Abstract
Background
Immune checkpoint inhibitors (ICI) are regarded as a standard of care in multiple malignancies. We hypothesized that serum parameters are of prognostic value in ICI treated patients suffering from solid tumours.
Methods
Data from 114 patients treated with ICIs for solid malignancies from 2015 to 2019 at the Medical University of Vienna were collected retrospectively. Data included baseline characteristics, cancer type, serum parameters such as lactate dehydrogenase (LDH), C-reactive protein (CRP), albumin (Alb) and lymphocyte counts as well as overall survival (OS) and progression free survival. Additionally, the Gustave Roussy Immune Score (GRIm score) and the Glasgow prognostic score (GPS) were calculated. Cox regression models including time-dependent effects and strata for tumour type were used. Prognostic factors were pre-selected using a relaxed LASSO approach.
Results
The majority of patients were male (64.9%). The most common cancer types were non-small cell lung cancer (30.7%) and renal cell carcinoma (21.9%). Increased LDH and CRP were associated with poor 6-month OS (Hazard ratios (HR)=1.16 and 1.06 per 20% LDH/CRP increase; 95% CI 1.07–1.26 and 95% CI 1.03–1.09, respectively; p < .001). Both GRIm Score and GPS had a significant influence on OS (GRIm: HR = 2.84, 95% CI 1.72–4.69; p < .001 for high vs. low; GPS HR 3.57, 95% CI 1.76–7.25; p < .001 for poor vs. good). The proportion of explained variation (PEV) of our full multivariable model was significantly higher compared to the GRIm and GPS (PEV = 29.5% vs. 14.8% and 14.65%). When grouped into quartiles according to the individual 8-weeks change, both increased LDH and CRP correlated with poor OS (LDH (p=.001) and CRP (p < .001)).
Conclusion
The results of this analysis suggest that serum parameters might have prognostic value for the outcome of cancer patients treated with ICI, regardless of the tumour type.
In this retrospective analysis, 114 patients with solid tumours were included. The results of this analysis point out that pre-treatment LDH, CRP and albumin levels are strongly prognostic for a poor 6-month OS.
In addition to that, a high GRIm-score and poor GPS were associated with a worse OS (GRIm: HR = 2.84, 95% CI 1.72–4.69; p < .001 for high vs. low; GPS HR = 3.57, 95% CI 1.76–7.25; p < .001 for poor vs. good).
Pre-treatment serum parameters might have prognostic value for the outcome of cancer patients treated with ICI, regardless of the tumour type.
Key messages
1. Introduction
Immune checkpoint inhibitors (ICI) targeting the programmed cell death protein ligand 1 (PD-1/PDL-1) axis have entered the clinical routine during the last couple of years. ICI therapy is regarded as the standard of care for a wide range of malignancies such as metastatic non-small cell lung cancer (NSCLC), head and neck squamous cell carcinoma (HNSCC), renal cell carcinoma (RCC). While ICI therapy was shown to enable unprecedented response duration and even long-term survival in patients with stage IV disease, ICI are pricey and economic criteria have to be taken into account as well: In patients suffering from RCC or HNSCC, ICI were less likely to be cost-effective than in patients with melanoma or lung cancer as reported recently [Citation1]. From a clinical perspective, however, not all patients benefit from immunotherapy: A recent analysis of 262 patients suffering from 19 different malignancies demonstrated an objective response rate of 29% across all tumour types and a long-term survivor rate (i.e. longer than 2 years) of 11.8% [Citation2]. Response to single agent ICI was shown to vary considerably between highly sensitive tumours such as Hodgkin’s lymphoma and resistant tumours such as microsatellite stable colorectal cancer [Citation3,Citation4].
In the real-life setting, immunotherapy is frequently given in patient populations, which were initially excluded from the respective clinical trials, due to its beneficial side effect profile. In HNSCC, for instance, it was demonstrated that the prognosis of patients with an ECOG PS ≥2 or in the platinum resistant setting is worse compared to platinum sensitive patients with a better ECOG PS [Citation5]. In this context, it seems evident that the identification prognostic biomarkers for cancer patients treated with ICI is highly desirable both from an economic and from a clinical perspective.
Peripheral blood is an easily accessible source for potential biomarkers and serum parameters such as lactate dehydrogenase (LDH) are usually measured for routine purposes. Therefore, various scores such as the Gustave Roussy Immune Score (GRIm) and the Glasgow prognostic score (GPS) were established as prognostic tools in patients undergoing ICI therapy [Citation6–9]. The GRIm score is based on the three independent biomarkers albumin (Alb), LDH and neutrophil-to-lymphocyte ratio, while the GPS incorporates the inflammation marker C-reactive protein (CRP) and Alb to predict outcome of cancer patients [Citation6–8,Citation10,Citation11]. However, the majority of these studies investigated the GRIm score, GPS or selected serum parameters in a single tumour type.
In this retrospective analysis we investigated both the potential prognostic value of multiple serum parameters and prognostic scores (such as GRIm or GPS) in a heterogeneous patient population treated with ICI and suffering from a wide range of solid tumours.
2. Methods
2.1. Study population
This study was designed as retrospective analysis of observational data. The data of patients who started single-agent pembrolizumab or nivolumab between 01 January 2015 and 31 November 2016 were included and followed up until 01 July 2019, which was defined as data cut-off date for this analysis. This work was approved by the ethics committee of the Medical University of Vienna (2132/2016). Reporting of the study conforms to broad EQUATOR guidelines [Citation12].
2.1.1. Inclusions criteria
Stage IV pembrolizumab or nivolumab treated solid tumours except melanoma patients.
Serum LDH, CRP, Alb levels, white blood cell (WBC) count < 1 week before start of treatment known.
2.1.2. Exclusions criteria
LDH, CRP, Alb levels prior to treatment unknown.
2.2. Data collection and endpoint assessment
LDH, CRP, Alb, WBC, NLR, lymphocyte count (LC) and body mass index (BMI) were collected < 1 week prior to therapy start and after 6–8 weeks after therapy start. The data was retrospectively extracted from the medical files. The GRIm score and GPS were calculated as described previously [Citation8,Citation9]. As for GPS, we used 0.5 mg/dl (instead of 0.1 mg/dl) as the upper limit of normal for CRP as outlined above.
For graphical representation of NLR, patients were divided into three groups (group 1: NLR < 2.5, group 2: NLR = 2.5–6 and group 3: NLR > 6) [Citation8].
Differences in LDH, Alb and CRP levels prior treatment and 6–8 weeks after therapy start were calculated. Patients were classified according to quartiles of this difference. Patients in the lower quartile groups had the highest increase in LDH, Alb or CRP level, whereas patients in the higher quartile groups had a lower increase or decrease of the respective parameter. Overall survival (OS; time from the first ICI dose until death from any cause) and progression free survival (PFS; time from the first ICI dose until disease progression or death from any cause) were chosen as primary endpoints.
Raw data were generated at the Medical University of Vienna. Derived data supporting the findings of this study are available from the corresponding author TF on request.
2.3. Statistical analysis
Categorical variables are presented as counts and percentages. Due to asymmetrical distributions, continuous variables are presented as median and quartiles or range. For selected serum parameters as well as for proposed classification scores, Kaplan-Meier curves for OS and PFS are presented according to established cut-off values. Due to time-dependent effects, no log-rank test results are provided. Instead, p-values are derived from the univariable Cox regression models detailed below. A relaxed LASSO approach was used to select prognostic factors out of a pre-selected list (LDH, CRP, Alb, LC, BMI, WBC, neutrophil granulocytes (NG), monocytes (MO), basophile granulocytes). This was done separately for OS and PFS and did not include potential time-dependent effects. Univariable Cox regression models were then applied to each of the selected variables to test cubic and quadratic terms and to check the proportional hazards assumption (by testing interactions with time or log of time and selecting the one with lower AIC). Independent variables were log-transformed with basis 1.2 if necessary, to avoid overly influential extreme observations. Hazard ratios (HR) for such variables consequently quantify the effect of a 20% increase. For the three-category GPS score, an overall p-value from a 2 degrees-of-freedom test is reported while HRs with 95% confidence intervals (CI) are given with respect to category “good” as a reference.
All Cox regression models include a stratification for four tumour types (NSCLC, HNSCC, RCC, other) to account for potential heterogeneity in the baseline hazard function and to test for effects that are uniform across malignancies.
For independent variables exhibiting a significant time-dependence of their effect, hazard ratios are presented at 6, 12 and 24 months with 95% CI. Overall, p-values from a 2 degrees-of-freedom test for the main effect and the interaction with (log of) time are given. For the remaining variables, a single hazard ratio with 95% CI and the corresponding p-value is provided. A multivariable model includes all variables selected by the relaxed LASSO approach and time-dependent effects if necessary. Results are presented in the same way as for the univariable models.
Due to time-dependent effects, the proportion of explained variation (PEV [Citation13]) is reported only for short-term prediction by censoring all observations at 6 months. In order to investigate the potential effect of a change in serum parameters on OS, a landmark approach was used: All patients died or censored before 8 weeks after therapy start were deleted, and the time starting from 8 weeks after start of ICI therapy was investigated using Cox regression models with the difference of the serum parameter between baseline and 8 weeks as independent variable. Due to extreme outliers in these differences, results are presented for quartile groups (i.e. the 8-weeks differences were categorized into four groups according to observed quartiles). The p-values are reported which cover the effect for all quartile groups as well as time-dependent terms.
R package “penalized” was used for employing the LASSO approach. All remaining analyses were done using SAS 9.4 (SAS Inc., 2016). Two-sided p-values below .05 are regarded to indicate statistical significance.
3. Results
One hundred and fourteen patients met the inclusion criteria and were eligible for this analysis. The most common cancer types were NSCLC (30.7%) and RCC (21.9%). The majority of patients (62.3%) received >1 prior therapy lines. ICI therapy was either nivolumab (48.1%) or pembrolizumab (51.8%), mean number of ICI therapy cycles was 6 (range 1–36). In addition to that, smoking status was collected: 19 (21.1%) were current smokers, 35 (38.9%) were former smokers and 36 (40%) were never smokers. From 24 patients, smoking status was not available. Demographic data are shown in .
Table 1. Demographic data.
In order to account for this heterogeneous patient population and in attempt to identify prognostic markers beyond tumour biology, we employed stratification for four tumour types as outlined above. Heterogeneity of included tumour types and the influence of the distinct tumour biology on OS is depicted in .
Figure 1. Comparison of OS by tumour entity. Heterogenity of included cancer subtype and influence on OS. HNSCC: Head and neck squamous cell carcinoma; Lung: lung cancer; Renal: renal cell carcinoma.
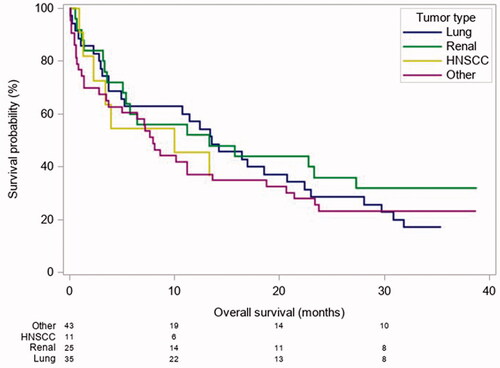
3.1. Association of pre-treatment serum parameters with outcome
Thirty-one (27%) and 93 (82%) patients presented with an increased LDH or CRP. Increased LDH and CRP were associated with both a shorter median OS (mOS) (3.7 months vs. 13.6 months, p < .001; 7.9 months vs. 23.3 months, p < .001) and PFS (2 months vs. 5 months, p < .001; 3.0 months vs. 7.0 months, p < .001; ; ).
Figure 2. Pre-treatment serum levels and their unadjusted association with OS and PFS. (A) OS curve for LDH, (B) PFS for LDH, (C) OS curve for CRP, (D) PFS for CRP levels, (E) OS curve for serum Alb, (F) PFS curve for serum Alb, (G) OS curve for LC and (H) PFS curve for LC.
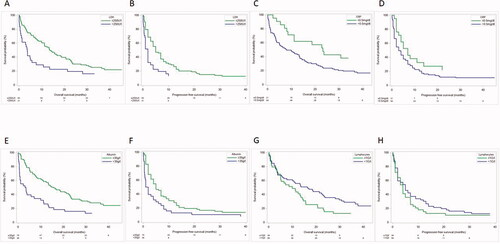
Table 2. Median OS and 25–75 percentile according to serum LDH, CRP, Alb and LC at therapy start.
An inverse association was found regarding Alb or LC: decreased Alb (33% of patients) and LC counts), (27% of patients), had a negative impact on OS (15.6 months vs. 3.3 months, p < .001 and 16.4 months vs. 11.2 months, p=.001). PFS was not associated with Alb or LC count (; ).
To further confirm the effect of these serum parameters based on their continuous values we present results from Cox regression models for a 20% increase of baseline LDH, CRP and LC) or an increase by 10 g/l Alb on OS and PFS.
In the univariable analysis using stratification by tumour type, both LDH and CRP were significantly associated with OS (p < .001 for both) but exhibited a time-dependent effect. While OS was significantly worse in patients with LDH or CRP increase at 6 months (for 20% increase: LDH HR = 1.16, 95% CI 1.06–1.26; CRP HR = 1.06, 95% CI 1.03–1.09), the effect subsided within 12 months (LDH: HR = 1.03, 95% CI 0.93–1.16; CRP: HR = 1.03, 95% CI 1.00–1.07). However, overall p-values showed a statistically significant association of increased LDH and CRP levels with PFS (p < .001). Particularly for a time period of 24 months increased LDH was associated with better PFS (HR 0.66, 95% CI 0.44–0.99), although, this effect was diminished in the multivariable model ().
Figure 3. Univariable and multivariable analysis of the effect of baseline covariable value on OS (A) and PFS (B). Hazard ratios (with 95% confidence intervals) quantify the effect resulting from a change in the respective baseline covariable value. Effects of covariables entering the model after log-transformation refer to 20% increases, those of other variables to increases by the given units. Time-dependent effects are reported at 6, 12 and 24 months. Confidence intervals not including 1 indicate statistical significance. Both, univariable and multivariable models are stratified for tumour type.
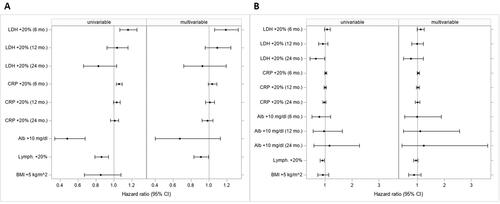
Conversely, an increase of Alb or LC was associated with a significantly improved OS in the univariable models (10 g/dl increase of Alb: HR = 0.48, 95% CI 0.34–0.68; p < .001; 20% increase of LC: HR = 0.86, 95% CI 0.79–0.94; p=.001). Similarly, an increase of LC was associated with significantly improved PFS (HR = 0.90, 95% CI 0.82–0.98; p=.013; ). There was no statistically significant association between BMI and OS or PFS.
In the multivariable analysis, the relaxed LASSO approach revealed LDH, CRP, Alb and LC as prognostic factors for OS (while BMI, WBC, NG, MO, BG were not selected; BMI was selected for PFS only). LDH, CRP and LC remained statistically significant predictors of OS (overall, p=.002, .013 and .038, respectively). For LDH, the point estimate at 6 months proved to be statistically significant (HR = 1.19, 95% CI 1.06–1.33).
An increase in LC was significantly associated with better OS (no time-dependent effect; HR = 0.91, 95% CI 0.83–0.99; p=.038; ).
3.2. Gps, NLR and GRIm score
The GPS, a validated tool to stratify prognostic groups in cancer patients, was calculated for 113 patients. Subsequently, patients were grouped accordingly (good: 17 (15%), intermediate: 61 (54%) and poor: 35 (31%)). The mOS and PFS differed markedly between the groups ((good: 23.3 months, intermediate: 14.2 months, poor: 2.3 months; overall p < .001) and good: 7 months, intermediate: 5 months, poor: 1 month, overall p=.004)) (). The “poor” cohort had a significantly shorter OS and PFS compared to the “good” group (HR = 3.57, 95% CI 1.76–7.23 and HR = 2.70, 95% CI 1.39–5.27; ; ).
Figure 4. Different scores and their influence on outcome parameters in cancer patients. The GPS has prognostic value regarding OS (A) and PFS (B) in our cohort of patients undergoing ICI therapy. Neutrophil to lymphcyte Ratio is strongly prognostic regarding OS (C) and PFS (D) in our cohort of patients. GRIm Score has prognostic value regarding OS (E) and PFS (F) in our cohort of patients treated with ICIs.
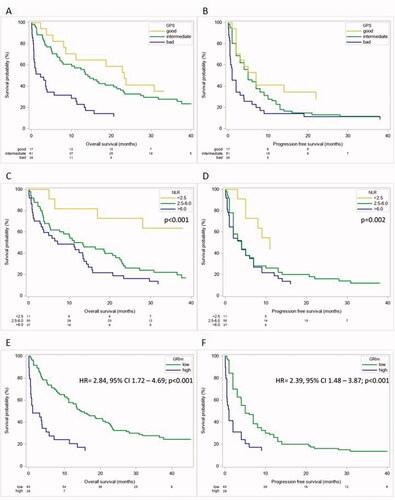
Table 3. Median OS and HR of predefined scores.
NLR was available for 98 patients. The mOS and PFS were significantly shorter the higher the NLR (mOS group 1: not reached, group 2: 13.8 months, group 3: 10.8 months; mPFS group 1: 11 months, group 2: 3.5 months, group 3: 4 months). An increase in NLR was associated with significantly shorter OS and PFS (20% increase: mOS: HR = 1.18, 95% CI 1.09–1.28; p < .001; PFS: HR = 1.12, 95% CI 1.04–1.20; p= .002; ).
The GRIm Score was calculated for 112 patients; 83 (74%) and 29 (26%) patients had a “low” or “high” score, respectively. Patients with a low score had a significantly better median OS and PFS (14.7 months vs. 1.1 months; and 5 months vs. 1 month, respectively). Furthermore, a high GRIm Score was associated with a shorter OS and PFS (OS: HR = 2.84, 95% CI 1.72–4.69; p < .001 and PFS: HR = 2.39, 95% CI 1.48–3.87; p < .001; ).
PEV for each score was calculated to compare the performance of our model based on continuous serum parameters with the above-mentioned scores within the first 6 months after start of ICI therapy. GPS explains 14.6% of the variability in overall survival between patients. The continuous NLR accounts for 11.3% and the GRIm Score explains 14.8% of variability in OS, while our multivariable model including LDH, CRP (both with time-dependent effect), Alb and LC explains 29.5% of the variability of the differences in OS between our patients.
3.3. Serum parameter changes and association with outcome
Finally, we analysed the effect of a change of the respective parameters within the first 6 − 8 weeks after therapy start on OS and PFS starting from week 8. Patients were grouped into quartiles 1-4 (Q1-Q4) according the individual 8-weeks change in LDH, CRP, and Alb respectively, with quartile group 1 (Q1) representing the highest increase of each serum parameter and subsequently a lower increase or decrease in the other groups.
An increase in LDH remained a factor significantly associated with poor outcome. Q1, which represents patients with the strongest LDH increase during treatment, had a shorter mOS compared to the remaining quartile groups (mOS: Q1 2.6 months, Q2 16.8 months, Q3: 20.4 months, Q4: 20.8 months; p=.001; ; ). Patients in Q1 had the worst outcome when grouped according to CRP (mOS: Q1 3.2 months, Q2: 21 months, Q3: 22.4 months, Q4: 12.4 months; p < .001; ; ). An increase of Alb was associated with a better outcome (mOS: Q1 17.6 months, Q2 19.9 months, Q3 21.3 months, G4 2.4 months; p= .02). Respective KM-curves and HRs are outlined in and .
Figure 5. OS according to the individual 8-weeks change in LDH (A), CRP (B) and Alb (C) when grouped into quartiles 1–4 (Q1–Q4). Q1 representing the highest increase and Q4 the lowest increase of each serum parameter. p<.05 shows a statistically significant effect between all quartiles including a time dependent effect.
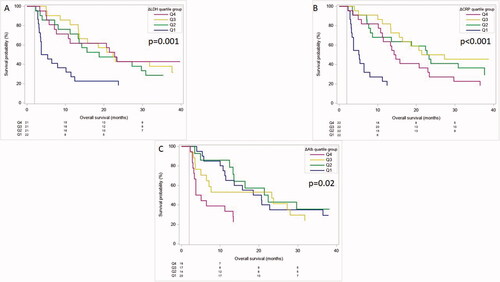
Table 4. Median OS and 25–75 percentile and HR and 95% CI for differences between baseline and 8 weeks regarding 6 month – OS for LDH, CRP and Alb.
4. Discussion
We showed that both serum parameters (LDH, CRP, Alb or NLR) and prognostic scores (GRIm and GPS) are associated with the outcome of real-world cancer patients receiving ICI regardless of the tumour type. Implementing those parameters into our model resulted in a higher PEV than one single parameter or score alone.
The advent of ICI led to new therapeutic options in a wide range of tumour types. However, response rates in an unselected population rarely exceeds 20%–30% [Citation14–23]. Recently, the economic impact due to the widespread use of ICIs on health care systems has come more into focus. An excess of costs compared to conventional chemotherapy has been reported by various groups [Citation24,Citation25]. Additionally, patients with an ECOG PS > 1 are rarely included into phase III trials [Citation5,Citation26]. A higher ECOG is associated with a poorer prognosis for cancer patients treated with ICI [Citation5,Citation27]. ECOG PS is highly influenced by the physicians perception and vulnerable to bias [Citation28–31]. As a consequence, more reliable and less observer influenced parameters are needed to assess the prognosis of cancer patients undergoing ICI therapy in the real-world setting.
Increased CRP and LDH levels were associated with a poor outcome in multiple malignancies [Citation32–34], which was confirmed in our study [Citation35–40].
LDH, CRP, Alb and LC were correlated with OS in the in the univariable analysis. A more pronounced increase in LDH or CRP and a decrease in Alb resulted in a worse outcome, indicating that the systemic inflammatory response is prognostic for shorter survival. Similar data was shown in melanoma patients [Citation36]. Interestingly, BMI had no effect on the outcome of our patient collective. Several studies in lung and renal cancer patients showed an association between a higher BMI and superior OS and PFS [Citation41–43]. Especially, Cortellini et al. found that obese patients (BMI ≥ 30 kg/m2) had a significantly longer PFS in the ICI cohort (HR = 0.61, 95% CI 0.45–0.82) compared to the chemotherapy cohort (HR = 1.27, 95% CI 1.01–1.60). Additionally, OS for obese patients was superior in the ICI arm (HR = 0.70, 95% CI 0.49–0.99) [Citation44]. In this context, it has to be noted that the inclusion criteria (e.g. TPS ≥ 50) or the cut-offs for BMI differed markedly between these studies, while we did not apply cut-off values to BMI before using them as independent variable in our models.
Furthermore, we investigated the prognostic value of GPS, GRIm score and the NLR in our patient cohort.
A strong association with a longer OS and PFS for a good GPS (0 points) compared to an intermediate (1 point) or poor (2 points) score was evident. There are just a few studies in the literature using GPS in ICI treated patients. In two retrospective studies, especially NSCLC patients showed that a good GPS was correlated with an improved PFS [Citation9,Citation45].
The GRIm Score was developed to provide a prognostic tool for patients in Phase I studies and was further tested in NSCLC patients receiving immunotherapy [Citation6–8]. We showed that this tool may be applicable in other solid tumours, since a high GRIm Score was significantly associated with both shorter OS and shorter PFS.
The NLR has been shown to be prognostic in a variety of malignancies and was also used in ICI analysis. A meta-analysis including 12 studies with a total of 1699 patients confirmed that a high pre-treatment NLR is associated with a worse OS in NSCLC patients [Citation46]. Different cut-off values for NLR were used in these studies, ranging from 2.8 to 6.46. We chose a cut-off of >6 for the graphical display, because it is used as a component of the GRIm Score and confirmed the results obtained in NSCLC [Citation8]. However, when implemented into our model the continuous NLR change was incorporated. We observed that an increase in the continuous NLR translated into worse OS and PFS ().
Finally, it has to be emphasized that our model, which uses the continuous baseline parameters in an optimized linear combination had the highest PEV as outlined above. This indicates a higher prognostic relevance for 6-month OS. The PEV allows to quantify different factors regarding their individual importance to predict outcome, even if different variable types (categorical scores vs. continuous laboratory parameters) or groups of several variables (combination of laboratory parameters) are compared [Citation13]. We acknowledge that the calculated PEV of 29.5% might be over-estimated, since it was obtained in the same sample that was used for model estimation, and should be evaluated employing an external validation cohort.
Limitations of this analysis include the retrospective nature and the low sample size. The latter does not allow to generalize negative (i.e. statistically non-significant) results beyond the sample at hand. Although one might assume that the heterogeneous group of different tumour types limits the applicability of this analysis, we have to state again that we included the tumour biology into our statistical model and took this issue into account as described above. Therefore, we demonstrated that pre-treatment serum parameters, the GRIm Score, GPS and NLR have a prognostic role in cancer patients receiving ICI regardless of their underlying malignancy.
Although this analysis was designed as a hypothesis generating exploratory study, which precludes an immediate impact on clinical decision making, this study adds to the evidence of a significant prognostic role of serum parameters in patients treated with ICI. Based on both the published literature and the results of this study serum parameters should be evaluated in prospective clinical trials as potential prognostic biomarkers in patients treated with ICI.
Consent
Not applicable. No individual person’s personal details, images or videos are being used in this study.
Ethical approval
This work was approved by the ethics committee of the Medical University of Vienna (2132/2016).
Author contributions
Minichsdorfer C.: Methodology, Resources, Data curation, Writing original draft, and Final approval
Gleiss A.: Formal Analysis, Validation, Writing, Reviewing and Editing, and Final approval
Aretin M-B.: Resources, Writing, Reviewing and Editing, and Final approval
Wagner C.: Resources, Writing, Reviewing and Editing, and Final approval
Schmidinger M.: Resources, Writing, Reviewing and Editing and Final approval
Fuereder T.: Conceptualisation, Supervision, Project administration, Writing, Reviewing and Editing, and Final approval
All authors agree to be accountable for all aspects of the work
Disclosure statement
Minichsdorfer C. received honoraria from Boehringer Ingelheim, MSD, Amgen. Travel grants from MSD, Merck Darmstadt.
Schmidinger S. received honoraria for lectures and/or Advisory boards from Roche, BMS, Pfizer, MSD, Merck, Exelixis, Ipsen, Alkermes, EUSA, EISAI
Travel grants from EUSA, Pfizer, Roche
Fuereder T. received honoraria from MSD; Merck Darmstadt, Roche, BMS, Accord; Sanofi, Boehringer Ingelheim; Amgen, Pfizer.
All other authors stated no conflicts of interest.
Data availability statement
Data and materials supporting the results are available upon reasonable request from the corresponding author TF ([email protected])
References
- Couchoud C, Fagnoni P, Aubin F, et al. Economic evaluations of cancer immunotherapy: a systematic review and quality evaluation. Cancer Immunol Immunother. 2020;69(10):1339–1958.
- Gauci ML, Lanoy E, Champiat S, et al. Long-Term survival in patients responding to anti-PD-1/PD-L1 therapy and disease outcome upon treatment discontinuation. Clin Cancer Res. 2019;25(3):946–956.
- Maly J, Alinari L. Pembrolizumab in classical Hodgkin's lymphoma. Eur J Haematol. 2016;97(3):219–227.
- Le DT, Durham JN, Smith KN, et al. Mismatch repair deficiency predicts response of solid tumors to PD-1 blockade. Science. 2017;357(6349):409–413.
- Dietz A, Welslau M, Hahn D, et al. HANNA: real-world outcomes from an observational study with nivolumab in patients with recurrent or metastatic squamous cell carcinoma of the head and neck in Germany. J Clin Oncol. 2020;38(15_suppl):6532–6532.
- Minami S, Ihara S, Ikuta S, et al. Gustave Roussy immune score and Royal Marsden hospital prognostic score are biomarkers of immune-checkpoint inhibitor for non-small cell lung cancer. World J Oncol. 2019;10(2):90–100.
- Minami S, Ihara S, Komuta K. Gustave Roussy immune score is a prognostic factor for chemotherapy-naive pulmonary adenocarcinoma with wild-type epidermal growth factor receptor. World J Oncol. 2019;10(1):55–61.
- Bigot F, Castanon E, Baldini C, et al. Prospective validation of a prognostic score for patients in immunotherapy phase I trials: the Gustave Roussy immune score (GRIm-Score). Eur J Cancer. 2017;84:212–218.
- Takamori S, Takada K, Shimokawa M, et al. Clinical utility of pretreatment Glasgow prognostic score in non-small-cell lung cancer patients treated with immune checkpoint inhibitors. Lung Cancer. 2021;152:27–33.
- Minami S, Ihara S, Komuta K. Gustave Roussy immune score and Royal Marsden hospital prognostic score are prognostic markers for extensive disease of small cell lung cancer. World J Oncol. 2020;11(3):98–105.
- Lenci E, Cantini L, Pecci F, et al. The Gustave Roussy immune (GRIm)-score variation is an early-on-treatment biomarker of outcome in advanced non-small cell lung cancer (NSCLC) patients treated with first-line pembrolizumab. J Clin Med. 2021;10(5):1005.
- Simera I, Moher D, Hoey J, et al. A catalogue of reporting guidelines for health research. Eur J Clin Invest. 2010;40(1):35–53.
- Gleiss A, Zeillinger R, Braicu EI, et al. Statistical controversies in clinical research: the importance of importance. Ann Oncol. 2016;27(7):1185–1189.
- Motzer RJ, Escudier B, McDermott DF, et al. Nivolumab versus everolimus in advanced Renal-Cell carcinoma. N Engl J Med. 2015;373(19):1803–1813.
- Motzer RJ, Tannir NM, McDermott DF, et al. Nivolumab plus ipilimumab versus sunitinib in advanced renal-cell carcinoma. N Engl J Med. 2018;378(14):1277–1290.
- Rini BI, Plimack ER, Stus V, et al. Pembrolizumab plus axitinib versus sunitinib for advanced renal-cell carcinoma. N Engl J Med. 2019;380(12):1116–1127.
- Cohen EEW, Soulieres D, Le Tourneau C, et al. Pembrolizumab versus methotrexate, docetaxel, or cetuximab for recurrent or metastatic head-and-neck squamous cell carcinoma (KEYNOTE-040): a randomised, open-label, phase 3 study. Lancet. 2019;393(10167):156–167.
- Garon EB, Hellmann MD, Rizvi NA, et al. Five-year overall survival for patients with advanced non‒small-cell lung cancer treated with pembrolizumab: results from the phase I KEYNOTE-001 study . J Clin Oncol. 2019;37(28):2518–2527.
- Herbst RS, Baas P, Kim DW, et al. Pembrolizumab versus docetaxel for previously treated, PD-L1-positive, advanced non-small-cell lung cancer (KEYNOTE-010): a randomised controlled trial. Lancet. 2016;387(10027):1540–1550.
- Mok TSK, Wu YL, Kudaba I, et al. Pembrolizumab versus chemotherapy for previously untreated, PD-L1-expressing, locally advanced or metastatic non-small-cell lung cancer (KEYNOTE-042): a randomised, open-label, controlled, phase 3 trial. Lancet. 2019;393(10183):1819–1830.
- Paz-Ares L, Luft A, Vicente D, et al. Pembrolizumab plus chemotherapy for squamous non-small-cell lung cancer. N Engl J Med. 2018;379(21):2040–2051.
- Reck M, Rodriguez-Abreu D, Robinson AG, et al. Pembrolizumab versus chemotherapy for PD-L1-positive non-small-cell lung cancer. N Engl J Med. 2016;375(19):1823–1833.
- Bellmunt J, de Wit R, Vaughn DJ, et al. Pembrolizumab as second-line therapy for advanced urothelial carcinoma. N Engl J Med. 2017;376(11):1015–1026.
- Guirgis HM. The impact of PD-L1 on survival and value of the immune check point inhibitors in non-small-cell lung cancer; proposal, policies and perspective. J Immunother Cancer. 2018;6(1):15.
- Nesline MK, Knight T, Colman S, et al. Economic burden of checkpoint inhibitor immunotherapy for the treatment of non-small cell lung cancer in US clinical practice. Clin Ther. 2020;42(9):1682–1698.e7.
- Abi Jaoude J, Kouzy R, Mainwaring W, et al. Performance status restriction in phase III cancer clinical trials. J Natl Compr Canc Netw. 2020;18(10):1322–1326.
- Khaki AR, Li A, Diamantopoulos LN, et al. Impact of performance status on treatment outcomes: a real-world study of advanced urothelial cancer treated with immune checkpoint inhibitors. Cancer. 2020;126(6):1208–1216.
- Simcock R, Wright J. Beyond performance status. Clin Oncol (R Coll Radiol). 2020;32(9):553–561.
- Chow R, Bruera E, Temel JS, et al. Inter-rater reliability in performance status assessment among healthcare professionals: an updated systematic review and Meta-analysis. Support Care Cancer. 2020;28(5):2071–2078.
- Chow R, Zimmermann C, Bruera E, et al. Inter-rater reliability in performance status assessment between clinicians and patients: a systematic review and Meta-analysis. BMJ Support Palliat Care. 2020;10(2):129–135.
- Kim YJ, Hui D, Zhang Y, et al. Differences in performance status assessment among palliative care specialists, nurses, and medical oncologists. J Pain Symptom Manage. 2015;49(6):1050–1058 e1052.
- Fang S, Sui D, Wang Y, et al. Association of vitamin D levels with outcome in patients with melanoma after adjustment for C-Reactive protein. J Clin Oncol. 2016;34(15):1741–1747.
- Fang S, Wang Y, Sui D, et al. C-reactive protein as a marker of melanoma progression. J Clin Oncol. 2015;33(12):1389–1396.
- Iivanainen S, Ahvonen J, Knuuttila A, et al. Elevated CRP levels indicate poor progression-free and overall survival on cancer patients treated with PD-1 inhibitors. ESMO Open. 2019;4(4):e000531.
- Nosrati A, Tsai KK, Goldinger SM, et al. Evaluation of clinicopathological factors in PD-1 response: derivation and validation of a prediction scale for response to PD-1 monotherapy. Br J Cancer. 2017;116(9):1141–1147.
- Diem S, Kasenda B, Spain L, et al. Serum lactate dehydrogenase as an early marker for outcome in patients treated with anti-PD-1 therapy in metastatic melanoma. Br J Cancer. 2016;114(3):256–261.
- Gao D, Ma X. Serum lactate dehydrogenase is a predictor of poor survival in malignant melanoma. Panminerva Med. 2017;59(4):332–337.
- Petrelli F, Cabiddu M, Coinu A, et al. Prognostic role of lactate dehydrogenase in solid tumors: a systematic review and Meta-analysis of 76 studies. Acta Oncol. 2015;54(7):961–970.
- Oya Y, Yoshida T, Kuroda H, et al. Predictive clinical parameters for the response of nivolumab in pretreated advanced non-small-cell lung cancer. Oncotarget. 2017;8(61):103117–103128.
- Taniguchi Y, Tamiya A, Isa SI, et al. Predictive factors for poor progression-free survival in patients with non-small cell lung cancer treated with nivolumab. Anticancer Res. 2017;37(10):5857–5862.
- Ichihara E, Harada D, Inoue K, et al. The impact of body mass index on the efficacy of anti-PD-1/PD-L1 antibodies in patients with non-small cell lung cancer. Lung Cancer. 2020;139:140–145.
- Zhang S, Pease DF, Kulkarni AA, et al. Real-World outcomes and clinical predictors of immune checkpoint inhibitor monotherapy in advanced lung cancer. Clin Med Insights Oncol. 2021;15:11795549211004489.
- Herrmann T, Mione C, Montoriol PF, et al. Body mass index, sarcopenia, and their variations in predicting outcomes for patients treated with nivolumab for metastatic renal cell carcinoma. Oncology. 2022;100(2):114–123.
- Cortellini A, Ricciuti B, Tiseo M, et al. Baseline BMI and BMI variation during first line pembrolizumab in NSCLC patients with a PD-L1 expression >/= 50%: a multicenter study with external validation. J Immunother Cancer. 2020;8(2):e001403.
- Kasahara N, Sunaga N, Tsukagoshi Y, et al. Post-treatment Glasgow prognostic score predicts efficacy in advanced non-small-cell lung cancer treated with anti-PD1. Anticancer Res. 2019;39(3):1455–1461.
- Li Y, Zhang Z, Hu Y, et al. Pretreatment neutrophil-to-Lymphocyte ratio (NLR) may predict the outcomes of advanced non-small-cell lung cancer (NSCLC) patients treated with immune checkpoint inhibitors (ICIs). Front Oncol. 2020;10:654.