wisconsin.
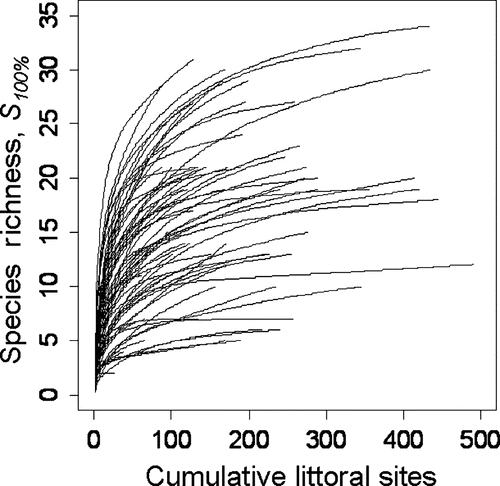
Figure 3 Relationship in 72 Wisconsin lakes between species richness estimated using the point-intercept method at 100% effort (S 100%) and species richness estimated by predicting the asymptote of the species-accumulation curve (S max ) calculated using the Eadie-Hofstee transformation of the Michaelis-Menten equation and Raaijmakers' maximum likelihood estimators.
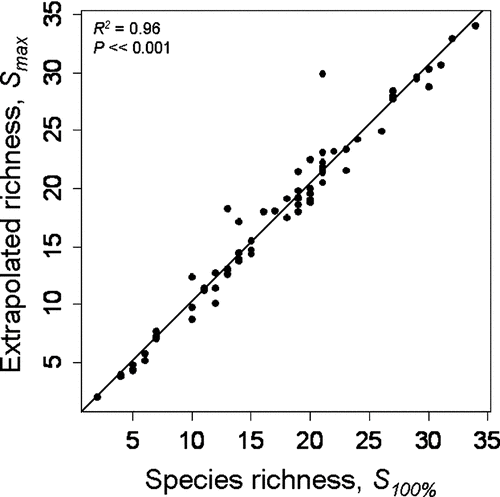
Stepwise reduction of sampling effort resulted in the underestimation of species richness values by progressively greater increments (), with coefficients of determination ranging from 0.81 to 0.99. Additionally, while the cost of surveying decreases linearly with reduced effort, the change in the accuracy of the species richness estimation is not as straightforward, with most rapid loss of species between ∼ 50 and 90% (). Reducing sampling intensity to 70% resulted in an average of one species per lake missed, and richness was underestimated in 70% of lakes. At 10% intensity, any given sampling effort would miss 8 species on average, ranging from 0 to 17 depending on the lake. Higher levels of sampling intensity tended to be more accurate and show less variability.
Figure 4 Mean from 100 iterations producing median species richness estimates from each of 72 Wisconsin lakes across sampling intensities (90% to 10%; S reduced ) versus species richness estimated using the point-intercept method at 100% effort (S 100% ). Also shown are R 2 values, the 1:1 line (solid) and the slope of the regression equation (dashed) relating S reduced and S 100% at each level of effort.
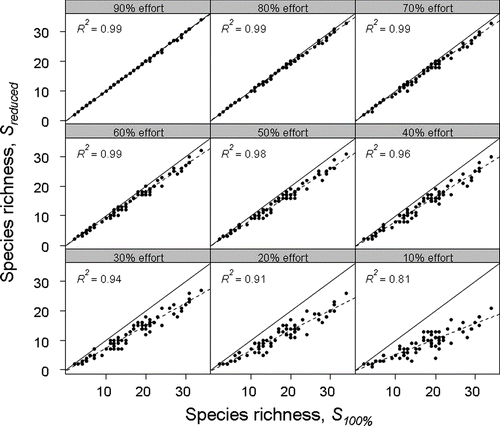
Figure 5 Data quality versus level of sampling intensity in the 72 Wisconsin study lakes. The proportion of lakes in which S reduced = S 100% is used to estimate data quality, where S reduced was calculated using Monte Carlo methods to simulate reduction in sampling effort, and S 100% was estimated using the point-intercept plant survey method at 100% effort.
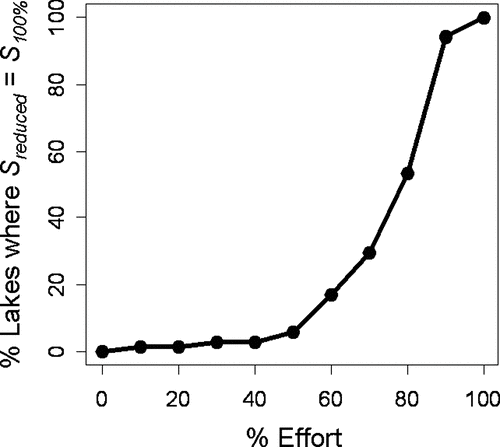
Estimations of percent littoral area and MDC were much less sensitive to sampling effort than was species richness. Both measurements yielded accurate estimates at 50–60% of original effort ().
Table 2 Effect of decreasing sampling effort of the point-intercept sampling method on estimation of mean parameters in 72 lakes in northern, central and southeastern Wisconsin. Mean species richness (S), maximum depth of colonization in meters (MDC), % littoral area, and frequency of occurrence of a typically abundant (Chara spp.) and a typically sparsely-distributed (Potamogeton oakesianus) species are shown as calculated from simulated datasets.
To determine the effect of reducing sample size on presence–absence data for individual species, we examined the impact of reducing sampling intensity on certain taxa distributed either widely or sparsely in a given lake. The frequencies of occurrence of common taxa (e.g., Chara spp.) were well preserved over the entire range of effort while populations of a sparsely distributed species (e.g., Potamogeton oakesianus) were overestimated as sampling effort decreased until around 50%, when it was most likely that any given survey attempt would fail to detect them entirely ().
We evaluated the effect of sampling intensity on species richness across lake types and ecoregions. The sampling intensity necessary to return species richness estimates equal to S 100% varied from region to region, but we observed no difference between seepage, headwater or lowland drainage lakes. One-way analysis of variance revealed a statistically significant regional influence (F = 4.05, P = 0.022) and pairwise Tukey HSD testing revealed a statistically significant difference in the effect of reducing sampling intensity between the Northern Lakes and Forests (mean species richness = 21, S 100% reached at mean 85% sampling intensity) and the Southeastern Wisconsin Till Plains regions (mean species richness = 14, S 100% reached at mean 73% sampling intensity). Species richness estimates from lakes across the state responded similarly to severe reductions in sampling size. Testing for multiple comparisons among regional slopes of the regression functions of 10% effort on those of 100% effort revealed no statistically significant differences (F = 0.96, P > 0.05).
Results of the power analysis indicate the number of sampling points required to have an 80% probability of detecting change of differing magnitudes for species occurrences of varying frequencies (). In general, results demonstrate wide variation in the number of points needed to detect changes of 10, 20 or 30%; depending on the frequency of occurrence of a species, detection of 30% change versus 10% change can require 3–10 times the number of sample points. For example, if a species in a hypothetical community is found at 10% of sampling points, the numbers of points needed to detect a real change in that species' distribution to 20, 30 or 40% frequency of occurrence are 198, 60 and 29, respectively. In addition, if a species is found at 50% frequency, the numbers of points necessary to detect a significant shift to 60, 70 or 80% frequency are 388, 91 and 36, respectively. Because reducing sampling effort changes the number of sample points, we can link the power analysis back to the Monte Carlo simulation to assess our ability to detect changes of differing magnitudes when effort varies. Reducing sampling intensity reduces ability to detect change in frequency of occurrence over time ().
Table 3 Hypothetical example linking chi-square power and Monte Carlo analyses. If we compare 2 hypothetical populations at times t 1 and t 2 where the initial frequency of occurrence at t 1 is 10% and increases to either 20, 30 or 40% at t 2, the number of sample points (n) returned at each level of sampling effort conveys the requisite power (standard level, β = 0.40, P′ = 0.80) to declare such changes statistically significant in the following percentage of the total 72 lakes sampled.
Discussion
Species accumulation curves leveled in 94% of lakes sampled, S max asymptotes estimated using the Michaelis-Menten equation strongly predicted observed species richness and S 100% and S 200% were similar, indicating that it is valid to make comparisons among lake plant communities despite variable sampling resolutions. However, caution should be exercised in equating the extrapolated S max with true total species richness. While certain studies have emphasized the Michaelis-Menten model's empirical accuracy and robustness (Citationde Caprariis et al. 1976, Citation1981), others have demonstrated its bias in heterogeneous environments (CitationKeating 1998). Yet, if species accumulation curves can be demonstrated to adequately represent uniform sampling of relatively homogenous environments, extrapolation to true species richness should still be possible (CitationColwell and Coddington 1994). Our use of presence–absence data may help dampen the effect of patchiness (following CitationLongino et al. 2002) and, indeed, when we distributed all species occurrences randomly over all points sampled, species accumulation curves were virtually identical to the mean accumulation curves (well within one standard deviation). In an attempt to further contextualize S max , we compared values to several other nonparametric species richness estimators including Chao 2, first- and second-order Jackknife and Bootstrap methods (CitationSmith and van Belle 1984, CitationChao 1987, CitationPalmer 1990) and found they were quite similar and highly correlated to S max (Mikulyuk, unpublished data). These estimates may possibly under- or over-predict total species richness, but a more detailed discussion is beyond the scope of this paper.
Lakes in this study supported more species when they had larger littoral zones, although there is a great deal of unexplained variation in this relationship. While the relationship between SDF is positive, we did not find it to be statistically significant, indicating that this factor may not be as important when setting sampling intensity. We have also shown that reducing sampling effort has a predictable effect on the estimation of aquatic plant community parameters and results in increased variability of those estimations. Although species richness estimates made based on only 10% of original sampling points are not usually accurate, they are predictable, indicating it might be possible to extrapolate actual species richness from the low-intensity estimate. Whereas accurate community surveys and complete species lists are very important for lake managers, it is possible that comparative studies may successfully employ the point-intercept method with greatly reduced effort. Additionally, although each parameter we examined increased in accuracy with increasing effort, the rate of the observed increase in accuracy varied. Species richness estimates are highly sensitive to increased sampling intensity, whereas those relating to depth require significantly less effort for accurate characterization.
Rare species are easily missed during sampling efforts, and the more intensively one samples, the more likely one is to detect them. Rarity describes the condition of having low abundance and/or small range size, and when measured based on the proportions of species abundances in an assemblage, rarity is a direct function of species richness (CitationGaston 1994). Consistent with this characterization, we observed a decrease in the accuracy of estimates at low sampling intensities more readily in diverse systems than in those with fewer species. Aquatic plant communities in the Northern Lakes and Forests region were more sensitive to reductions in effort than those in the Southeastern Wisconsin Till Plains, likely because they are more diverse. The fact that the degree of rarity is higher in the north helps explain the increased sensitivity to reduction in sampling intensity observed among northern lakes relative to southern lakes. Given this relationship, we can see that it is important to keep sampling intensity high when surveying diverse systems.
While having investigated empirically the relationship between sampling effort and the estimation of various community parameters, we find it useful to place this relationship in a practical context. By linking a power analysis comparing proportional shifts across sampling intensities to our Monte Carlo simulations, we can see that reducing sampling effort diminishes our ability to detect changes in frequency of occurrence. It is apparent that small shifts in proportions require rather large sample sizes to detect. Monitoring a population of native plants during chemical treatment of an invasive species, for instance, may require sampling at a level that would ensure the detection of small community shifts over time. General monitoring activities or comparison studies among lakes may preferentially look to document only large community shifts; these may successfully employ a reduced sampling intensity and still accomplish their objectives.
In summary, we reviewed a number of limitations imposed on data quality by reducing the sampling intensity of a point-intercept macrophyte sampling protocol. Understanding the relationship between sampling effort and the estimations produced will allow researchers and managers to plan monitoring strategies and effectively assess management actions. We hope that this study will aid in the design of large- and small-scale sampling programs that are cost-effective and efficient, while maximizing the quality of information collected for specific management or research objectives.
Acknowledgments
We wish to thank the Wisconsin Department of Natural Resources for providing funding for this project; Donna Perleberg of the Minnesota Department of Natural Resources, the WDNR aquatic plant management staff and the Wisconsin Lakes Partnership members for valuable input on protocol design; comments of the editor and three anonymous reviewers for input that greatly improved the manuscript; Kevin Bartz and Christina Caruso for formative guidance and Raffica LaRosa for her capable assistance in the field.
References
- Andrew , N L and Chen , Y . 1997 . Optimal sampling for estimating the size structure and mean size of abalone caught in a New South Wales fishery . Fish B-NOAA , 95 : 403 – 413 .
- Bartodziej , W and Ludlow , J . 1997 . Aquatic vegetation monitoring by natural resource agencies in the United States . Lake Reserv Manage , 13 ( 2 ) : 109 – 117 .
- Brose , U , Martinez , N D and Williams , R J . 2003 . Estimating species richness: sensitivity to sample coverage and insensitivity to spatial patterns . Ecology , 84 ( 9 ) : 2364 – 2377 .
- Canfield , R H . 1941 . Application of the line interception method in sampling range vegetation . J. Forest , 39 : 388 – 394 .
- Capers , R S . 2000 . A comparison of two sampling techniques in the study of submersed macrophyte richness and abundance . Aquat Bot , 68 : 87 – 92 .
- Chao , A . 1987 . Estimating the population size for capture-recapture data with unequal catchability . Biometrics , 43 ( 4 ) : 783 – 791 .
- Cheruvelil , K S , Soranno , P A , Madsen , J D and Roberson , M J . 2002 . Plant architecture and epiphytic macroinvertebrate communities: the role of an exotic dissected macrophyte . J N Am Benthol Soc , 21 ( 2 ) : 261 – 277 .
- Clench , H . 1979 . How to make regional lists of butterflies: Some thoughts . J Lepid Soc , 33 ( 4 ) : 216 – 231 .
- Colwell , R K and Coddington , J A . 1994 . Estimating terrestrial biodiversity through extrapolation . Philos T Roy Soc B , 345 ( 1311 ) : 101 – 118 .
- Crow , G E and Hellquist , C B . 2000a . Aquatic and wetland plants of northeastern North America. Volume I. Pteridophytes, gymnosperms and angiosperms: dicotyledons , Madison (WI) : University of Wisconsin Press .
- Crow , G E and Hellquist , C B . 2000b . Aquatic and wetland plants of northeastern North America. Volume 2. Angiosperms: monocotyledons , Madison (WI) : University of Wisconsin Press .
- de Caprariis , P , Lindemann , R H and Collins , C M . 1976 . A method for determining optimum sample size in species diversity studies . Math Geol , 8 ( 5 ) : 575 – 581 .
- de Caprariis , P , Lindemann , R H and Haimes , R . 1981 . A relationship between sample size and accuracy of species richness predictions . Math Geol , 13 ( 4 ) : 351 – 355 .
- Deppe , E R and Lathrop , R C . 1992 . A comparison of two rake sampling techniques for sampling aquatic macrophytes , Madison (WI) : Wisconsin Department of Natural Resources Research Findings . PUBL-RS-732
- Dodd-Williams , L , Dick , G O , Smart , R M and Owens , C S . 2008 . Point Intercept and Surface Observation GPS (SOG): A Comparison of Survey Methods—Lake Gaston, NC/VA , Vicksburg (MS) : Army Engineer Research and Development Center . http://el.erdc.usace.army.mil/elpubs/pdf/apcea-19.pdfTechnical note ERDC/TN APCRP-EA-19. Accessed 24 Sep 2009
- Gaston , K J . 1994 . Rarity , London (UK) : Chapman & Hall .
- Gibbs , J P and Melvin , S M . 1997 . Power to detect trends in waterbird abundance with call-response surveys . J Wildlife Manage , 61 : 1262 – 1268 .
- Gotelli , N J and Colwell , R K . 2001 . Quantifying biodiversity: procedures and pitfalls in the measurements and comparison of species richness . Ecol Lett , 4 : 379 – 391 .
- Graves , G A . 2004 . A Monte Carlo simulation of biotic metrices as a function of subsample size . J Freshwater Ecol , 19 ( 2 ) : 195 – 201 .
- Hauxwell , J , Knight , S , Wagner , K , Mikulyuk , A , Nault , M , Porzky , M and Chase , S . 2010 . Recommended baseline monitoring of aquatic plants in Wisconsin: Sampling design, field and laboratory procedures, data entry and analysis, and applications , Madison (WI) : Wisconsin Department of Natural Resources . Miscellaneous publication PUB-SS-1068 2010
- Hayes , K R , Cannon , R , Neil , K and Inglis , G . 2005 . Sensitivity and cost considerations for the detection and eradication of marine pests in ports . Mar Pollut Bull , 50 : 823 – 834 .
- Keating , K A . 1998 . Estimating species richness: the Michaelis-Menten model revisited . Oikos , 81 ( 2 ) : 411 – 416 .
- Levy , E B and Madden , E A . 1933 . The point method of pasture analysis . New Zeal J Agr. , 46 : 267 – 279 .
- Longino , J T , Coddington , J and Colwell , R K . 2002 . The ant fauna of a tropical rain forest: estimating species richness three different ways . Ecology , 83 ( 3 ) : 689 – 702 .
- MacArthur , R H and Wilson , E O . 1967 . The theory of island biogeography , Princeton (NJ) : Princeton Univ. Press .
- Madsen , J D . 1999 . Point intercept and line intercept methods for aquatic plant management , U. S. Vicksburg (MS) : Army Engineer Waterways Experiment Station . http://el.erdc.usace.army.mil/elpubs/pdf/apcmi-02.pdfAquatic Plant Control Technical Note MI-02. Accessed 24 Sep 2009
- Madsen , J D and Bloomfield , J A . 1993 . Aquatic vegetation quantification symposium: An overview . Lake Reserv Manage , 7 ( 2 ) : 137 – 140 .
- Madsen , J D , Stewart , R M , Getsinger , K D , Johnson , R L and Wersal , R M . 2008 . Aquatic plant communities in Waneta Lake and Lamoka Lake, New York . Northeast Nat , 15 ( 1 ) : 97 – 110 .
- Marshall , E . 1988 . Field-scale estimates of grass weed populations in arable land . Weed Res , 28 : 191 – 198 .
- Oakley , K L , Thomas , L P and Fancy , S G . 2003 . Guidelines for long-term monitoring protocols . Wildlife Soc B , 31 ( 4 ) : 1000 – 1003 .
- Oksanen , J , Kindt , R , Legendre , P , O'Hara , B , Simpson , G L , Solymos , P , Stevens , M HH and Wagner , H . 2008 . vegan: Community ecology package. R package version 1.15-0 http://cran.r-project.org/ http://vegan.r-forge.r-project.org/Accessed 11 March 2009
- Omernik , J M , Chapman , S H , Lillie , R A and Dumke , R T . 2000 . Ecoregions of Wisconsin . T Wisc Acad Sci , 88 : 77 – 103 .
- Palmer , M W . 1990 . The estimation of species richness by extrapolation . Ecology , 71 : 1195 – 1198 .
- Parsons , J K , Hamel , K S , Madsen , J D and Getsinger , K D . 2001 . The use of 2,4-D for selective control of an early infestation of Eurasian Watermilfoil in Loon Lake, Washington . J Aquat Plant Manage , 39 : 117 – 125 .
- Poissonet , P S , Daget , P M , Poissonet , J A and Long , G A . 1972 . Rapid point survey by bayonet blade . J Range Manage , 25 ( 4 ) : 313
- R Development Core Team . 2008 . R: A language and environment for statistical computing , Vienna : R Foundation for Statistical Computing . http://www.R-project.orgAccessed 16 September 2008
- Raaijmakers , J GW . 1987 . Statistical analysis of the Michaelis-Menten Equation . Biometrics , 43 : 793 – 803 .
- Riera , J L , Manguson , J J , Kratz , T K and Webster , K E . 2000 . A geomorphic template for the analysis of lake districts applied to the Northern Highland Lake District, Wisconsin, U. S. A. . Freshwater Biol , 43 : 301 – 318 .
- 2003 . SAS System for Windows, Version 9.1 , Cara (NC) : SAS Insitute Inc . SAS v9.1
- Scheffer , M . 1998 . Ecology of Shallow Lakes , London (UK) : Chapman & Hall .
- Smith , E P and van Belle , G . 1984 . Nonparametric estimation of species richness . Biometrics , 40 ( 1 ) : 119 – 129 .
- Snedecor , G W and Cochran , W G . 1967 . Statisical methods , Ames (IA) : Iowa State University Press .
- Statzner , B , Gore , J A and Resh , V H . 1998 . Monte Carlo simulations of benthic macroinvertebrate populations: estimates using random, stratified, and gradient sampling . J N Am Benthol Soc , 17 ( 3 ) : 324 – 337 .
- Steinbeck , J R , Schiel , D R and Foster , M S . 2005 . Detecting long-term change in complex communities: A case study from the rocky intertidal zone . Ecol Appl , 15 ( 5 ) : 1813 – 1832 .
- Stohlgren , T J , Bull , K A and Otsuki , Y . 1998 . Comparison of rangeland vegetation sampling techniques in the Central Grasslands . J Range Manage , 51 : 164 – 172 .
- Stohlgren , T J , Falkner , M B and Schell , L D . 1995 . A modified-Whittaker nested vegetation sampling method . Vegetatio , 117 : 113 – 121 .
- Wersal , R M , Madsen , J D and Tagert , M L . Littoral zone plant communities in the Ross Barnett Reservoir, MS . 37th Annual Mississippi Water Resources Conference, Jackson (MS): unpublished conference proceedings .
- Wetzel , R G . 2001 . Limnology, , 3 rd ed. , London (UK) : Academic Press .