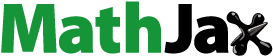
Abstract.
This article suggests a doubly robust method of estimating potential outcome means for multivariate fractional outcomes when the treatment of interest is unconfounded and can take more than two values. The method involves maximizing a propensity score weighted multinomial quasi-log-likelihood function with a multinomial logit conditional mean. We show that this estimator, which we call weighted multivariate fractional logit (wmflogit), consistently estimates the potential outcome means if either the propensity score model or the conditional mean model is misspecified. Our simulations demonstrate this double robustness property for the case of shares generated using a Dirichlet distribution. Finally, we advocate for the use of wmflogit by applying it to estimate time-use shares of women participating in the Mexican conditional cash transfer program, Progresa, using Stata’s fmlogit command developed by Buis.
1. Introduction
In applied work, it is common to encounter outcomes measured as shares or proportions from some total such as pension plan participation rates, expenditure shares, or proportion of time allocated to different household activities. This means that rather than observing a scalar outcome, one obtains a vector of shares that are each bounded in the unit interval and sum to one. Because of their restricted nature, researchers frequently use the multivariate fractional logit estimator (mflogit), proposed by Mullahy (Citation2015), for modeling the conditional mean of multivariate fractional outcomes.
When interest lies in causal inference, one drawback of the mflogit estimator is that consistent estimation of treatment effects (ATEs and ATTs), defined using unconditional potential outcome (PO) means, depends crucially on correct multinomial logit specification of the conditional mean. In this article, we relax this requirement by proposing a propensity score (PS) weighted multivariate fractional logit estimator (wmflogit) that is consistent for the PO means if either the multinomial logit mean or the PS model is correctly specified, but does not require both to be correct at the same time. This makes wmflogit doubly robust (DR) for the PO means.
Our framework considers the general case of multivalued treatments with more than two treatment levels. Such a generalization is useful in a variety of contexts, such as estimating returns to years of education (Uysal, Citation2015), evaluating impacts of different programs bundled together in some over-arching policy (Frölich, Citation2004), and even experiments (Negi and Wooldridge, Citation2020). We use this framework to describe the PO means for multivariate share data. An implication of estimating all PO means in a DR manner is that all pairwise treatment effects, corresponding to any two levels g and h, are also DR.
This article contributes to two different strands of the econometrics literature. The first is to multivariate fractional models, which have been employed frequently in modeling multiple share equations. Linear models rarely provide a good approximation to the conditional mean of share data. These neither guarantee predicted values in the desired range nor are able to account for other specialized features, such as observing outcomes on the boundary. In addition, these do not satisfy the adding up restriction with more than two outcome levels. For fractional outcomes that do not take values on the boundary, Papke and Wooldridge (Citation1996) discuss that a common practice is to model the conditional mean of the log-odds ratio as a linear function
The appeal of this formulation is that the log-odds has unbounded support, making a linear model for its conditional mean plausible. However, the conditional mean of the outcome, y, cannot be recovered from using the log-odds transformation without imposing additional distributional assumptions. Moreover, it requires ad-hoc adjustments when there are boundary values in the data.
In contrast, multivariate fractional logit provides a tractable functional form for the conditional mean of shares by accommodating their boundary and adding-up constraints. Papke and Wooldridge (Citation1996) were the first to propose fractional logit for a univariate response and Mullahy (Citation2015) and Murteira and Ramalho (Citation2016) generalized it to the case of multivariate fractional responses. Estimation of mflogit uses results from the linear exponential family (LEF) of distributions where an appropriate choice of quasi-log-likelihood (QLL) function delivers consistent parameter estimates. The key result assumes correct specification of the mean but allows the density associated with the QLL to be misspecified (Gourieroux et al., Citation1984).
This article is also related to the broad literature on DR estimators.Footnote1 For example, Cattaneo (Citation2010) and Ao et al. (Citation2021) propose non parametric doubly robust estimators for the ATE and ATT with multivalued treatments. More specifically, our article contributes directly to the literature that studies the DR property using QML with the LEF. This include Wooldridge (Citation2007), Sloczyński and Wooldridge (Citation2018), Uysal (Citation2015), and Negi (Citation2020). For instance, Wooldridge (Citation2007) studies inverse probability weighted M-estimators for missing data problems. One application of his approach is to the study of DR estimators for the average treatment effect which use QML estimation with specific mean functions. Our article is closest to the univariate analysis of Sloczyński and Wooldridge (Citation2018) and Uysal (Citation2015) in the sense that both these papers focus on DR estimators for treatment effect parameters under unconfoundedness using a multivalued treatment framework. The most salient difference between these papers and our article is that we are interested in multivariate fractional outcomes for which weighted linear regression, as advocated in Uysal (Citation2015), is not an attractive option. We recommend using wmflogit which is a more appropriate DR estimator given the restricted nature of the outcomes. It uses another property of quasi-maximum-likelihood (QML) estimation with the LEF which is that if the mean function is chosen to be the canonical link of an associated QLL, then one obtains consistent PO mean estimates despite the link function being misspecified for the conditional mean (see Wooldridge, Citation2007; Sloczyński and Wooldridge, Citation2018). In the case of wmflogit, one uses a multinomial logit mean specification embedded in a weighted multinomial QLL to obtain an estimator that is consistent for the PO means.
Our simulations demonstrate this double robustness property quite well for a 4×1 vector of shares generated using the Dirichlet distribution, where we consider three different treatment levels. We compare wmflogit to four other estimators, namely, linear regression adjustment (lra), inverse probability weighting (ipw), weighted linear regression adjustment (ipwlra), and mflogit. We look at four different cases of misspecification that can arise: correct mean with correct weights, misspecified mean with correct weights, correct mean with misspecified weights, and misspecified mean with misspecified weights. We find that wmflogit outperforms all others estimators in terms of root mean squared error (RMSE) in the vast majority of cases. To illustrate the method in empirical settings, we use wmflogit to estimate the average share of time spent on three different time-use activities by women who participated in the Mexican conditional cash transfer program, Progresa.
The rest of the article is as follows. Section 2 discusses the multivariate PO framework with applications to multinomial or fractional multinomial outcomes. Section 3 considers identification of treatment effects under unconfoundedness and discusses the main assumptions. Section 4 introduces the proposed wmflogit estimator and establishes the result on double robustness along with a discussion of its asymptotic properties. Section 5 talks about other estimators such as ipw, lra, and ipwlra as comparisons to wmflogit and why these are not attractive options when dealing with multivariate fractional outcomes. The same section also discusses the special case of a randomized treatment. Section 6 presents the results from a simulation exercise which generates a 4×1 vector of shares using the Dirichlet distribution. Section 7 applies wmflogit to estimating time use shares of women in Progresa, and Section 8 concludes.
2. Multivariate outcomes and multivalued treatments
Let 𝐘(g) be a J×1 vector of potential outcomes corresponding to treatment state such that
Examples include shares, proportions, or a binary choice from among J mutually exclusive alternatives. Then, one can define the unconditional PO means corresponding to the j-th alternative as
(1)
(1)
Also, let
(2)
(2)
be the PO mean for treatment state g among those receiving treatment h. We also let
denote the vector of binary treatment indicators such that
. The probability of being assigned to treatment group, g, is positive,
such that
. The observed multivariate fractional outcome can then be written as
(3)
(3)
We can then use (Equation1(1)
(1) ) and (Equation2
(2)
(2) ) to define pairwise average treatment effects for any two treatment levels
as
(4)
(4)
where τjgh is the alternative-j specific average treatment effect of treatment g relative to treatment h (ATEs) whereas τjhg|h is alternative-j specific average treatment effect of g relative to h among the sub population receiving treatment h, also known as ATTs. These alternative-specific treatment effects can be stacked to define the vector of ATEs or ATTs as
(5)
(5)
3. Unconfounded treatment
In many studies, a perfectly randomized treatment or intervention is hard to achieve. Let be a vector of observed pre-treatment covariates. In this article, we assume that the treatment is random once we condition on these covariates.Footnote2 Formally,
Assumption 1.
(Unconfounded Assignment). Assignment is independent of the potential outcomes conditional on the observed covariates.
Define to be the conditional mean of the g-th PO corresponding to the j-th alternative. Unconfoundedness allows us to recover or identify this conditional potential outcome mean as a function of the observed population vector
such that
Let mjg(𝐗, 𝜸g) be a parametric model for . This model is said to be correctly specified for the true mean if for some true parameter value,
,
.
Assumption 1 also implies that for each
(6)
(6)
such that,
where ρg(𝐗) is also known as the generalized PS (Imbens, Citation2000) for treatment level g.
Assumption 2.
(Overlap). ρg(𝐗) > 0 for all and for all 𝐱 in the support of 𝐗.
Assumption 2 also rules out ρg(𝐗) = 1 since the probabilities have to sum to one. Overlap is needed in addition to unconfoundedness to identify treatment effects. The assumption implies that for all values of 𝐗, the probability of receiving treatment level g is positive. This allows us to identify conditional average treatment effects which can then be averaged over the marginal distribution of 𝐗 to estimate 𝝉gh. For the case of estimating ATTs (or 𝝉hg|h), one can get by with a weaker version of overlap. In that case, we only need ρh(𝐗) < 1.
Assumption 3.
(Random Sampling). For a non random integer N,
(7)
(7)
is independent and identically distributed.
The above assumption restricts dependence between draws and assumes that we have access to a random sample from the population of interest. With standard regularity conditions, we can use i.i.d sequences of the random vector above to apply law of large numbers and central limit theorem.
Given unconfoundedness and overlap, consistent estimators of pairwise ATEs and ATTs can be obtained as
(8)
(8)
where the j-th element of
and
is obtained as
(9)
(9)
respectively, with
representing a consistent estimator of
and Nh denoting the total number of units receiving treatment level h in the sample.
The next section discusses the proposed weighted multivariate fractional logit estimators of 𝝁g and 𝝁g|h that are doubly robust for the PO means. These estimators involve models for both; the propensity scores and the conditional mean of the outcome, and remain consistent if either of the two models are misspecified.
4. Doubly robust weighted multinomial fractional logit
For each treatment level g, we choose the conditional mean function to be
(10)
(10)
where
,
, and αJg = 0 and 𝜷Jg = 𝟎 for
. Since we are dealing with outcomes that are binary or fractional in nature, using a multivariate fractional logit specification for the conditional mean ensures that fitted values lie in the unit interval since
and satisfies the adding-up constraint i.e.,
for each g. We will call this as the mflogit specification even though it applies to binary outcomes as well.
Also, let pg(𝐗, 𝜹) be a parametric model for the generalized propensity score for treatment level g which is said to be correctly specified if there exists some true parameter value, 𝜹0∈Δ, such that .
Then the proposed wmflogit estimator for μjg is obtained using (Equation9(9)
(9) ) where
solves a propensity score weighted multinomial QLL given byFootnote3
(11)
(11)
In the equation above, is the estimated PS obtained from solving a multinomial logit problem in a first-step.Footnote4 Similarly, for the case of μjg|h,
will solve
(12)
(12)
Essentially, wmflogit can be thought of as an inverse probability weighted regression adjustment (ipwra) estimator which uses the multinomial logit conditional mean. Later we will be comparing wmflogit to the ipwra estimator which assumes the conditional means to be linear. To emphasize it’s linear, we term this estimator ipwlra.
We formalize the double robustness result for the stacked vector of wmflogit PO mean estimators, and
, in the proposition below.
Proposition 1.
Assume 1, 2, and 3.
If the propensity score model is correctly specified i.e.
, then
where
’s solve the population version of the QLL given in (4.2) and (4.3), respectively.
If the mean model is correctly specified i.e
, then
where
and
’s solve the population version of the QLL given in (4.2) and (4.3), respectively.
The proof uses the first-order condition corresponding to the intercept in the population QLL and shows how wmflogit identifies 𝝁g and 𝝁g|h under both cases; when the propensity score model is misspecified and when the conditional mean model is misspecified.
The first half of double robustness implies that is consistent for 𝝁g even if we allow for the possibility that
as long as
. In contrast, the second half allows for the possibility that if
, then
is still consistent for PO means as long as
. In other words, when the mflogit model is correctly specified for the true conditional mean, weighting is not needed to guarantee consistent estimation of the unconditional PO means. However, when the mean is misspecified, a correctly specified propensity score model will guarantee consistent estimation of the PO means despite misspecification of 𝐦g(𝐗, 𝜸g). The resulting estimator of 𝝁g is then doubly robust for the corresponding population PO means and consequently,
will also be doubly robust for 𝝉g.
The double robustness result relies on estimation of using quasi-maximum likelihood in the linear exponential family (LEF) of distributions. We use features of LEF combined with particular mean functions to show that if one chooses a combination of canonical link and quasi log-likelihood functions carefully, then consistent estimation of PO mean is possible despite misspecification in the conditional mean function. As shown in Table 2 of Negi and Wooldridge (Citation2021), this choice depends on the nature of outcomes one wishes to study. For the case of multivariate fractional outcomes, the multinomial-logit canonical link and the multinomial QLL will guarantee this result. The next section uses the theory of two-step estimators to obtain the asymptotic variance of the wmflogit estimator of 𝝁g and 𝝁g|h.
4.1. Asymptotic results
Let be the (J−1) vector of scores (or first order conditions) corresponding to the wmflogit problem for
which solves (Equation11
(11)
(11) ) and
be the (G−1) vector of scores for the mflogit problem for estimating the propensity score parameter, 𝜹, where each of these scores are evaluated at the probability limits of
, respectively. Also, let
,
and
. Under standard regularity conditions, the influence function representation for
is
(13)
(13)
The influence function (IF) representation for is obtained from the wmflogit first-order conditionsFootnote5 given by
(14)
(14)
Using a mean value expansion around and using Eq. (Equation13
(13)
(13) ), we obtain
(15)
(15)
where
. Then, given that
is both consistent and asymptotically normal, consistency and normality of
follows from standard results in econometrics (see Wooldridge, Citation2010, chapter 12) where
Then stacking all such IF’s for all ,
Then, provided it exists and
where
is evaluated at
,
.
Let be the score of the wmflogit problem solved by
in (Equation12
(12)
(12) ),
, and
. The influence function representation for
is
(16)
(16)
where
. The IF representation for μjg|h is given as
where
. Again, stacking all the IF’s for
we get
Then provided it exists and
where
is evaluated at
,
. Consistency of the asymptotic variance estimator for
and
then follows from theorem 4.1 of Newey and McFadden (Citation1994).
5. Other estimators
This section discusses alternative estimators like propensity score weighting, linear regression adjustment, and weighted linear regression adjustment, which can be used for consistently estimating 𝝁g and 𝝁g|h. However, as we discuss below, these estimators are not attractive choices when dealing with multivariate fractional outcomes.
5.1. Weighting with propensity score
With unconfoundedness and overlap, a common strategy to estimate PO means is inverse propensity score weighting (ipw). Since ipw estimators do not involve models for the conditional mean, one can obtain these estimators directly using Lemma 3.2 of Sloczyński and Wooldridge (Citation2018) Footnote6 where
(17)
(17)
respectively, where
for all
. In other words, for propensity score weighting to produce consistent estimators of the PO means, it is necessary that the propensity score model is correctly specified. This is again restrictive, as it offers practitioners only one possibility of obtaining consistent PO mean estimates. As a practical matter, the estimators in (17) are limited because the weights do not sum to unity, although this is easy to fix (see Sloczyński and Wooldridge, Citation2018).
5.2. Linear regression adjustment
Another common approach is to use linear regression adjustment (lra) to model the PO means. In this case, one assumes the conditional means to be linear in parameters i.e.,
(18)
(18)
It is also useful to express the conditional means as where
are the population-demeaned covariates. This representation makes it clear how Eq. (Equation9
(9)
(9) ) would give us
and
if we estimate regressions
where
are the sample-demeaned covariates. We can then stack these estimates for all j to obtain
and
which can then consequently be plugged in Eq. (Equation8
(8)
(8) ) to get estimates of pairwise ATEs and ATTs.
It is important to note that the lra estimator of PO means will be consistent only if the conditional mean vector of the outcomes is truly linear in parameters. As we mention in the introduction, this is hard to justify for multivariate fractional responses. An issue with linear models is that they are rarely appropriate for fractional outcomes much like how linear probability models are ill-suited for studying binary outcomes. In addition, lra does not satisfy the adding-up constraint when G > 2. More importantly, since it relies on only one feature of the conditional distribution (the mean), it also does not share the double robustness property of wmflogit, thereby making it less desirable in the current setting.
5.3. Linear regression adjustment with weighting
A method that overcomes the shortcomings of PS weighting and linear regression adjustment is weighted linear regression adjustment (ipwlra). Much like lra, this estimator also assumes a linear conditional mean function. However, ipwlra estimators of and
solve propensity score weighted linear regressions
(19)
(19)
and
(20)
(20)
respectively, where
,
, and
,
is an estimator of ρg(𝐗). In principle, ipwlra is also robust to misspecification of the propensity score or the outcome model, as long as both are not misspecified at the same time. But because a linear functional form for the mean is rarely appropriate for fractional data, ipwlra is most likely going to rely on correct specification of the propensity scores to obtain a consistent linear approximation to the mean. In contrast, it makes more sense to think that wmflogit, which models the conditional mean as a multinomial logit, will provide a better approximation to the true conditional mean function and consequently has a better chance of being truly DR.
5.4. Randomized experiment
When the vector of treatment indicators, 𝐖, is randomized, 𝐖 is independent of – the vectors of potential outcomes and the covariates 𝐗. In this case, the subsample averages for each treatment level are both unbiased and consistent estimators of the PO means, 𝝁g (which are the same as the PO means conditional on different treatment levels by randomization). As shown by Negi and Wooldridge (Citation2020) when the potential outcomes 𝐘(g) are scalars, linear regression adjustment separately for each treatment preserves consistency – via a linear projection argument – and is generally asymptotically more efficient than the subsample averages if 𝐗 helps to predict the potential outcomes. Here, the potential outcomes 𝐘(g) are vectors, but it follows immediately from Negi and Wooldridge (Citation2020) that linear regression adjustment applied to each outcome Yj and treatment level g consistently estimates μjg and is generally more efficient than using the subsample averages. One unsatisfying feature of applying linear RA to multinomial or multivariate fractional outcomes is that the fitted values are not guaranteed to be in the unit interval and the adding-up restriction – that fitted values should sum to unity – will not hold. By contrast, the multinomial logit (or fractional logit) estimator will produce fitted values that satisfy the logical restrictions. Importantly, our double robustness result implies that the mflogit estimator is consistent under random assignment even if the conditional means are arbitrarily misspecified. The logic is simple: under random assignment, the weighted mflogit estimator is equivalent to the unweighted estimator because the propensity scores do not depend on 𝐗, and so using separate weighted estimation for each g is the same as using separate mflogit estimation for each g.
In the scalar outcome case, Negi and Wooldridge (Citation2020) show that if the conditional mean is correctly specified, then non linear regression adjustment that uses the canonical link function in the linear exponential family is asymptotically more efficient than linear regression adjustment. We will not show formally what happens in the current case where 𝐘(g) is a vector that satisfies adding-up restrictions. Practically, the important point is that, under random assignment, mflogit is very attractive because it is fully robust to conditional mean misspecification, ensures that fitted values are in the unit interval and sum to one, and is likely to be more efficient – perhaps substantially more efficient – than linear regression adjustment (which is in turn more efficient than subsample averages). See Negi and Wooldridge (Citation2020) for simulation evidence using logistic regression and Poisson regression with an exponential mean.
6. Monte Carlo simulations
This section studies the finite-sample bias and standard deviation of wmflogit in comparison to ipw, lra, ipwlra, and mflogit for estimating PO means of a 4×1 vector of shares (J = 4). These are generated through a Dirichlet distribution where the true conditional mean is multinomial with a quadratic index. We consider sample sizes of and three different treatment levels (G = 3).Footnote7 So,
where
is the outcome-vector of shares for treatment level,
. The population vector
is generated using a hundred thousand observations from which samples are drawn without replacement. The empirical distributions are then obtained from 1,000 monte carlo draws corresponding to each sample size. We study the behavior of wmflogit with respect to the other estimators under four realistic estimation scenarios: correct mean and weight, misspecified mean with correct weight, correct mean with misspecified weight, and misspecified mean and weight. These are enumerated in .
Table 1. Estimation scenarios for simulations.
Given that the true DGP generates share data using a Dirichlet distribution with multinomial mean, it is more appropriate to think that linear regression and weighted linear regression provide the best linear approximation for multivariate fractional outcomes. In that sense, lra will always be inconsistent for the PO mean vector, 𝝁g, whereas ipwlra can only be consistent if the PS model is correctly specified. The latter argument also holds for ipw which explicitly depends on correct weights for identification of the PO means given an unconfounded treatment. While mflogit specifies the mean correctly, it is not robust to misspecification of the mean function. Therefore, wmflogit is the estimator of choice and is an appropriate doubly robust estimator for multivariate fractional outcome means. The only exception is when both the mean and PS models are misspecified, in which case all estimators are equally inconsistent in theory.
6.1. Population
First, we consider two covariates where X1 is continuously distributed, whereas X2 is binary
where
and
is distributed bivariate normal with zero mean and variance-covariance matrix
To generate the vector of treatment assignments, 𝐖i, we use the following rule where the true propensity score is a multinomial logit with an index that includes square and interaction terms.
where
and
. Using these probabilities and a uniform random variable,
,
The outcome of shares 𝐘(g) for each of the three treatment levels follows Dirichlet distribution denoted by Dir(𝜼g) with parameters with
and
where we choose ϕg = 10. Given this parametrization,
(21)
(21)
where
and
. The parameter values for 𝜹g and 𝜸jg are given in and , respectively. Note that we normalize the outside option to 1 by setting the parameter values to 0. When estimating the wmflogit and mflogit estimators, misspecification of the mean and weights in corresponds to mis-specifying the index, 𝐙𝜷, which includes squares and interaction terms to instead be linear in X1 and X2. Meanwhile, the multinomial functional form for the PO mean and propensity score is kept intact. Also note that, given this DGP, the linear means are always misspecified.
Table 2. Parameter values, δ, indexing the PS model.
Table 3. Parameter values, γ, indexing the conditional mean model.
6.2. Discussion
report bias and RMSE of lra, ipw, ipwlra, mflogit, and wmflogit for μjg, and
for the four estimation scenarios listed in . The results from the simulation exercise line up with the theory. One can see that lra remains inconsistent for share means in all four cases. This bias does not diminish as sample size increases. For the cases where the weight is correctly specified (see and ), all weighted estimators are consistent, whereas all unweighted estimators are inconsistent. For the case where the weight is misspecified but mean is correct (), estimators that depend crucially on the PS such as ipw and ipwlra are inconsistent, whereas wmflogit which is doubly robust remains consistent. Finally, case 4 corresponds to all elements of the proposed estimator being misspecified. Despite being completely wrong, we do see that wmflogit has the lowest RMSE relative to all other estimators for most treatment levels g and categories j even though all estimators are inconsistent in theory. Therefore, this article advocates for wmflogit which is doubly robust for multivariate fractional outcomes and exhibits favorable finite sample behavior.
Table 4. Bias and RMSE for μjg when mean and weights are both correct.
Table 5. Bias and RMSE for μjg when mean is misspecified but weight is correct.
Table 6. Bias and RMSE for μjg when mean is correct but weight is misspecifed.
Table 7. Bias and RMSE for μjg when mean and weight are both misspecified.
7. Women’s time-use with Progresa
In this section, we use data from the Mexican conditional cash transfer program, Progresa, to construct time-use shares for women aged 15-65 years corresponding to three broad types of activities, namely, domestic work, farm work, and market work. Our objective here is to estimate the mean proportion of time spent by women using wmflogit in each of these activities depending upon their participation status in the program.
Progresa is a well-studied conditional cash transfer program which began in Mexico in 1997. The objective of the program was to encourage investment in human capital and reduce the transmission of poverty by offering cash assistance to women in poor Mexican households conditional on children’s school enrollment, school attendance, and visits to health clinics.
Eligibility for the program was determined in two phases where the first phase of the study used a deprivation index to determine which localities or communities in Mexico were highly deprived. A second phase of the program collected data on socioeconomic characteristics of households identified in the first phase using the Survey of Household Socio-Economic Characteristics (ENCASEH survey). Each locality selected in this phase was then randomly allocated to the intervention or control group. A subsequent poverty index was used to determine eligibility at the household level and those that were eligible received program benefits in the intervention localities.
We use the Evaluation Survey of Progresa (ENCEL surveys) which is a panel dataset with information on all rounds of data collection before and after Progresa was implemented. This panel is used to identify the baseline survey (round one) along with the time-use survey (round seven) to construct the final dataset used in this empirical study. Even though the original sample contains 24,077 households (142,697 observations), we restrict our analysis to households that have complete information on time-use shares and covariates for women between ages 15-65 years. This helps to ensure comparability of estimates across the different regression specifications.
We use this sample to study the effect of being a beneficiary or non beneficiary household on the time-use of women. To construct the dummy variable indicating the participation status of a given household, we interact the treatment-control locality dummy, contba, along with the dummy for being poor, pobre. This is important since only eligible households in the intervention localities were allowed to be Progresa participants.
The time-use shares for these women are then calculated by classifying 16 different types of activities (see ) recorded in the time-use module of Progresa into three broad categories, namely, domestic work, farm work, and market work.Footnote8 Shares are then calculated by dividing time spent on each category by the total time spent in all categories.
Table 8. Types of activity in Progresa’s time-use module.
The share equation in each type of regression controls for both individual level characteristics such as age, education, relationship with the head, and household level factors like household size, assets (such as owning a television, radio, refrigerator and other consumer durables), availability of electricity, poverty index, total agricultural land, number of dependents, head’s gender and education. The PS is estimated as a logit which controls for the marginalization indexFootnote9 and characteristics that were used to determine households’ poverty status in order to be eligible to receive program benefits. The latter includes running water, electricity, ownership of durable goods, animals and land, number of dependents, and presence of disabled individuals (Parker and Skoufias, Citation2000; Schultz, Citation2004).
We then use lra, ipw, ipwlra, mflogit, and wmflogit to estimate PO means for beneficiary and non beneficiary households (see ). The proposed estimator is implemented by using Stata’s fmlogit command developed by Buis (Citation2008). These PO means are then used to estimate the effect of Progresa on time spent by women in each category of work. reports the average treatment effect estimates for Progressa beneficiaries using the proposed wmflogit and the other above mentioned alternatives. We find that the difference in time spent on domestic work between beneficiary and non beneficiary households is marginally insignificant when using wmflogit and ipwlra but significant if one uses ipw and mflogit. Similary, the difference in time spent on market work is highly insignificant when using wmflogit and ipwlra but significant if one believes lra, ipw, and mflogit. However, for the case of farm activity, the difference is highly significant with wmflogit and insignificant if one believes the other estimators. The fact that wmflogit is doubly robust should engender more confidence in the results obtained from it compared to the other estimators considered.
Table 9. Estimate of potential time-use share means (PO means) by intervention group.
Table 10. Average treatment effect estimates.
8. Conclusion
This article proposes a doubly robust estimator for estimating the vector of potential outcome means for outcomes that are expressed as a vector of mutually exclusive and exhaustive binary outcomes, shares, or proportions. Our framework allows for multivalued treatments, which means that all pairwise treatments effects are also estimated in a doubly robust manner. The estimator is a propensity score weighted multivariate fractional logit, termed wmflogit, which uses features of the linear exponential family of distributions to guarantee robustness to misspecification of either the propensity score model or the multivariate fractional logit mean as long as both are not misspecified at the same time. A practical advantage of this estimator is that it only requires one of the features (either the conditional mean or the propensity score) to be correctly specified thereby offering practitioners some hope of obtaining valid treatment effect estimates even in the face of uncertainty about the accuracy of model specification.
Our simulations illustrate the double robustness property quite well. We generate a 4×1 vector of shares from a Dirichlet distribution for three treatment levels. On comparing wmflogit with linear, weighted linear, inverse probability weighted, and unweighted multivariate fractional logit (mflogit) regressions, we find that the RMSE associated with wmflogit declines as sample size increases in all cases including the one where mean and weights are both incorrect. For mflogit, this happens only when the mean model is correctly specified. Linear regression is never consistent and that is easily seen in the simulations where the finite sample bias persists even as the sample size increases. Weighted linear regression adjustment (ipwlra) has an RMSE comparable to wmflogit only when the weight is correct. Since the mean will rarely be well represented by a linear function for multivariate fractional outcomes, the consistency argument for ipwlra rests entirely on correct weight. A similar argument holds for ipw. The only procedure that has a real chance at being doubly robust is wmflogit and this is easily observed in the simulations.
An application to time-use shares of women from the Mexican conditional cash transfer program, Progresa, also shows that the significance of ATE estimates is different when using wmflogit vs. when using other linear and unweighted alternatives. Given that wmflogit is robust to inconsistency in two directions, it should invoke more confidence in the results obtained from wmflogit compared to other alternatives when explaining multivariate fractional outcomes.
Acknowledgments
We would like to thank Dalia Ghanem and Karen del Mar Ortiz Becerra for providing us the Progresa dataset.
Disclosure statement
No potential conflict of interest was reported by the author(s).
Notes
1. A version, known as AIPW, was proposed and studied in a string of papers by Robins et al. (Citation1994), Robins et al. (Citation2000), and Scharfstein et al. (Citation1999). More recently, improved versions of the AIPW estimator have been proposed in both the missing data and causal inference literatures.
2. Including pre-treatment outcomes in the covariate vector makes unconfoundedness even more plausible as an identifying assumption. As in the case of Hirano and Imbens (Citation2001), unconfoundedness is also easy to justify when we have a rich set of controls in the data that make it approximately true.
3. This QLL can be obtained by applying the general result on weighting given in Lemma 3.2 of Sloczyński and Wooldridge (Citation2018) to the multinomial QLL function.
4. As mentioned in Imbens (Citation2000), one may estimate the propensity scores using discrete response models if there is no natural ordering among the treatment levels or ordered response models if there is a natural ordering to the alternatives.
5. See section A for a derivation of the first-order conditions for the wmflogit estimator.
6. Similar equations for the ATE and the ATT can also be found in Hirano et al. (Citation2003) for the binary case.
7. These sample sizes ensure that we have enough variation in the different share categories across the three treatment levels.
8. Two school related activities, namely, min_esc and min_tar are not used in construction of any of the three activities.
9. Progresa used marginalization index to geographically target highly marginalized localities in the seven states of Mexico. These localities were then randomized to either be in the intervention or control group.
References
- Ao, W., Calonico, S., Lee, Y.-Y. ( 2021). Multivalued treatments and decomposition analysis: An application to the WIA program. Journal of Business & Economic Statistics 39(1):358–371. doi:10.1080/07350015.2019.1660664
- Buis, M. L. ( 2008). FMLOGIT: Stata module fitting a fractional multinomial logit model by quasi maximum likelihood. Statistical Software Components: n. pag.
- Cattaneo, M. D. ( 2010). Efficient semiparametric estimation of multi-valued treatment effects under ignorability. Journal of Econometrics 155(2):138–154. doi:10.1016/j.jeconom.2009.09.023
- Frölich, M. ( 2004). Programme evaluation with multiple treatments. Journal of Economic Surveys 18(2):181–224. doi:10.1111/j.0950-0804.2004.00001.x
- Gourieroux, C., Monfort, A., Trognon, A. ( 1984). Pseudo maximum likelihood methods: Theory. Econometrica 52(3):681. doi:10.2307/1913471
- Hirano, K., Imbens, G. W. ( 2001). Estimation of causal effects using propensity scoreweighting: An application to data on right heart catheterization. Health Services and Outcomes Research Methodology 2(3/4):259–278. doi:10.1023/A:1020371312283.
- Hirano, K., Imbens, G. W., Ridder, G. ( 2003). Efficient estimation of average treatment effects using the estimated propensity score. Econometrica 71(4):1161–1189. doi:10.1111/1468-0262.00442
- Imbens, G. ( 2000). The role of the propensity score in estimating dose-response functions. Biometrika 87(3):706–710. doi:10.1093/biomet/87.3.706
- Mullahy, J. ( 2015). Multivariate fractional regression estimation of econometric sharemodels. Journal of Econometric Methods 4(1):71–100. doi:10.1515/jem-2012-000630079291.
- Murteira, J. M. R., Ramalho, J. J. S. ( 2016). Regression analysis of multivariate fractional data. Econometric Reviews 35(4):515–552. doi:10.1080/07474938.2013.806849
- Negi, A. ( 2020). Doubly weighted M-estimation for nonrandom assignment and missing outcomes, Journal of Causal Inference, in press. arXiv:2011.11485.
- Negi, A., Wooldridge, J. M. ( 2020). Robust and efficient estimation of potential outcome means under random assignment, arXiv preprint, arXiv:2010.01800.
- Negi, A., Wooldridge, J. M. ( 2021). Revisiting regression adjustment in experiments with heterogeneous treatment effects. Econometric Reviews 40:504–534.
- Newey, W. K., Mcfadden, D. ( 1994). Large sample estimation and hypothesis testing. In: Robert Engle and Daniel McFadden, eds., Handbook of Econometrics, Vol. 4, pp. 2111–2245. Elsevier Science.
- Papke, L. E., Wooldridge, J. M. ( 1996). Econometric methods for fractional response variables with an application to 401(k) plan participation rates. Journal of Applied Econometrics 11(6):619–632.
- Parker, S. W., Skoufias, E. ( 2000). The impact of Progresa on work, leisure and time allocation: Final report, Techical report no. 600-2016-40136. Washington, D.C.: International Food Policy Research Institute.
- Robins, J. M., Rotnitzky, A., Van Der Laan, M. ( 2000). Comment. Journal of the American Statistical Association 95(450):477–482. doi:10.1080/01621459.2000.10474224
- Robins, J. M., Rotnitzky, A., Zhao, L. P. ( 1994). Estimation of regression coefficients when some regressors are not always observed. Journal of the American Statistical Association 89(427):846–866. doi:10.1080/01621459.1994.10476818
- Scharfstein, D., Rotnitzky, A., Robins, J. ( 1999). Comments and rejoinder. Journal of the American Statistical Association 94:1121–1146.
- Schultz, T. P. ( 2004). enquoteSchool subsidies for the poor: Evaluating the mexican progresa poverty program. Journal of Development Economics, 74:199–250.
- Słoczyński, T., Wooldridge, J. M. ( 2018). A general double robustness result for estimating average treatment effects. Econometric Theory 34(1):112–133. doi:10.1017/S0266466617000056
- Uysal, S. D. ( 2015). Doubly robust estimation of causal effects with multivalued treatments: An application to the returns to schooling. Journal of Applied Econometrics 30(5):763–786. doi:10.1002/jae.2386
- Wooldridge, J. M. ( 2007). Inverse probability weighted estimation for general missing data problems. Journal of Econometrics 141(2):1281–1301. doi:10.1016/j.jeconom.2007.02.002
- Wooldridge, J. M. ( 2010). Econometric Analysis of Cross Section and Panel Data. Cambridge, Massachusetts: MIT press.
Appendix A
first order conditions for weighted multivariate fractional logit
The first-order conditions for the weighted multinomial logistic regression can be derived by considering the following quasi maximum likelihood problem
Then, the multinomial log likelihood function is given as
(A.1)
(A.1)
Now differentiating the above with respect to gives us the following vector of first-order conditions
where
and for each
(A.2)
(A.2)
If we stack first order conditions corresponding to all the intercepts and slopes separately, one may write them compactly as
(A.3)
(A.3)
(A.4)
(A.4)
Similarly, the first-order conditions corresponding to which solves (Equation12
(12)
(12) ) can be stacked to obtain
(A.5)
(A.5)
(A.6)
(A.6)
Appendix B
Proofs
Proof of Proposition 1.
Case 1: When the propensity score model is correctly specified: In this case, the population analogue of the sample first-order conditions corresponding to the intercept in (EquationA.3(A.3)
(A.3) ) is given by
(B.1)
(B.1)
where
. The above can be rearranged to give us
(B.2)
(B.2)
Using (Equation2.3(3)
(3) ) we know that for each g,
Rewriting the left hand side of Eq. (EquationB.2(B.2)
(B.2) ),
Using law of iterated expectations, we can rewrite
Using iterated expectations again,
Similarly, rewrite the right hand side of Eq. (EquationB.2(B.2)
(B.2) ),
Therefore, combining the two results we get
(B.3)
(B.3)
Part 2: For 𝝁g|h, the population first-order conditions for the intercept are given by
(B.4)
(B.4)
which can again be re-arranged to give us
Consider the left hand side,
Now,
(B.5)
(B.5)
Now let’s consider the right hand side,
(B.6)
(B.6)
Now, comparing Eqs. (EquationB.5(B.5)
(B.5) ) and (EquationB.6
(B.6)
(B.6) ),
Case 2: When the mean model is correctly specified
In this case, the population first order conditions are given by
(B.7)
(B.7)
where
may or may not be equal to the true propensity score parameter, 𝜹0. Rewriting the left hand side of eq (EquationB.7
(B.7)
(B.7) ) and using the fact that for each g,
we get
(B.8)
(B.8)
Using law of iterated expectations,
Using iterated expectations again,
(B.9)
(B.9)
Therefore, combining Eq. (EquationB.9(B.5)
(B.5) ) with (EquationB.8
(B.8)
(B.8) ), we get
(B.10)
(B.10)
Since the above will be true only when
or in order words, when the mflogit mean is correctly specified.
Part 2: For 𝝁g|h, consider the population first-order conditions given by
(B.11)
(B.11)
Again, because of ,
(B.12)
(B.12)
where the second equality uses iterated expectations, fourth equality uses unconfoundedness, and the last equality uses a second application of iteration expectations. Given that
, Eqs. (EquationB.11
(B.11)
(B.11) ) and (EquationB.12
(B.12)
(B.12) ) implies that
(B.13)
(B.13)
only when the multinomial logit mean is correctly specified. ▪