Abstract
Purpose
Few risk-forecasting models of allergic rhinitis (AR) exist that may aid AR pre-exposure prophylaxis (PrEP) in clinical practice. Therefore, this study aimed to develop and validate an effective clinical model for identifying candidates for AR PrEP using a routine medical questionnaire.
Methods
This study was conducted in 10 Chinese provinces with 13 medical centers (n = 877) between 2019 and 2021. Clinical characteristics and exposure history were collected via face-to-face interviews. Well-trained physicians diagnosed patients with AR based on skin prick test results and clinical performance. The least absolute shrinkage and selection operator model was used to identify potential risk factors for AR, and the logistic regression model was used to construct the risk-forecasting model. Predictive power and model reliability were assessed using area under the receiver operating characteristic curve and calibration curves, respectively.
Results
This study diagnosed 625 patients with AR who had positive responses to at least one indoor or outdoor allergen and 460 to at least one outdoor pollen allergen. Two nomograms were established to identify two types of AR with various sensitization patterns. Both models had an area under curve of approximately 0.7 in the development and internal validation datasets. Additionally, our findings found good agreement for the calibration curves of both models.
Conclusion
Early identification of candidates for AR PrEP using routine medical information may improve the deployment of limited resources and effective health management. Our models showed good performance in predicting AR; therefore, they can serve as potential automatic screening tools to identify AR PrEP candidates.
Background
Allergic rhinitis (AR) is a chronic disease characterized by itchiness, runny nose, sneezing, and nasal congestion. AR is associated with type I hypersensitivity, mediated by immunoglobulin E after exposure to allergens [Citation1,Citation2]. AR and asthma-related allergic diseases can cause a series of health, economic, and social burdens as well as decreased quality of life, expensive medical costs, and decreased learning and working efficiency [Citation3,Citation4]. Much evidence suggests that AR has become the most common allergic disease in children and imposes a substantial burden on quality of life [Citation1].
Emerging evidence has confirmed that AR initiation is caused by the interaction of genetic and environmental factors [Citation5]. The main environmental risk factors for AR include inhaled indoor and outdoor allergens [Citation6–8]. Many studies have investigated the risk and protective factors for allergic diseases to provide effective screening methods for establishing preventive measures such as allergen avoidance; however, with low or very low evidence, the effect is not ideal [Citation9,Citation10].
Constructing a clinical predictive model such as a nomogram is an effective disease prevention and progression control method. However, there is no universally accepted AR risk-forecasting model in clinical practice yet. Additionally, there is no effective approach for curing AR; hence, it is important to develop and validate a clinical model to identify candidates for AR pre-exposure prophylaxis (PrEP). Therefore, we aimed to develop and validate an effective clinical predictive model to identify PrEP candidates for AR using a routine medical questionnaire.
Patients and methods
Research design and data sources
This multi-center case-control study was conducted at 13 medical centers in China between October 2019 and June 2021 to develop and validate a clinical model for predicting the risk of AR. All patients in this study were from the Allergy, Pediatrics, and Respiratory Departments in the hospital during routine medical visits. The cases were defined as patients with AR who had a positive response to at least one common allergen in this study. In contrast, the corresponding control group was defined as rhinitis patients without a skin prick test (SPT) response to allergens.
The clinical characteristics and questionnaire information were collected from routine medical follow-up via face-to-face interviews, and SPT (Inmunotek, Inmunotek SL, Spain) was performed after obtaining written informed consent forms [Citation11]. The ethics committee of the First Affiliated Hospital of Guangzhou Medical University (reference number: GYFYY-2018-93) approved the research design and project protocol.
Patients and testing methods
This study included 877 patients with rhinitis. The inclusion criteria were as follows: (1) self-reported history of rhinitis, (2) diagnosis of rhinitis by a well-trained physician, and (3) completion of the required questionnaire and detection of 18 allergens using SPT. The exclusion criteria included patients (1) without information on SPT results; (2) without rhinitis severity score; and (3) with serious skin damage, autoimmune diseases, parasitic infections, or cancer.
Potential predictive variables and outcome definition
This study obtained data on demographics and clinical information, including age, sex, mode of delivery, smoking exposure, ethnic group, family history of allergies, rhinitis control score, use of a mattress, allergic symptoms when in contact with pollen, flower, or grass, house style, address, floor style, and pillow style. Additionally, the visual analog scale of rhinitis symptoms (sneezing, runny nose, nasal congestion, and nasal itching); the effect of rhinitis on sleep, work, or study; and social and physical activities were obtained.
According to SPT results and clinical diagnosis, outcome 1 was defined as patients with rhinitis and a positive response to at least one allergen (Dermatophagoides pteronyssinus, Blattella germanica, Aspergillus fumigatus, Penicillium notatum, Ambrosia elatior, Artemisia vulgaris, Chenopodium album, Betula verrucose, Phleum pratense, Brassica napus, Triticum aestivum, Ulmus campestris, Salix fragilis, Cynodon dactylon, Populus alba, Mediterranean cypress, Platanus hispanica, and Phragmites communis). Furthermore, outcome 2 was determined as patients with rhinitis who were allergic to at least one outdoor pollen allergens (Ambrosia elatior, Artemisia vulgaris, Chenopodium album, Betula verrucose, Phleum pratense, Brassica napus, Triticum aestivum, Ulmus campestris, Salix fragilis, Cynodon dactylon, Populus alba, Mediterranean cypress, Platanus hispanica, and Phragmites communis). Detailed information regarding the experimental procedure of SPT has been described previously [Citation11].
Statistical analysis
Median values combined with the first and third quartiles were used to describe continuous variables. The Mann–Whitney U test or Kruskal–Wallis H test was performed to compare the difference between and among groups. Frequency (proportion) was used to present categorical variables, and the Chi-square test was performed for comparisons.
We divided the enrolled participants into three datasets, namely, development, internal, and external validation, to ensure the reliability and generalization of the models. The development and internal validation datasets from 10 medical healthcare centers were obtained randomly at a ratio of 8:2. Additionally, three medical centers were selected for external validation based on the geographical distribution and sample representativeness in this study. The least absolute shrinkage and selection operator (LASSO) regression model with 10-fold cross-validation was used to select the significant risk factors for AR. Using the logistic regression model, nomograms were set up based on the variable screening results. The receiver operating characteristic curve (ROC) was used to assess the predictive power of the models, and a calibration curve was used to estimate the robustness of the models.
All data cleaning and statistical analyses were performed using SAS 9.4 (Copyright 2002–2012 by SAS Institute Inc., Cary, NC, USA), and all figures were drawn using RStudio (Version 1.2.1335 Copyright 2009–2019 RStudio, Inc.). A P-value of <0.05 was considered statistically significant.
Results
Characteristics of the study population
In this study, the median (first quartile, third quartile) age of the patients was 10 (6–26) years. SPT results suggested that 71.3% of the patients were allergic to at least one allergen and 52.5% to at least one outdoor allergen. We randomly selected 587 (67%) patients as development datasets to set up the predictive model and 147 (17%) as internal validation datasets to verify model stability. Moreover, 143 (16%) patients were grouped as external validation datasets to appraise model generalization.
summarizes that the demographics and clinical features of the development, internal, and external validation datasets were overall balanced and comparable. However, the external validation patients had a lower age, higher frequency of history of allergy and allergic symptoms when in contact with allergens, as well as a lower score for the effect of rhinitis on work or social activities. The results () suggest that minorities have itchy noses, stuffy noses, or sprays after contact with grass, trees, or flowers. Furthermore, the urban living environment is a risk factor for rhinitis patients combined with SPT positivity (allergic to at least one allergen). Additionally, patients with AR have lower rhinitis control and rhinitis symptom severity scores. Patients with SPT positivity had a higher rhinitis score for sleep, work, and social and physical activity than the corresponding patients with SPT positivity (p < 0.05).
Table 1. Baseline demographics and clinical characteristics of patients in the training, internal, and external validation datasets.
Table 2. Demographics and questionnaire among patients in the development datasets who were allergic to at least one allergen.
Optimal clinical features detection
In this study, we constructed Model 1 to predict the risk of AR in patients who were allergic to indoor or outdoor allergens. Moreover, Model 2 was used to predict the risks of AR in those who were allergic to outdoor pollen allergens. As the optimal variables selected for this study, a LASSO regression model with 10-fold cross-validation in the training dataset was used to include sufficient potential predictors and rule out unimportant predictors. The results (Supplementary Figure 1(A)) reveal that Model 1 consisted of sex, ethnicity, allergy symptoms, the use of a mattress, address, the severity of sneezing, nasal itching and runny nose, and impact on work or study. Furthermore, Model 2 (Supplementary Figure 1(B)) included age, ethnicity, allergy symptoms, address, the severity of sneezing and nasal itching, and impact on work or study.
Clinical predictive model development and external evaluation
Depending on the variable selection results, two nomogram-scoring systems () were formulated using logistic regression in the development dataset. The effect on the performance, discrimination, and calibration of the nomogram model was assessed based on the development, internal, and external validation datasets. In nomogram 1, the area under the curve (AUC) based on the ROC analysis () revealed satisfactory and consistent prognostic performance in the development (AUC = 0.69, 95% confidence interval [CI]: 0.64–0.73) and internal validation sets (AUC = 0.71, 95% CI: 0.61–0.81). Moreover, the external validation results demonstrated the predictive stability of nomogram 1 (AUC = 0.70, 95% CI: 0.60–0.80). The nomogram was used to predict participants with risks of AR, and good calibration was observed ().
Figure 1. Nomogram to predict the risk of allergic rhinitis. (A) Outcome 1 was defined as patients with allergic rhinitis (AR) who had a positive response to at least one indoor or outdoor allergen; (B) outcome 2 was defined as patients with AR who had a positive response to at least one outdoor pollen allergen.
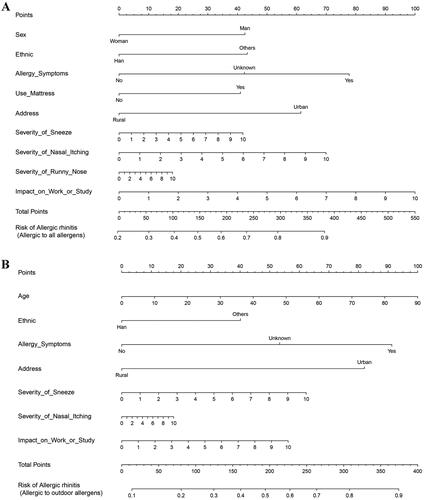
Figure 3. Calibration curves of nomograms a and B. (A1 and B1) training datasets; (A2 and B2) internal validation datasets; and (A3 and B3) external training datasets.
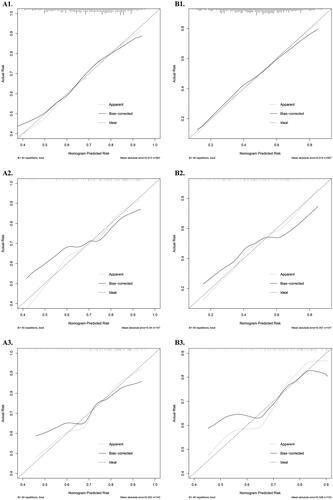
In this study, a total of 18 allergens were tested to identify allergen sensitization, 14 of which were outdoor pollen allergens. Therefore, it was necessary to develop another nomogram to identify the risks of outdoor allergen sensitization among patients with rhinitis. The results show that the AUCs of ROC of nomogram 2 () in both development (AUC = 0.71, 95% CI: 0.67–0.75) and internal validation datasets (AUC = 0.69, 95% CI: 0.61–0.78) had better performance and consistency. However, the AUC in the external validation set was relatively low (AUC = 0.66, 95% CI: 0.56–0.77). The disagreement between the development and external validation datasets may be due to differences in population characteristics, such as age (p < 0.001). Nevertheless, the calibration curve () suggests that nomogram 2 has satisfactory predictive performance.
Discussion
This multi-center, real-world study assessed the clinical characteristics of patients with rhinitis based on subjective and objective tests. To the best of our knowledge, this is the first study to develop and externally validate a nomogram for predicting AR risk based on a large-scale epidemiological survey in China. This study developed two nomograms to identify AR patients among those reported to have rhinitis. The results demonstrated that Models 1 and 2 could distinguish between AR and non-AR patients in the development datasets, with an acceptable AUC of ROC, respectively. In addition, the internal and external validation datasets support our model’s robustness and good calibration.
The AUC of ROC analysis greater than 0.7 may be considered a meaningful separation in clinical practice. For instance, AUCs of 0.66–0.72 were reported in a similar study concerning human immunodeficiency virus (HIV) risk prediction [Citation12]. Additionally, many researchers have developed several models with AUCs between 0.71 and 0.82 in other medical fields [Citation13], and both had good discriminating abilities. In comparison with others, we revealed that our models have good performance and are easy to conduct for clinicians. The major novelty of this study is that we divided patients with AR into two groups to consider various allergens comprehensively. The first group was allergic to at least one common indoor or outdoor allergen in China, whereas the other was allergic to at least one common outdoor pollen allergen. Our findings may be beneficial for precision prevention and health management, as the models can serve as a potential automatic screening tool to identify PrEP candidates for AR.
This study contributes to the secondary prevention of AR using a common medical information questionnaire designed for patients with allergies. Clinical predictive models have been widely used in medical care and clinical assessment. For instance, an automated cardiovascular risk prediction tool has been embedded in electronic medical records to guide clinicians in prescribing aspirin and statins [Citation14]. Under the coronavirus disease 2019 epidemic, Liang et al. used the LASSO model to develop and validate a predictive tool for predicting the occurrence of critical illness among coronavirus disease 2019 inpatients, thereby guiding appropriate treatment plans and optimizing medical resource use [Citation15]. several predictive models for allergic diseases have been developed in clinical practice. For instance, Silver et al. [Citation16] established a predictive risk model by collecting pollen, weather, particulate matter, demographic characteristics, and AR symptoms, achieving personalized prediction of AR. In addition, De Weger et al. developed and verified a hay fever symptom model for the next 5 days based on temperature and grass pollen for patients with hay pollen allergy, and the prediction power of the model was good [Citation17]. PrEP is widely used in the field of HIV infection. Marcus et al. [Citation18] reported that HIV prediction models could be established using information from electronic medical records to identify potential HIV candidates, which is beneficial for preventing HIV. Similarly, our study used some demographic and exposure information of patients enrolled in multi-center research, combined with accurate clinical diagnosis, to develop easy-to-use nomogram models for identifying PrEP candidates for AR.
The nomogram is believed to be an effective tool in various healthcare systems owing to its convenience and practicality [Citation19]. The predictive factors in our nomogram are common clinical information that can be obtained from routine medical follow-ups. For example, in Model 1, a female of Han nationality living in the countryside, had no allergy symptoms, did not use a mattress, did not have symptoms of severe sneezing, itchy nose, and runny nose, had no impact on life, had an overall risk score of 0, and a risk prediction of 0.2 for AR. In contrast, a male of other ethnic groups living in an urban area with allergy symptoms, mattress use, moderately severe sneezing, itchy nose, and runny nose had a moderate impact on life and school, with an overall risk score of 384 and a risk prediction for AR of 0.9. This nomogram is relatively easy to understand and can be stored for a short period by accessing an online risk-scoring calculator during routine medical follow-up. It should be noted that the severity of nasal itching, sneezing, and runny nose were positively correlated with the risk of AR, reflecting that the greater the severity of rhinitis symptoms, the greater the tendency to suffer from AR. Consistent with our findings, many studies suggest that patients with sneezing, runny nose, and moderate or severe nasal inflammation tend to have AR. Additionally, patients with AR showed more “persistent” and “moderate-to-severe” symptoms [Citation20,Citation21]. In Model 2, older age was associated with a higher risk of AR. Previous studies have shown that rhinitis is more common in the elderly than in the young [Citation22], but more reports show that young people are more prone to developing AR than the elderly, which may be related to the reduced immunoglobulin E level in the elderly [Citation23,Citation24]. In our model, urban residents were more likely to have AR, which has been highlighted in our previous study [Citation25]. Using simple questionnaires and clinical information, it is easier and more effective to identify candidates for AR using these two models. The internal validation of models 1 and 2 was lower than the external validation. The possible reasons might be the differences in demographic characteristics (such as age), allergy history, frequency of allergic symptoms when exposed to allergens, and scores in the impact of rhinitis on work or social activities between the external validation dataset and the development and internal validation datasets.
In our study, we further developed the LASSO regression model to identify patients with AR who were allergic to outdoor pollen since 14 of the 18 allergens were detected as outdoor pollen allergens. Our results showed that Model 2 had better risk prediction performance for patients with AR who were allergic to outdoor pollen allergens. Outdoor pollen allergens are the main cause of AR because an increasing number of people are affected by AR associated with pollen allergens. Additionally, pollen exposure affects pollen-allergic people. Studies have shown that hay fever allergies often dismiss the deterioration in quality of life caused by pollen exposure. However, there is still lack of studies identifying the risk factors that cause symptoms and continuous aggravation of allergies [Citation26]. Therefore, Model 2 can identify high-risk patients with outdoor allergen-positive AR and effectively prevent the aggravation of allergic symptoms.
This study has several limitations. Most importantly, our models did not include the seasonal variability of AR symptoms. However, our previous study confirmed no significant seasonal variability (spring, summer, autumn, and winter) among various sensitization patterns [Citation11]. Previous studies have shown that AR can be divided into persistent and intermittent rhinitis [Citation27]. Persistent AR is usually caused by indoor allergens, such as house dust mites or cat and dog hair. In contrast, intermittent AR is commonly caused by outdoor allergens, of which grass pollen is the main allergen. Therefore, this study assessed two sensitization patterns among patients with AR, partly reducing bias. In addition, the patients in this study were mainly children and young adults, limiting the application of our models. However, our study has several advantages. First, this study was based on a large-scale multi-center population randomly divided into development and external datasets. Therefore, our models are robust in clinical application because of their strong generalization abilities. Subsequently, we simultaneously developed two nomograms, and both had a good performance, which would help identify high-risk AR patients with a specific type of allergy pattern.
In conclusion, a predictive model based on a medical questionnaire survey of patients with rhinitis can be used to identify high-risk AR patients. We successfully developed and validated two clinical models that combined patients’ demographic characteristics and clinical information to predict the risk of AR. These models showed good performance and were easy to perform. Our models can be used to identify patients with AR early in routine medical follow-up, which may improve the deployment of limited medical resources.
Author contributions
Wenting Luo, Xiangqing Hou, Baoqing Sun and Chuangli Hao designed the study. Wenting Luo, Xiangqing Hou, Yun Sun, Hong Zhang, Huali Ren, Xiangping Ma, Guoping Li, Yongmei Yu, Jianxin Sun, Huajie Wu, Jing Wu, Ruifen Zhong, Siqin Wang, Zhenan Li, Yan Zhao, Liting Wu, Xianhui Zheng, Miaoyuan Xu, Qingyuan Ye completed the data management and data cleaning as well as interpretation. Wenting Luo and Xiangqing Hou performed the statistical analysis. Wenting Luo and Xiangqing Hou drafted the manuscript. Wenting Luo, Xiangqing Hou, Baoqing Sun and Chuangli Hao edited the manuscript. All authors contributed to critically revision of the manuscript, and approved the final version.
Supplemental Material
Download MS Word (143 KB)Acknowledgments
The authors thank everyone involved in the sample collection, detection, and data management. The authors thank the Biobank for Respiratory Disease at the National Clinical Research Center for Respiratory Disease (BRD-NCRCRD, Guangzhou, Southern China).
Disclosure statement
No potential conflict of interest was reported by the author(s).
Data availability statement
The data that support the findings of this study are available from the corresponding author upon reasonable request.
Additional information
Funding
References
- Selnes A, Nystad W, Bolle R, et al. Diverging prevalence trends of atopic disorders in norwegian children. Results from three cross-sectional studies. Allergy. 2005;60(7):1–11. doi: 10.1111/j.1398-9995.2005.00797.x.
- Hellings PW, Fokkens WJ. Allergic rhinitis and its impact on otorhinolaryngology. Allergy. 2006;61(6):656–664. doi: 10.1111/j.1398-9995.2006.01109.x.
- Bousquet J, Van Cauwenberge P, Khaltaev N, World Health Organization. Allergic rhinitis and its impact on asthma. J Allergy Clin Immunol. 2001;108(5 Suppl):S147–S334. doi: 10.1067/mai.2001.118891.
- Zhang F, Nie C, Wang L, et al. The disease burden of patients with allergic rhinitis from a hospital surveillance in beijing. Sustainability. 2017;9(3):427. doi: 10.3390/su9030427.
- Liang XH, Cheung W, Heng CK, et al. Risk factors of allergic rhinitis: genetic or environmental. Ther Clin Risk Manag. 2005;1(3):243–246.
- Blanc PD, Eisner MD, Katz PP, et al. Impact of the home indoor environment on adult asthma and rhinitis. J Occup Environ Med. 2005;47(4):362–372. doi: 10.1097/01.jom.0000158708.32491.9d.
- Luo W, Hu H, Tang W, et al. Allergen sensitization pattern of allergic adults and children in Southern China: a survey based on real life data. Allergy Asthma Clin Immunol. 2019;15(1):42. doi: 10.1186/s13223-019-0357-y.
- Luo W, Wang D, Zhang T, et al. Prevalence patterns of allergen sensitization by region, gender, age, and season among patients with allergic symptoms in mainland China: a four-year multicenter study. Allergy. 2021;76(2):589–593. doi: 10.1111/all.14597.
- Brozek JL, Bousquet J, Baena-Cagnani CE, et al. Allergic rhinitis and its impact on asthma (ARIA) guidelines: 2010 revision. J Allergy Clin Immunol. 2010;126(3):466–476. doi: 10.1016/j.jaci.2010.06.047.
- von Berg A. Dietary interventions for primary allergy prevention–what is the evidence. World Rev Nutr Diet. 2013;108:71–78. doi: 10.1159/000351487.
- Hou X, Luo W, Wu L, et al. Associations of four sensitization patterns revealed by latent class analysis with clinical symptoms: a multi-center study of China. EClinicalMedicine. 2022;46:101349. doi: 10.1016/j.eclinm.2022.101349.
- Owens DK, Davidson KW, Krist AH, et al. Preexposure prophylaxis for the prevention of HIV infection: US preventive services task force recommendation statement. JAMA. 2019;321(22):2203–2213. doi: 10.1001/jama.2019.6390.
- Goff DC, Lloyd-Jones DM, Bennett G, et al. 2013 ACC/AHA guideline on the assessment of cardiovascular risk: a report of the American college of cardiology/American heart association task force on practice guidelines. J Am Coll Cardiol. 2014;63(25 Pt B):2935–2959. doi: 10.1016/j.jacc.2013.11.005.
- Sekaran NK, Sussman JB, Xu A, et al. Providing clinicians with a patient’s 10-year cardiovascular risk improves their statin prescribing: a true experiment using clinical vignettes. BMC Cardiovasc Disord. 2013;13(1):90. doi: 10.1186/1471-2261-13-90.
- Liang W, Liang H, Ou L, et al. Development and validation of a clinical risk score to predict the occurrence of critical illness in hospitalized patients with COVID-19. JAMA Intern Med. 2020;180(8):1081–1089. doi: 10.1001/jamainternmed.2020.2033.
- Silver JD, Spriggs K, Haberle SG, et al. Using crowd-sourced allergic rhinitis symptom data to improve grass pollen forecasts and predict individual symptoms. Sci Total Environ. 2020;720:137351. doi: 10.1016/j.scitotenv.2020.137351.
- de Weger LA, Beerthuizen T, Hiemstra PS, et al. Development and validation of a 5-day-ahead hay fever forecast for patients with grass-pollen-induced allergic rhinitis. Int J Biometeorol. 2014;58(6):1047–1055. doi: 10.1007/s00484-013-0692-5.
- Marcus JL, Hurley LB, Krakower DS, et al. Use of electronic health record data and machine learning to identify candidates for HIV pre-exposure prophylaxis: a modelling study. Lancet HIV. 2019;6(10):E688–E695. doi: 10.1016/S2352-3018(19)30137-7.
- Hou X, Wang D, Zuo J, et al. Development and validation of a prognostic nomogram for HIV/AIDS patients who underwent antiretroviral therapy: data from a China population-based cohort. EBioMedicine. 2019;48:414–424. doi: 10.1016/j.ebiom.2019.09.031.
- Topal E, Bakirtas A, Yılmaz O, et al. Predictive factors to differentiate between allergic and nonallergic rhinitis in children. Int Forum Allergy Rhinol. 2014;4(6):447–452. doi: 10.1002/alr.21312.
- Vichyanond P, Suratannon C, Lertbunnaphong P, et al. Clinical characteristics of children with non-allergic rhinitis vs with allergic rhinitis. Asian Pac J Allergy Immunol. 2010;28(4):270–274.
- Nyenhuis SM, Mathur SK. Rhinitis in older adults. Curr Allergy Asthma Rep. 2013;13(2):171–177. doi: 10.1007/s11882-013-0342-3.
- Cazzoletti L, Ferrari M, Olivieri M, et al. The gender, age and risk factor distribution differs in self-reported allergic and non-allergic rhinitis: a cross-sectional population-based study. Allergy Asthma Clin Immunol. 2015;11(1):36. doi: 10.1186/s13223-015-0101-1.
- Wüthrich B, Schmid-Grendelmeier P, Schindler C, et al. Prevalence of atopy and respiratory allergic diseases in the elderly SAPALDIA population. Int Arch Allergy Immunol. 2013;162(2):143–148. doi: 10.1159/000351416.
- Li CW, Chen DH, Zhong JT, et al. Epidemiological characterization and risk factors of allergic rhinitis in the general population in guangzhou city in China. PLoS One. 2014;9(12):e114950. doi: 10.1371/journal.pone.0114950.
- Šaulienė I, Šukienė L, Kainov D, et al. The impact of pollen load on quality of life: a questionnaire-based study in Lithuania. Aerobiologia. 2016;32(2):157–170. doi: 10.1007/s10453-015-9387-1.
- Bousquet J, Schünemann HJ, Samolinski B, et al. Allergic rhinitis and its impact on asthma (ARIA): achievements in 10 years and future needs. J Allergy Clin Immunol. 2012;130(5):1049–1062. doi: 10.1016/j.jaci.2012.07.053.