Abstract
Background: Saliva represents a promising non-invasive source of novel biomarkers for diagnosis and prognosis cancer. This meta-analysis evaluates the diagnostic value of salivary biomarkers for detection of malignant non-oral tumours to better define the value of saliva as an alternative liquid biopsy.
Materials and methods: We performed a systematic review and meta-analysis. PubMed, Embase, LILACS and the Cochrane Library were searched to identify articles that examined the potential of salivary biomarkers for the diagnosis of malignant non-oral tumours. To assess the overall accuracy, we calculated the diagnostic odds ratio (DOR), area under hierarchical summary receiver operating characteristic (AUC) curve, sensitivity, specificity, positive likelihood ratio (PLR) and negative likelihood ratio (NLR) using a random- or fixed-effects model. Heterogeneity and publication bias were assessed. Statistical tests were two-sided.
Results: One hundred fifty-five study units from 29 articles with 11,153 subjects were included. The pooled sensitivity, specificity, PLR, NLR, DOR and AUC were 0.76 (95% confidence intervals (CI), 0.74–0.77), 0.76 (95% CI, 0.75–0.77), 3.22 (95% CI, 2.92–3.55), 0.31 (95% CI, 0.28–0.34), 13.42 (95% CI, 12.28–15.96) and 0.85 (95% CI, 0.84–0.87), respectively.
Conclusion: Salivary biomarkers may be potentially used for non-invasive diagnosis of malignant non-oral tumours.
This meta-analysis evaluates the diagnostic value of salivary biomarkers for detection of malignant non-oral tumours to better define the role of saliva as an alternative liquid biopsy.
Salivary biomarkers showed 85% accuracy for cancer distant to the oral cavity.
Saliva represents a promising non-invasive source of novel biomarkers in cancer.
Key messages
1. Introduction
Nowadays cancer represents one of the leading causes of morbidity and mortality worldwide, being the second leading cause of death globally. In 2015, cancer was responsible for 8.8 million of deaths and in the next two decades the number of new cases is expected to rise by about 70%. It is widely known that early detection of cancer benefits the patient, increasing the opportunity of an efficient treatment [Citation1], therefore it is critical to promote non-invasive strategies which allow an early cancer diagnosis [Citation2].
During the last years, liquid biopsy approaches have aroused great interest for early cancer detection, prediction of recurrent disease and assessment of therapeutic resistance mechanisms [Citation3]. Liquid biopsy represents an attractive, novel and minimally invasive source of tumour derived biomarkers (cells, proteins, vesicles and nucleic acids) shed into the bloodstream and other body fluids, such as saliva or urine [Citation4]. The clinical application of liquid biopsy holds a promising approach to develop personalized medicine strategies the opportunity to determine the molecular landscape of the primary malignancy and metastases to perform real-time cancer management [Citation5]. Importantly, the implementation of liquid biopsy routinely in the clinical practice will help to have a better picture of the tumoral heterogeneity, at the moment of diagnosis and during the evolutive course of tumour. Furthermore, circulating biomarkers will be crucial to diagnostic cancer before any clinical symptoms or/and radiological findings and to detect and track minimal residual disease [Citation6].
Recently, accumulating evidence has demonstrated the diagnostic and prognostic value of saliva as promising novel and revolutionary liquid biopsy [Citation7]. Saliva is a complex body fluid that contains a wide collection of proteins as well as DNA, mRNA, microRNAs (miRNA/miR), metabolites and microbiota [Citation8]. As diagnostic approach, saliva offers many biochemical advantages over blood and tissue as non-invasive, easy storage, cost-effectiveness collection and, besides, dynamic availability for monitoring with less discomfort to the patient [Citation9]. Saliva-based molecular diagnostics reflects the physiological conditions of the body, and therefore, offers the opportunity to monitor oral and systemic health and disease [Citation10]. Ongoing advances in saliva research have allowed to the scientific community coined the term “salivaomics” [Citation11]. Salivary based approaches have been developed for the discovery of potential biomarkers into the six salivaomics which englobe the genome, the microbiome, the epigenome, the transcriptome, the proteome and the metabolome [Citation12]. Alterations in salivary concentrations of these molecules can be applied for the diagnosis, individual risk assessment, prognosis and monitoring of disease. To this regard a large number of studies provided different salivary biomarkers in head and neck cancers [Citation13] and also in cancers distant to the oral cavity [Citation12,Citation14].
In spite of, the great interest of saliva, to the best of our knowledge, this is the first meta-analysis that evaluates the diagnostic value of salivary biomarkers for malignant non-oral tumours detection. We summarized the scientific evidence and carried out a systematic review and meta-analysis evaluating the impact of salivary biomarkers for the diagnostic of malignant non-oral tumours.
2. Materials and methods
2.1. Search strategy
A comprehensive literature search was carried out up through March 2018, including PubMed, EMBASE, LILACS and the Cochrane Library databases, without language restrictions. The keywords used for searching retrieval were free-style words and Medical Subjects Headings (MeSH): (saliva OR salivary) AND (cancer OR carcinoma OR tumour) AND (marker OR biomarker) AND (diagnosis OR diagnostic) AND (sensitivity OR specificity OR ROC curve). Free-text search was additionally performed. The references cited in eligible articles were also hand-checked to identify additional studies that could be inadvertently omitted during the electronic database search.
2.2. Study selection
Two authors (ORG and MMSQ) independently assessed the literature extracted by the search strategy. These authors selected articles that appeared to meet the inclusion criteria based on their titles and abstracts. The eligibility criteria for inclusion were as follows: (1) diagnostic studies using salivary biomarkers for malignant non-oral tumours; (2) subjects included cancer patients and non-cancer controls and (3) enough data available for generating in each study unit a two-by-two (2 × 2) table which contains true positive (TP), false positive (FP), true negative (TN) and false negative (FN) values. The exclusion criteria were the following: (1) reviews, letters, personal opinions, book chapters, case reports, conference abstracts and meetings; (2) duplicate publications; and (3) those experimental in vitro and in vivo studies that reported associations between saliva and cancer. Then, full articles in which salivary biomarkers were used as potential diagnostic markers for malignant non-oral tumours were independently evaluated. Disagreements between the two authors were discussed until a consensus was reached.
We defined a “study unit” (or dataset) as the analysis of the relation between salivary biomarkers and each anatomic tumour location. As different salivary biomarkers may be reported in the same manuscript, a single publication could then report more than one study unit.
2.3. Data extraction and quality assessment
Data extraction was performed by two researchers (ORG and MMSQ); who independently screened and retrieved data from each eligible study. The following data were collected for each study: name of first author, year of publication, anatomic tumour location, country, ethnicity, study design, number of cases and controls, type of biomarkers (proteomic, metabolomic, epigenomic, microbiomic, transcriptomic and genomic), fraction of saliva (whole, supernatant and pellet), type of salivary sample (stimulated or unstimulated) and candidate biomarkers selected for the analysis. If the required data were incomplete, attempts were made to contact the authors to get the missing information.
The quality assessment of the selected studies was separately conducted by three independent investigators (LMR, ORG and MMSQ) using the Quality Assessment of Diagnostic Accuracy Studies 2 checklist (QUADAS-2) [Citation15] as recommended by the Healthcare Research and Quality Agency. When discrepancies existed among investigators, an agreement was reached after discussion. The QUADAS-2 is a revised tool developed to assess the quality of studies of diagnostic tests which analyses four key domains: (1) patient selection, (2) index test, (3) reference test and (4) flow and times. Each domain was evaluated in terms of its risk of bias and besides, the first three domains were also evaluated in terms of applicability concerns. Risk of bias and concern of applicability for each domain were considered as “low”, “high” or “unclear” [Citation15]. If the response to the risk of bias and applicability questions were “low” risk or “low” concern, one point for each item was given. The articles were grouped based on their score into high (6 and 7 points), moderate (4 and 5 points) and low (0–3 points) quality categories.
2.4. Data synthesis and statistical analyses
The data analysis was performed using free R software (v.3.4.4; https://www.r-project.org), Reviewing Manager ver. 5.3 (Cochrane Collaboration, Oxford, UK), MetaDiSc software (v.1.4) [Citation16] and STATA (v.14.0; https://www.stata.com). Raw data were used to calculate sensitivity and specificity of each study unit. To assess the overall accuracy, we calculated diagnostic odds ratio (DOR), area under hierarchical summary receiver operating characteristic (AUC), sensitivity, specificity, positive likelihood ratio (PLR) and negative likelihood ratio (NLR) using the DerSimonian-Laird’s estimator for random-effects or Mantel-Haenszel for fixed-effects. Interpretation of AUC values were as follows: >0.97 “excellent”, 0.93–0.96 “very good”, 0.75–0.92 “good” and 0.5–0.75 “reasonable” [Citation17]. According to Grimes et al. [Citation18] PLR in the range of 2–5, 5–10 and >10 represents small, moderate and large increases in the probability of disease when the test is positive and NLR in the range of 0.2–0.5, 0.2–0.1 and <0.1 represents small, moderate and large decreases in the probability of disease when the test is negative.
To assess the impact of the heterogeneity in the meta-analysis Higgins’s I2 test was used. This statistic represents the proportion of variability that is due to heterogeneity rather than to sampling error [Citation19]. According to the I2 test statistic the heterogeneity could be low (I2<50%) or high (I2>50%). If heterogeneity was high, the possible sources of heterogeneity were explored using Moses-Shapiro-Littenberg regression [Citation19] and subgroup analyses. Publication bias was investigated using Deek´s funnel plot asymmetry test [Citation20]. All statistical tests were two-sided. A p value less than .05 was considered statistically significant.
3. Results
The systematic search identified a total of 751 studies (Supplementary Figure S1). After evaluating the titles and abstracts, a total of 38 articles were selected to a full-text review, of which nine were excluded according to the exclusion criteria. Finally, 29) eligible articles were used for the meta-analysis according to the inclusion criteria [Citation21–48].
3.1. Study characteristics
The main characteristics of 29 articles are shown in . In total, 155 study units were included in the present meta-analysis. We identified eight studies with 71 study units on breast cancer, six studies with 29 study units on pancreatic cancer, four studies with 11 study units on oesophageal cancer, four studies with five study units on gastric cancer, three studies with 27 study units on lung cancer, three studies with 11 study units on ovarian cancer and only one study on colorectal cancer. Sample sizes ranged from 7–111 cancer patients and from 4–101 non-cancer controls. The articles were published between 1990 and 2018 and conducted in five countries: 17 in China, eight in USA, two in Japan, one in Russia and one in France. Twenty-seven articles were written in English and two articles in Chinese. All articles evaluated salivary biomarkers in adults and some studies appraised both saliva and blood. According to saliva-omics classification, 11 were proteomic studies, nine epigenomic studies, four transcriptomic studies, two metabolomic studies, two microbiome studies and two genomic studies. One of the studies included two complementary approaches (both transcriptomic and proteomic biomarkers) [Citation46]. The saliva sample type was unstimulated in 19 studies and stimulated in eight studies, while no data regarding the saliva characteristics were available for two studies. Finally, eight studies evaluated the diagnostic value of a single biomarker whereas 21 studies analyzed several biomarkers.
Table 1. Main characteristics of the 29 studies included in meta-analysis.
3.2. Quality of included studies
There were five, 22 and two articles with good, moderate and low quality, respectively. Four studies presented a low risk of bias in three of the four domains and eight studies had a low risk in two of the four domains. Twelve studies did not show high risk of bias, eight studies were considered of high risk in two of the four domains and seven studies had high risk in one of the four domains. According to the applicability component the majority of, the studies were adequate with respect to the patient selection (93.10%), index test (96.55%) and reference standard (96.55%; Supplementary Figure S2). There were 20.69% (N = 66/319) sub-questions with “unclear” response. The most “unclear” responses were observed in the patient selection domain (N = 24, 36.36%) and in the reference standard domain (N = 39, 59.09%) of the risk of bias assessment. Most authors did not report clearly if a consecutive or a random patients’ recruitment was performed and did not report a detailed description of the interval between index test and reference standard, and about the application of reference standard to the patients.
3.3. Diagnostic accuracy
The total sample size for this meta-analysis was 11,153 adult subjects (cancer patients and non-cancer controls), including 155 study units. The diagnostic accuracy (sensitivity, specificity and 95% confidence intervals (CI)) of each study unit included in this meta-analysis is shown in . Biomarkers sensitivity and specificity varied widely from 31 to 100% and 34 to 100%, respectively. The overall diagnostic accuracy showed 0.76 (95% CI, 0.74–0.77) of sensitivity and 0.76 (95% CI, 0.75–0.77) of specificity. The overall DOR was 13.42 (95% CI, 12.28–15.96) and the pooled PLR and NLR were 3.22 (95% CI, 2.92–3.55) and 0.31 (95% CI, 0.28–0.34), respectively (). In addition to diagnostic accuracy, the summary receiver operating characteristic (SROC) curve was plotted and the AUC was 0.85 (95% CI, 0.84–0.87), indicating “good” accuracy of the salivary biomarkers in malignant non-oral tumours ().
Figure 1. Paired forest plot with the diagnostic test accuracy (sensitivity, specificity and 95% confidence interval) of each unit study for the salivary biomarkers in the diagnosis of breast cancer.
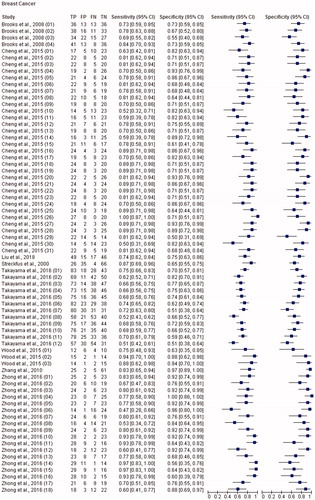
Figure 2. Paired forest plot with the diagnostic test accuracy (sensitivity, specificity and 95% confidence interval) of each unit study for the salivary biomarkers in the diagnosis of pancreatic cancer.
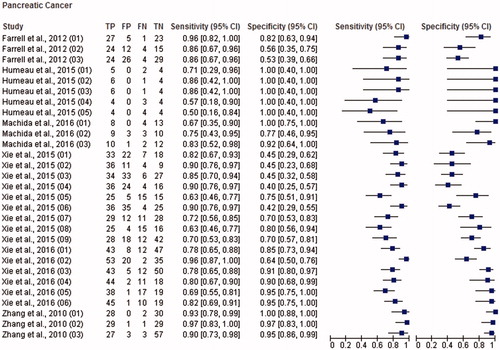
Figure 3. Paired forest plot with the diagnostic test accuracy (sensitivity, specificity and 95% confidence interval) of each unit study for the salivary biomarkers in the diagnosis of lung cancer.
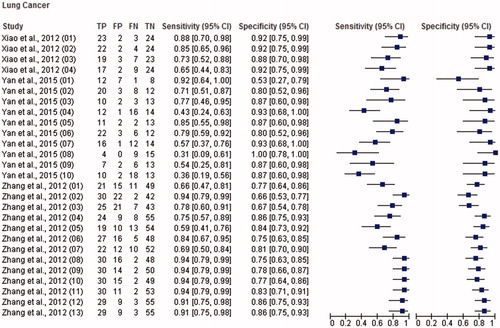
Figure 4. Paired forest plot with the diagnostic test accuracy (sensitivity, specificity and 95% confidence interval) of each unit study for the salivary biomarkers in the diagnosis of ovarian cancer.
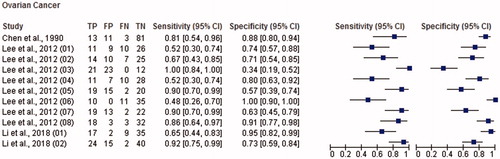
Figure 5. Paired forest plot with the diagnostic test accuracy (sensitivity, specificity and 95% confidence interval) of each unit study for the salivary biomarkers in the diagnosis of oesophageal cancer.
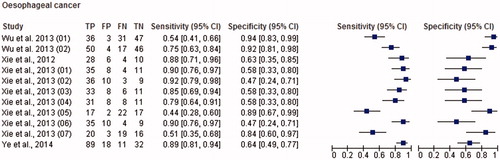
Figure 6. Paired forest plot with the diagnostic test accuracy (sensitivity, specificity and 95% confidence interval) of each unit study for the salivary biomarkers in the diagnosis of gastric cancer.

Figure 7. SROC curve with pooled estimates of sensitivity, specificity and AUC on the diagnostic value of salivary biomarkers in cancer.
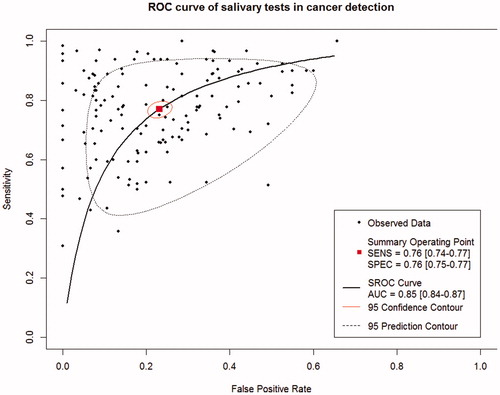
Table 2. Subgroup analysis of saliva testing for cancer diagnosis based on different covariates.
3.4. Meta-regression and subgroup analysis
The studies included in the meta-analysis showed an overall heterogeneity (I2= 61.81%). In order to assess the sources of heterogeneity between the studies, we used the meta-regression with the following covariates as predictor variables: saliva fraction, type of saliva, anatomic tumour location, sample size, type of biomarker and type of control. The results indicated that anatomic tumour location was a potential source of heterogeneity in this study (p = .002; Supplementary Table S1). Consequently, we conducted a subgroup analysis according to the cancer anatomic location. The salivary biomarkers showed a high sensitivity and specificity for detecting each anatomic tumour location (sensitivities ranged from 0.73–0.91 and specificities ranged from 0.71–0.89; ), indicating “good” diagnostic accuracy for the detection of malignant non-oral tumours. Summary data stratified into several subgroups are shown in and Supplementary Figures (S3–S6).
3.5. Publication bias
The analysis of funnel plot asymmetry showed a significant asymmetry (p < .001), evidencing the presence of publication bias (Supplementary Figure S7).
4. Discussion
In recent years, numerous studies [Citation50] have highlighted the potential of saliva as a novel liquid biopsy in the field of personalized medicine. In this sense, saliva-based diagnostics offers a unique opportunity for precision medicine in cancer. To the best of our knowledge, this study is the first systematic review and meta-analysis analyzing the potential of salivary biomarkers for the diagnosis of malignant non-oral tumours. A total of 155 study units from 29 eligible articles with 5700 cancer patients and 5453 non-cancer controls were included in this meta-analysis. Overall, our analysis showed that salivary biomarkers had “good” diagnostic accuracy with an AUC of 0.85, sensitivity of 0.76 and specificity of 0.76. To further evaluate the diagnostic accuracy of salivary biomarkers described in the 29 studies, we also examined the DOR, PLR and NLR. The pooled DOR value was 13.42, which indicates a moderate overall accuracy for salivary tests. In addition, the pooled PLR value was of 3.22, indicating that patients with cancer have a 3.22-fold higher chance of having a positive test result compared to cancer free-controls. By contrary, the pooled NLR value was 0.31, indicating that the probability of a patient having cancer is 31% if the salivary test shows a negative result. These results indicate the potential of salivary tests for detection of malignant non-oral tumours, but further controlled studies are necessary to provide evidence of their real clinical utility. Furthermore, we also conducted a meta-regression to determine the effect of saliva fraction, type of saliva, anatomic tumour location, type of biomarker and type of control on heterogeneity. While anatomic tumour location may be a potential source of heterogeneity, our subgroup analysis clearly indicated that salivary tests have a high diagnostic efficiency for each type of cancer. According to the criteria described by Jones and Athanasiou [Citation17], saliva tests showed “very good” accuracy for discriminating gastric cancer from cancer-free controls, with an AUC of 0.96, sensitivity of 0.91 and specificity of 0.89. Moreover, analysis of saliva tests in pancreatic, oesophageal, ovarian, lung and breast cancer revealed “good” diagnostic accuracy with AUC values of 0.87, 0.84, 0.86, 0.88 and 0.83, respectively.
Although our study represents the first work which analyze the utility of salivary biomarkers for non-oral cancers diagnosis that taking into account the omic fields and tumour types, two previous meta-analyses addressed the potential of salivary biomarkers as a clinical tool for head and neck carcinoma [Citation51] as well as oesophageal, oral and pancreatic cancer detection [Citation52]. Guerra et al. [Citation51] evaluated a total of 15 articles that analyzed different types of biomarkers such as mRNA, proteins, metabolites and DNA. Biomarker sensitivity and specificity varied from 14to 100% and 38 to 100%, respectively. However, Ding et al. [Citation52] only evaluated the overall diagnostic values of salivary miRNAs for cancer detection, being the sensitivity and specificity reported of 77%. This data evidenced the interest of salivary miRNAs as a diagnostic tool for cancer detection.
On the other hand, salivary-based tests may be a promising tool for screening and diagnosis of cancer since several biomarkers detected in saliva are able to discriminate cancer patients from healthy individuals with a high degree of sensitivity and specificity. A number of molecular screening tests [Citation2] have been established to detect cancer in early stages. However, current clinical blood-based screening/diagnostic tests are not accurate and specific enough [Citation53]. For example, the prostate specific antigen (PSA), a clinically used biomarker for prostate cancer screening and diagnosis, has been associated with different deficiencies including false-positive results and overdiagnosis [Citation54]. Molecular markers with a higher degree of sensitivity and specificity are urgently needed to improve the cancer screening and diagnosis. Saliva-based tests has shown potential as a tool for clinical diagnostics, accounting with even higher diagnostic sensitivity and specificity compared plasma-based test for cancer diagnosis [Citation30].
To this regard, our study provides relevant information about the accuracy and the applicability of salivary biomarkers to improve cancer detection by using a dynamic and non-invasive sample. However, we found some important limitations during our analysis. Publication bias is one of the main limitations. This could be explained by the fact that positive diagnostic accuracy results are easier to publish than studies revealing negative results [Citation55]. Moreover, small-size studies have a higher risk of uncontrolled bias. Therefore, future larger and randomized studies are needed to refute or support the actual scientific evidence. The methodological design of the studies included in the present meta-analysis represents another limitation. Several studies reported sensitive and specificity values without a validation phase. Furthermore, saliva-based diagnosis in cancer is still in its infancy for numerous tumours, limiting the number of studies included for specific types of cancer. Most of the selected studies did not perform a correlation analysis between the salivary biomarkers and TNM stage. From those studies evaluating the correlation with TNM stage, in two the salivary miRNA expression levels were not significantly correlated with cancer stage [Citation29,Citation39]. By contrary, another two studies reported a positive correlation between different salivary biomarkers and the cancer stage [Citation33,Citation44]. In spite of these data, the number of studies that correlated both variables were too low, it was not possible to perform a subgroup analysis based on TNM stage. Although this meta-analysis provides evidence about the potential clinical application of different salivary biomarkers, one of the most important circulating biomarker, the cell free-DNA (cfDNA) [Citation56], was not included in the study because of the unique three studies focussed on salivary cfDNA in non-oral cancer did not meet our inclusion criteria [Citation57–59]. Despite of the potential clinical application of salivary cfDNA for detection EGFR mutation, not significant differences were observed among the salivary cfDNA concentration among lung cancer patients and control groups, which suggests that salivary cfDNA might not be an ideal marker for the diagnosis of tumours distant to the oral cavity [Citation57]. In this sense, more research is required to effectively evaluate the potential of salivary cfDNA as diagnostic biomarker in cancer. In addition, most of the studies included in our meta-analysis are based on Asian populations, so subsequent studies should be performed in other ethnic populations. To face these challenges, research efforts should be addressed to validate salivary biomarkers for tumours diagnosis, characterization and monitoring. Importantly, our study provides important information on the current virtues and limitations of using salivary biomarkers that can help researchers improve future study designs.
5. Conclusion
In conclusion, our findings provide evidence on the potential application of saliva as a novel liquid biopsy for cancer screening and early diagnosis. Despite some limitations, our data demonstrate that salivary biomarkers have enough sensitivity and specificity to be applied for non-oral cancer detection. Fortunately, ongoing advances in salivaomics technology point towards a promising future of salivary biomarkers in cancer management. However, future guidelines on saliva research should require a randomized independent validation phase and multicenter design.
Supplemental Material
Download MS Word (795.7 KB)Disclosure statement
R.L.-L. reported Nasasbiotech during the conduct of the study; received grants and personal fees from Roche and Merck, personal fees from AstraZeneca, Pharmamar, Leo and Bayer and personal fees and non-financial support from BMS outside the submitted work. The rest of the authors have nothing to disclose.
References
- Schiffman JD, Fisher PG, Gibbs P. Early detection of cancer: past, present, and future. Am Soc Clin Oncol Educ Book. 2015:57–65.
- Nair M, Sandhu SS, Sharma AK. Cancer molecular markers: a guide to cancer detection and management. Semin Cancer Biol. 2018;52:39–55.
- Babayan A, Pantel K. Advances in liquid biopsy approaches for early detection and monitoring of cancer. Genome Med. 2018;10:21.
- Domínguez-Vigil IG, Moreno-Martínez AK, Wang JY, et al. The dawn of the liquid biopsy in the fight against cancer. Oncotarget. 2018;9:2912–2922.
- Crowley E, Di Nicolantonio F, Loupakis F, et al. Liquid biopsy: monitoring cancer-genetics in the blood. Nat Rev Clin Oncol. 2013;10:472–484.
- Bardelli A, Pantel K. Liquid biopsies, what we do not know (Yet). Cancer Cell. 2017;31:172–179
- Rapado-González Ó, Majem B, Muinelo-Romay L, et al. Cancer salivary biomarkers for tumours distant to the oral cavity. Int J Mol Sci. 2016;17:1531.
- Bonne NJ, Wong DT. Salivary biomarker development using genomic, proteomic and metabolomic approaches. Genome Med. 2012;4:82.
- Yoshizawa JM, Schafer CA, Schafer JJ, et al. Salivary biomarkers: toward future clinical and diagnostic utilities. Clin Microbiol Rev. 2013;26:781–791.
- Spielmann N, Wong DT. Saliva: diagnostics and therapeutic perspectives. Oral Dis. 2011;17:345–354.
- Ai J, Smith B, Wong DT. Saliva ontology: an ontology-based framework for a salivaomics knowledge base. BMC Bioinformatics. 2010;11:302.
- Rapado-González M, Álvarez-Castro DP, Abalo SC. A novel saliva-based miRNA signature for colorectal cancer diagnosis. J Clin Med. 2019;8:2029.
- Kaur J, Jacobs R, Huang Y, et al. Salivary biomarkers for oral cancer and pre-cancer screening: a review. Clin Oral Invest. 2018;22:633–640.
- Li F, Yoshizawa JM, Kim KM, et al. Discovery and validation of salivary extracellular RNA biomarkers for noninvasive detection of gastric cancer. Clin Chem. 2018;64:1513–1521.
- Whiting PF, Rutjes AWS, Westwood ME, et al. Quadas-2: a revised tool for the quality assessment of diagnostic accuracy studies. Ann Intern Med. 2011;155:529–536.
- Zamora J, Abraira V, Muriel A, et al. Meta-DiSc: a software for meta-analysis of test accuracy data. BMC Med Res Methodol. 2006;6:31.
- Jones CM, Athanasiou T. Summary receiver operating characteristic curve analysis techniques in the evaluation of diagnostic tests. Ann Thoracic Surg. 2005;79:16–20.
- Grimes DA, Schulz KF. Refining clinical diagnosis with likelihood ratios. Lancet. 2005;365:1500–1505.
- Lijmer JG, Bossuyt PMM, Heisterkamp SH. Exploring sources of heterogeneity in systematic reviews of diagnostic tests. Statist Med. 2002;21:1525–1537.
- Deeks JJ, Macaskill P, Irwig L. The performance of tests of publication bias and other sample size effects in systematic reviews of diagnostic test accuracy was assessed. J Clin Epidemiol. 2005;58:882–893.
- Brooks MN, Wang J, Li Y, et al. Salivary protein factors are elevated in breast cancer patients. Mol Med Report. 2008;1:375–378.
- Chen DX, Schwartz PE, Li FQ. Saliva and serum CA 125 assays for detecting malignant ovarian tumors. Obstet Gynecol. 1990;75:701–704.
- Cheng F, Wang Z, Huang Y, et al. Investigation of salivary free amino acid profile for early diagnosis of breast cancer with ultra performance liquid chromatography-mass spectrometry. Clin Chim Acta. 2015;447:23–31.
- Farrell JJ, Zhang L, Zhou H, et al. Variations of oral microbiota are associated with pancreatic diseases including pancreatic cancer. Gut. 2012;61:582–588.
- Humeau M, Vignolle-Vidoni A, Sicard F, et al. Salivary microRNA in pancreatic cancer patients. Plos One. 2015;10:e0130996.
- Lee YH, Kim JH, Zhou H, et al. Salivary transcriptomic biomarkers for detection of ovarian cancer: for serous papillary adenocarcinoma. J Mol Med. 2012;90:427–434.
- Li Y, Tan C, Liu L, et al. Significance of blood and salivary IEX-1 expression in diagnosis of epithelial ovarian carcinoma. J Obstet Gynaecol Res. 2018;44:764–771.
- Liu X, Yu H, Qiao Y, et al. Salivary glycopatterns as potential biomarkers for screening of early-stage breast cancer. EBioMedicine. 2018;28:70–79.
- Machida T, Tomofuji T, Maruyama T, et al. MiR 1246 and miR-4644 in salivary exosome as potential biomarkers for pancreatobiliary tract cancer. Oncol Rep. 2016;36:2375–2381.
- Sazanov AA, Kiselyova EV, Zakharenko AA, et al. Plasma and saliva miR-21 expression in colorectal cancer patients. J Appl Genetics. 2017;58:231–237.
- Shu J, Yu H, Li X, et al. Salivary glycopatterns as potential biomarkers for diagnosis of gastric cancer. Oncotarget. 2017;8:35718–35727.
- Streckfus C, Bigler L, Tucci M, et al. A preliminary study of CA15-3, c-erbB-2, epidermal growth factor receptor, cathepsin-D, and p53 in saliva among women with breast carcinoma. Cancer Invest. 2000;18:101–109.
- Takayama T, Tsutsui H, Shimizu I, et al. Diagnostic approach to breast cancer patients based on target metabolomics in saliva by liquid chromatography with tandem mass spectrometry. Clin Chim Acta. 2016;452:18–26.
- Wood N, Streckfus CF. The expression of lung resistance protein in saliva: a novel prognostic indicator protein for carcinoma of the breast. Cancer Invest. 2015;33:510–515.
- Wu W, Hou W, Wu Z, et al. miRNA-144 in the saliva is a genetic marker for early diagnosis of esophageal cancer. Nan Fang Yi Ke Da Xue Xue Bao. 2013;33:1783–1786.
- Wu ZZ, Wang JG, Zhang XL. Diagnostic model of saliva protein finger print analysis of patients with gastric cancer. World J Gastroenterol. 2009;15:865–870.
- Xiao H, Zhang L, Zhou H, et al. Proteomic analysis of human saliva from lung cancer patients using two-dimensional difference gel electrophoresis and mass spectrometry. Mol Cell Proteomics. 2012;11:M111.012112.
- Xiao H, Zhang Y, Kim Y, et al. Differential proteomic analysis of human saliva using tandem mass tags quantification for gastric cancer detection. Sci Rep. 2016;6:22165.
- Xie ZJ, Chen G, Zhang XC, et al. Saliva supernatant miR-21: a novel potential biomarker for esophageal cancer detection. Asian Pac J Cancer. 2012;13:6145–6149.
- Xie Z, Chen X, Li J, et al. Salivary HOTAIR and PVT1 as novel biomarkers for early pancreatic cancer. Oncotarget. 2016;7:25408–25419.
- Xie Z, Chen G, Zhang X, et al. Salivary microRNAs as promising biomarkers for detection of esophageal cancer. PloS One. 2013;8:e57502.
- Xu YG, Cheng M, Zhang X, et al. Mutual information network-based support vector machine strategy identifies salivary biomarkers in gastric cancer. J BUON. 2017;22:119–125.
- Yan X, Yang M, Liu J, et al. Discovery and validation of potential bacterial biomarkers for lung cancer. Am J Cancer Res. 2015;5:3111–3122.
- Ye M, Ye P, Zhang W, et al. Diagnostic values of salivary versus and plasma microRNA-21 for early esophageal cancer. Nan Fang Yi Ke Da Xue Xue Bao. 2014;34:885–889.
- Zhang L, Farrell JJ, Zhou H, et al. Salivary transcriptomic biomarkers for detection of resectable pancreatic cancer. Gastroenterology. 2010;138:949–957.e1-7.
- Zhang L, Xiao H, Karlan S, et al. Discovery and preclinical validation of salivary transcriptomic and proteomic biomarkers for the non-invasive detection of breast cancer. PloS One. 2010;5:e15573.
- Zhang L, Xiao H, Zhou H, et al. Development of transcriptomic biomarker signature in human saliva to detect lung cancer. Cell Mol Life Sci. 2012;69:3341–3350.
- Zhong L, Cheng F, Lu X, et al. Untargeted saliva metabonomics study of breast cancer based on ultra performance liquid chromatography coupled to mass spectrometry with HILIC and RPLC separations. Talanta. 2016;158:351–360.
- Xie Z, Yin X, Gong B, et al. Salivary microRNAs show potential as a noninvasive biomarker for detecting resectable pancreatic cancer. Cancer Prev Res. 2015;8:165–173.
- Kaczor-Urbanowicz KE, Martin Carreras-Presas C, Aro K, et al. Saliva diagnostics – current views and directions. Exp Biol Med. 2017;242:459–472.
- Guerra ENS, Acevedo AC, Leite AF, et al. Diagnostic capability of salivary biomarkers in the assessment of head and neck cancer: a systematic review and meta-analysis. Oral Oncol. 2015;51:805–818.
- Ding Y, Ma Q, Liu F, et al. The potential use of salivary miRNAs as promising biomarkers for detection of cancer: a meta-analysis. PLoS One. 2016;11:e0166303.
- Baron JA. Screening for cancer with molecular markers: progress comes with potential problems. Nat Rev Cancer. 2012;12:368–371.
- Fenton JJ, Weyrich MS, Durbin S, et al. Prostate-specific antigen-based screening for prostate cancer: evidence report and systematic review for the US Preventive services task force. JAMA. 2018;319:1914–1931.
- Egger M, Smith GD, Schneider M, et al. Bias in meta-analysis detected by a simple, graphical test. BMJ. 1997;315:629–634.
- Wan JCM, Massie C, Garcia-Corbacho J, et al. Liquid biopsies come of age: towards implementation of circulating tumour DNA. Nat Rev Cancer. 2017;17:223–238.
- Ding S, Song X, Geng X, et al. Saliva-derived cfDNA is applicable for EGFR mutation detection but not for quantitation analysis in non-small cell lung cancer. Thorac Cancer. 2019;10:1973–1983.
- Pu D, Liang H, Wei F, et al. Evaluation of a novel saliva-based epidermal growth factor receptor mutation detection for lung cancer: a pilot study. Thoracic Cancer. 2016;7:428–436.
- Wei F, Lin CC, Joon A, et al. Noninvasive saliva-based EGFR gene mutation detection in patients with lung cancer. Am J Respir Crit Care Med. 2014;190:1117–1126.