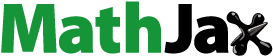
Abstract
The intricate web of cancer biology is governed by the active participation of long non-coding RNAs (lncRNAs), playing crucial roles in cancer cells’ proliferation, migration, and drug resistance. Pioneering research driven by machine learning algorithms has unveiled the profound ability of specific combinations of lncRNAs to predict the prognosis of cancer patients. These findings highlight the transformative potential of lncRNAs as powerful therapeutic targets and prognostic markers. In this comprehensive review, we meticulously examined the landscape of lncRNAs in predicting the prognosis of the top five cancers and other malignancies, aiming to provide a compelling reference for future research endeavours. Leveraging the power of machine learning techniques, we explored the predictive capabilities of diverse lncRNA combinations, revealing their unprecedented potential to accurately determine patient outcomes.
KEY MESSAGES
lncRNAs play crucial roles in cancer biology, regulating proliferation, migration, and drug resistance.
Emerging evidence suggests that machine learning can predict cancer prognosis using specific lncRNA combinations.
Comprehensive information on the predictive abilities of lncRNA combinations in oncology concerning machine learning is lacking.
This review offers up-to-date vital references on diverse lncRNA combinations pertinent to future research and clinical trials.
Introduction
Most genes in the human genome are non-coding genes [Citation1]. A non-coding RNA (ncRNA) lacks an open, readable frame and is non-transcribable and untranslatable. Long-chain non-coding RNAs (lncRNA) is a functional RNA molecules transcribed from RNA polymerase II, with a 5′- terminal methylation cap and 3′-terminal poly A tail, similar to mRNA in structure and more than 200 nucleotides [Citation2]. For a long time, lncRNA has been considered genomic transcription noise. Recent studies have found that lncRNAs play a role in regulating gene expression, including gene silencing, chromatin modification, epigenetic gene expression, transcription or post-transcriptional modification and translation [Citation3], thus participating in biological processes such as cell proliferation, cell cycle regulation, and apoptosis [Citation4–7].
lncRNAs are closely related to human diseases, especially cancer, including breast cancer [Citation8, Citation9], prostate cancer [Citation10, Citation11], lung cancer [Citation12, Citation13], and other cancers. Abnormal expression of lncRNAs may be a key regulatory factor for cancer occurrence. lncRNAs may play different biological roles in various cancers, such as promoting angiogenesis in breast cancer tissue [Citation14], combining with miRNAs to accelerate the invasion of lung cancer cells [Citation15], regulating cancer drug resistance [Citation16], and influencing cancer susceptibility [Citation17].
Machine learning, a captivating scientific discipline, empowers computers to learn from data and has sparked growing interest in its application to clinical and biological challenges [Citation18, Citation19]. Divided into supervised, unsupervised, and reinforcement learning, machine learning encompasses a range of algorithms like linear regression, support vector machines (SVM), nearest neighbours (KNN), and decision trees, to name a few [Citation18, Citation19]. With its versatility, machine learning has made remarkable strides in various fields, particularly healthcare, by offering predictive insights such as disease incidence or patient prognosis [Citation20, Citation21]. This article provides an in-depth review of how machine learning can be utilised to predict the prognosis of the most commonly occurring cancers: breast cancer, lung cancer, colorectal cancer, prostate cancer, and gastric cancer. Specifically, it focuses on the examination of lncRNAs as potential markers for prognosis. By leveraging the power of machine learning algorithms and analysing the outcomes, this review aims to serve as a valuable reference for future research in this field.
lncRNAs in the prognostic prediction of the top five cancer types
Breast cancer (BC)
BC is the leading cause of death in women; the prevalence of BC exceeded that of lung cancer in 2020, with more than 685,000 deaths, making BC the most common cancer globally [Citation22]. According to the estimation, nearly 680 million women worldwide suffered from BC in 2018 [Citation23]. In addition to women, a minority of men have BC [Citation23]. BC is characterized by high morbidity and mortality [Citation23]. Breast cancers with different histological subtypes and risk profiles have traditionally been diagnosed using histopathological characteristics like tumour size, grade, and lymph node involvement. However, in the last decade, a more refined classification based on intrinsic molecular subtypes has emerged, including luminal A, luminal B, basal, ERBB2, and normal-like, each associated with distinct clinical features and responses to treatment [Citation24,Citation25].
The clinical model of multiple lncRNA is associated with BC prognosis prediction [Citation26]. Meng et al. [Citation26] used the BRB-Array tool to perform univariate Cox proportional hazard regression analysis on the lncRNA expression clinical profile data samples of 887 BC patients. They found that 30 lncRNAs were closely related to the overall survival rate of BC patients [Citation26]. Random survival forest model analysis yielded a predictive marker model for 4-lncRNA (lncRNA U79277, AK024118, BC0204, AK000974) [Citation26].
The univariate Cox regression model and risk score analysis showed that these 4-lncRNAs were closely related to the survival rate of patients and could be used for survival prediction [Citation26]. ROC analysis showed that the 4-lncRNA gene characteristics were highly consistent with the genetic characteristics of the prognostic prediction group of Oncotype DX in clinical BC recurrence risk assessment, indicating that the 4-lncRNA marker has certain clinical practicability [Citation26].
Zhou et al. [Citation27] analyzed mRNASeq gene expression data of 1222 subjects (1101 BC patients, 104 normal, 17 replication data) using a weighted gene co-expression network, competitive endogenous RNA network and screened 23 hub lncRNA biomarkers. Univariate Cox regression model analysis and ROC curve evaluation revealed an 8-lncRNA marker (AC007731.1, AL513123.1, C10orf126, WT1-AS, TLR8-AS1, HOTAIR, ADAMTS9-AS1, SRGAP3-AS2) that was significantly associated with BC clinical prognosis [Citation27]. Finally, 8-lncRNA markers were used to test the experimental cohort of 251 patients, and it was found that 8-lncRNA had the value of clinical prognosis prediction [Citation27].
Liu et al. [Citation28] used a univariate Cox regression model to establish a 7-lncRNA (MAPT-IT1, SLC26A4-AS1, VPS9D1-AS1, PCAT18, LINC01234, SPATA41, LINC01215).
Stratified analysis showed that 7-lncRNA markers could be used to predict the overall survival prognosis of BC with multiple clinicopathological risk factors, and the prediction efficiency can be improved by evaluating and analyzing different degrees of immune cell infiltration and BC cell burden [Citation28]. In addition, it has been proved that different molecular subtypes of BC are rich in different BC stem cells [Citation29]. The 12-lncRNA marker (Z68871.1,LINC00578, AC097639.1, AP003119.3, AP001207.3, LINC00668, AL122010.1, AC245297.3, LINC01871, AP000851.2, AC022509.2, and SEMA3B-AS1) associated with BC stem cell regulation [Citation30] has been verified to be highly correlated with BC prognosis.
Li et al. also categorized the genetic data samples of BC patients from the TCGA database into a high-risk group and a low-risk group [Citation30]. They found that the status of 12-lncRNA-related BC stem cells in the low-risk and high-risk groups was different, which may be an indicator for evaluating the prognosis of BC patients [Citation30]. However, additional related functional experiments and molecular mechanism research investigations are required to confirm this study. The integrated lncRNA biomarkers can improve prediction accuracy. Since most studies are still in the initial stage of machine learning, there is still much room for the clinical application of multi-lncRNA biological prediction models in prognosis prediction.
Lung cancer (LC)
According to the global cancer statistics of 2020, LC stands as the second most prevalent malignancy worldwide [Citation22]. About 1.8 million people are diagnosed with LC yearly, and about 1.6 million die from it [Citation31]. Five-year survival rates range from 1% to 5%, depending on stage and region [Citation32]. Long-term smoking is an important risk factor for LC [Citation33]. LC is categorized into two main groups; small-cell lung cancer (SCLC) and non-small cell lung cancer (NSCLC) [Citation34]. Non-small cell lung cancer (NSCLC) constitutes 80% of all lung cancer cases, posing the most significant threat to the well-being of lung cancer patients. Within NSCLC, it is categorized into lung adenocarcinoma (LUAD) and lung squamous cell carcinoma (LUSC) based on the histological type and clinical stage assessed using a CT image model [Citation35]. Clinical staging for NSCLC patients involves three grades: I, II, and III [Citation36]. Diagnosing early-stage LC poses a challenge due to its asymptomatic nature or the absence of specific symptoms [Citation34]. It is typically discovered through chest radiographs or chest CTs [Citation34]. The most routine LC screening method is low-dose CT[Citation37], but the probability of screening out early LC is not high. Finding new biomarkers is a crucial objective to advance the diagnosis and treatment of LC, particularly due to the challenges associated with its early [Citation38]. lncRNAs hold promising potential as prognostic indicators in this regard [Citation39].
Zhang [Citation40] et al. collected data from 227 SCLC patients, including 79 samples from the gene expression synthesis (GEO) open database (GSE60052), and 148 cases with formalin-fixed and paraffin-embedded (FFPE) tissues; the minimum absolute contraction and selection operator (LASSO) model was adopted to identify immune-related lncRNA (irLncRNA) with SCLC characteristics, a marker of 8-lncRNA(ENOX1-AS1, AC005162, LINC00092, RPL34-AS1, AC104135, AC015971, AC126544, AP001189) was established. The preliminary verification showed that this marker could predict the prognosis and adjuvant chemotherapy response of NSCLC patients [Citation40].
The utilization of an 8-lncRNA marker has demonstrated the ability to effectively stratify and accurately predict various risk factors in patients with SCLC [Citation40]. In cohort validation studies, it has been further substantiated that the 8-lncRNA marker can independently predict the overall survival time, relapse-free survival time, and efficacy of chemotherapy in patients with SCLC. As identified by the marker, high-risk patients exhibit poorer prognosis and derive lesser benefit from adjuvant chemotherapy than low-risk patients [Citation40].
Strong immune-related lncRNA can predict the prognosis and immunotherapy response of lung adenocarcinoma (LUAD). Sun et al. [Citation41] comprehensively analyzed and calculated the lncRNA, immune and clinical samples of 115 immune cell lines, 187 NSCLC cell lines, and 1533 NSCLC patients, and determined the lncRNA markers (TILSig) composed of 7 lncRNA related to tumour immune infiltration, including HCP526, PSMB8-AS1, TNRC6C-AS1, and CARD8-AS1. Further analysis of the correlation between TILSig and immune checkpoint gene expression showed that a high TILSig level was associated with increased immune checkpoint gene expression and high immune checkpoint inhibitory gene expression was associated with poor prognosis in patients [Citation41]. The survival time was significantly prolonged for patients lacking this gene marker and low immune checkpoint gene expression [Citation41]. Taking together, the clinical applicability of the 7-lncRNA marker has been established, making it a viable predictive model for NSCLC in future research endeavours [Citation41].
Cao et al. [Citation42] obtained differentially expressed immune-related lncRNA(irLncRNA) by crossing the transcriptome data set of the Cancer Genome Map (TCGA)-LUAD queue and ImmLnc database. The model was constructed by selecting irRNA pairs with the highest ranking and differential expression based on the ranking algorithm, and 33 pairs of irLncRNA prediction signals were established in the TCGA-LUAD training set [Citation42]. According to these immune signals and the overall survival rate of LUAD patients, LUAD patients were categorized into low-risk and high-risk groups [Citation42]. In contrast, the low-risk patients had high immunogenicity and a good survival rate, while the high-risk patients showed the opposite characteristics and were highly sensitive to toxic drugs [Citation42]. The aforementioned characteristics have the potential to provide valuable guidance in the clinical application of anti-LUAD drugs and immunotherapy. They hold substantial clinical significance in predicting the prognosis, tumour immunogenicity, and treatment response of LUAD patients [Citation42].
Recent research showed neutrophil extracellular traps (NETs) are closely related to cancer progression [Citation43,Citation44]. Fang et al. [Citation45] identified a NETs-related co-expression network consisting of 12 lncRNAs and revealed the correlations among NSCLC and NETs-related genes and lncRNAs. A 12 lncRNAs predictive model was constructed by univariate Cox regression analysis and Lasso Cox regression. By evaluating 12 NETs-related lncRNAs, they were able to acquire the risk score of each patient [Citation45]. Next, the Kaplan-Meier curve suggested that patients in the high-risk group had a significantly worse OS than their low-risk counterparts [Citation45]. At the same time, further verification revealed that the expressions of the 3 adverse prognostic lncRNAs (AP000695.2, MYOSLID, and NKILA) at the NSCLC cell level align with previous research [Citation46,Citation47]. The expression of three lncRNAs increased in the NETs-treated group compared to the untreated group, confirming the predictive model above. However, one of the drawbacks of this study is that the probable mechanisms of NETs-related lncRNAs remain unknown in the absence of functional enrichment analysis [Citation45].
LncRNAs can also influence cancer progression by modifying the lactate metabolism [Citation48, Citation49]. Mai et al. [Citation50] found the lactate metabolism-related lncRNAs (LMR-lncRNAs) by the GO enrichment analysis and established a 14 LMR-lncRNAs prognostic signature based on univariate Cox regression findings to calculate the risk score of patients with Lung adenocarcinoma. The risk score was closely related to patients’ overall survival (OS), in which patients in the high-risk group exhibited a shorter OS than those in the low-risk group [Citation50]. Further bioinformatic analysis illustrated that the LMR-lncRNA signature might be associated with MYC targets, glycolysis, hypoxia, PI3K/AKT/mTOR signalling, fatty acid metabolism, interferon-gamma response, bile acid metabolism, and inflammatory response [Citation50]. Then, they comprehensively analyzed the relation of LMRs-lncRNAs signature and ICB Molecules, TIICs, Chemotherapy, and Targeted Therapy. Results revealed that the risk model could predict chemosensitivity and guide the use of targeted therapeutics and chemotherapeutics in the clinic [Citation50].
Colorectal cancer (CRC)
CRC is the most common malignant tumour in the gastrointestinal tract [Citation22]. According to the global cancer statistics in 2020, CRC has become the third most common cancer in the world in terms of incidence rate and the second most common cancer in terms of mortality [Citation22]. CRC typically originates from the growth of polyps, which can eventually become cancerous [Citation51]. CRC is known to have both hereditary and sporadic forms, and it generally develops gradually over several years through the adenoma-cancer sequence [Citation52]. The occurrence of sporadic CRC is related to long-term colorectal inflammation [Citation52]. Most early symptoms of CRC are not obvious and easy to ignore [Citation53]. Endoscopy and pathological biopsy have become routine methods for screening CRC [Citation54]. Unfortunately, the early detection rate of colorectal cancer (CRC) remains low due to the absence of highly sensitive and specific laboratory tests [Citation54].
The unfavourable prognosis of colorectal cancer (CRC) is primarily attributed to its tendency to metastasize to distant sites, notably the liver, and the presence of cancer cells with high stem cell characteristics [Citation55]. Studies have demonstrated that the utilisation of compound lncRNA markers can enhance the accuracy of prognosis prediction in CRC [Citation56–58]. These markers represent a novel biomarker with predictive value and have the potential to serve as a valuable tool in predicting the prognosis of CRC patients [Citation56,Citation59–69].
Liu et al. [Citation57] analyzed the gene expression profiles of 459 non-metastatic CRC samples and 87 metastatic CRC samples in the TCGA dataset and found 628 dysfunctional differentials expressed mRNAs, 144 dysfunctional lncRNAs, and 25 dysfunctional miRNAs. Subsequently, miRcode results showed that the predicted 9 miRNAs interacted with 12 lncRNAs, and finally, 12 related lncRNAs were screened to construct a ceRNA network [Citation57]. Multivariate Cox regression analysis confirmed that a 3-lncRNA (LINC00114, LINC00261, and HOTAIR) marker was an independent factor in predicting CRC [Citation57].
In addition, Cheng et al. [Citation58] conducted lncRNA analysis on 39 non-tumour samples and 398 tumour samples in the (TCGA) database and screened 26 related lncRNAs using univariate Cox regression analysis and gene set enrichment analysis (GSEA), Through LASSO regression analysis and multivariate Cox regression analysis, it was finally determined that another group of 6-lncRNA (AC125603.2, LINC00909, AC016876.1, MIR210HG, AC009237.14, and LINC01063) composite labelling group [Citation58] was closely related to the prognosis of patients. The c-index was calculated according to the AUC of the ROC curve [Citation58]. The results show that the 6-lncRNA risk assessment model has high accuracy in predicting the overall survival rate of CRC patients, which may become a powerful indicator for clinical prognosis prediction [Citation58]. Xing et al. [Citation70] also downloaded 326 samples (285 tumour samples and 41 normal tissue samples adjacent to the tumour) through the TCGA data portal, used edger + lima for differential expression analysis, and identified 169 differentially expressed lncRNAs. KM survival analysis of differentially expressed lncRNAs yielded 17 lncRNAs significantly related to survival. A group of lncRNAs with the lowest error rate was selected to form a 14 lncRNA (AP000525.9, LL22NC03-N64E9.1, RP11-115D19.1, MAMDC2-AS1, DRAIC, BVES-AS1, PGM5-AS1, RP11-353N14.4, RP11-542B15. 1, BMS1P17, LINC01234, RP11-10A14.5, LINC01354, and BMS1P18) marker [Citation70]. Additional survival analysis, incorporating BVES-AS1, revealed a distinct separation of survival curves among groups of patients with high or low-risk scores in both the training and test datasets. This significant separation signifies the robust predictive capability of the 14 lncRNA characteristics. In a study conducted by Liu et al. [Citation71], an immune-related lncRNA signature was developed using a machine-learning integration approach. The researchers proposed that this signature outperformed traditional clinical variables, molecular characteristics, and 109 other composite markers in terms of prediction accuracy. However, further confirmation through animal experiments, cell experiments, and clinical studies is necessary to validate its efficacy.
Ferroptosis is a newly defined form of regulated cell death driven by the loss of activity of the lipid repair enzyme, glutathione peroxidase 4 (GPX4) and the subsequent accumulation of lipid-based reactive oxygen species (ROS), particularly lipid hydroperoxides [Citation72]. Ferroptosis may have a tumour suppressor function that could be harnessed for cancer therapy [Citation73,Citation74]. Based on Spearman correlation analysis, Wu et al. [Citation49] constructed a 4-ferroptosis-related lncRNAs (FRLs) signature after 705 FRLs were identified. Next, Cox univariate regression analysis demonstrated that the risk score of this signature was associated with the OS rates of colon adenocarcinoma patients, suggesting that individuals from the high-risk group may have higher mortality rates than low-risk individuals [Citation75]. They performed GSEA, GO and KEGG analysis to find the potential biological functions and signalling pathways between the risk groups classified by the 4-FRL signature [Citation75]. Results revealed that the risk score of the 4-lncRNA signature was mainly related to tumour metastasis, tumour immunity, biological metabolism and drug resistance in CRC [Citation75]. Also, they explored the relationship between the ferroptosis-related signature and antitumor immunity. Results showed the expression levels of many immune checkpoints, such as PD-1, PD-L1, and CTL4A, were higher in the high-risk than in the low-risk group [Citation75]. Therefore, these patients might benefit from many immune checkpoint blockades [Citation76], which might improve the prognosis of high-risk patients by enhancing their immunoreactivity or inducing ferroptosis [Citation75].
Hypoxia is the most common phenomenon in solid tumours and causes high oxygen consumption by cancer cells and abnormal blood supply in the tumour tissue [Citation77]. Luan et al. [Citation78] established and validated a 6- hypoxia-immune–related lncRNAs signature to predict the prognosis of patients with CRC. Later, they divided the patients into low- and high-risk groups based on the signature and found significant differences in the tumour microenvironment and immunotherapy response between the two groups [Citation78]. Functional analysis suggested that immune-related pathways may be important mechanisms for hypoxia-immune-related lncRNAs in regulating the tumour immune microenvironment and progression of CRC [Citation78]. To explore the sensitivity to different drugs, they identified 18 drugs with significant IC50 values between the two groups [Citation78]. In conclusion, the risk signature established in this study can better predict the sensitivity of CRC patients to immunotherapy and has a reference value for the choice of immunotherapy for CRC patients [Citation78]. They constructed a hypoxia-immune–related lncRNAs–miRNA–mRNA ceRNA network to explain the potential regulatory molecular mechanism mediated by lncRNAs [Citation78].
Prostate cancer (PCa)
PCa is a common malignant tumour in elderly men [Citation22]. In 2020, PCa became the second most common tumour and the fifth leading cause of death in men worldwide, with a low 5-year survival rate [Citation22]. It is estimated that there are nearly 1.4 million new cases and 375,000 deaths from PCa worldwide [Citation22]. The male mortality rate in European and American countries is generally higher than in Asian countries [Citation22]. Most early-stage PCa do not present with apparent clinical symptoms [Citation22]. The main causes of death in patients with advanced PCa are metastatic diseases, such as bone metastasis [Citation79], lung metastasis [Citation80], and liver metastasis [Citation81]. Patients with bone metastasis have the highest mortality rate among all patient groups. At present, androgen-deprivation therapy (ADT) is one of the main treatments. According to the tumour’s ADT response, prostate cancer can be divided into hormone-sensitive prostate cancer (HSPC) and castration-resistant prostate cancer (CRPC) [Citation82–84]. Recent studies have found that many lncRNAs are expressed in PCa, which can be used as a new marker for PCa prognosis prediction.
Existing studies have found that lncRNAs are associated with the treatment and prognosis of PCa [Citation85]. Li et al. [Citation85] collected RNA-seq data and clinical information from 495 PCa tissues and 52 adjacent PCa tissues in the TCGA database. By comparing the TCGA and GTEx databases, they observed that SNHG9 exhibited elevated expression levels in cancer tissues compared to normal tissues [Citation85]. Subsequent ROC curve analysis revealed that the expression level of SNHG9 could serve as a distinguishing marker between PCa and non-tumour tissues [Citation85].
Kaplan-Meier analysis was then used to assess the correlation between SNHG9 expression and progression-free survival (PFS) in PCa patients [Citation85]. The results showed that SNHG9 expression was associated with shorter PFS in PCa patients [Citation85]. Finally, Cox univariate and multivariate analysis showed that SNHG9 was an independent prognostic factor for PFS in PCa patients [Citation85]. This provides some new test methods for predicting the prognosis of PCa patients. Chen et al. [Citation86] obtained differentially expressed genes (DEG)/lncRNA (DELs) and miRNA (DEM) by analyzing the microarray expression profile GSE32269 (22 local and 29 bone metastasis PCa samples), microarray expression profile GSE26964 (6 primary PCa samples and 7 bone metastasis PCa samples), and mRNA and miRNA expression profiles of 490 patients with prostate adenocarcinoma (PRAD) in the TCGA database. Univariate Cox regression analysis of TCGA data revealed 7 DELs (INE1, SNHG20, LINC00342, PVT1, HCG18, MCM3AP-AS1, and LINC01399) in the co-expression and ceRNA networks [Citation86]. In the context of PCa bone metastasis, the study identified 7 differentially expressed miRNAs (hsa-miR-301b, hsa-miR-508, hsa-miR-326, hsa-miR-127, hsa-miR-330, hsa-miR-532, and hsa-miR-331) and 73 differentially expressed genes (DEGs) that exhibited significant associations. Notably, the study highlighted two specific lncRNAs, namely HCG18 and MCM3AP-AS1, as the most significantly associated LncRNAs in relation to PCa bone metastasis [Citation86]. According to the median expression value of each gene, the samples were divided into a high-expression group and a low-expression group, and then the Kaplan-Meier survival curve was drawn [Citation86]. It was found that co-expression (HCG18/MCM3AP-AS1-KNTC1) or ceRNA axis (MCM3AP-AS1-has-miR-508-3p-DTL and HCG1/MCM3AP-AS1-has-miR-127-3p-CDKN3) were also important candidate biomarkers for PCa bone metastasis and prognosis [Citation86]. lncRNA HCG18 and MCM3AP-AS1 may be important targets for treating PCa metastasis and improving poor prognosis [Citation86]. The results of valproic acid targeting HCG18 and MCM3AP-AS1 show that it may become a potential small-molecule drug for treating PCa [Citation86].
Ferroptosis has been confirmed to induce diverse physiological conditions, contributing to the progression of many diseases, such as malignant tumours, including PCa [Citation87]. Based on LASSO regression, five FRLs were selected to construct a risk model to determine the risk score of patients with PCa. In the training group, 330 patients were categorized into low-risk and high-risk groups. The survival curves revealed that the patients in the high-risk group had a shorter PSA-free survival time [Citation88]. Verification group results suggested that the five frlncRNAs, which could transform the immune landscape, could be used to evaluate the risk of experiencing biochemical relapse (BCR) [Citation88]. The Next GSEA discovered that frlncRNAs influence PCa development via traditional metabolic pathways, implying that regulating FRLs levels could be a potential cancer therapy [Citation88].
Gastric cancer (GC)
GC is one of the malignant cancers threatening human health. National cancer statistics for 2020 show that GC is the fifth most common cancer and the third most fatal human cancer [Citation22]. Despite advances in surgical techniques, radiotherapy, chemotherapy, and neoadjuvant therapy, mortality rates of approximately 75% were maintained in most parts of the world by 2020 [Citation89]. The molecular characteristics of primary gastric cancer were analyzed based on the Cancer Genome Atlas (TCGA) [Citation90]. GC was divided into four subtypes: microsatellite-unstable MSI-H tumours, EBV-positive tumours, tumours showing chromosome instability (CIN), and genome-stable (GS) tumours [Citation91]. Presently, endoscopic pathological biopsies, CT, endoscopic ultrasonography, PET, and laparoscopic staging are the primary methods used to diagnose and treat GC [Citation92]. Although there has been a gradual decrease in the occurrence of GC in recent decades, the 5-year overall survival rate for GC patients remains low. This is partially because patients with GC often do not exhibit symptoms in the early stages of the disease [Citation92]. By the time symptoms become noticeable and the disease is diagnosed as advanced GC, there is a higher likelihood of metastasis, leading to a dismal prognosis and limited effectiveness of chemotherapy in GC patients [Citation93]. To increase patient survival rates, efforts should be made to provide markers that can be used to identify early GC development and accurately assess GC prognosis [Citation93]. lncRNA has recently become a research hotspot for GC [Citation93]. Additionally, studies have discovered the significant role of lncRNA in unravelling prognostic indicators and mechanisms related to chemotherapeutic drug resistance in GC [Citation94]. Due to the presence of moderate symptoms during the initial stages of GC and the challenges associated with machine detection, a considerable number of patients are often diagnosed with GC at an advanced stage [Citation95]. The prognosis of patients with advanced GC must be monitored by determining related indicators [Citation95]. lncRNA integration markers can be a good choice for future GC prognosis prediction.
Nie et al. [Citation96] obtained lncRNA expression data and related clinical information of 341 GC patients from the Gene Expression Omnibus (GEO) database. They constructed 14-lncRNAs (ADAMTS9-AS2, FLG-AS1, RN F144A-AS1, LINCO0922, C15orf54, LINCO1210, ERVMER61-1, POU6F2-AS2, LINCO0973, ERI CH3-AS1, LINC00326, LINCO1208, LINCO0645, and DSCR10) associated with GC prognosis through TCGA and external cohorts [Citation96]. A nomogram was constructed by combining the risk characteristics of the 14-lncRNA with relevant clinical factors of GC patients [Citation96]. Following a comprehensive risk assessment, it was observed that the identified marker holds the potential to predict the 5-year survival rate of gastric cancer (GC) patients to a certain extent. This indicates that the 14-lncRNA signature could be an effective prognostic predictor in the detection process for GC outcomes [Citation96].
Ferroptosis is a non-apoptotic form of cell death [Citation97]. Wei et al. [Citation98] carried out research on the application of lncRNA markers related to ferroptosis in prognosis prediction. A feature model consisting of four ferroptosis-related lncRNAs (AP003392.1, AC245041.2, AP001271.1, and BOLA3-AS1) was established by multivariate Cox regression analysis and TCGA dataset screening [Citation98]. Using this feature model, a nomogram was constructed by combining patient risk scores, and the prediction results showed good performance in predicting 1-year, 2-year, and 3-year overall survival (OS) of GC patients [Citation98]. Furthermore, the expression levels of these four ferroptosis-related lncRNAs were upregulated in GC cell lines by qRT-PCR [Citation98]. By detecting and observing the nomogram constructed by using 4 lncRNAs, it was found that the OS prediction results of GC patients were ideal. Studies have shown that ferroptosis-related lncRNA characteristics predict GC treatment and provide a theoretical basis for ferroptosis-related GC-targeted therapy [Citation98]. However, the interaction mechanism between ferroptosis and the above lncRNAs in GC warrants further investigation [Citation98].
LncRNA, as a new prognostic marker, can further promote the progress of individualized treatment [Citation99]. LncRNAs could become ideal new markers for GC prognosis prediction if more future clinical trials are conducted [Citation99].
Zhu et al. [Citation100] identified and constructed a 24-lncRNA signature based on 300 GC samples from the GEO database to predict the prognosis of GC patients. They also calculated the risk score for each patient based on the signature, and patients were divided into high-risk or low-risk groups. Patients in the high-risk group had significantly shorter median DFS than those in the low-risk group [Citation100]. They tested the 24-lncRNA signature independent of postoperative chemotherapy, TNM stage [Citation100]. The next GSEA they performed exhibited a high-risk score accompanied by up-regulation of several cancer-related networks, including recurrence, metastasis, and cancer stemness-associated pathways [Citation100]. This lncRNA signature may help to personalize GC prognosis prediction and act as a possible biomarker for GC prognosis [Citation100].
lncRNAs in the prognostic prediction of other cancer types
The emergence of lncRNAs as key players in cancer research is not limited to just the top five prevalent cancer types but extends to various other cancer types as well. In the context of prognostic prediction, lncRNAs have shown promise as valuable biomarkers for assessing cancer outcomes in a wide spectrum of other malignancies, including brain cancer, pancreatic cancer, liver cancer, and others, as summarized in .Their diverse mechanisms of action, such as transcriptional regulation [Citation101], metabolic reprogramming [Citation102], and immune interaction [Citation103], make them versatile candidates for deciphering the intricate landscape of cancer prognosis.
Table 1. lncRNAs as markers for prognostic prediction in other cancer types.
Development and application of lncRNA prognostic models across diverse cancer types in clinical practice
The future directions for prognosis based on lncRNAs encompass several essential factors to be considered. First, it is imperative to continue exploring and identifying lncRNAs that exhibit robust prognostic value across various cancer types. This necessitates in-depth research into the functional roles of lncRNAs in tumorigenesis and their interactions with other cellular components. Additionally, comprehensive validation through extensive clinical trials is essential to establish the reliability and accuracy of lncRNA-based prognostic models. Furthermore, efforts should focus on refining and optimizing machine learning approaches to integrate lncRNA data into clinical practice. This includes developing user-friendly tools and algorithms that can assist healthcare professionals in making informed decisions regarding cancer prognosis and treatment strategies. Ultimately, the successful incorporation of lncRNAs into cancer prognosis and treatment strategies will require collaboration between researchers, clinicians, and bioinformaticians. This interdisciplinary approach will be pivotal in translating promising findings into tangible benefits for cancer patients, offering more personalized and effective therapeutic interventions. While challenges lie ahead, the potential impact of lncRNA-based prognosis on the future of cancer care is highly promising and underscores the need for continued research and clinical validation.
Conclusion and prospect
Over the last decade, research into the role of lncRNAs in cancer diagnosis and treatment has steadily increased, becoming an emerging focus in cancer diagnosis and treatment. Also, the expression of lncRNAs may be utilized to predict cancer patients’ prognoses. Based on current studies, this article has summarized the lncRNAs utilized as prognostic markers for the top five most common cancers (), providing comprehensive and up-to-date information pertinent to future clinical trials. While certain lncRNAs can be unique to specific cancer types, others may be highly expressed in multiple types of cancer (). Though these lncRNAs present in different cancer types can serve as general cancer biomarkers for screening purposes, they lack the specificity to pinpoint a particular cancer type.
Table 2. lncRNAs as markers for prognostic prediction in the top five cancer types.
Table 3. lncRNAs in different cancers.
In terms of clinical application, research on composite lncRNA clusters as diagnostic and prognostic tools is mostly still at the initial stage of machine learning. Many clinical experiments require further verification of whether lncRNAs can become reliable new markers and therapeutic targets. However, developing new diagnostic approaches based on lncRNAs holds great promise and represents a significant advancement in cancer research. It has the potential to revolutionize cancer diagnoses and may emerge as a leading method for cancer detection in the foreseeable future.
Consent for publication
All authors read and approved the final manuscript.
Authors’ contributions
RT, JY, JZ, and LJ: conceptualisation and resources, original draft preparation. YM, JA, and WQ: original draft preparation, review, and editing. TG, XH, MF, and XL: review and editing. All authors have read and approved the final version of the manuscript.
Disclosure statement
No potential conflict of interest was reported by the author(s).
Data availability statement
The original contributions presented in the study are included in the article, further inquiries can be directed to the corresponding author.
Additional information
Funding
References
- Nurk S, Koren S, Rhie A, et al. The complete sequence of a human genome. Science. 2022;376(6588):1–16. doi:10.1126/science.abj6987.
- Chan JJ, Tay Y. Noncoding RNA: RNA regulatory networks in cancer. Int J Mol Sci. 2018;19(5):1310. doi:10.3390/ijms19051310.
- Fang Y, Fullwood MJ. Roles, functions, and mechanisms of long non-coding RNAs in cancer. GPB. 2016;14(1):42–54. doi:10.1016/j.gpb.2015.09.006.
- Loewer S, Cabili MN, Guttman M, et al. Large intergenic non-coding RNA-RoR modulates reprogramming of human induced pluripotent stem cells. Nat Genet. 2010;42(12):1113–1117. doi:10.1038/ng.710.
- Fernandes JCR, Acuña SM, Aoki JI, et al. Long non-coding RNAs in the regulation of gene expression: physiology and disease. Noncoding RNA. 2019;5(1):17. doi:10.3390/ncrna5010017.
- Yang Z, Jiang S, Shang J, et al. LncRNA: shedding light on mechanisms and opportunities in fibrosis and aging. Ageing Res Rev. 2019;52:17–31. doi:10.1016/j.arr.2019.04.001.
- Wu H, Li A, Zheng Q, et al. LncRNA LZTS1-AS1 induces proliferation, metastasis and inhibits autophagy of pancreatic cancer cells through the miR-532/TWIST1 signaling pathway. Cancer Cell Int. 2023;23(1):130. doi:10.1186/s12935-023-02979-7.
- Xu J, Wu KJ, Jia QJ, et al. Roles of miRNA and lncRNA in triple-negative breast cancer. J Zhejiang Univ Sci B. 2020;21(9):673–689. doi:10.1631/jzus.B1900709.
- Liang J, Ye C, Chen K, et al. Non-coding RNAs in breast cancer: with a focus on glucose metabolism reprogramming. Discov Oncol. 2023;14(1):72. doi:10.1007/s12672-023-00687-2.
- Misawa A, Takayama KI, Inoue S. Long non-coding RNAs and prostate cancer. Cancer Sci. 2017;108(11):2107–2114. doi:10.1111/cas.13352.
- Cheng X, Zeng Z, Yang H, et al. Novel cuproptosis-related long non-coding RNA signature to predict prognosis in prostate carcinoma. BMC Cancer. 2023;23(1):105. doi:10.1186/s12885-023-10584-0.
- Poulet C, Njock MS, Moermans C, et al. Exosomal long non-coding RNAs in lung diseases. Int J Mol Sci. 2020;21(10):3580. doi:10.3390/ijms21103580.
- Entezari M, Ghanbarirad M, Taheriazam A, et al. Long non-coding RNAs and exosomal lncRNAs: potential functions in lung cancer progression, drug resistance and tumor microenvironment remodeling. Biomed Pharmacother. 2022;150:112963. doi:10.1016/j.biopha.2022.112963.
- Zhang Q, Li T, Wang Z, et al. lncRNA NR2F1-AS1 promotes breast cancer angiogenesis through activating IGF-1/IGF-1R/ERK pathway. J Cell Mol Med. 2020;24(14):8236–8247. doi:10.1111/jcmm.15499.
- Wu W, Zhao Y, Gao E, et al. LncRNA DLEU2 accelerates the tumorigenesis and invasion of non-small cell lung cancer by sponging miR-30a-5p. J Cell Mol Med. 2020;24(1):441–450. doi:10.1111/jcmm.14749.
- Zhou Y, Sun W, Qin Z, et al. LncRNA regulation: new frontiers in epigenetic solutions to drug chemoresistance. Biochem Pharmacol. 2021;189:114228. doi:10.1016/j.bcp.2020.114228.
- Gao P, Wei GH. Genomic insight into the role of lncRNA in cancer susceptibility. Int J Mol Sci. 2017;18(6):1239. doi:10.3390/ijms18061239.
- Lip GY, Nieuwlaat R, Pisters R, et al. Refining clinical risk stratification for predicting stroke and thromboembolism in atrial fibrillation using a novel risk factor-based approach: the euro heart survey on atrial fibrillation. Chest. 2010;137(2):263–272. doi:10.1378/chest.09-1584.
- O’Mahony C, Jichi F, Pavlou M, et al. A novel clinical risk prediction model for sudden cardiac death in hypertrophic cardiomyopathy (HCM risk-SCD). Eur Heart J. 2014;35(30):2010–2020. doi:10.1093/eurheartj/eht439.
- Paixião GMM, Santos BC, Araujo RM, Ribeior MH, Moraes JL, Ribeiro: Machine Learning in Medicine: Review and Applicability. Arg Bras Cardiol. 2022;118(1):95–102.
- Moshawrab M, Adda M, Bouzouane A, et al. Reviewing federated machine learning and its use in diseases prediction. Sensors. 2023;23(4):2112. doi:10.3390/s23042112.
- Sung H, Ferlay J, Siegel RL, et al. Global cancer statistics 2020: GLOBOCAN estimates of incidence and mortality worldwide for 36 cancers in 185 countries. CA Cancer J Clin. 2021;71(3):209–249. doi:10.3322/caac.21660.
- Bray F, Ferlay J, Soerjomataram I, et al. Global cancer statistics 2018: GLOBOCAN estimates of incidence and mortality worldwide for 36 cancers in 185 countries. CA Cancer J Clin. 2018;68(6):394–424. doi:10.3322/caac.21492.
- Perou CM, Sørlie T, Eisen MB, et al. Molecular portraits of human breast tumours. Nature. 2000;406(6797):747–752. doi:10.1038/35021093.
- Sørlie T, Perou CM, Tibshirani R, et al. Gene expression patterns of breast carcinomas distinguish tumor subclasses with clinical implications. Proc Natl Acad Sci U S A. 2001;98(19):10869–10874. doi:10.1073/pnas.191367098.
- Meng J, Li P, Zhang Q, et al. A four-long non-coding RNA signature in predicting breast cancer survival. J Exp Clin Cancer Res. 2014;33(1):84. doi:10.1186/s13046-014-0084-7.
- Sun M, Wu D, Zhou K, et al. An eight-lncRNA signature predicts survival of breast cancer patients: a comprehensive study based on weighted gene co-expression network analysis and competing endogenous RNA network. Breast Cancer Res Treat. 2019;175(1):59–75. doi:10.1007/s10549-019-05147-6.
- Liu Z, Mi M, Li X, et al. A lncRNA prognostic signature associated with immune infiltration and tumour mutation burden in breast cancer. J Cell Mol Med. 2020;24(21):12444–12456. doi:10.1111/jcmm.15762.
- Bianchini G, Balko JM, Mayer IA, et al. Triple-negative breast cancer: challenges and opportunities of a heterogeneous disease. Nat Rev Clin Oncol. 2016;13(11):674–690. doi:10.1038/nrclinonc.2016.66.
- Li X, Li Y, Yu X, et al. Identification and validation of stemness-related lncRNA prognostic signature for breast cancer. J Transl Med. 2020;18(1):331. doi:10.1186/s12967-020-02497-4.
- Loyez M, Larrieu JC, Chevineau S, et al. In situ cancer diagnosis through online plasmonics. Biosens Bioelectron. 2019;131:104–112. doi:10.1016/j.bios.2019.01.062.
- Zhang Y, Wang L, Deng Y, et al. Fraxetin suppresses proliferation of non-small-cell lung cancer cells via preventing activation of signal transducer and activator of transcription 3. Tohoku J Exp Med. 2019;248(1):3–12. doi:10.1620/tjem.248.3.
- Tannenbaum SL, Koru-Sengul T, Zhao W, et al. Survival disparities in non-small cell lung cancer by race, ethnicity, and socioeconomic status. Cancer J. 2014;20(4):237–245. doi:10.1097/PPO.0000000000000058.
- Birring SS, Peake MD. Symptoms and the early diagnosis of lung cancer. Thorax. 2005;60(4):268–269. doi:10.1136/thx.2004.032698.
- Jain D, Nambirajan A, Chen G, et al. NSCLC subtyping in conventional cytology: Results of the international association for the study of lung cancer cytology working group survey to determine specific cytomorphologic criteria for adenocarcinoma and squamous cell carcinoma. J Thorac Oncol. 2022;17(6):793–805. doi:10.1016/j.jtho.2022.02.013.
- Lin J, Yu Y, Zhang X, et al. Classification of histological types and stages in non-small cell lung cancer using radiomic features based on CT images. J Digit Imaging. 2023;36(3):1029–1037. doi:10.1007/s10278-023-00792-2.
- Nooreldeen R, Bach H. Current and future development in lung cancer diagnosis. Int J Mol Sci. 2021;22(16):8661. doi:10.3390/ijms22168661.
- Riihimäki M, Hemminki A, Fallah M, et al. Metastatic sites and survival in lung cancer. Lung Cancer. 2014;86(1):78–84. doi:10.1016/j.lungcan.2014.07.020.
- Xie W, Yuan S, Sun Z, et al. Long noncoding and circular RNAs in lung cancer: advances and perspectives. Epigenomics. 2016;8(9):1275–1287. doi:10.2217/epi-2016-0036.
- Zhang Z, Luo Y, Zhang C, et al. An immune-related lncRNA signature predicts prognosis and adjuvant chemotherapeutic response in patients with small-cell lung cancer. Cancer Cell Int. 2021;21(1):691. doi:10.1186/s12935-021-02357-1.
- Sun J, Zhang Z, Bao S, et al. Identification of tumor immune infiltration-associated lncRNAs for improving prognosis and immunotherapy response of patients with non-small cell lung cancer. J Immunother Cancer. 2020;8(1):e000110. doi:10.1136/jitc-2019-000110.
- Cao K, Liu M, Ma K, et al. Prediction of prognosis and immunotherapy response with a robust immune-related lncRNA pair signature in lung adenocarcinoma. Cancer Immunol Immunother. 2022;71(6):1295–1311. doi:10.1007/s00262-021-03069-1.
- Wang W, Zhang J, Zheng N, et al. The role of neutrophil extracellular traps in cancer metastasis. Clin Transl Med. 2020;10(6):e126.
- Yang L, Liu Q, Zhang X, et al. DNA of neutrophil extracellular traps promotes cancer metastasis via CCDC25. Nature. 2020;583(7814):133–138. doi:10.1038/s41586-020-2394-6.
- Fang C, Liu F, Wang Y, et al. A innovative prognostic symbol based on neutrophil extracellular traps (NETs)-related lncRNA signature in non-small-cell lung cancer. Aging. 2021;13(13):17864–17879. doi:10.18632/aging.203289.
- Li JP, Li R, Liu X, et al. A seven immune-related lncRNAs model to increase the predicted value of lung adenocarcinoma. Front Oncol. 2020;10:560779. doi:10.3389/fonc.2020.560779.
- Zhu Q, Yang H, Cheng P, et al. Bioinformatic analysis of the prognostic value of the lncRNAs encoding snoRNAs in hepatocellular carcinoma. Biofactors. 2019;45(2):244–252. doi:10.1002/biof.1478.
- Chen F, Chen J, Yang L, et al. Extracellular vesicle-packaged HIF-1α-stabilizing lncRNA from tumour-associated macrophages regulates aerobic glycolysis of breast cancer cells. Nat Cell Biol. 2019;21(4):498–510. doi:10.1038/s41556-019-0299-0.
- Balihodzic A, Barth DA, Prinz F, et al. Involvement of long non-coding RNAs in glucose metabolism in cancer. Cancers (Basel). 2021;13(5):977. doi:10.3390/cancers13050977.
- Mai S, Liang L, Mai G, et al. Development and validation of lactate metabolism-related lncRNA signature as a prognostic model for lung adenocarcinoma. Front Endocrinol. 2022;13:829175. doi:10.3389/fendo.2022.829175.
- Nguyen LH, Goel A, Chung DC. Pathways of colorectal carcinogenesis. Gastroenterology. 2020;158(2):291–302. doi:10.1053/j.gastro.2019.08.059.
- Brenner H, Kloor M, Pox CP. Colorectal cancer. Lancet. 2014;383(9927):1490–1502. doi:10.1016/S0140-6736(13)61649-9.
- Burnett-Hartman AN, Lee JK, Demb J, et al. An update on the epidemiology, molecular characterization, diagnosis, and screening strategies for early-onset colorectal cancer. Gastroenterology. 2021;160(4):1041–1049. doi:10.1053/j.gastro.2020.12.068.
- Kastenberg D, Bertiger G, Brogadir S. Bowel preparation quality scales for colonoscopy. World J Gastroenterol. 2018;24(26):2833–2843. doi:10.3748/wjg.v24.i26.2833.
- Fearnhead NS, Wilding JL, Bodmer WF. Genetics of colorectal cancer: hereditary aspects and overview of colorectal tumorigenesis. Br Med Bull. 2002;64(1):27–43. doi:10.1093/bmb/64.1.27.
- Sun F, Liang W, Qian J. The identification of CRNDE, H19, UCA1 and HOTAIR as the key lncRNAs involved in oxaliplatin or irinotecan resistance in the chemotherapy of colorectal cancer based on integrative bioinformatics analysis. Mol Med Rep. 2019;20(4):3583–3596. doi:10.3892/mmr.2019.10588.
- Liu S, Cao Q, Yan AG, et al. L: identification of the 3-lncRNA signature as a prognostic biomarker for colorectal cancer. Int J Mol Sci. 2020;21(24):9359. doi:10.3390/ijms21249359.
- Cheng L, Han T, Zhang Z, et al. Identification and validation of six autophagy-related long non-coding RNAs as prognostic signature in colorectal cancer. Int J Med Sci. 2021;18(1):88–98. doi:10.7150/ijms.49449.
- Zhou J, Lin J, Zhang H, et al. LncRNA HAND2-AS1 sponging miR-1275 suppresses colorectal cancer progression by upregulating KLF14. Biochem Biophys Res Commun. 2018;503(3):1848–1853. doi:10.1016/j.bbrc.2018.07.125.
- Jiang Z, Li L, Hou Z, et al. LncRNA HAND2-AS1 inhibits 5-fluorouracil resistance by modulating miR-20a/PDCD4 axis in colorectal cancer. Cell Signal. 2020;66:109483. doi:10.1016/j.cellsig.2019.109483.
- Gaur AB, Holbeck SL, Colburn NH, et al. Downregulation of Pdcd4 by mir-21 facilitates glioblastoma proliferation in vivo. Neuro Oncol. 2011;13(6):580–590. doi:10.1093/neuonc/nor033.
- Wei NA, Liu SS, Leung TH, et al. Loss of programmed cell death 4 (Pdcd4) associates with the progression of ovarian cancer. Mol Cancer. 2009;8(1):70. doi:10.1186/1476-4598-8-70.
- Bian Z, Jin L, Zhang J, et al. LncRNA-UCA1 enhances cell proliferation and 5-fluorouracil resistance in colorectal cancer by inhibiting miR-204-5p. Sci Rep. 2016;6(1):23892. doi:10.1038/srep23892.
- Xue YB, Ding MQ, Xue L, et al. CircAGFG1 sponges miR-203 to promote EMT and metastasis of non-small-cell lung cancer by upregulating ZNF281 expression. Thorac Cancer. 2019;10(8):1692–1701. doi:10.1111/1759-7714.13131.
- Qin CJ, Bu PL, Zhang Q, et al. ZNF281 regulates cell proliferation, migration and invasion in colorectal cancer through wnt/β-catenin signaling. Cell Physiol Biochem. 2019;52(6):1503–1516.
- Zhuang ST, Cai YJ, Liu HP, et al. LncRNA NEAT1/miR-185-5p/IGF2 axis regulates the invasion and migration of Colon cancer. Mol Genet Genomic Med. 2020;8(4):e1125.
- Wang X, Jiang G, Ren W, et al. LncRNA NEAT1 regulates 5-Fu sensitivity, apoptosis and invasion in colorectal cancer through the MiR-150-5p/CPSF4 axis. Onco Targets Ther. 2020;13:6373–6383. doi:10.2147/OTT.S239432.
- Chen B, Dragomir MP, Fabris L, et al. The long noncoding RNA CCAT2 induces chromosomal instability through BOP1-AURKB signaling. Gastroenterology. 2020;159(6):2146–2162.e2133. doi:10.1053/j.gastro.2020.08.018.
- Zhu Y, Hu H, Yuan Z, et al. LncRNA NEAT1 remodels chromatin to promote the 5-Fu resistance by maintaining colorectal cancer stemness. Cell Death Dis. 2020;11(11):962. doi:10.1038/s41419-020-03164-8.
- Xing Y, Zhao Z, Zhu Y, et al. Comprehensive analysis of differential expression profiles of mRNAs and lncRNAs and identification of a 14-lncRNA prognostic signature for patients with colon adenocarcinoma. Oncol Rep. 2018;39(5):2365–2375. doi:10.3892/or.2018.6324.
- Liu Z, Liu L, Weng S, et al. Machine learning-based integration develops an immune-derived lncRNA signature for improving outcomes in colorectal cancer. Nat Commun. 2022;13(1):816. doi:10.1038/s41467-022-28421-6.
- Yang WS, Stockwell BR. Ferroptosis: death by lipid peroxidation. Trends Cell Biol. 2016;26(3):165–176. doi:10.1016/j.tcb.2015.10.014.
- Stockwell BR, Friedmann Angeli JP, Bayir H, et al. Ferroptosis: a regulated cell death nexus linking metabolism, redox biology, and disease. Cell. 2017;171(2):273–285. doi:10.1016/j.cell.2017.09.021.
- Xu T, Ding W, Ji X, et al. Molecular mechanisms of ferroptosis and its role in cancer therapy. J Cell Mol Med. 2019;23(8):4900–4912. doi:10.1111/jcmm.14511.
- Wu Z, Lu Z, Li L, et al. Identification and validation of ferroptosis-related LncRNA signatures as a novel prognostic model for colon cancer. Front Immunol. 2021;12:783362. doi:10.3389/fimmu.2021.783362.
- Cristescu R, Mogg R, Ayers M, et al. Pan-tumor genomic biomarkers for PD-1 checkpoint blockade-based immunotherapy. Science. 2018;362(6411): earr3593. doi:10.1126/science.aar3593.
- Jiang X, Wang J, Deng X, et al. The role of microenvironment in tumor angiogenesis. J Exp Clin Cancer Res. 2020;39(1):204. doi:10.1186/s13046-020-01709-5.
- Luan L, Dai Y, Shen T, et al. Development of a novel hypoxia-immune-related LncRNA risk signature for predicting the prognosis and immunotherapy response of colorectal cancer. Front Immunol. 2022;13:951455. doi:10.3389/fimmu.2022.951455.
- Zhu Z, Wen Y, Xuan C, et al. Identifying the key genes and microRNAs in prostate cancer bone metastasis by bioinformatics analysis. FEBS Open Bio. 2020;10(4):674–688. doi:10.1002/2211-5463.12805.
- Zhao F, Wang J, Chen M, et al. Sites of synchronous distant metastases and prognosis in prostate cancer patients with bone metastases at initial diagnosis: a population-based study of 16,643 patients. Clin Transl Med. 2019;8(1):30.
- Cui PF, Cong XF, Gao F, et al. Prognostic factors for overall survival in prostate cancer patients with different site-specific visceral metastases: a study of 1358 patients. World J Clin Cases. 2020;8(1):54–67. doi:10.12998/wjcc.v8.i1.54.
- Nevedomskaya E, Baumgart SJ. Haendler B: recent advances in prostate cancer treatment and drug discovery. Int J Mol Sci. 2018;19(5):1359. doi:10.3390/ijms19051359.
- Gravis G, Boher JM, Joly F, et al. Androgen deprivation therapy (ADT) plus docetaxel versus ADT alone in metastatic non castrate prostate cancer: impact of metastatic burden and long-term survival analysis of the randomized phase 3 GETUG-AFU15 trial. Eur Urol. 2016;70(2):256–262. doi:10.1016/j.eururo.2015.11.005.
- Damodaran S, Kyriakopoulos CE, Jarrard DF. Newly diagnosed metastatic prostate cancer: has the paradigm changed? Urol Clin North Am. 2017;44(4):611–621. doi:10.1016/j.ucl.2017.07.008.
- Li C, Hu J, Hu X, et al. LncRNA SNHG9 is a prognostic biomarker and correlated with immune infiltrates in prostate cancer. Transl Androl Urol. 2021;10(1):215–226. doi:10.21037/tau-20-1134.
- Chen Y, Chen Z, Mo J, et al. Identification of HCG18 and MCM3AP-AS1 that associate with bone metastasis, poor prognosis and increased abundance of M2 macrophage infiltration in prostate cancer. Technol Cancer Res Treat. 2021;20:1533033821990064. doi:10.1177/1533033821990064.
- Lei G, Zhang Y, Koppula P, et al. The role of ferroptosis in ionizing radiation-induced cell death and tumor suppression. Cell Res. 2020;30(2):146–162. doi:10.1038/s41422-019-0263-3.
- Liu C, Gao Y, Ni J, et al. The ferroptosis-related long non-coding RNAs signature predicts biochemical recurrence and immune cell infiltration in prostate cancer. BMC Cancer. 2022;22(1):788. doi:10.1186/s12885-022-09876-8.
- Fock KM. Review article: the epidemiology and prevention of gastric cancer. Aliment Pharmacol Ther. 2014;40(3):250–260. doi:10.1111/apt.12814.
- Comprehensive molecular characterization of gastric adenocarcinoma. Nature. 2014;513(7517):202–209.
- Joshi SS, Badgwell BD. Current treatment and recent progress in gastric cancer. CA Cancer J Clin. 2021;71(3):264–279. doi:10.3322/caac.21657.
- Smyth EC, Nilsson M, Grabsch HI, et al. Gastric cancer. Lancet. 2020;396(10251):635–648. doi:10.1016/S0140-6736(20)31288-5.
- Tan Z. Recent advances in the surgical treatment of advanced gastric cancer: a review. Med Sci Monit. 2019;25:3537–3541. doi:10.12659/MSM.916475.
- Ghafouri-Fard S, Taheri M. Long non-coding RNA signature in gastric cancer. Exp Mol Pathol. 2020;113:104365. doi:10.1016/j.yexmp.2019.104365.
- Lansdorp-Vogelaar I, Kuipers EJ. Screening for gastric cancer in Western countries. Gut. 2016;65(4):543–544. doi:10.1136/gutjnl-2015-310356.
- Nie K, Deng Z, Zheng Z, et al. Identification of a 14-lncRNA signature and construction of a prognostic nomogram predicting overall survival of gastric cancer. DNA Cell Biol. 2020;39(9):1532–1544. doi:10.1089/dna.2020.5565.
- Mou Y, Wang J, Wu J, et al. Ferroptosis, a new form of cell death: opportunities and challenges in cancer. J Hematol Oncol. 2019;12(1):34. doi:10.1186/s13045-019-0720-y.
- Wei J, Zeng Y, Gao X, et al. A novel ferroptosis-related lncRNA signature for prognosis prediction in gastric cancer. BMC Cancer. 2021;21(1):1221. doi:10.1186/s12885-021-08975-2.
- Duan F, Song C, Wang P, et al. Polygenic risk scores for prediction of gastric cancer based on bioinformatics screening and validation of functional lncRNA SNPs. Clin Transl Gastroenterol. 2021;12(11):e00430. doi:10.14309/ctg.0000000000000430.
- Zhu X, Tian X, Yu C, et al. A long non-coding RNA signature to improve prognosis prediction of gastric cancer. Mol Cancer. 2016;15(1):60. doi:10.1186/s12943-016-0544-0.
- Engreitz JM, Haines JE, Perez EM, et al. Local regulation of gene expression by lncRNA promoters, transcription and splicing. Nature. 2016;539(7629):452–455. doi:10.1038/nature20149.
- Tan YT, Lin JF, Li T, et al. LncRNA-mediated posttranslational modifications and reprogramming of energy metabolism in cancer. Cancer Communications. 2021;41(2):109–120. doi:10.1002/cac2.12108.
- Pefanis E, Wang J, Rothschild G, et al. RNA exosome-regulated long non-coding RNA transcription controls super-enhancer activity. Cell. 2015;161(4):774–789. doi:10.1016/j.cell.2015.04.034.
- Lin W, Huang Z, Xu Y, et al. A three-lncRNA signature predicts clinical outcomes in low-grade glioma patients after radiotherapy. Aging. 2020;12(10):9188–9204. doi:10.18632/aging.103189.
- Yu W, Ma Y, Hou W, et al. Identification of immune-related lncRNA prognostic signature and molecular subtypes for glioblastoma. Front Immunol. 2021;12:706936. doi:10.3389/fimmu.2021.706936.
- Gao L, Lin P, Chen P, et al. A novel risk signature that combines 10 long noncoding RNAs to predict neuroblastoma prognosis. J Cell Physiol. 2020;235(4):3823–3834. doi:10.1002/jcp.29277.
- Xu Z, Chen H, Sun J, et al. Multi-omics analysis identifies a lncRNA-related prognostic signature to predict bladder cancer recurrence. Bioengineered. 2021;12(2):11108–11125. doi:10.1080/21655979.2021.2000122.
- Liu J, Cui J, Zhao S, et al. Ferroptosis-related long noncoding RNAs have excellent predictive ability for multiomic characteristics of bladder cancer. Oxid Med Cell Longev. 2022;2022:1–30. doi:10.1155/2022/9316847.
- Chi H, Peng G, Wang R, et al. Cuprotosis programmed-cell-death-related lncRNA signature predicts prognosis and immune landscape in PAAD patients. Cells. 2022;11(21):3436. doi:10.3390/cells11213436.
- Yuan Q, Ren J, Li L, et al. Development and validation of a novel N6-methyladenosine (m6A)-related multi- long non-coding RNA (lncRNA) prognostic signature in pancreatic adenocarcinoma. Bioengineered. 2021;12(1):2432–2448. doi:10.1080/21655979.2021.1933868.
- Chen E, Yi J, Jiang J, et al. Identification and validation of a fatty acid metabolism-related lncRNA signature as a predictor for prognosis and immunotherapy in patients with liver cancer. BMC Cancer. 2022;22(1):1037. doi:10.1186/s12885-022-10122-4.
- Li X, Zhang Z, Liu M, et al. Establishment of a lncRNA-based prognostic gene signature associated with altered immune responses in HCC. Front Immunol. 2022;13:880288. doi:10.3389/fimmu.2022.880288.
- Tian S, Tang M, Li J, et al. Identification of long non-coding RNA signatures for squamous cell carcinomas and adenocarcinomas. Aging. 2021;13(2):2459–2479. doi:10.18632/aging.202278.
- Qian L, Ni T, Fei B, et al. An immune-related lncRNA pairs signature to identify the prognosis and predict the immune landscape of laryngeal squamous cell carcinoma. BMC Cancer. 2022;22(1):545. doi:10.1186/s12885-022-09524-1.
- Wang X, Lu Y, Liu Z, et al. A 9-LncRNA signature for predicting prognosis and immune response in diffuse large B-Cell lymphoma. Front Immunol. 2022;13:813031. doi:10.3389/fimmu.2022.813031.
- Liu W, Yang HS, Zheng SY, et al. Thymic epithelial tumors: examining the GTF2I mutation and developing a novel prognostic signature with LncRNA pairs to predict tumor recurrence. BMC Genomics. 2022;23(1):656. doi:10.1186/s12864-022-08880-3.
- Zhou M, Zhao H, Wang Z, et al. Identification and validation of potential prognostic lncRNA biomarkers for predicting survival in patients with multiple myeloma. J Exp Clin Cancer Res. 2015;34(1):102. doi:10.1186/s13046-015-0219-5.
- Zhong Q, Lu M, Yuan W, et al. Eight-lncRNA signature of cervical cancer were identified by integrating DNA methylation, copy number variation and transcriptome data. J Transl Med. 2021;19(1):58. doi:10.1186/s12967-021-02705-9.
- Song Y, Qu H. Identification and validation of a seven m6A-related lncRNAs signature predicting prognosis of ovarian cancer. BMC Cancer. 2022;22(1):633. doi:10.1186/s12885-022-09591-4.
- Niu L, Wu Z. Identification and validation of oxeiptosis-associated lncRNAs and prognosis-related signature genes to predict the immune status in uterine corpus endometrial carcinoma. Aging (Albany NY). 2023;15(10):4236–4252. doi:10.18632/aging.204726.
- Zhu J, Deng J, Zhang L, et al. Reconstruction of lncRNA-miRNA-mRNA network based on competitive endogenous RNA reveals functional lncRNAs in skin cutaneous melanoma. BMC Cancer. 2020;20(1):927. doi:10.1186/s12885-020-07302-5.
- Rong J, Wang H, Yao Y, et al. Identification of m7G-associated lncRNA prognostic signature for predicting the immune status in cutaneous melanoma. Aging. 2022;14(12):5233–5249. doi:10.18632/aging.204151.
- Zhang YP, Cheng YB, Li S, et al. An epithelial-mesenchymal transition-related long non-coding RNA signature to predict overall survival and immune microenvironment in kidney renal clear cell carcinoma. Bioengineered. 2021;12(1):555–564. doi:10.1080/21655979.2021.1880718.
- Li S, Ran MY, Qiao H. A cell cycle-related lncRNA signature predicts the progression-free interval in papillary thyroid carcinoma. Front Endocrinol. 2023;14:1110987. doi:10.3389/fendo.2023.1110987.
- Liu B, Liu Z, Feng C, et al. Identification of cuproptosis-related lncRNA prognostic signature for osteosarcoma. Front Endocrinol. 2022;13:987942. doi:10.3389/fendo.2022.987942.
- Li J, Chen Z, Tian L, et al. LncRNA profile study reveals a three-lncRNA signature associated with the survival of patients with oesophageal squamous cell carcinoma. Gut. 2014;63(11):1700–1710. doi:10.1136/gutjnl-2013-305806.