Abstract
Tumour classifications play a pivotal role in prostate cancer (PCa) management. It can predict the clinical outcomes of PCa as early as the disease is diagnosed and then guide therapeutic schemes, such as active monitoring, standalone surgical intervention, or surgery supplemented with postoperative adjunctive therapy, thereby circumventing disease exacerbation and excessive treatment. Classifications based on clinicopathological features, such as prostate cancer-specific antigen, Gleason score, and TNM stage, are still the main risk stratification strategies and have played an essential role in standardized clinical decision-making. However, mounting evidence indicates that clinicopathological parameters in isolation fail to adequately capture the heterogeneity exhibited among distinct PCa patients, such as those sharing identical Gleason scores yet experiencing divergent prognoses. As a remedy, molecular classifications have been introduced. Currently, molecular studies have revealed the characteristic genomic alterations, epigenetic modulations, and tumour microenvironment associated with different types of PCa, which provide a chance for urologists to refine the PCa classification. In this context, numerous invaluable molecular classifications have been devised, employing disparate statistical methodologies and algorithmic approaches, encompassing self-organizing map clustering, unsupervised cluster analysis, and multifarious algorithms. Interestingly, the classifier PAM50 was used in a phase-2 multicentre open-label trial, NRG-GU-006, for further validation, which hints at the promise of molecular classification for clinical use. Consequently, this review examines the extant molecular classifications, delineates the prevailing panorama of clinically pertinent molecular signatures, and delves into eight emblematic molecular classifications, dissecting their methodological underpinnings and clinical utility.
Introduction
Prostate cancer (PCa) is famous for its high incidence rate and is characterized by high heterogeneity. It was reported that nearly more than 1,414,259 onsets were recorded in 2020, and 375,304 would die from it, imposing a huge burden on the world [Citation1]. Age is tightly associated with the emergence of PCa. Rare cases were reported among younger than 40 years, while the incidence increases dramatically after age 55 [Citation2]. The striking difference in incidence and prostate cancer-related death was also observed across racial and ethnic groups and was more than 2-fold as common among African Americans as among Caucasians. Meanwhile, the mortality of African Americans is 2.4 times greater than that of Caucasians [Citation3]. Compared to those without a positive family history, men with a father or brother who have been diagnosed with PCa are one or two times as likely to gain such disease, and the probability would increase with a number of positive [Citation4]. PCa has a long natural disease history that has been conceptualized into different terms via prostate-specific antigen (PSA) level, Gleason score (GS), tumour node metastasis (TNM) classification and clinical status, such as ‘localized PCa’, ‘advanced PCa’, and ‘metastatic PCa’. For tumours at different stages, therapeutic schemes are variable. Early-stage PCa is considered to develop slowly and can be treated with active surveillance, radical prostatectomy or external-beam radiation therapy alone. For localized advanced PCa and metastatic disease, monotherapy cannot prevent or delay the progression of such malignancy, and multidisciplinary management strategies are needed, such as a combination of resection and androgen deprivation therapy (ADT) and/or chemotherapy [Citation5,Citation6]. Metastatic PCa is divided into metastatic hormone-sensitive prostate cancer (mHSPC) and metastatic castration-resistant prostate cancer (mCRPC) based on the response to ADT. Among various treatment options for mHSPC, ADT remains the first-line treatment schema. Recent studies have shown that combinations of ADT and docetaxel or endocrine therapy are able to significantly improve the overall survival (OS) of mHSPC patients [Citation7]. A randomized, controlled clinical trial described that combined enzalutamide with ADT significantly reduced radiographic disease progression or death by 61% compared with placebo plus ADT [Citation8]. The randomized phase-III E3805 CHAARTED trial confirmed the benefit of docetaxel in combination with ADT for mHSPC patients, especially for those with high-volume disease [Citation9]. However, nearly all patients with mHSPC treated with ADT will develop lethal stage mCRPC, characterized by maintenance of castrated levels (< 50 ng/ml) with testosterone but with PSA progression or radiographic progression [Citation10]. The median survival of men with mCRPC is 10 to 12 months if effective treatment schemes are lacking [Citation11]. Therefore, optimizing the treatment strategy for mCRPC patients is required to prolong patient survival, delay disease progression and improve quality of life, and investigation of molecular changes in PCa brings hope to current dilemma. For instance, a randomized, open-label, phase III clinical trial identified that a poly(adenosine diphosphate-ribose) polymerase (PARP) inhibitor, Olaparib significantly prolonged recurrence-free survival (RFS) for patients with BRCA1, BRCA2, or ATM mutations [Citation12]; MAGNITUDE is an ongoing randomized phase III clinical trial enrolling 423 patients with mCRPC with homologous recombination repair gene alterations. It sought to investigate whether treatment with niraparib plus abiraterone acetate and prednisone could be used to prolong progression-free survival (PFS) in mCRPC patients with homologous recombination repair gene alterations. The interim analysis reports encouraging results for combination strategy, extending median PFS from 10.9 months to 19.5, particularly in patients with BRCA1/BRCA2 alterations [Citation13].
Most recent risk classifications relied on PSA, GS, and TNM stages, while considerable heterogeneity in prognosis was still observed using the existing risk stratification. For instance, GS 3 + 4 tumours were described to have a better clinical outcome than 4 + 3 tumours, but their overall GSs were equal [Citation14]; one would intuit that a high level of PSA represents a poor prognosis, while the detailed connections between PCa and the level of PSA have not been validated, and PSA will lose its predictive power when it reaches 70 ng/ml [Citation15]. In this setting, researchers tried to establish novel risk stratification schemes for making up for the shortcomings of the classical classifications and optimizing tumour management. Tumour molecular typing was first proposed by the National Cancer Institute of the United States. It is used to classify tumours through molecular analysis technology, which transforms tumour classification from traditional morphology to molecular typing based on molecular features. With the development of high-throughput sequencing and bioinformatics methods, oncogenesis and progression-driven genomic events have been revealed. These findings provide a chance for urologists to generate a novel classification system based on molecular features. In this study, we systematically reviewed the molecular alterations of PCa and analysed several representative molecular classification systems from their methodology to clinical value and limitations ().
Table 1. Details of the eight molecular classifications for prostate cancer, including molecular subtypes, methods, and clinical relevance.
Clinicopathological classification
Traditionally, prognostic prediction of PCa mainly relies on PSA, GS and TNM classification [Citation16–18]. They have been comprehensively used in clinical practice for decades and have improved the RFS of PCa. However, there are still many limitations in current risk stratification systems: 1. High GS implies rapid progression of PCa and requires extra adjuvant therapy even after radical resection. Regrettably, GS cannot guide adjuvant agent selection. Treatment status, such as resistance to androgen ablation therapy, and clinical stages, with or without bone metastasis, are employed to construct the framework for drug selection. This framework does not harbour a molecular mechanism foundation and thus often fails to guide targeted agent therapy. 2. To increase the reliability of the outcomes predicted by traditional pathological classification, D’ Amico et al. first defined ‘high-risk’ for PCa combined with PSA, GS, and TNM in 1998 [Citation19]. In such schema, ‘high-risk’ is defined as T stage ≥ cT2c, a Gleason score ≥8, or a PSA > 20 ng/ml, and it has been adopted by the American Urological Association [Citation20]. Subsequently, the Radiation Therapy Oncology Group, the Cancer of the Prostate Risk Assessment, and the National Comprehensive Cancer Networks successfully proposed similar classifications [Citation21–23]. However, significant heterogeneity exists in the prognosis predicted with those risk stratification models. For a given patient, 5-year RFS probabilities vary from 49% to 80% in different models, resulting in a dilemma for clinical decision-making [Citation24]. 3. It was reported that 23.5% of clinical T3a tumours misdiagnosed by digital rectal exam were eventually found to be pathologic T2 disease [Citation25]. GS and T stages rely on histopathological parameters, and the diagnostic accuracy is determined by pathologists with different experiences. In this context, misdiagnoses are inevitable. Schemes based solely on clinicopathological features are inaccurate in clinical practice and cannot meet medical needs. A novel classification system is imperative to further refine ‘high-risk’ and guide adjuvant drug selection to avoid overdiagnosis and overtreatment, and eight representative classifications were reviewed and analysed as follows ().
Molecular classification systems
Tomlins classification
Tomlins et al. generated microarray-based classifications based on 1577 samples from eight clinical research centers in Europe and America [Citation26]. Before the final model was established, they preestablished ERG, ETV1, ETV4, ETV5, FLI1, and SPINK1 microarray-based classification models. Only ERG status was identified via fluorescence in situ hybridization, and a random forest supervised model was generated to predict ERG status combined with cutoff optimization methods to establish the ERG model. The value of the ERG model ranges from 0 to 1, and the higher the score is, the greater the probability of ERG rearrangement. If the score of a patient exceeds 0.6, it is defined as ERG+. For the remaining models, unsupervised outlier analysis was performed to screen the outlier distribution in MCI, which was subsequently filtered with regression analysis. The minimum value of outliers in MCI was set as the threshold, and patients with higher outliers were defined as m-ETV1+, m-ETV4+, m-ETV5+, m-FLI1+ or m-SPINK1+. They classified patients in this study into four previously reported clusters based on the results of m-ERG, m-ETS, and m-SPINK1 models, including m-ERG+ (high m-ERG score, m- ETV1−, m-ETV4−, m-ETV5−, m-FLI1− and m-SPINK1−), m-ETS+ (m-ETV1+, m-ETV4+, m-ETV5+ or m-FLI1+, and m-ERG−), m-SPINK1+ (m-SPINK1+ and m-ERG−) and triple-negative subtypes (TripleNeg), and the proportions were 45%, 9%, 8%, and 38%, respectively.
Associations between molecular subtypes and clinicopathological features were revealed via univariate analysis and multivariate analysis. TripleNeg and m-SPINK1+ are highly associated with high preoperative PSA and GS PCa and have a half chance of developing to extraprostatic extension (EPE) compared with m-ERG + PCa. m-ETS + exhibits the highest probability of having seminal vesicle invasion (SVI) among the four subtypes. It was reported that more than 80% of primary PCa presented multifocal tumour foci [Citation27,Citation28], and the challenge faced by clinicians is how to confirm the anatomic origin of larger advanced PCa. Faisal et al. classified 300 radical prostatectomy specimens into m-ERG+, m-ETS+, SPINK1+, and TripleNeg subtypes. They found that non-ERG + subtypes were independent predictors of anterior tumours [Citation29], indicating that the ERG classifier might be a potential tool for verifying tumour location. Regrettably, although the m-ETS + and m-ERG + subtypes showed distinct pathological features suggestive of prognosis, no association was found in further survival studies. On the other hand, these seemingly contradictory observations that preoperative PSA and GS PCa and unmatched EPE and SVI status indicated the clinicopathological and molecular heterogeneity of PCa, hinting at the importance of further molecular feature investigation [Citation30].
This classifier represented the largest multicentre study on molecular subtyping of primary PCa until 2015. Despite its limitation in prognosticating the clinical outcomes of PCa and the lack of multifocal analysis for individual patients, different clinicopathological features are preliminarily obtained at the molecular level [Citation31]. Interestingly, this classifier has the potential to predict the response of PCa to chemotherapy. Taxanes are the first-line agents for CRPC, but the treatment often fails as resistance develops within a short time. Galletti et al. found that ERG can reduce patient susceptibility to taxane agents by binding soluble tubulins and altering tubulin kinetics, and further research found that ERG can serve as a marker in response to taxane therapy in CRPC patients [Citation32]. Therefore, for patients with ETG+, avoiding the taxane scheme and choosing other chemotherapy drugs may be better options. SPINK1 is a PCa autocrine carcinogenetic molecule, and RNA interference or blocking antibodies developed as a target can inhibit the development of cancer. Mechanistically, SPINK1 is structurally similar to epidermal growth factor, which can bind to epidermal growth factor receptor (EGFR) and induce EGFR dimerization and phosphorylation to enhance downstream signalling activation, thereby promoting the progression of PCa [Citation33–35]. The evidence above suggests that for patients in the SPINK1+ subtype, treatments with SPINK1 inhibitors or EGFR inhibitors are likely to achieve better clinical results. Collectively, despite the ERG classifier being limited in predicting the prognosis of PCa, it revealed the correlations between clinicopathological features and molecular alterations and had the potential to avoid inappropriate treatment schemas and indicate therapeutic targets for new drug development.
The cancer genome atlas (TCGA) classification
TCGA enrolled 333 primary PCa tumours from 425 available cases and 39 normal prostate tissues (23 underwent pathology review and excluded malignant diseases). All of the frozen blocks were made of tumour tissues and benign prostate tissues and were cut into 5-micron sections. The tissue images were confirmed via eight genitourinary pathologists to ensure accuracy. Multiplatform analysis of DNA and RNA sequencing data was employed to estimate tumour purity to acquire malignant tissues with high tumour cellularity. Based on 333 primary PCa samples, they characterized the biomolecules of PCa via four platforms and comprehensively elucidated the molecular alteration landscape from somatic mutations to DNA methylation of the 333 tumors. They subsequently applied unsupervised clustering and iCluster integrative clustering algorithms on the four molecular platforms. The results showed that 74% of PCa cases were driven by canonical oncogenic events, which included specific fusions (ERG, ETV1/4, FLI1) or mutations (SPOP, FOXA1, IDH1). Therefore, they classified 74% of PCa into seven subtypes based on corresponding driven events [Citation36].
The seven subtypes presented diverse genomic, transcriptomic, and epigenomic patterns. Overall, ETS-family gene fusions drove 53% of PCa oncogenesis, which included fusions of ERG, cETV1, ETV4, and FLI1. ERG-TMPRSS2 gene fusion was the most frequent event in primary PCa, with a proportion of 46%. TMPRSS2 was the most common but not the only fusion partner among ETS fusion cases, and others such as SLC45A3 and NDRG1 were also observed. The four gene fusions are mutually independent in most cases, while there are still exceptions involving two or more gene fusions. In some cases, they observed the overexpression of the whole ETS exon, although they were generally considered mutually exclusive. This phenomenon might be explained by the fact that the overexpression of the whole ETS exon was mediated via epigenetic modification or cryptic translocations of the entire gene locus to a transcriptionally active neighbourhood. A cryptic genomic rearrangement 3′ of the ETV1 locus, which is a region on chromosome 14 near FOXA1 and MIPOL1, has been identified. Similarly, the SPOP subtype was mutually independent of ETS fusion but generally accompanied by FOXA1 mutation in the same PCa cells. In addition, multiple patterns of concurrent gene alterations in the seven PCa were found, such as the deletion of PTEN in most PCa, indicating that the heterogeneity of PCa was not limited to the driven genes. CHD1 deletion often co-occurs with SPOP mutation, and they defined a new subtype with molecular features distinct from those described above. The novel subtype had a higher DNA methylation level, upregulated expression of SPINK1, and homogenous gene expression patterns.
To reveal the epigenomic characteristics of primary PCa, unsupervised hierarchical clustering was performed, and four distinct groups with different levels of hypermethylated CpGs were identified. They were divided into four clusters in descending order of methylation; cluster 1 represented the highest methylation level, and cluster 3 was the lowest. Two hypermethylation patterns were found in the ERG subtype: one-third presented unique hypermethylation, which belonged to cluster 1, whereas the rest belonged to the moderate hypermethylation cluster, which belonged to cluster 3, and the difference between them was three times. Compared to the ERG + subtype, ETV1/4+ and FLI1+ showed heterogeneous hypermethylation changes. Although no clue about the difference has been reported to date, epigenetic heterogeneity proved the differences in molecular features and clinicopathology between ERG + and non-ERG + ETS fusion PCa, which was consistent with the results in mouse modelling [Citation26,Citation37]. In addition, the SPOP and FOXA1 subtypes showed homogenous methylation and almost belonged to cluster 2. It is worth mentioning that IDH1+ had prominent increased genome-wide DNA hypermethylation, which was also reported in other tumors, such as gliomas and acute myeloid leukaemias [Citation38,Citation39]. The specific role of IDH1 hypermethylation remains unclear but may be related to cancer types. Methylation is responsible for the silencing of genes that are differentially expressed in primary PCa, downregulated in metastatic PCa, and involved in prostate organ development [Citation40,Citation41]. Combining epigenetic and mRNA data identified 164 genes that were silenced via epigenetic modulation, and more than 85% of them were shared by all seven types of PCa. Nevertheless, there were still differences in epigenomic features among the seven subtypes. For instance, STAT6 silencing was mainly observed in ETS + tumours but not in SPOP + and IDH1+ subtypes. Regrettably, it is still a mystery to date how the variable methylation phenotypes impact PCa and what the consequences of these differences might be. Although several studies considered methylation as a novel prognostic marker for PCa and a potential therapeutic target for hormone-relapsed PCa, they were not demonstrated based on the TCGA classification system, and the clinical utility is to be classified [Citation42].
The cell proliferation and survival of PCa are highly dependent on androgen receptor (AR) signalling, and different types of primary PCa exhibit variable AR activities, which hinder response prediction before treatment begins. In TCGA classification, AR activity was quantified via 20 genes that have been validated as AR transcriptional targets [Citation43]. Relied on the AR activity score, TCGA validated that the highest AR transcriptional activity exists in SPOP + or FOXA1+ subtypes, while the activity of AR is variable in the ERG + subtype, similar to the results in the published literature [Citation44–46]. AR and AR coactivators serve as substrates of SPOP. Mutant-type tumours enforce oncogenic signalling through AR activation, while wild-type SPOP tumours drive tumorigenesis in part via ERG signalling, which indicates a different response to ADT [Citation46–48]. According to a published article, mutation of SPOP enhances the susceptibility to ADT, and SPOP + subtypes might benefit more from abiraterone and enzalutamide [Citation49,Citation50]. For mutated-SPOP PCa, which is driven via ERG signalling, small-molecule targeting of the E3 ligase adaptor might be more favourable [Citation51]. In addition, there is an antagonistic relationship between SPOP and ERG mutation, and the susceptibility of the ERG + subtype to androgen depends on the dosage; that is, mutated ERG increases the susceptibility of the tumour to a high dosage of androgen, but the SPOP + subtype does not [Citation52].
14-Pathway (PCS) classification
You et al. built a prognostic classifier based on 14 pathways [Citation53]. A total of 38 cohorts from three publicly available databases, including Gene Expression Omnibus (GEO), ArrayExpress, and UCSC Cancer Genomics Browser, were selected. The raw data were processed with median centering and the quantile normalization method and merged into a single cohort incorporating 1321 tumour samples as the discovery cohort. An additional 12 cohorts were enrolled as the validation cohort, which included 2728 tumours from primary and CRPC/bone metastasis samples. First, 22 pathway signatures were broken down into 14 pathway signatures, which included three categories: 1) PC-relevant signalling pathways, including activation of AR, AR-V, EZH2, FOXA1, and rat sarcoma viral oncogene homolog (RAS) and inactivation by polycomb repression complex 2; 2) genetic and genomic alterations, including mutation of SPOP, TMPRSS2–ERG fusion, and deletion of PTEN; and 3) biological features related to aggressive PC progression, including stemness (ES), cell proliferation (PRF), epithelial-mesenchymal transition (MES), pro-neural (PN), and aggressive PC with neuroendocrine differentiation (AV). Pathway activation scores were computed in each specimen in the DISC cohort using the Z score method [Citation22]. The Z score was calculated to quantify pathway activation [Citation54], and nonnegative matrix factorization (NMF) clustering was employed to identify the optimal numbers of clusters [Citation55]. Three distinct clusters with different signalling pathway activation patterns were identified and defined as PCS1, PCS2, and PCS3.
The three subtypes presented mutually exclusive molecular and clinical features. PCS1 presented high activation of EZH2, PTEN, PRF, ES, AV, and AR-V pathways. In the PCS2 subgroup, ERG, AR, FOXA1, and SPOP pathway activation predominate, and PCS3 exhibits high activation scores for RAS, PN and MES but downregulation of AR and AR-V. To verify the PCS schema, a 14-pathway classifier was established via a naïve Bayes machine learning algorithm and was applied to eight local PCa cohorts and two CRPC/bone metastasis cohorts. Across different practice settings, the classifier exhibited amazing consistency. All the samples in different cohorts were successfully divided into three subtypes with similar proportions. Interestingly, when the PCS schema was applied to human PC cell lines and genetically engineered mouse models, different numbers of clusters were observed. Human cell lines were divided into PCS1, PCS2, and PCS3 subtypes, while only two subtypes were in genetically engineered mouse models. Thus, the difference between humans and mice must be noted when detailed experimental validation is conducted in corresponding models.
PCS can be independent of GS to distinguish tumours with different risks of progression. Tumours in PCS1 indicated the highest risk of progression even with a low GS. This characteristic is valuable in differentiating high-risk PCa from low GS. There is controversy regarding which subtype is most likely to cause CRPC and bone metastasis. In the initial study, 66% of tumours were reported to potentially develop into CRPC and bone metastasis. However, Thysell et al. analysed the PCS classification using principal component analysis in their biobank and found that PCS2 predominated in bone metastases PCa (77%) [Citation56], which contradicts the result of You et al. To further confirm the most aggressive phenotype, You and colleagues applied a naïve Bayes machine learning algorithm to Thysell and colleagues’ datasets, yet minority bone metastases were designated into the PCS2 subgroup [Citation57]. Interestingly, both studies manifested high mutation frequencies of AR-V in PCS1. This type of variant has been widely reported in CRPC and is highly related to resistance to AR-targeted therapy [Citation58]. Therefore, although the most aggressive subtype among PCS has not yet been agreed upon, it seems that more evidence tends towards PCS1.
PCS is a novel molecular subtyping system that has been validated with clear correspondence between molecular subtypes and clinicopathological features in PCa. There is crosstalk between the PCS and previously published molecular classifications, such as the TCGA and ERG classifiers. TCGA/SPOP PCa is tightly correlated with PCS1, PCS2 is enriched in TCGA/ERG subtype, while the other subtypes in TCGA are mainly classified into PCS3. Similarly, a high frequency of ERG/ETS + and ERG/SPINK + subtypes is observed in PCS1, and PCS2 predominates in the ERG/ERG + subgroup, while all patients with PCS3 are designated the TripleNeg subtype.
PAM50 classification
PAM50 was first built to define the main molecular subtypes of breast cancer via Parker and colleagues in 2009 [Citation59]. Five distinct subtypes of breast cancer are identified as luminal A, luminal B, basal, normal-like, and amplified human epidermal growth factor receptor 2 (HESR2). Considering that both PCa and breast cancer are driven by gonadal steroid hormones and share a number of transduction pathways, Zhao et al. applied PAM50 in 3782 PCa samples from six published retrospective cohorts (n = 1567) and a prospective cohort to analyse the value of PAM50 in PCa risk stratification. They divided PCa into three subtypes via PAM50, and named luminal A, luminal B, and basal subtypes, yet excluded the HER2 subtype for lacking ERBB2/HER2 amplification in PCa [Citation60].
Based on the clinical outcome profiles from six retrospective cohorts that included distant metastasis-free survival, biochemical recurrence-free survival, prostate cancer-specific survival, and OS, they investigated the clinical value of PAM50 in PCa. The luminal B subtype presented the poorest outcomes compared with the luminal A and basal subtypes as well as the highest levels of PSA and GS and the probabilities of extraseminal invasion and lymph node invasion. Notably, the prognostic role remained stable even after removing the effects of confounding factors in multivariate Cox regression analysis. Despite the luminal A subtype sharing similar biological features with luminal B, it has a moderate prognosis, which is more similar to the basal subtype. In addition, elevated proliferation genes, such as MK167, are found in the luminal B subtype, which is considered to be responsible for the different clinical outcomes between luminal A and luminal B subtypes.
Meanwhile, gene set enrichment analysis (GSEA) in this study enriched more AR pathways to luminal subtypes compared to basal subtypes, which encouraged them to further explore the predictive role of PAM50 in distinguishing susceptible patients to ADT. They applied PAM50 to postoperative cohorts with patients who received ADT or not and retrospectively matched the clinical outcomes mentioned above and positive surgical margin status and radiotherapy treatment status to perform exploratory subgroup analysis. Luminal B was finally confirmed to benefit from ADT, while luminal A and basal B did not. A possible explanation for the different susceptibilities between luminal A and luminal B is that the former already have a good clinical outcome and thereby gain little benefit from aggressive therapy.
Zhao et al. confirmed that PAM50 is a promising tool for risk stratification and therapy prediction in PCa. Further validations are advised in prospective trials. A phase-2 multicentre open-label trial, NRG-GU-006, enrolled patients with a high risk for progression (an increase in PSA after prostatectomy) and randomly stratified samples into salvage radiotherapy alone or salvage radiotherapy concurrent with a second-generation AR antagonist (apalutamide, ARN-509) based on the PAM50 classifier [Citation61]. This is the first prospective study to stratify local PCa based on a molecular classification, the PAM50 classifier, and the results provide new insights into ADT in PCa management. However, the high similarity between prostate and breast cancers does not mean that both are identical tumours. The PAM50 algorithm originated from breast cancer and was then applied in PCa patients, which will take into account inherent limitations. A more tailored algorithm based on molecular features in PCa is suggested to improve the accuracy of predicting prognosis and therapy response. In addition, PCa is a heterogeneous disease from multifocal distinct tumour foci resulting in basal and luminal subtypes perhaps coexisting within the same tumour [Citation62]. However, methodological limitations of PAM50 make the measure of intratumoural heterogeneity absent [Citation63]. Integration of PAM50 with genomic analysis might be a way to further improve this model.
Bone-metastatic classification
Bone is the most common site of distant metastasis for PCa, and bone metastasis represents the terminal stage of the disease. AR drivers in 80% of PCa bone metastases, and 20% were correlated with high immune cell and inflammatory activities [Citation64]. Rapid resistance to ADT and a lack of available tools for optimizing management contribute to the high case fatality rate of bone-metastatic PCa [Citation65]. In this study, the author used the largest set of clinical PCa bone metastasis cohorts to date, which included both fresh frozen and formalin-fixed paraffin-embedded biopsy samples from Umeå University Hospital. The samples that contained 72 cases of different states and treatment were used to explore the whole gene expression pattern of bone-metastatic PCa. Subsequently, they employed the pattern of the unsupervised cluster in principal component analysis to determine the best clusters of bone-metastatic PCa and five clustering algorithms to perform cluster analysis for PCa, including hierarchical clustering using the Euclidean distance and Ward linkage, hierarchical clustering using the Manhattan distance and Ward linkage, k-means clustering, self-organizing maps, and affinity propagation [Citation66]. Latent structures discriminant analysis was performed to generate the prediction model with the transcriptional expression level of the top 20 signature genes among different clusters [Citation67]. Three subtypes were eventually identified via hierarchical cluster analysis and the first two principal components, termed MetA, MetB, and MetC subtypes, had proportions of 71%, 17%, and 12%, respectively [Citation68].
The author also analysed the distinct features of clinicopathology, histology, morphology, and transcriptome among the three metastatic subtypes. Compared to MetA and MetC subtypes, patients in MetB have a shorter PCa-related specific survival and lower level of serum PSA. Further morphological and immunohistochemical analysis revealed that MetA-C subtypes have distinct epithelium/stroma ratios. The poorest subtype, MetB, more likely originated from non-luminal cells, as elevated Ki-67 tumour cells and cellular atypia, decreased tissue PSA and a lack of glandular structures were observed. Meanwhile, decreased luminal cell markers, such as KLK3 and CDH1, were enriched in MetB compared to MetA. However, MetB remained KRT18 and AR expression, the author, therefore, suggested classifying it into dedifferentiated luminal phenotype. From this, we speculated that there are differences in the histological type of the worst phenotype in primary PCa and metastatic PCa, and the former with luminal origin has the poorest prognosis, while the latter more likely originates from non-luminal cells. As mentioned above, both in primary and metastatic PCa, AR plays a pivotal role in accelerating tumour progression [Citation69–72], and its activity could be regulated via coactivators or corepressors [Citation73]. The MetB subtype showed decreased androgen-stimulated gene expression but a high tumour cell proliferation status. This finding seems contradictory but can be explained via the high expression of FOXM1, which has been confirmed to initiate mitosis [Citation74]. Inhibitors of FOXM1, such as STL427944 and siomycin A [Citation75], might be more suitable for MetB than anti-androgens. The most frequent subtype, MetA, was more likely to represent the luminal cell origin as elevated KRT18, FOXA1 and KLK3 enrichment, and signs of glandular structure were observed. Further analysis of the intermediate subtype of MetA and MetB showed that the MetA subtype can dedifferentiate into the more aggressive MetB subtype by altering AR activity. MetC tended to have a basal cell origin due to a lack of luminal cell markers but high basal cell markers, such as CEBPB and GSTP1, but more evidence is required to prove this hypothesis. The high levels of immune responses, inflammation, cell adhesion and remodelling in MetC suggested that it may belong to the non-AR-driven clusters mentioned above. For this subtype, the C/EBP transcription factor is considered the upstream regulator, and it can also be downregulated via AR pathways and is associated with inflammation and apoptosis, thereby leading to therapeutic resistance in CRPC [Citation76]. Meanwhile, PCa cells accelerate cellular senescence in response to ADT, which is tightly associated with castrate resistance for the selection effect. The expression of C/EBP enhances senescence during ADT through multiple transcriptional targets, thereby promoting the progression of chemoresistance and castration-resistant emergence. Thus, monotherapy of ADT is harmful to MetC, and the utility of targeting C/EBPβ in combination with ADT might be more suitable [Citation77, Citation78].
Interestingly, the author revealed that the morphological features of primary PCa would continue to the bone metastasis stage. The phenotypes of previously proposed molecular subtypes of primary PCa, which included the PCS2, PCS1, and PCS3 subtypes defined in the 14-pathway classifier and the luminal A, luminal B, and basal subtypes defined in the PAM50 classifier, were likely to be MetA-C subtypes. However, more noteworthy is the huge arguments of biological behaviors and intrinsic property changes between primary PCa and metastatic disease [Citation79], which indicate that different treatment strategies are required for them. Realizing the high proliferation, unusually decreased PSA levels, and global proteome variations in bone metastatic PCa is beneficial for developing novel management schemes for this disease.
Collectively, Thysell et al. defined the molecular subtypes of bone-metastatic PCa based on comprehensive transcription profiling. MetA-C subtypes were also able to be clustered via immunohistochemistry method: high PSA and low Ki67 represent MetA, low PSA and high Ki67 represent MetB and the rest with no specific parameters represent MetC, which highlights the possibility to apply it in clinical.
Epithelial-defined classification
PCa is famous for its intratumoural heterogeneity. One important point that cannot be neglected is that stromal cells such as endothelial cells, fibroblasts, and immunocytes in PCa tissues would add extra heterogeneity [Citation80,Citation81]. In this context, molecular profiling of PCa based on global transcriptomic analysis of tumor tissues is often susceptible to the confounding of the extra heterogeneity. Han et al. classified PCa based on single-cell analysis defining epithelial-expressed genes to avoid potential confounding. In this classification [Citation82], 1592 overexpressed genes in five preselected epithelial cell populations were identified by comparison with the remaining cell populations from single-cell RNA-seq data of three male prostate specimens [Citation83]. Samples in the TCGA-PRAD cohort were further purified by the ABSOLUTE and ISOpure methods to perform self-organizing map clustering to find the four robust clusters. Based on the 1271 differentially expressed genes generated in the four clusters, a proportion estimate for each cluster was calculated in a CIBERSORT deconvolution analysis. PE > 0.5 was set as the threshold value to cluster the whole samples in TCGA-PRAD cohort into five subtypes, including luminal subtype A (30.0%), B (26.0%), and non-luminal subtype C (14.7%), D (4.2%), and mixed (25.0%).
They focused on the subtypes of luminal subtypes A and B and non-luminal subtypes C and D and elucidated the distinct clinicopathological features and molecular alterations among the four subtypes. The luminal A and B subtypes were characterized by luminal cells; the former was associated with a high level of adipogenesis and androgen response, and the latter was associated with a high level of spermatogenesis and protein secretion and was therefore termed luminal S. The non-luminal subtype C was characterized by endothelial cells and immunocytes and was related to a high level of cell cycle and angiogenesis, and D was related to a high level of DNA repair gene sets. Interestingly, luminal A represented ERG fusion-negative PCa as the overexpression of SPINK1 and TFF3, which have been validated as the coexpressed genes in ERG fusion-negative tumors, while luminal B represented ERG fusion-positive PCa [Citation84]. Elevated neuroendocrine PCa markers was observed in non-luminal C and D subtypes, such as CHGA, NKX2-1, or CEACAM5, and thus represented neuroendocrine PCa [Citation85]. Neuroendocrine PCa is characterized by its highly aggressive tendencies, shorter OS and poor responsiveness to ADT [Citation86,Citation87]. Consistently, non-luminal C and D subtypes exhibited a higher GS and more advanced TNM stages than luminal subtypes, indicating a poor prognosis. They applied the classification in three extra PCa cohorts with different clinical features, which included localized non-indolent cohort CPC-GENE 2017, mCRPC cohort SU2C-PCF2019 and early-onset PCa (age < 55) cohort DKFZ 2018. The results showed that 93% of the localized PCa were luminal subtypes, 85% of early-onset PCa belonged to the luminal A subtype, and 88% of mCRPC consisted of the non-luminal C subtype. The unified clinical and genetic outcomes highlighted the classification accuracy. Meanwhile, non-luminal C and D presented the clinical and molecular characteristics of aggressive variant PCa (AVPC), including high GS, advanced T/N stage, PTEN and TP53 alterations.
AVPC is a phenotypic syndrome that has a shorter interval (less than 6 months) than CRPC following initial hormonal therapy according to the definition [Citation88]. Han et al. utilized the AR activity score, which was reported in TCGA, to reflect AR transcriptional activity. In line with expectations, the lowest score was found in the two AVPC subtypes, suggesting a poor response to AR inhibitors. To evaluate the responsiveness to docetaxel, they enrolled tumour drug response-related genes and analysed them via ssGSEA algorithms. Luminal B and non-luminal C might be potential responders to docetaxel, while luminal A and non-luminal D might not. They also revealed that the AR activation and loss of KDM5D expression resulted in PCa resistance to docetaxel, and luminal A showed the lowest level of KDM5D. This evidence supports the value of the novel classification for clinical decision-making. For the luminal A phenotype with the strongest AR activity, AR target agents might be the most eligible options, but if PCa develops to the advanced stage, taxanes should be avoided [Citation49]. Non-luminal D represents the phenotype that might be resistant to both AR inhibitors and docetaxel, and DNA damage-inducing chemotherapies would be triable.
The PSA-encoded gene KLK3 is an AR target gene and a factor for the AR activity score [Citation36]. Han and colleagues found a higher level of KLK3 mRNA in luminal subtypes than in AVPC subtypes with the same tumor burden. The ACP3 gene encodes the expression of prostatic acid phosphatase (PAP), which was used as an early marker for PCa detection [Citation89]. Luminal subtypes also exhibited a higher expression level of ACP3 than AVPC subtypes. Computer-based drug susceptibility testing and serological characteristics and retrospective cohort, they hypothesized and identified the value of PSA/PAP in predicting PFS after docetaxel in mCRPC, and a higher pretreatment PSA/PAP ratio represented a shorter PFS, although there was no difference in OS.
Taken together, Han and colleagues provided a new idea for the construction of PCa molecular typing. They highlighted the bias introduced by stromal cells when using whole-transcriptome analysis of tumour tissues, and the use of single-cell analysis would allow for a better focus on prostate epithelial cell-relevant heterogeneity. It is worth mentioning that their risk model is linked to the existing knowledge frameworks, which could deepen our understanding of AVPC and facilitate clinical decision-making. Docetaxel is the first chemotherapy drug added to treat mCRPC, but only certain patients would benefit from it [Citation90]. PCa with a high PSA/PAP ratio, which might be recognized as luminal A or AVPC-M subtypes, cannot benefit from docetaxel and should receive other treatments.
Immune-related classification
To improve the response rate of immunotherapy in PCa, our team has proposed a robust immunogenomic classification based on NMF algorithms. A total of 495 transcriptome profiles from TCGA-PRAD cohort were virtually microdissected via NMF algorithms into 11 factors, and the immunologically relevant factor, also named immune factor, was selected and characterized by 150 exemplar genes which were identified via ssGSEA algorithms. With consensus clustering and multidimensional scaling random forest, 495 patients were divided into immune and nonimmune phenotypes. It has been validated that a strong stromal response is negatively correlated with immune activation and that TGF-β, WNT and C-ECM are highly relevant to stromal status [Citation91,Citation92]. For the immune phenotype, patients with high activation of these pathways were therefore further defined as immune-activated subgroups, and the rest were immune-suppressed subgroups. All the patients in the TCGA-PRAD cohort were eventually classified into immune-activated (14.9%), immune-suppressed (25.5%) and nonimmune subtypes (59.6%) [Citation93].
The three newly defined subtypes have a tight association with clinicopathological features. Most patients in the immune-suppressed subgroup had a higher T stage (T > 2), higher GS (GS >7) and poorer RFS than those in the immune-activated and nonimmune subgroups. PCa is generally considered a ‘cold tumour’ due to its features of a non-immunoreactive and immunosuppressive TME, low expression of PD-L1 and a low infiltration pattern of T cells but an elevated number of regulator T cells, indicating a weak probability of response to immune checkpoint inhibitor (ICI) therapy. However, many clinical trials have shown that a subset of PCa patients would sustainably benefit from CTLA-4 inhibitors and anti-PD-1 inhibitors [Citation94–96], which implies that PCa contains a specific phenotype with a relatively higher TME and immunocyte infiltration. Increasing evidence has revealed that the heterogeneity of the response is from complex genotype mutations in PCa. Antonarakis and colleagues reported that two out of four patients (50%) with both mismatch repair (MMR) and MSH6 mutations showed a response to anti-PD-1 therapy [Citation97]. Abida et al. reviewed 1551 tumours from 1346 PCa patients and found that 3.1% of patients had high microsatellite instability (MSI) or MMR mutations. Among the 11 patients out of the 3.1% who received anti-PD-1/anti-PD-L1 therapy, six experienced a clinically meaningful PSA response greater than 50% [Citation98]. In addition, ATM or BRCA1/2 mutations were also found to be related to sensitivity to pembrolizumab, and patients with ATM or BRCA1/2 mutations had an ORR of 12%, which was higher than those without [Citation99]. Along with the single molecular alterations mentioned above, this immune-related classifier supplied another available tool for selecting sensitive patients to receive anti-PD-1 therapy. For the immune-activated phenotype, monotherapy with anti-PD-1 might be sufficient to meet clinical requirements, whereas for the immune-activated phenotype and nonimmune phenotype, the goal is to reverse the ‘cold tumour’ to a ‘hot tumour’. Ongoing clinical trials revealed that combination immune checkpoint inhibitors with other drugs, such as tyrosine kinase inhibitors, chemotherapy, radiopharmaceuticals, radiation, poly-ADP ribose polymerase inhibitors, A2B adenosine receptor inhibitors, IL-2 or CD11b agonists, have the potential to modulate the TME and reverse immunotherapy resistance in PCa, thereby bringing a new dawn for nonimmune-activated phenotypes [Citation12,Citation100–107].
We reappeared the NFM algorithm in a real-world AHMU-PC cohort along with three external cohorts obtained from GEO datasets, and all patients were successfully divided into three identical subgroups. The immune-suppressed subgroup was confirmed to have a poorer RFS and higher GS and pathological T stage, and patients in the immune-activated subgroup were sensitive to anti-PD-1 therapy. CD163 is a biomarker of macrophages, and α-SMA is a biomarker of stromal activation. Immunohistochemistry obtained similar results with the NFM algorithm, indicating that the three subtypes can be classified via immunohistochemistry and alleviate the economic burden for patients.
In addition, the immune classification elucidated the correlation between three newly defined subtypes and published classifications. A total of 495 patients in the TCGA-PRAD cohort were classified into luminal A, luminal B and basal phenotypes using PAM50 algorithms. We found that immune-activated phenotypes contained more luminal A patients, while more luminal B patients were enriched in the immune-suppressed subclass. Immunogenomic analysis assigned tumors into six subtypes, including wound healing, IFN-γ dominant, inflammatory, lymphocyte depleted, immunologically quiet, and TGF-β dominant [Citation108]. This study reported that 92% of immune-activated patients belong to the inflammatory subtype, which is characterized by high Th17-cell infiltration as well as an immune-activated phenotype. The high degree of consistency and reproducibility between different molecular classifications indicated that the heterogeneity of PCa is traceable, and comprehensive molecular profiling is gradually uncovering the pattern. Based on the MutsigCV algorithm, different mutation landscapes among the three subtypes were revealed, and the immune-activated phenotype had a higher SPOP, which was previously reported in the PCS1 subtype, which represented a poor prognosis phenotype. Despite similar SPOP patterns appearing both in the two subtypes, they exhibit contrary clinical outcomes, implying that the weight of SPOP for prognosis in PCa is debatable.
We constructed this classification based on the TME features and focused on predicting the responsiveness to immunotherapy. The three immunotypes can be recognized by immunohistochemistry, indicating the potential for clinical use at low cost. In addition, the successful establishment of this risk model re-emphasized that PCa is a complex of tumor cells and the microenvironment.
Multi-omics classification
We first proposed a multiomics classification for PCa based on the package ‘MOVICS’, which was newly proposed by our team [Citation109]. We downloaded the multiomics profiles from the TCGA platform to identify the survival-relevant subtypes. Five PCa cohorts that recorded detail clinicopathological information were enrolled as external validation cohorts, including the GSE54460, GSE70770, MSKCC, GSE116918, and AHMU-PC cohorts. We enrolled the most relevant molecules to RFS from each omics for the subsequent analysis, which included 1526 mRNA, 242 lncRNA, 30 miRNA, 1073 DNA CpG methylation sites, and 23 mutant genes. The clustering prediction index and Gaps-statistics were conducted to identify the optimal numbers of clusters. Ten multi-omics clustering algorithms in the “MOVICS” package were performed to obtain the best cluster configuration. Three distinct subgroups were eventually identified, termed PMOC1, PMOC2, and PMOC3 [Citation110]. The three PMOCs exhibited diverse clinicopathological characteristics. Compared to PMOC1 and PMOC3, PMOC2 was related to a higher T stage, indicating a poor phenotype, while PMOC3 had the longest RFS.
The three phenotypes exhibited distinct molecular features. The PMOC1 subtype exhibited elevated immune checkpoint proteins, aberrant immune-associated pathways, and activated inflammatory metabolic pathways. Nicotinamide adenine dinucleotide is one of the essential modulators of immune-metabolic circuits [Citation111], cyclooxygenase regulates immunity and lipid metabolism by catalyzing the conversion of arachidonic acid to prostaglandins [Citation112], and the cortisol and aldosterone biosynthesis pathways have also been reported to be correlated with tumor inflammation [Citation113]. Therefore, PMOC1 was also termed the inflammatory subtype. As the worst phenotype, PMOC2 activated the cell cycle and DNA repair pathways. The predictive value of CDK1 in PCa was reported as early as 2011 [Citation114], and an orally active CDK inhibitor, PD-0332991, was developed for this target, which can delay proliferation of HSPC and CRPC cells [Citation115]. The most frequent genetic alterations were also observed in the PMOC2 subtype, which contained mutations of TP53 and APC and copy number amplification of 8q24.21. Compared to wild-type APC, mutated APC decreased mRNA expression and was related to an elevated recurrence probability. In an APC-deficient mouse model, the tumorigenesis of PCa occurs early to 7 months, which might be correlated with the activation of Wnt/β-catenin signalling. After restoring APC expression in APC-deficient mice, the cell proliferation and differentiation of intestinal crypt epithelium cells gradually returned to normal [Citation116,Citation117]. Meanwhile, the PMOC2 subtype showed the highest copy number alteration at the 8q24.21 region, which has been identified to be related to the inherited risk of PCa and the level of PSA [Citation118, Citation119]. These findings can advance our understanding of the genetic susceptibility to high-risk prostate cancer and poor-prognosis PCa with a low PSA, but the detailed connections remain to be elucidated. The PMOC3 subtype enriched numerous oncogenic signalling pathways, such as epithelial mesenchymal transition and PI3K/AKT pathways, as well as activated proinflammatory pathways, such as Notch and TNF-α signaling. Collectively, substantial suppression pathway activation antagonizes the action of the oncogenic pathway, resulting in a relatively favourable clinical outcome for PMOC3, which is therefore called the balance subtype. Furthermore, the POMC3 subtype presented the highest androgen activity score and AR activation signature, which was consistent with the high sensitivity to androgen receptor signalling inhibitors (ARSI) or bicalutamide. Transcriptional network analysis also revealed many relevant transcriptions factor regulons, including AR, HIF1A, STAT3, and KLF4. Siu et al. [Citation120] reported that KLF4 is an activator of AR through reciprocal feedback, which means that not only is KLF4 impacted by AR through transcription, but KLF4 can also promote the expression of AR. In hypoxia settings, glucose-6-phosphate isomerase (GPI) can be inhibited by AR and contribute to suppress cell growth, whereas ARSI treatment can restore the expression of GPI and switch the pattern to hypoxia-induced glycolysis. This evidence suggests that the AR and HIF1α regulons can switch glucose metabolism in the TME [Citation121].
Taken together, we constructed a novel classification system for PCa based on multi-omics data and a newly developed R package. Compared with single omics, multi-omics analysis integrates information at several omics levels, provides more evidence for biological mechanisms, identifies candidate key factors from a deep level, integrates information between different levels, builds a gene regulatory network, and in-depth elucidation of the regulatory and causation between relevant molecules to deeper understand the molecular mechanism and genetic alterations of complex phenotypes in biological behaviours and disease processes. The multiomics classification had considerable overlap with the PAM50 and TCGA classification. For instance, we applied the PMOC algorithms in the PAM50 classification, refined the luminal B subtype and found survival differences still remained within the luminal B subtype. Luminal B/PMOC2 had significantly shorter RFS than luminal B/PMOC1 and luminal B/PMOC3, and we speculated that activation of immune pathways is responsible for this difference. Although PAM50 classification demonstrated excellent diagnostic performance, its origin in breast cancer is inherently flawed, which contributes to the survival heterogeneity within luminal B tumours. As noted above, combining PAM50 with characterized molecules in PCa would be more helpful in identifying high-risk patients, such as multiomics features.
Conclusion and perspectives
The eight classifications represent the advances in the molecular taxonomy of PCa. The basis for classification evolves from single-molecular alteration to multi-omics signatures, while different molecular classifications still show a relatively stable consistency. For example, the immune-activated subtype corresponds to the PAM50/luminal A subtype, the immune-suppressed subtype overlaps with the PAM50/luminal B subtype, and their judgments on clinical prognosis are basically the same. Yoon et al. compared the gene expression patterns and clinical indications between PAM50 and PCS classification schemes, and found they are surprisingly similar in conserved gene expression status and survival outcomes, while PCS performance better than PAM50 in distinguishing luminal or basal sources [Citation122]. It was worth mentioning that several clinical studies were undergoing to further validate the clinical value of molecular classification in PCa. For instance, PAM50 was used in NRG-GU-006 to test whether it can identify patients sensitive to apalutamide, and also as a predictive marker of docetaxel therapy in E3805 CHAARTED. This is a big step forward in molecular typing research, and we hope that there will be more clinical trials to test molecular typing. In addition, we observed a high level of AR activation among many subtypes, PCS/PCS2, PAM50/luminal A, epithelial-defined/luminal A and multi-omics/PMOC3 represented the favourable phenotypes, contradicting previous studies in which high AR expression related to a poor clinical outcome. One possible explanation is that AR is not the only driver of PCa, and more complex molecular mechanisms regulate the prognosis of PCa, which are urgent to explore ().
Figure 2. The subtypes with high activation of androgen receptor, and their prognostic features, sensitivity to androgen-deprivation therapy (ADT), and molecular features.
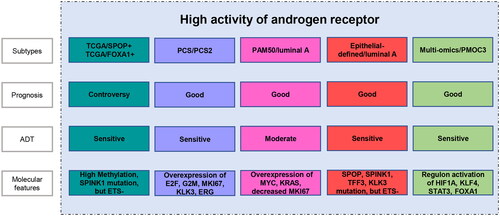
Molecular diagnosis provides important information for clinical diagnosis and treatment, but it still has the following limitations: ① At present, molecular subtypes are mainly based on transcriptome, gene mutation or DNA methylation. The proteomics and immune status of tumors are also closely related, so multi-omics combined typing is more meaningful; ② PCa usually shows both intertumoral and intratumor heterogeneity, while present classifications were established based on bulk sequencing and will not reveal the intratumor heterogeneity, in this setting, classifications established based on single-cell sequencing might provide a chance for solving this dilemma; ③ With the development of PCa, molecular changes are also dynamic, therefore, it is important to identify stable molecules to establish consensus classification systems; ④ Almost all of the conclusions come from bioinformatics analyses, and higher level of evidence for molecular classification is needed, which included cell, animal experiments or longitudinal clinical trials.
Taken together, the complex constellation of genomic and epigenetic diversity has been gradually revealed over the past decades, and in parallel, the development of robust methods such as machine learning provides a chance to better integrate multidimensional data. In this context, molecular classification would be more reliable and can be a supplementary method for traditional clinicopathological typing.
Authors’ contributions
CL, JM and QG conceived, designed and interpreted this study. QG, JL, FY, XT and MZ drafted the manuscript and collected the related references. QG, JL and FY contributed to the revision of final manuscript. ZH, CL and JM participated in discussion and designed this review and inspected the manuscript. All authors contributed to this manuscript. All authors read and approved the final manuscript.
Ethics approval and consent to participate
Not applicable.
Consent for publication
Not applicable.
Availability of data and material
Not applicable.
Acknowledgements
We greatly appreciate all participants in this study.
Disclosure statement
No potential conflict of interest was reported by the author(s).
Additional information
Funding
References
- Wang L, Lu B, He M, et al. Prostate cancer incidence and mortality: global status and temporal trends in 89 countries from 2000 to 2019. Front Public Health. 2022;10:1. doi: 10.3389/fpubh.2022.811044.
- Ferlay J, Soerjomataram I, Dikshit R, et al. Cancer incidence and mortality worldwide: sources, methods and major patterns in GLOBOCAN 2012. Int J Cancer. 2015;136(5):E359–19.
- Krieger KL, Gohlke JH, Lee KJ, et al. Repair-assisted damage detection reveals biological disparities in prostate cancer between African Americans and European Americans. Cancers (Basel). 2022;14(4):1012. doi: 10.3390/cancers14041012.
- Plym A, Zhang Y, Stopsack KH, et al. Family history of prostate and breast cancer integrated with a polygenic risk score identifies men at highest risk of dying from prostate cancer before age 75 years. Clin Cancer Res. 2022;28(22):4926–4933. doi: 10.1158/1078-0432.CCR-22-1723.
- Shore N. Management of early-stage prostate cancer. Am J Manag Care. 2014;20(12 Suppl):S260–S72.
- Gillessen S, Bossi A, Davis ID, et al. Management of patients with advanced prostate cancer. Part I: intermediate-/high-risk and locally advanced disease, biochemical relapse, and side effects of hormonal treatment: report of the advanced prostate cancer consensus conference 2022. Eur Urol. 2023;83(3):267–293. doi: 10.1016/j.eururo.2022.11.002.
- Sandhu S, Moore CM, Chiong E, et al. Prostate cancer. Lancet. 2021;398(10305):1075–1090. doi: 10.1016/S0140-6736(21)00950-8.
- Armstrong AJ, Szmulewitz RZ, Petrylak DP, et al. ARCHES: a randomized, phase III study of androgen deprivation therapy with enzalutamide or placebo in men with metastatic Hormone-Sensitive prostate cancer. Prostate. 2022;82(13):1237–1247. doi: 10.1200/JCO.19.00799.
- Kyriakopoulos CE, Chen YH, Carducci MA, et al. Chemohormonal therapy in metastatic Hormone-Sensitive prostate cancer: long-term survival analysis of the randomized phase III E3805 CHAARTED trial. J Clin Oncol. 2018;36(11):1080–1087. doi: 10.1200/JCO.2017.75.3657.
- Wang L, Dehm SM, Hillman DW, et al. A prospective genome-wide study of prostate cancer metastases reveals association of wnt pathway activation and increased cell cycle proliferation with primary resistance to abiraterone acetate-prednisone. Ann Oncol. 2018;29(2):352–360. doi: 10.1093/annonc/mdx689.
- Shih TC, Liu R, Wu CT, et al. Targeting galectin-1 impairs castration-resistant prostate cancer progression and invasion. Clin Cancer Res. 2018;24(17):4319–4331. doi: 10.1158/1078-0432.CCR-18-0157.
- de Bono J, Mateo J, Fizazi K, et al. Olaparib for metastatic castration-resistant prostate cancer. N Engl J Med. 2020;382(22):2091–2102. doi: 10.1056/NEJMoa1911440.
- Chi KN, Sandhu S, Smith MR, et al. Niraparib plus abiraterone acetate with prednisone in patients with metastatic castration-resistant prostate cancer and homologous recombination repair gene alterations: second interim analysis of the randomized phase III MAGNITUDE trial. Ann Oncol. 2023;34(9):772–782. doi: 10.1016/j.annonc.2023.06.009.
- Stark JR, Perner S, Stampfer MJ, et al. Gleason score and lethal prostate cancer: does 3 + 4 = 4 + 3? J Clin Oncol. 2009;27(21):3459–3464. doi: 10.1200/JCO.2008.20.4669.
- Iwamoto H, Izumi K, Kadono Y, et al. Prognosis of patients with prostate cancer and Middle range prostate - specific antigen levels of 20 - 100 ng/mL. Int Braz J Urol. 2019;45(1):61–67. doi: 10.1590/S1677-5538.IBJU.2018.0143.
- Van Poppel H, Albreht T, Basu P, et al. Serum PSA-based early detection of prostate cancer in Europe and globally: past, present and future. Nat Rev Urol. 2022;19(9):562–572. doi: 10.1038/s41585-022-00638-6.
- Williams IS, McVey A, Perera S, et al. Modern paradigms for prostate cancer detection and management. Med J Aust. 2022;217(8):424–433. doi: 10.5694/mja2.51722.
- Sun C, Yang D, Zhu J, et al. Modified the 8th AJCC staging system for patients with advanced prostate cancer: a study based on SEER database. BMC Urol. 2022;22(1):185. doi: 10.1186/s12894-022-01135-z.
- D’Amico AV, Whittington R, Malkowicz SB, et al. Biochemical outcome after radical prostatectomy, external beam radiation therapy, or interstitial radiation therapy for clinically localized prostate cancer. Jama. 1998;280(11):969–974. doi: 10.1001/jama.280.11.969.
- Chang AJ, Autio KA, Roach M, 3rd, et al. High-risk prostate cancer-classification and therapy. Nat Rev Clin Oncol. 2014;11(6):308–323. doi: 10.1038/nrclinonc.2014.68.
- Roach M, Lu J, Pilepich MV, et al. Four prognostic groups predict long-term survival from prostate cancer following radiotherapy alone on radiation therapy oncology group clinical trials. Int J Radiat Oncol Biol Phys. 2000;47(3):609–615. doi: 10.1016/s0360-3016(00)00578-2.
- Cooperberg MR, Broering JM, Carroll PR. Risk assessment for prostate cancer metastasis and mortality at the time of diagnosis. J Natl Cancer Inst. 2009;101(12):878–887. doi: 10.1093/jnci/djp122.
- Huang J, Vicini FA, Williams SG, et al. Percentage of positive biopsy cores: a better risk stratification model for prostate cancer? Int J Radiat Oncol Biol Phys. 2012;83(4):1141–1148. doi: 10.1016/j.ijrobp.2011.09.043.
- Yossepowitch O, Eggener SE, Bianco FJ, Jr., et al. Radical prostatectomy for clinically localized, high risk prostate cancer: critical analysis of risk assessment methods. J Urol. 2007;178(2):493–499; discussion 499. doi: 10.1016/j.juro.2007.03.105.
- Gosselaar C, Kranse R, Roobol MJ, et al. The interobserver variability of digital rectal examination in a large randomized trial for the screening of prostate cancer. Prostate. 2008;68(9):985–993. doi: 10.1002/pros.20759.
- Tomlins SA, Alshalalfa M, Davicioni E, et al. Characterization of 1577 primary prostate cancers reveals novel biological and clinicopathologic insights into molecular subtypes. Eur Urol. 2015;68(4):555–567. doi: 10.1016/j.eururo.2015.04.033.
- Andreoiu M, Cheng L. Multifocal prostate cancer: biologic, prognostic, and therapeutic implications. Hum Pathol. 2010;41(6):781–793. doi: 10.1016/j.humpath.2010.02.011.
- Miller GJ, Cygan JM. Morphology of prostate cancer: the effects of multifocality on histological grade, tumor volume and capsule penetration. J Urol. 1994;152(5 Pt 2):1709–1713. doi: 10.1016/s0022-5347(17)32368-6.
- Faisal FA, Sundi D, Tosoian JJ, et al. Racial variations in prostate cancer molecular subtypes and androgen receptor signaling reflect anatomic tumor location. Eur Urol. 2016;70(1):14–17. doi: 10.1016/j.eururo.2015.09.031.
- Haffner MC, Zwart W, Roudier MP, et al. Genomic and phenotypic heterogeneity in prostate cancer. Nat Rev Urol. 2021;18(2):79–92. doi: 10.1038/s41585-020-00400-w.
- Mills IG. Molecular subtyping of prostate cancer: a partnership model. Eur Urol. 2015;68(4):568–569. doi: 10.1016/j.eururo.2015.05.012.
- Galletti G, Matov A, Beltran H, et al. ERG induces taxane resistance in castration-resistant prostate cancer. Nat Commun. 2014;5(1):5548. doi: 10.1038/ncomms6548.
- Brenner JC, Ateeq B, Li Y, et al. Mechanistic rationale for inhibition of poly(ADP-ribose) polymerase in ETS gene fusion-positive prostate cancer. Cancer Cell. 2011;19(5):664–678. doi: 10.1016/j.ccr.2011.04.010.
- Scheving LA. Primary amino acid sequence similarity between human epidermal growth factor-urogastrone, human pancreatic secretory trypsin inhibitor, and members of porcine secretin family. Arch Biochem Biophys. 1983;226(2):411–413. doi: 10.1016/0003-9861(83)90309-0.
- Yang J, Zhang W. New molecular insights into osteosarcoma targeted therapy. Curr Opin Oncol. 2013;25(4):398–406. doi: 10.1097/CCO.0b013e3283622c1b.
- The molecular taxonomy of primary prostate cancer. Cell. 2015;163(4):1011–1025. doi: 10.1016/j.cell.2015.10.025.
- Baena E, Shao Z, Linn DE, et al. ETV1 directs androgen metabolism and confers aggressive prostate cancer in targeted mice and patients. Genes Dev. 2013;27(6):683–698. doi: 10.1101/gad.211011.112.
- Noushmehr H, Weisenberger DJ, Diefes K, et al. Identification of a CpG island methylator phenotype that defines a distinct subgroup of glioma. Cancer Cell. 2010;17(5):510–522. doi: 10.1016/j.ccr.2010.03.017.
- Figueroa ME, Abdel-Wahab O, Lu C, et al. Leukemic IDH1 and IDH2 mutations result in a hypermethylation phenotype, disrupt TET2 function, and impair hematopoietic differentiation. Cancer Cell. 2010;18(6):553–567. doi: 10.1016/j.ccr.2010.11.015.
- Chandran UR, Ma C, Dhir R, et al. Gene expression profiles of prostate cancer reveal involvement of multiple molecular pathways in the metastatic process. BMC Cancer. 2007;7(1):64. doi: 10.1186/1471-2407-7-64.
- Schaeffer EM, Marchionni L, Huang Z, et al. Androgen-induced programs for prostate epithelial growth and invasion arise in embryogenesis and are reactivated in cancer. Oncogene. 2008;27(57):7180–7191. doi: 10.1038/onc.2008.327.
- Massie CE, Mills IG, Lynch AG. The importance of DNA methylation in prostate cancer development. J Steroid Biochem Mol Biol. 2017;166:1–15. doi: 10.1016/j.jsbmb.2016.04.009.
- Hieronymus H, Lamb J, Ross KN, et al. Gene expression signature-based chemical genomic prediction identifies a novel class of HSP90 pathway modulators. Cancer Cell. 2006;10(4):321–330. doi: 10.1016/j.ccr.2006.09.005.
- Correction For G, et al. Prostate cancer-associated mutations in speckle-type POZ protein (SPOP) regulate steroid receptor coactivator 3 protein turnover. Proc Natl Acad Sci U S A. 2019;116(28):14386–14387.
- An J, Wang C, Deng Y, et al. Destruction of full-length androgen receptor by wild-type SPOP, but not prostate-cancer-associated mutants. Cell Rep. 2014;6(4):657–669. doi: 10.1016/j.celrep.2014.01.013.
- Geng C, Rajapakshe K, Shah SS, et al. Androgen receptor is the key transcriptional mediator of the tumor suppressor SPOP in prostate cancer. Cancer Res. 2014;74(19):5631–5643. doi: 10.1158/0008-5472.CAN-14-0476.
- Chen Y, Chi P, Rockowitz S, et al. ETS factors reprogram the androgen receptor cistrome and prime prostate tumorigenesis in response to PTEN loss. Nat Med. 2013;19(8):1023–1029. doi: 10.1038/nm.3216.
- Yu J, Yu J, Mani RS, et al. An integrated network of androgen receptor, polycomb, and TMPRSS2-ERG gene fusions in prostate cancer progression. Cancer Cell. 2010;17(5):443–454. doi: 10.1016/j.ccr.2010.03.018.
- Boysen G, Rodrigues DN, Rescigno P, et al. SPOP-Mutated/CHD1-Deleted lethal prostate cancer and abiraterone sensitivity. Clin Cancer Res. 2018;24(22):5585–5593. doi: 10.1158/1078-0432.CCR-18-0937.
- Abida W, Armenia J, Gopalan A, et al. Prospective genomic profiling of prostate cancer across disease states reveals germline and somatic alterations that may affect clinical decision making. J Clin Oncol Precis Oncol. 2017;2017(1):1–16 doi: 10.1200/PO.17.00029.
- Guo ZQ, Zheng T, Chen B, et al. Small-molecule targeting of E3 ligase adaptor SPOP in kidney cancer. Cancer Cell. 2016;30(3):474–484. doi: 10.1016/j.ccell.2016.08.003.
- Bernasocchi T, El Tekle G, Bolis M, et al. Dual functions of SPOP and ERG dictate androgen therapy responses in prostate cancer. Nat Commun. 2021;12(1):734. doi: 10.1038/s41467-020-20820-x.
- You S, Knudsen BS, Erho N, et al. Integrated classification of prostate cancer reveals a novel luminal subtype with poor outcome. Cancer Res. 2016;76(17):4948–4958. doi: 10.1158/0008-5472.CAN-16-0902.
- Levine DM, Haynor DR, Castle JC, et al. Pathway and gene-set activation measurement from mRNA expression data: the tissue distribution of human pathways. Genome Biol. 2006;7(10):R93. doi: 10.1186/gb-2006-7-10-r93.
- Brunet JP, Tamayo P, Golub TR, et al. Metagenes and molecular pattern discovery using matrix factorization. Proc Natl Acad Sci U S A. 2004;101(12):4164–4169. doi: 10.1073/pnas.0308531101.
- Thysell E, Ylitalo EB, Jernberg E, et al. A systems approach to prostate cancer classification-letter. Cancer Res. 2017;77(24):7131–7132. doi: 10.1158/0008-5472.CAN-16-3231.
- You S, Freeman MR. A systems approach to prostate cancer classification-response. Cancer Res. 2017;77(24):7133–7135. doi: 10.1158/0008-5472.CAN-17-0239.
- Watson PA, Arora VK, Sawyers CL. Emerging mechanisms of resistance to androgen receptor inhibitors in prostate cancer. Nat Rev Cancer. 2015;15(12):701–711. doi: 10.1038/nrc4016.
- Parker JS, Mullins M, Cheang MC, et al. Supervised risk predictor of breast cancer based on intrinsic subtypes. J Clin Oncol. 2009;27(8):1160–1167. doi: 10.1200/JCO.2008.18.1370.
- Zhao SG, Chang SL, Erho N, et al. Associations of luminal and basal subtyping of prostate cancer with prognosis and response to androgen deprivation therapy. JAMA Oncol. 2017;3(12):1663–1672. doi: 10.1001/jamaoncol.2017.0751.
- Smith MR, Antonarakis ES, Ryan CJ, et al. Phase 2 study of the safety and antitumor activity of apalutamide (ARN-509), a potent androgen receptor antagonist, in the high-risk nonmetastatic castration-resistant prostate cancer cohort. Eur Urol. 2016;70(6):963–970. doi: 10.1016/j.eururo.2016.04.023.
- Karthaus WR, Iaquinta PJ, Drost J, et al. Identification of multipotent luminal progenitor cells in human prostate organoid cultures. Cell. 2014;159(1):163–175. doi: 10.1016/j.cell.2014.08.017.
- Mian OY, Tendulkar RD, Abazeed ME. The evolving role of molecular profiling in prostate cancer: basal and luminal subtyping transcends tissue of origin. Transl Cancer Res. 2017;6(Suppl 9):S1441–S1445. doi: 10.21037/tcr.2017.10.49.
- Ylitalo EB, Thysell E, Jernberg E, et al. Subgroups of castration-resistant prostate cancer bone metastases defined through an inverse relationship between androgen receptor activity and immune response. Eur Urol. 2017;71(5):776–787. doi: 10.1016/j.eururo.2016.07.033.
- Omlin A, Pezaro C, Gillessen Sommer S. Sequential use of novel therapeutics in advanced prostate cancer following docetaxel chemotherapy. Ther Adv Urol. 2014;6(1):3–14. doi: 10.1177/1756287213509677.
- Frey BJ, Dueck D. Clustering by passing messages between data points. Science. 2007;315(5814):972–976. doi: 10.1126/science.1136800.
- Pérez-Guaita D, Kuligowski J, Lendl B, et al. Assessment of discriminant models in infrared imaging using constrained repeated random sampling - cross validation. Anal Chim Acta. 2018;1033:156–164. doi: 10.1016/j.aca.2018.05.019.
- Thysell E, Vidman L, Ylitalo EB, et al. Gene expression profiles define molecular subtypes of prostate cancer bone metastases with different outcomes and morphology traceable back to the primary tumor. Mol Oncol. 2019;13(8):1763–1777. doi: 10.1002/1878-0261.12526.
- Cai C, He HH, Chen S, et al. Androgen receptor gene expression in prostate cancer is directly suppressed by the androgen receptor through recruitment of lysine-specific demethylase 1. Cancer Cell. 2011;20(4):457–471. doi: 10.1016/j.ccr.2011.09.001.
- Gao S, Gao Y, He HH, et al. Androgen receptor tumor suppressor function is mediated by recruitment of retinoblastoma protein. Cell Rep. 2016;17(4):966–976. doi: 10.1016/j.celrep.2016.09.064.
- Yang S, Jiang M, Grabowska MM, et al. Androgen receptor differentially regulates the proliferation of prostatic epithelial cells in vitro and in vivo. Oncotarget. 2016;7(43):70404–70419. doi: 10.18632/oncotarget.11879.
- Korsen JA, Kalidindi TM, Khitrov S, et al. Molecular imaging of neuroendocrine prostate cancer by targeting Delta-like ligand 3. J Nucl Med. 2022;63(9):1401–1407. doi: 10.2967/jnumed.121.263221.
- Chen M, Lingadahalli S, Narwade N, et al. TRIM33 drives prostate tumor growth by stabilizing androgen receptor from Skp2-mediated degradation. EMBO Rep. 2022;23(8):e53468.
- Wierstra I, Alves J. FOXM1, a typical proliferation-associated transcription factor. Biol Chem. 2007;388(12):1257–1274. doi: 10.1515/BC.2007.159.
- Chesnokov MS, Halasi M, Borhani S, et al. Novel FOXM1 inhibitor identified via gene network analysis induces autophagic FOXM1 degradation to overcome chemoresistance of human cancer cells. Cell Death Dis. 2021;12(7):704. doi: 10.1038/s41419-021-03978-0.
- Barakat DJ, Zhang J, Barberi T, et al. CCAAT/enhancer binding protein β controls androgen-deprivation-induced senescence in prostate cancer cells. Oncogene. 2015;34(48):5912–5922. doi: 10.1038/onc.2015.41.
- Collado M, Serrano M. Senescence in tumours: evidence from mice and humans. Nat Rev Cancer. 2010;10(1):51–57. doi: 10.1038/nrc2772.
- Coppé JP, Desprez PY, Krtolica A, et al. The senescence-associated secretory phenotype: the dark side of tumor suppression. Annu Rev Pathol. 2010;5(1):99–118. doi: 10.1146/annurev-pathol-121808-102144.
- Iglesias-Gato D, Thysell E, Tyanova S, et al. The proteome of prostate cancer bone metastasis reveals heterogeneity with prognostic implications. Clin Cancer Res. 2018;24(21):5433–5444. doi: 10.1158/1078-0432.CCR-18-1229.
- Ge G, Han Y, Zhang J, et al. Single-Cell RNA-seq reveals a developmental hierarchy Super-Imposed over subclonal evolution in the cellular ecosystem of prostate cancer. Adv Sci (Weinh). 2022;9(15):e2105530.
- Peng S, Hu P, Xiao YT, et al. Single-Cell analysis reveals EP4 as a target for restoring T-Cell infiltration and sensitizing prostate cancer to immunotherapy. Clin Cancer Res. 2022;28(3):552–567. doi: 10.1158/1078-0432.CCR-21-0299.
- Han H, Lee HH, Choi K, et al. Prostate epithelial genes define therapy-relevant prostate cancer molecular subtype. Prostate Cancer Prostatic Dis. 2021;24(4):1080–1092. doi: 10.1038/s41391-021-00364-x.
- Henry GH, Malewska A, Joseph DB, et al. A cellular anatomy of the normal adult human prostate and prostatic urethra. Cell Rep. 2018;25(12):3530–3542 e5. doi: 10.1016/j.celrep.2018.11.086.
- Terry S, Nicolaiew N, Basset V, et al. Clinical value of ERG, TFF3, and SPINK1 for molecular subtyping of prostate cancer. Cancer. 2015;121(9):1422–1430. doi: 10.1002/cncr.29233.
- Lee JK, Bangayan NJ, Chai T, et al. Systemic surfaceome profiling identifies target antigens for immune-based therapy in subtypes of advanced prostate cancer. Proc Natl Acad Sci U S A. 2018;115(19):E4473–e82.
- Spetsieris N, Boukovala M, Patsakis G, et al. Neuroendocrine and aggressive-variant prostate cancer. Cancers (Basel). 2020;12(12):3792. doi: 10.3390/cancers12123792.
- Islam R, Mishra J, Polavaram NS, et al. Neuropilin-2 axis in regulating secretory phenotype of neuroendocrine-like prostate cancer cells and its implication in therapy resistance. Cell Rep. 2022;40(3):111097. doi: 10.1016/j.celrep.2022.111097.
- von Amsberg G, Zilles M, Mansour W, et al. Salvage chemotherapy with cisplatin, ifosfamide, and paclitaxel in aggressive variant of metastatic castration-resistant prostate cancer. Int J Mol Sci. 2022;23(23):14948.
- Xu H, Wang F, Li H, et al. Prostatic acid phosphatase (PAP) predicts prostate cancer progress in a Population-Based study: the renewal of PAP? Dis Markers. 2019;2019:7090545–7090510. doi: 10.1155/2019/7090545.
- Karanika S, Karantanos T, Kurosaka S, et al. GLIPR1-ΔTM synergizes with docetaxel in cell death and suppresses resistance to docetaxel in prostate cancer cells. Mol Cancer. 2015;14(1):122. doi: 10.1186/s12943-015-0395-0.
- Batlle E, Massagué J. Transforming growth factor-β signaling in immunity and cancer. Immunity. 2019;50(4):924–940. doi: 10.1016/j.immuni.2019.03.024.
- Monteran L, Erez N. The dark side of fibroblasts: cancer-Associated fibroblasts as mediators of immunosuppression in the tumor microenvironment. Front Immunol. 2019;10:1835. doi: 10.3389/fimmu.2019.01835.
- Meng J, Zhou Y, Lu X, et al. Immune response drives outcomes in prostate cancer: implications for immunotherapy. Mol Oncol. 2021;15(5):1358–1375. doi: 10.1002/1878-0261.12887.
- Mirzaei S, Paskeh MDA, Okina E, et al. Molecular landscape of LncRNAs in prostate cancer: a focus on pathways and therapeutic targets for intervention. J Exp Clin Cancer Res. 2022;41(1):214. doi: 10.1186/s13046-022-02406-1.
- Wang F, Wu L, Yin L, et al. Combined treatment with anti-PSMA CAR NK-92 cell and anti-PD-L1 monoclonal antibody enhances the antitumour efficacy against castration-resistant prostate cancer. Clin Transl Med. 2022;12(6):e901.
- Jagodinsky JC, Bates AM, Clark PA, et al. Local TLR4 stimulation augments in situ vaccination induced via local radiation and anti-CTLA-4 checkpoint blockade through induction of CD8 T-cell independent Th1 polarization. J Immunother Cancer. 2022;10(10):e005103. doi: 10.1136/jitc-2022-005103.
- Antonarakis ES, Shaukat F, Isaacsson Velho P, et al. Clinical features and therapeutic outcomes in men with advanced prostate cancer and DNA mismatch repair gene mutations. Eur Urol. 2019;75(3):378–382. doi: 10.1016/j.eururo.2018.10.009.
- Abida W, Cheng ML, Armenia J, et al. Analysis of the prevalence of microsatellite instability in prostate cancer and response to immune checkpoint blockade. JAMA Oncol. 2019;5(4):471–478. doi: 10.1001/jamaoncol.2018.5801.
- Antonarakis ES, Piulats JM, Gross-Goupil M, et al. Pembrolizumab for treatment-refractory metastatic castration-resistant prostate cancer: multicohort, open-label phase II KEYNOTE-199 study. J Clin Oncol. 2020;38(5):395–405. doi: 10.1200/JCO.19.01638.
- García-Aranda M, Redondo M. Targeting protein kinases to enhance the response to anti-PD-1/PD-L1 immunotherapy. Int J Mol Sci. 2019;20(9):2296.
- Leonetti A, Wever B, Mazzaschi G, et al. Molecular basis and rationale for combining immune checkpoint inhibitors with chemotherapy in non-small cell lung cancer. Drug Resist Updat. 2019;46:100644. doi: 10.1016/j.drup.2019.100644.
- van Bussel MTJ, Awada A, de Jonge MJA, et al. A first-in-man phase 1 study of the DNA-dependent protein kinase inhibitor peposertib (formerly M3814) in patients with advanced solid tumours. Br J Cancer. 2021;124(4):728–735. doi: 10.1038/s41416-020-01151-6.
- Shaverdian N, Lisberg AE, Bornazyan K, et al. Previous radiotherapy and the clinical activity and toxicity of pembrolizumab in the treatment of non-small-cell lung cancer: a secondary analysis of the KEYNOTE-001 phase 1 trial. Lancet Oncol. 2017;18(7):895–903. doi: 10.1016/S1470-2045(17)30380-7.
- Wei Q, Costanzi S, Balasubramanian R, et al. A2B adenosine receptor blockade inhibits growth of prostate cancer cells. Purinergic Signal. 2013;9(2):271–280. doi: 10.1007/s11302-012-9350-3.
- Diab A, Tannir NM, Bentebibel SE, et al. Bempegaldesleukin (NKTR-214) plus nivolumab in patients with advanced solid tumors: phase I dose-escalation study of safety, efficacy, and immune activation (PIVOT-02). Cancer Discov. 2020;10(8):1158–1173. doi: 10.1158/2159-8290.CD-19-1510.
- Panni RZ, Herndon JM, Zuo C, et al. Agonism of CD11b reprograms innate immunity to sensitize pancreatic cancer to immunotherapies. Sci Transl Med. 2019;11(499):eaau9240. doi: 10.1126/scitranslmed.aau9240.
- Bansal D, Reimers MA, Knoche EM, et al. Immunotherapy and immunotherapy combinations in metastatic castration-resistant prostate cancer. Cancers (Basel). 2021;13(2):334. doi: 10.3390/cancers13020334.
- Thorsson V, Gibbs DL, Brown SD, et al. The immune landscape of cancer. Immunity. 2018;48(4):812–830.e14. doi: 10.1016/j.immuni.2018.03.023.
- Lu X, Meng J, Zhou Y, et al. MOVICS: an R package for multi-omics integration and visualization in cancer subtyping. Bioinformatics. 2020;36(22-23):5539–5541.
- Meng J, Lu X, Jin C, et al. Integrated multi-omics data reveals the molecular subtypes and guides the androgen receptor signalling inhibitor treatment of prostate cancer. Clin Transl Med. 2021;11(12):e655.
- Singhal A, Cheng CY. Host NAD + metabolism and infections: therapeutic implications. Int Immunol. 2019;31(2):59–67. doi: 10.1093/intimm/dxy068.
- Tian JJ, Lei CX, Ji H, et al. Role of cyclooxygenase-mediated metabolites in lipid metabolism and expression of some immune-related genes in juvenile grass carp (ctenopharyngodon idellus) fed arachidonic acid. Fish Physiol Biochem. 2017;43(3):703–717. doi: 10.1007/s10695-016-0326-z.
- Ferreira NS, Tostes RC, Paradis P, et al. Aldosterone, inflammation, immune system, and hypertension. Am J Hypertens. 2021;34(1):15–27. doi: 10.1093/ajh/hpaa137.
- Ding Z, Wu CJ, Chu GC, et al. SMAD4-dependent barrier constrains prostate cancer growth and metastatic progression. Nature. 2011;470(7333):269–273. doi: 10.1038/nature09677.
- Comstock CE, Augello MA, Goodwin JF, et al. Targeting cell cycle and hormone receptor pathways in cancer. Oncogene. 2013;32(48):5481–5491. doi: 10.1038/onc.2013.83.
- Bruxvoort KJ, Charbonneau HM, Giambernardi TA, et al. Inactivation of apc in the mouse prostate causes prostate carcinoma. Cancer Res. 2007;67(6):2490–2496. doi: 10.1158/0008-5472.CAN-06-3028.
- Dow LE, O’Rourke KP, Simon J, et al. Apc restoration promotes cellular differentiation and reestablishes crypt homeostasis in colorectal cancer. Cell. 2015;161(7):1539–1552. doi: 10.1016/j.cell.2015.05.033.
- Lin X, Wu Y, Liu F, et al. A germline variant at 8q24 contributes to the serum p2PSA level in a chinese prostate biopsy cohort. Front Oncol. 2021;11:753920. doi: 10.3389/fonc.2021.753920.
- Hoffmann TJ, Passarelli MN, Graff RE, et al. Genome-wide association study of prostate-specific antigen levels identifies novel loci independent of prostate cancer. Nat Commun. 2017;8(1):14248. doi: 10.1038/ncomms14248.
- Siu MK, Suau F, Chen WY, et al. KLF4 functions as an activator of the androgen receptor through reciprocal feedback. Oncogenesis. 2016;5(12):e282–e282. doi: 10.1038/oncsis.2016.79.
- Geng H, Xue C, Mendonca J, et al. Interplay between hypoxia and androgen controls a metabolic switch conferring resistance to androgen/AR-targeted therapy. Nat Commun. 2018;9(1):4972. doi: 10.1038/s41467-018-07411-7.
- Yoon J, Kim M, Posadas EM, et al. A comparative study of PCS and PAM50 prostate cancer classification schemes. Prostate Cancer Prostatic Dis. 2021;24(3):733–742. doi: 10.1038/s41391-021-00325-4.