Abstract
Purpose
The aim of this study is to determine the effectiveness and reliability of adding traditional Chinese medicine (TCM) in the clinical intervention and explore mechanisms of action for chronic atrophic gastritis (CAG) through meta- and network pharmacology analysis (NPAs).
Methods
A predefined search strategy was used to retrieve literature from PubMed, Embase database, Cochrane Library, China National Knowledge Infrastructure (CNKI), Chinese BioMedical Literature Database (CBM), Wan Fang Data and China Science and Technology Journal Database (VIP). After applying inclusion and exclusion criteria, a total of 12 randomized controlled trials (RCTs) were included for meta-analysis to provide clinical evidence of the intervention effects. A network meta-analysis using Bayesian networks was conducted to observe the relative effects of different intervention measures and possible ranking of effects. The composition of the TCM formulation in the experimental group was analysed, and association rule mining was performed to identify hub herbal medicines. Target genes for CAG were searched in GeneCards, Online Mendelian Inheritance in Man, PharmGKB, Therapeutic Target Database and DrugBank. A regulatory network was constructed to connect the target genes with active ingredients of the hub herbal medicines. Enrichment analyses were performed using the Gene Ontology (GO) and the Kyoto Encyclopedia of Genes and Genomes (KEGG) to examine the central targets from a comprehensive viewpoint. Protein–protein interaction networks (PPINs) were constructed to identify hub genes and conduct molecular docking with differentially expressed genes (DEGs) and corresponding active molecules.
Results
A total of 1140 participants from 12 RCTs were included in the statistical analysis, confirming that the experimental group receiving the addition of TCM intervention had better clinical efficacy. Seven hub TCMs (Paeonia lactiflora, Atractylodes macrocephala, Pinellia ternata, Citrus reticulata, Codonopsis pilosula, Salvia miltiorrhiza and Coptis chinensis) were identified through association rule analysis of all included TCMs. Thirteen hub genes (CDKN1A, CASP3, STAT1, TP53, JUN, MAPK1, STAT3, MAPK3, MYC, HIF1A, FOS, MAPK14 and AKT1) were obtained from 90 gene PPINs. Differential gene expression analysis between the disease and normal gastric tissue identified MAPK1 and MAPK3 as the significant genes. Molecular docking analysis revealed that naringenin, luteolin and quercetin were the main active compounds with good binding activities to the two hub targets. GO analysis demonstrated the function of the targets in protein binding, while KEGG analysis indicated their involvement in important pathways related to cancer.
Conclusions
The results of a meta-analysis of 12 RCTs indicate that TCM intervention can improve the clinical treatment efficacy of CAG. NPAs identified seven hub TCM and 13 target genes associated with their actions, while bioinformatics analysis identified two DEGs between normal and CAG gastric tissues. Finally, molecular docking was employed to reveal the mechanism of action of the active molecules in TCM on the DEGs. These findings not only reveal the mechanisms of action of the active components of the TCMs, but also provide support for the development of new drugs, ultimately blocking the progression from chronic gastritis to gastric cancer.
1. Introduction
Chronic atrophic gastritis (CAG) refers to the reduction of gastric glands, including non-biochemical atrophy and biochemical atrophy [Citation1]. CAG is often recognized as a precancerous lesion of gastric cancer (GC), which ranks the fifth most common cancer worldwide with the third highest cancer-related mortality rate [Citation2]. The prevalence of CAG among research populations from 2010 to 2020 is approximately 25% [Citation3]. In CAG patients, the annual probability of developing GC is 0.1%, and the incidence rates during 1, 5 and 10 years of follow-up are 0.3%, 0.6% and 0.8%, respectively [Citation2]. CAG represents a significant progression from gastritis to GC [Citation4], and effective interventions are crucial in reducing the occurrence of GC. In recent years, Chinese patent medicine and traditional Chinese medicine (TCM) have been proven to have a high clinical efficacy in treating CAG [Citation5–8]. Therefore, obtaining evidence on the target effects of Chinese medicine through evidence-based medicine can provide novel ideas and approaches for intervening in CAG.
Meta-analysis has emerged as a pivotal statistical method in the field of medicine and health sciences [Citation9], serving as the best available evidence to inform clinical practice [Citation10]. This approach overcomes limitations posed by small sample sizes or rare outcomes by integrating the findings of numerous individual studies to produce a single, optimal estimate [Citation11]. While meta-analysis provides an objective quantitative synthesis based on existing research, it cannot address the flaws or deficiencies within the included studies or data, necessitating the consideration of heterogeneity and potential biases in a comprehensive manner [Citation12]. Network meta-analysis (NMA) studies, on the other hand, offer advantages over traditional meta-analysis in comparing and evaluating various interventions or treatment approaches, enabling direct evidence comparisons and indirect evidence predictions [Citation13]. By combining these two techniques, it becomes possible to analyse and derive answers to specific questions based on reported research data.
Network pharmacology (NP) is an emerging field in systematic drug research that primarily focuses on analysing and establishing interactions between active drug molecules and multiple targets [Citation14]. Network-based approaches offer the potential to enhance researchers understanding of the multi-layered information regarding drug actions, while also providing support for drug design and mechanism elucidation through the construction of cellular or characterization networks and chemical networks [Citation15]. NP has become the next paradigm in drug discovery [Citation16]. Moreover, NP is anticipated to unveil and visualize the underlying interaction networks of TCM in relation to multifactorial diseases [Citation17], encompassing investigations into the pharmacological mechanisms of herbal formulas [Citation18], compound synergistic effects [Citation19], as well as advancements in new drug discovery and development [Citation20].
2. Materials and methods
2.1. Database search strategy
Researchers conducted literature searches using a combination of medical subject headings and free keywords in seven databases, without language restrictions, up until May 2023. They also searched the Chinese Clinical Trial Registry and the US ClinicalTrials.gov for relevant registered experiments. The main subject headings used in the search were ‘Gastritis, Atrophic’, ‘randomized controlled trial’, ‘Drugs, Chinese Herbal’ and ‘Traditional Chinese Medicine’. The primary databases searched included PubMed [Citation21], Embase database [Citation22], Cochrane Library [Citation23], China National Knowledge Infrastructure (CNKI) [Citation24], Chinese Biomedical Literature Database (CBM) [Citation25], Wanfang Data [Citation26] and China Science and Technology Journal Database (VIP) [Citation27]. All search results were downloaded and imported into EndNote X9.1 for literature management.
2.2. Inclusion and exclusion criteria
Our literature exclusion criteria were established as follows: (1) studies not utilizing a randomized controlled trial (RCT) design, (2) studies not related to CAG, (3) animal experiments, (4) literature reviews and (5) studies without access to the original text. The included literature needed to meet the following criteria: (1) the experimental group received TCM in addition to omeprazole, amoxicillin and clarithromycin, while the control group only received Western medicine (omeprazole, amoxicillin and clarithromycin), (2) the treatment duration was no less than seven days, (3) all studies were original and had not been previously published, (4) all participants were aged 18 and above (adults are more suitable for clinical analyses and therapeutic observations due to their relatively stable body functions and physiological states) and (5) a minimum Jadad score of three was obtained. The first round of screening was performed by two researchers based on the titles and abstracts of the literature, while the second round of screening required a full-text review. This study included the intersection of literature selected by the two clinical researchers, and in cases of substantial controversy, the judgment was deferred to a third clinical physician.
2.3. Data extraction and quality assessment
Two researchers have been assigned to independently extract data for reporting, including the following information: primary author name, publication date, sample size (control/experimental group), gender (male/female), age (mean ± standard deviation), disease duration (mean ± standard deviation), intervention measures, treatment duration, formulation names, Chinese herbal prescriptions, outcome measures, efficacy indicators, adverse reactions and Jadad scores. Consistency in data collection was ensured, and any discrepancies have been resolved through verification of the original text or third-party adjudication. We utilized the Jadad scale [Citation28] and the Cochrane Collaboration tool [Citation29] to assess the risk of bias in the included literature. The Jadad score primarily evaluates random sequence production, allocation concealment, blinding method and withdrawals. The Cochrane Collaboration tool assesses randomization methods, allocation concealment, blinding, completeness of outcome data, selective reporting of study results, and other sources of bias.
2.4. Data synthesis and analysis
Statistical analysis has been conducted using Review Manager 5.3 and Stata 14.0 software. A p value less than .05 indicated statistical significance for differences. For dichotomous variables, the evaluation has been done using relative risk (RR) ratios and 95% confidence intervals (CIs). For continuous variables, standardized mean differences (SMDs) or mean differences (MDs) were used [Citation30], along with their respective 95% CIs. The consistency of the literature has been assessed using the χ2 test and the inconsistency index statistic (I2) [Citation31]. If significant heterogeneity is present (I2 > 50% or p < .05), a random-effects model was used for pooling results; otherwise, a fixed-effects model has been employed. Sensitivity analysis was conducted to further evaluate the sources of heterogeneity and the stability of results. Funnel plots were used to assess potential publication bias.
2.5. Network construction and analysis
Network analysis of included studies was conducted using Stata 14.0 software and GeMTC software to predict the effects of different interventions. Network meta-analysis, also known as mixed treatment comparison (MTC), is a technique that allows for the simultaneous inclusion of two or more drugs in a meta-analysis. By utilizing a comprehensive Bayesian evidence network, all indirect comparisons are considered, resulting in a single, integrated estimate of treatment effects for all included interventions. In the parameter settings of the model, the number of chains has been set to 4, initial values scaling to 2.5, tuning iterations to 20,000, simulation iterations to 50,000, and thinning interval to 10. Through this analysis, we were able to observe the relative effects of the experimental and control groups, as well as the possible rankings of the intervention effects.
2.6. Hub Chinese medicine mining and targeting
The Chinese herbal medicines included in the literature were standardized according to ‘Chinese materia medica’ [Citation32] and ‘Pharmacopoeia of the People’s republic’ [Citation33]. Then, IBM SPSS Modeler 18.0 software (Armonk, NY) was used to conduct association rule analysis to generate a network of herbal medicine correlations. The support, confidence and lift measures have been calculated for each frequent itemset, and the hub herbal medicines within all prescriptions were identified. Using the search terms ‘Baishao’ (Paeonia lactiflora), ‘Baizhu’ (Atractylodes macrocephala), ‘Banxia’ (Pinellia ternata), ‘Chenpi’ (Citrus reticulata), ‘Dangshen’ (Codonopsis pilosula), ‘Danshen’ (Salvia miltiorrhiza) and ‘Huanglian’ (Coptis chinensis), the Traditional Chinese Medicine Systems Pharmacology Database and Analysis Platform [Citation34] (TCMSP, http://tcmspw.com/tcmsp.php) was utilized to select active ingredients with an oral bioavailability (OB) ≥30% and drug likeness (DL) ≥0.18. The UniProt database [Citation35] (https://www.uniprot.org/) has been used to annotate the gene targets of the drugs. The disease-associated genes were retrieved using ‘Gastritis, Atrophic’ as the search term from multiple databases, including GeneCards [Citation36] (http://www.genecards.org/), Online Mendelian Inheritance in Man [Citation37] (OMIM, https://omin.org), PharmGKB [Citation38] (http://www.pharmgkb.org), Therapeutic Target Database [Citation39] (TTD, http://db.idrblab.net/ttd/) and DrugBank [Citation40] (http://www.drugbank.ca/). The disease genes from these databases were merged, and the intersection of disease genes and drug target genes was identified. The Cytoscape software (v3.9.1) [Citation41] was used to visualize the regulatory network of compound herbal formulations.
2.7. Network pharmacology analysis of the based on hub Chinese medicine
Got the intersection genes from Section 2.6 and input them into the DAVID database [Citation42] (https://david.ncifcrf.gov/home.jsp) to obtain results using Gene Ontology (GO) analysis [Citation43] and the Kyoto Encyclopedia of Genes and Genomes (KEGG) analysis [Citation44]. Utilize the ‘ggplot2’ package [Citation45] in the R language (R-4.1.3) to perform count value filtering and visualize the graphs artistically. The protein–protein interaction networks (PPINs) result from the String database [Citation46] (https://cn.string-db.org/). The PPINs results imported into Cytoscape and analyse the core of the network employing the CytoNCA plugin [Citation47]. Retrieve the protein IDs of hub targets from the UniProt database and search for the 3D structure of protein receptors based on the IDs in the PDB database [Citation48] (https://www.rcsb.org/). Access the PubChem database [Citation49] (https://pubchem.ncbi.nlm.nih.gov/) to obtain the 2D chemical structures of small molecule ligands, then transform them into 3D structures using the ChemBio3D Ultra (14.0) software, followed by performing structure optimization calculations. Introduce hydrogen bonds to the protein receptors and identify the ligand binding sites with the Autodock Tools software [Citation50]. Lastly, carry out molecular docking using the Autodock Vina software to determine the site with the highest binding energy, and visualize the outcomes utilizing the PyMol software [Citation51].
3. Results
3.1. Description of studies
According to the predefined retrieval strategy, we searched a total of 16,777 studies from four Chinese databases (CNKI, CBM, Wang fang data and VIP) and three English databases (PubMed, EMBASE and Cochrane Library) since the establishment of our database. Combining all the literature retrieved from all the databases, 7014 studies were left after weight reduction of the literature. Following the initial screening, 214 studies were included. After careful examination of the full texts by two researchers, only 15 studies met our criteria. However, upon further comparison, we identified one study with duplicate publications and two studies with unclear baselines, resulting in the inclusion of 12 RCTs for meta-analysis [Citation52–62]. The flowchart () of this study’s database search and literature screening process is depicted.
Among the 12 RCT studies published between 2013 and 2022, a total of 1140 participants, including 631 males and 509 females, were included. The control group in these studies received interventions of omeprazole, amoxicillin and clarithromycin, while the experimental group received the addition of a TCM compound based on the control group. The intervention period ranged from a minimum of seven days to a maximum of 90 days. Clinical efficacy was the primary outcome measure for all experiments, categorized as effective, significant or ineffective. The main characteristics of all included studies in this meta-analysis are summarized in .
Table 1. Principal characteristics of all included studies in meta-analysis.
3.2. Risk of bias assessment
The risk of bias assessment using the Cochrane Collaboration’s risk of bias tool revealed that none of the included studies provided clear information regarding allocation concealment. The reason for the absence of blinding in the trials may be attributed to the significant cost differences in treatments between different groups. The quality assessment results () indicate that all studies were at high risk of bias due to lack of allocation concealment. As for the Jadad scores (), three studies [Citation53,Citation61,Citation62] employed random number tables for randomization, two studies [Citation55,Citation56] randomized participants based on admission sequence, and one study [Citation59] used simple randomization. The remaining studies did not mention the method of randomization. Allocation concealment and follow-up were not described. The included studies in the meta-analysis had Jadad scores ranging from 3 to 4. Overall, the literature quality was relatively balanced, and the included studies were of moderate quality.
Figure 2. Evaluation of the quality of the included research literature. (A) Risk of bias summary. (B) Risk of bias graph.
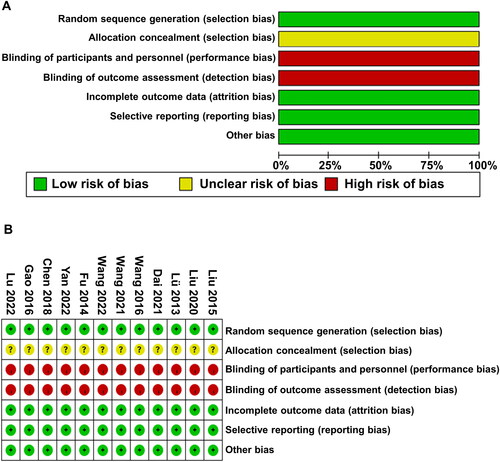
Table 2. Evaluation of methodologic quality of the included studies based on the Cochrane handbook.
3.3. Clinical outcomes
3.3.1. Comparison of all studies
The 12 included studies reported three levels of clinical efficacy (effective, significant, ineffective). The results of the heterogeneity test (I2 = 0% < 50%, and Q test p = .64 > .1) indicated that the heterogeneity among the selected literature was not statistically significant. Therefore, a fixed-effect model was chosen for the meta-analysis. The pooled RR value of the 12 studies was 4.87, with a 95% CI of (3.24, 7.30), indicating a statistically significant difference between the experimental and control groups. The overall effect (Z = 7.64, p = .00001 < .05) demonstrated that the clinical efficacy of combined Western medicine and TCM interventions was superior to the use of Western medicine alone. Further details can be found in the forest plot (). Sensitivity analysis () was performed to assess the stability of the clinical efficacy. Additionally, a radial plot () and L’Abbé plot () were used for supplementary illustrations. The results showed that none of the studies significantly influenced the meta-analysis results, indicating good stability of the research findings. A funnel plot () was generated to observe publication bias. Begg’s test yielded p = .244 > .05, and Egger’s test yielded p = .282 > .05, suggesting that the funnel plot was symmetric, and no publication bias was present in the studies.
Figure 3. Meta-analysis of clinical efficacy of all included studies. (A) Meta-analysis Forest plot of clinical efficacy. (B) Sensitivity analysis of studies included in the literature. (C) Label diagram for heterogeneity test. (D) Star chart for heterogeneity test. (E) Funnel plot of total effectiveness rate.

3.3.2. Comparison of subgroup studies
In our investigation of the characteristic statistics, we have identified that the clinical efficacy of the six studies can be further categorized into four levels: cure, significant improvement, effectiveness and ineffectiveness. The results of the heterogeneity test (I2 = 0% < 50%, and Q test p = .866 > .1) indicate that there is no heterogeneity among the subgroups of studies categorized into these four levels. The fixed-effect meta-analysis of the six articles yielded a RR value of 2.2, with a 95% CI of (1.65, 2.93) (), revealing a statistically significant overall effect (Z = 5.41, p = .000 < .05). The sensitivity analysis results () demonstrate that Wang [Citation60] has a significant impact. To examine publication bias, a funnel plot was generated (). Begg’s test yielded a p value of .024 < .05, and Egger’s test yielded p = .028 < .05, indicating clear asymmetry in the funnel plot. After removing the influential study, publication bias was assessed again. The funnel plot () exhibited a considerably symmetric shape. Begg’s test yielded p = .086 > .05, and Egger’s test yielded p = .1 > .05. These results suggest that there is no publication bias among the four subgroup analyses of the remaining five studies. The substantial influence of Wang [Citation60] may be attributed to reporting bias or an exaggeration of the experimental group’s clinical cure level effect.
Figure 4. Meta-analysis of clinical efficacy of subgroup studies. (A) Meta-analysis Forest plot of clinical efficacy. (B) Sensitivity analysis of studies included in the subgroup literature. (C) Funnel plot of total effectiveness rate. (D) Funnel plot of total effectiveness rate (exclusion of publication bias literature).

3.4. Network meta-analysis of drugs
We constructed a Bayesian network comparing the intervention measures of the control group and the 12 experimental groups () and compute the indirect comparisons of all intervention measures. During the computation, it was found that the intervention measures in the Liu [Citation57] study had a significant effect on all patients, resulting in data bias in the prediction results. Therefore, the decision was made to exclude this reference. The possible relative effects of the experimental and control groups in the literature study were calculated (), including effect size and 95% CI. The effects of different intervention drugs in the different groups were ranked (), and a histogram was plotted (). The results indicate that the experimental groups have higher cumulative probability rankings compared to the control group, with the experimental group in the Wang study [Citation61] having the highest ranking, this may be due to the fact that the study’s follow-up period was longer than 2 months and the addition of the TCM symptom score to the observational indicators.
Figure 5. A network meta-analysis on the clinical efficacy of the experimental groups included in the literature. (A) Network plots for the dug efficacy considered in the review. (B) “Drug-Rank probability” histogram of effectiveness treatment for chronic atrophic gastritis.

Table 3. Standardized mean differences (SMDs; 95% CI) of direct and overall network estimates of efficacy.
Table 4. Possible ranking of the effects of drug use in literature studies.
3.5. Hub Chinese medicine mining
The composition of TCM formulations () in the 12 studies was collected and standardized (), resulting in a total of 56 different medicinal herbs. Among them, the six most frequently used medicinal herbs were Codonopsis pilosula (eight times), Paeonia lactiflora (six times), PORIA (six times), Coptis chinensis (six times), Pinellia ternata (six times), Citrus reticulata (six times) and Salvia miltiorrhiza (five times). Inputting all the medicinal herbs into the association analysis network (), the significant associations were identified based on support, confidence and lift. The association analysis () results were ranked in descending order of support count (support count >3), with Codonopsis pilosula -> Atractylodes macrocephala having six association support counts. By conducting statistical analysis on the antecedents and consequents involved in , a total of seven hub medicinal herbs were identified.
Table 5. The ingredients of each formula.
Table 6. Standardization of Chinese medicine.
Table 7. Chinese medicine association rule analysis.
3.6. Hub Chinese medicine genes target analysis
In the TCMSP database, ‘Baishao’ (Paeonia lactiflora) was found to have 13 active ingredients and 960 drug targets, ‘Baizhu’ (Atractylodes macrocephala) has seven active ingredients and 774 drug targets, ‘Banxia’ (Pinellia ternata) has 13 active ingredients and 1287 drug targets, ‘Chenpi’ (Citrus reticulata) has five active ingredients and 479 drug targets, ‘Dangshen’ (Codonopsis pilosula) has 21 active ingredients and 911 drug targets and ‘Danshen’ (Salvia miltiorrhiza) has 65 active ingredients and 2536 drug targets. Additionally, ‘Huanglian’ (Coptis chinensis) has 14 active ingredients and 597 drug targets. By searching in five online databases, 905 disease-related genes were identified. After organizing the drug target genes for the hub medicinal herbs, a total of 248 genes were obtained. Among these, 90 genes were found to be common to both the hub medicinal herbs and the disease-related genes. The medicinal herb compound regulation network () was constructed in Cytoscape software based on the properties of nodes and the connections between them.
3.7. Results of GO and KEGG analysis
Utilizing the DAVID database, we conducted GO and KEGG enrichment analyses for 90 drug targets and disease-related genes. The results were visualized using R programming language. Regarding GO enrichment analysis, only the top 10 enriched results were displayed for biological process (BP), cellular component (CC) and molecular function (MF) (). In the MF category, genes were primarily enriched in the functionality of protein binding. Within the CC category, the functionalities of cytosol, nucleus and cytoplasm exhibited the highest enrichment. As for BP, the most enriched genes were involved in the positive regulation of transcription from RNA polymerase II promoter, negative regulation of apoptotic process, and positive regulation of gene expression. The top 30 enriched pathways from KEGG analysis were visualized (). Among the positively regulated pathways, pathways in cancer and lipid and atherosclerosis showed significant enrichment, while the pathways of neurodegeneration − multiple diseases pathway had the highest number of enriched genes among negatively regulated pathways.
3.8. PPINs construction and hub node analysis
By importing 90 drug-disease targets and genes into the STRING database with a parameter set at a highest confidence level of 0.9 and hiding disconnected nodes in the network, we eliminated seven nodes, resulting in PPINs of 83 genes (). This PPINs result was then imported into Cytoscape software, generating a primary PPIN (). Using the CytoNCA plugin, we calculated the topological parameters and applied the first filtering, resulting in a core sub-network structure consisting of 31 nodes and 946 edges (). The median values for these parameters were as follows: Closeness: 0.39047619, Degree: 12, Eigenvector: 0.056804042, LAC: 5.714285714 and Network: 7.111111111. After the second round of filtering, the core sub-network structure was reduced to 13 nodes and 428 edges (), with the following parameter values: Closeness: 0.6, Degree: 20, Eigenvector: 0.152755275, LAC: 11.6 and Network: 12.97607656. The 13 hub genes in this final sub-network are CDKN1A, CASP3, STAT1, TP53, JUN, MAPK1, STAT3, MAPK3, MYC, HIF1A, FOS, MAPK14 and AKT1.
3.9. Results of molecular docking
We obtained three datasets from the Gene Expression Omnibus (GEO) database [Citation66]. There were GSE116312, GSE27411 [Citation67] and GSE54129. Differentially expressed genes (DEGs) were screened for normal gastric tissue and CAG using p value <.05, |fold change| ≥ 2 [Citation68] as the threshold (). One thousand and twenty-four DEGs were taken and intersected with 13 hub genes of the PPI network to obtain MAPK1 and MAPK3 (). The protein corresponding to the MAPK1 gene target was found to have the identifier P28482 in the UniProt database, while the MAPK3 gene corresponds to Q16644.
Figure 11. Protein receptor-small molecule ligand docking of two differently expressed genes (DEGs). (A) Volcano plot with differentially expressed genes (DEGs) between normal stomach and chronic atrophic gastritis tissue. (B) Venn diagram showing the intersecting hub genes of 1204 DEGs and 13 hub genes. (C) MAPK1 (P28482) and naringenin. (D) MAPK1 (P28482) and luteolin. (E) MAPK1 (P28482) and quercetin. (F) MAPK3 (Q16644) and naringenin.

We have downloaded the 3D structures of both proteins from the PDB database and the 2D structures of the small molecule probes (naringenin, luteolin and quercetin) for three drugs from the PubChem database. Finally, we utilized software to perform molecular docking and visualization, resulting in four protein receptor-ligand docking results as presented in . The positions of residues with hydrogen bonds were visualized by PyMOL. The results reveal that naringenin luteolin and forms hydrogen bonds with MET-108 positions (), quercetin forms hydrogen bonds at the ASP-106, MET-108, ASN-154 and SER-153 positions (). For the MAPK3 gene, naringenin forms hydrogen bonds at the ASP-149 positions ().
Table 8. Binding energy between main active components and key protein targets (kJ/mol).
4. Discussion
The significance of CAG in the progression of GC necessitates the implementation of proactive and effective pharmacological interventions during the early stages of prevention and treatment. In this study, we employed a meta-analysis to examine whether the addition of TCM formula showed higher efficacy in treating CAG in the experimental group. Our research revealed the following findings: (1) among the three clinical outcome measures (effective, significant, ineffective), the clinical efficacy in the experimental group surpassed that of the control group; (2) in terms of the four clinical outcome grades (recovery, effective, significant, ineffective), the experimental group exhibited a higher recovery rate and demonstrated significantly better results compared to using western medicine alone; (3) the incidence rate of adverse events was lower statistically. Therefore, we believe that the intervention of TCM formula holds advantages in treating CAG. The results of the NMA indicated that the combined use of TCM formula showed higher cumulative probability compared to the use of western medicine alone. These studies unequivocally demonstrate the wisdom of incorporating TCM in the treatment plans for CAG. However, it must be acknowledged that the aforementioned meta-analyses have certain limitations: First, due to considerations of medication costs, the inclusion of studies may have exhibited poor methodological quality, with few experiments mentioning double-blinding. Second, there may be reporting bias due to inconsistent age distribution and disease duration among patients. Finally, CAG falls within the realm of chronic diseases, yet the intervention duration of the studies included is less than three months, making the treatment and follow-up periods insufficient to observe long-term clinical effects of intervention.
Utilizing association rule analysis, the following seven hub Chinese herbal medicines were identified: Paeonia lactiflora, Atractylodes macrocephala, Pinellia ternata, Citrus reticulata, Codonopsis pilosula, Salvia miltiorrhiza and Coptis chinensis. In TCM treatment, it is crucial to differentiate and treat diseases based on syndrome differentiation. For the spleen and stomach deficiency-cold type of CAG [Citation52,Citation63], Salvia miltiorrhiza has the effects of promoting blood circulation, removing blood stasis, regulating menstruation, alleviating pain and cooling the blood to resolve abscesses. Coptis chinensis, Pinellia ternata and Codonopsis pilosula have the effects of promoting blood circulation and invigorating Qi. Atractylodes macrocephala tonifies Qi, strengthens the spleen, and eliminates dampness. Citrus reticulata tonifies the spleen, warms the stomach, regulates Qi, and thereby relieves gastrointestinal symptoms, improves gastric mucosal circulation and enhances the body’s immune system. For patients with blood stasis in stomach collaterals syndrome [Citation60], Codonopsis pilosula and Atractylodes macrocephala are skilled at strengthening the spleen and consolidating the foundation, with the effects of invigorating Qi and promoting its smooth flow. Pinellia ternata can eliminate dampness, transform phlegm, reduce Rebellious Qi, stop vomiting and disperse masses. Salvia miltiorrhiza nourishes the blood, promotes blood circulation, removes blood stasis and generates new blood. Paeonia lactiflora has the function of soothing the liver, nourishing the blood, and restraining Yin. For patients with both Qi and Yin deficiency [Citation61], Atractylodes macrocephala can tonify the spleen, replenish Qi and eliminate dampness. By docking the active ingredients of Chinese herbs with the disease gene targets of CAG, the regulatory network of Chinese herbal compound can be observed for their interactions.
We have identified 13 hub genes through the exploration of the PPINs of drug targets and genes. In addition, we have selected the DEGs MAPK1 and MAPK3 in normal and CAG tissues. MAPK1 is a factor that is important in the value-added and metastasis of GC [Citation69], MAPK3 expression is an independent prognostic marker in GC patients [Citation70], which aligns with the findings of our KEGG analysis, where the most enriched pathways are associated with cancer. The PI3K-Akt signalling pathway was shown to inhibit precancerous lesions of GC in in vitro experiments [Citation64]. The MAPK signalling pathway also plays an important role in the progression of GC [Citation71,Citation72]. Therefore, it is reasonable to hypothesize that targeting these two genes may impede the progression of CAG to GC. In GO analysis, the targeted genes are predominantly enriched in protein binding, indicating the binding of drugs entering the bloodstream with plasma proteins.
Quercetin not only demonstrates significant anti-GC activity [Citation65]. Additionally, it demonstrates anti-inflammatory properties by restraining the expression of matrix metalloproteinase-9, induced by tumour necrosis factor-α-induced, in normal human gastric epithelial cells [Citation73]. Luteolin regulates GC cells through multiple signalling pathways [Citation74], exhibiting anti-proliferative and chemo sensitizing effects [Citation75]. Naringenin has been shown to have the potential to treat unused types of cancer [Citation76]. Based on the summarized published studies, we have found that the drug activities revealed by our research exhibit anti-proliferative effects in GC, which is a crucial process in the development of GC. Therefore, we boldly propose the hypothesis of incorporating active molecules into TCM formulations, particularly in the three herbs: Coptis chinensis (Huanglian), Codonopsis pilosula (Dangshen) and Citrus reticulata (Chenpi). Alternatively, these herbal plants can be utilized to extract effective components for new drug design and development. Of course, these ideas need to undergo a series of experiments, including cell, animal and clinical studies.
5. Conclusions
In conclusion, this research is based on meta-analysis studies and utilizes NP techniques to explore the potential mechanisms of action of hub herbal formulae in treating CAG. The results demonstrate that incorporating herbal formulae into the clinical treatment regimen for CAG yields better clinical outcomes compared to using Western medicine alone. Furthermore, it is elucidated that the herbal formulae primarily exert their effects through the interaction of three active molecular components with two DEGs. Finally, several potential targets for CAG treatment and their non-covalent interactions are identified, offering insights into the pathogenesis and providing new strategies for clinical management.
Author contributions
The idea, formal analysis, methodology and writing – original draft from J.Weng. The data curation, validation and visualization from X.F. Wu, P. Shao and X.P. Liu. The supervision, project administration, funding acquisition and writing – review and editing from C.X. Wang. All authors reviewed the manuscript.
Ethical approval
The studies we searched were taken from publicly available databases. The patients involved in the studies have received ethical approval. Our studies are based on published research, so there are no ethical issues or other conflicts of interest.
Disclosure statement
No potential conflict of interest was reported by the author(s).
Data availability statement
The studies for meta-analysis were all searchable in the databases mentioned in the study.
Additional information
Funding
References
- Rugge M, Correa P, Dixon MF, et al. Gastric mucosal atrophy: interobserver consistency using new criteria for classification and grading. Aliment Pharmacol Ther. 2002;16(7):1–22. doi: 10.1046/j.1365-2036.2002.01301.x.
- Yang H, Yang W-J, Hu B. Gastric epithelial histology and precancerous conditions. World J Gastrointest Oncol. 2022;14(2):396–412. doi: 10.4251/wjgo.v14.i2.396.
- Yin Y, Liang H, Wei N, et al. Prevalence of chronic atrophic gastritis worldwide from 2010 to 2020: an updated systematic review and meta-analysis. Ann Palliat Med. 2022;11(12):3697–3703. doi: 10.21037/apm-21-1464.
- Ma X, Zhang Z, Qin X, et al. Therapeutic effect of curcumol on chronic atrophic gastritis (CAG) and gastric cancer is achieved by downregulating SDF-1α/CXCR4/VEGF expression. J Oncol. 2022;2022:3919053.
- Wang L, Ding X, Li P, et al. Efficacy and safety of Weifuchun tablet for chronic atrophic gastritis: a systematic review and meta-analysis. PLOS One. 2023;18(4):e0284411. doi: 10.1371/journal.pone.0284411.
- Chen X, Shen K, Deng Y, et al. A randomized double-blind clinical trial of Weierkang pills for the treatment of chronic atrophic gastritis. J Clin Gastroenterol. 2023;57(2):165–171. doi: 10.1097/MCG.0000000000001663.
- Zhou W, Zhang H, Wang X, et al. Network pharmacology to unveil the mechanism of moluodan in the treatment of chronic atrophic gastritis. Phytomedicine. 2022;95:153837. doi: 10.1016/j.phymed.2021.153837.
- Zhang T, Zhang B, Xu J, et al. Chinese herbal compound prescriptions combined with Chinese medicine powder based on traditional Chinese medicine syndrome differentiation for treatment of chronic atrophic gastritis with erosion: a multi-center, randomized, positive-controlled clinical trial. Chin Med. 2022;17(1):142. doi: 10.1186/s13020-022-00692-7.
- Reichardt W, Schüler E, Sieber L, et al. Quantitative determination of the protein content of milk by ultraviolet spectrophotometry. 3. Determination of proteins in preserved milk samples. Nahrung. 1987;31(8):801–807. doi: 10.1002/food.19870310810.
- Wang X-M, Zhang X-R, Li Z-H, et al. A brief introduction of meta-analyses in clinical practice and research. J Gene Med. 2021;23(5):e3312. doi: 10.1002/jgm.3312.
- Nordmann AJ, Kasenda B, Briel M. Meta-analyses: what they can and cannot do. Swiss Med Wkly. 2012;142:w13518. doi: 10.4414/smw.2012.13518.
- Dawson DV, Pihlstrom BL, Blanchette DR. Understanding and evaluating meta-analysis. J Am Dent Assoc. 2016;147(4):264–270. doi: 10.1016/j.adaj.2015.10.023.
- Ahn E, Kang H. Concepts and emerging issues of network meta-analysis. Korean J Anesthesiol. 2021;74(5):371–382. doi: 10.4097/kja.21358.
- Noor F, Asif M, Ashfaq UA, et al. Machine learning for synergistic network pharmacology: a comprehensive overview. Brief Bioinform. 2023;24(3):bbad120. doi: 10.1093/bib/bbad120.
- Boezio B, Audouze K, Ducrot P, et al. Network-based approaches in pharmacology. Mol Inform. 2017;36(10):1700048. doi: 10.1002/minf.201700048.
- Hopkins AL. Network pharmacology: the next paradigm in drug discovery. Nat Chem Biol. 2008;4(11):682–690. doi: 10.1038/nchembio.118.
- Li X, Liu Z, Liao J, et al. Network pharmacology approaches for research of traditional Chinese medicines. Chin J Nat Med. 2023;21(5):323–332. doi: 10.1016/S1875-5364(23)60429-7.
- Zhao L, Zhang H, Li N, et al. Network pharmacology, a promising approach to reveal the pharmacology mechanism of Chinese medicine formula. J Ethnopharmacol. 2023;309:116306. doi: 10.1016/j.jep.2023.116306.
- Yuan H, Ma Q, Cui H, et al. How can synergism of traditional medicines benefit from network pharmacology? Molecules. 2017;22(7):1135. doi: 10.3390/molecules22071135.
- Noor F, Tahir Ul Qamar M, Ashfaq UA, et al. Network pharmacology approach for medicinal plants: review and assessment. Pharmaceuticals. 2022;15(5):572. doi: 10.3390/ph15050572.
- Sayers EW, Beck J, Bolton EE, et al. Database resources of the national center for biotechnology information. Nucleic Acids Res. 2021;49(D1):D10–D17. doi: 10.1093/nar/gkaa892.
- Frandsen TF, Eriksen MB, Hammer DMG, et al. Using Embase as a supplement to PubMed in Cochrane reviews differed across fields. J Clin Epidemiol. 2021;133:24–31. doi: 10.1016/j.jclinepi.2020.12.022.
- MacLehose H, Hilton J. Changes to the Cochrane library during the Cochrane Collaboration’s first 20 years. Cochrane Database Syst Rev. 2013;2013(2):ED000050.
- Huang R, Chen Y, Sun Y. An enhancer of autonomous innovation capability – "CNKI" (China National Knowledge Infrastructure) and its application in the Chinese Knowledge Resource Repository. Library World. 2006;2006(1):63–67.
- Li Z. China biomedical literature analysis and retrieval system. Med Intell Work. 1993;1993(1):49–51.
- Introduction to Wanfang database. China Inform Herald. 1995;1995(3):33–34.
- Wu S. A successful attempt to modernize searching with Chinese characteristics - an interview with "Chinese Science and Technology Journal Database”. Intell Work. 1995;1995(2):44–46.
- Moher D, Jadad AR, Nichol G, et al. Assessing the quality of randomized controlled trials: an annotated bibliography of scales and checklists. Control Clin Trials. 1995;16(1):62–73. doi: 10.1016/0197-2456(94)00031-w.
- Armijo-Olivo S, Stiles CR, Hagen NA, et al. Assessment of study quality for systematic reviews: a comparison of the Cochrane collaboration risk of bias tool and the effective public health practice project quality assessment tool: methodological research. J Eval Clin Pract. 2012;18(1):12–18. doi: 10.1111/j.1365-2753.2010.01516.x.
- Cuijpers P, Weitz E, Cristea IA, et al. Pre-post effect sizes should be avoided in meta-analyses. Epidemiol Psychiatr Sci. 2017;26(4):364–368. doi: 10.1017/S2045796016000809.
- Kelley K, Preacher KJ. On effect size. Psychol Methods. 2012;17(2):137–152. doi: 10.1037/a0028086.
- Bencao CMMZ. An editorial committee of the administration bureau of traditional Chinese medicine. Shanghai: Shanghai Science and Technology Press; 2000.
- Chinese Pharmacopoeia. Pharmacopoeia of the People’s Republic. 2020. Beijing: China Medical Science and Technology Press; 2022. p. 54–55.
- Ru J, Li P, Wang J, et al. TCMSP: a database of systems pharmacology for drug discovery from herbal medicines. J Cheminform. 2014;6(1):13. doi: 10.1186/1758-2946-6-13.
- UniProt: a worldwide hub of protein knowledge. Nucleic Acids Res. 2019;47(D1):D506–D515.
- Safran M, Dalah I, Alexander J, et al. GeneCards version 3: the human gene integrator. Database. 2010;2010:baq020.
- Amberger JS, Bocchini CA, Schiettecatte F, et al. OMIM.org: Online Mendelian Inheritance in Man (OMIM®), an online catalog of human genes and genetic disorders. Nucleic Acids Res. 2015;43:D789–D798. doi: 10.1093/nar/gku1205.
- Barbarino JM, Whirl-Carrillo M, Altman RB, et al. PharmGKB: a worldwide resource for pharmacogenomic information. Wiley Interdiscip Rev Syst Biol Med. 2018;10(4):e1417.
- Zhou Y, Zhang Y, Lian X, et al. Therapeutic Target Database Update 2022: facilitating drug discovery with enriched comparative data of targeted agents. Nucleic Acids Res. 2022;50(D1):D1398–D1407. doi: 10.1093/nar/gkab953.
- Svensson F, Westerman B, Würdinger T, et al. GBM Drug Bank—a new resource for glioblastoma drug discovery and informatics research. Neuro Oncol. 2018;20(12):1680–1681. doi: 10.1093/neuonc/noy122.
- Shannon P, Markiel A, Ozier O, et al. Cytoscape: a software environment for integrated models of biomolecular interaction networks. Genome Res. 2003;13(11):2498–2504. doi: 10.1101/gr.1239303.
- Huang DW, Sherman BT, Lempicki RA. Systematic and integrative analysis of large gene lists using DAVID bioinformatics resources. Nat Protoc. 2009;4(1):44–57. doi: 10.1038/nprot.2008.211.
- Gene Ontology Consortium: going forward. Nucleic Acids Res. 2015;43:D1049–D1056.
- Du J, Yuan Z, Ma Z, et al. KEGG-PATH: Kyoto Encyclopedia of Genes and Genomes-Based Pathway Analysis using a path analysis model. Mol Biosyst. 2014;10(9):2441–2447. doi: 10.1039/c4mb00287c.
- Ito K, Murphy D. Application of ggplot2 to pharmacometric graphics. CPT Pharmacometr Syst Pharmacol. 2013;2(10):e79. doi: 10.1038/psp.2013.56.
- Szklarczyk D, Gable AL, Nastou KC, et al. The STRING database in 2021: customizable protein–protein networks, and functional characterization of user-uploaded gene/measurement sets. Nucleic Acids Res. 2021;49(D1):D605–D612. doi: 10.1093/nar/gkaa1074.
- Gao X, Wang X, Zhang S. Bioinformatics identification of crucial genes and pathways associated with hepatocellular carcinoma. Biosci Rep. 2018;38(6):BSR20181441. doi: 10.1042/BSR20181441.
- Shin J-M, Cho D-H. PDB-Ligand: a ligand database based on PDB for the automated and customized classification of ligand-binding structures. Nucleic Acids Res. 2005;33:D238–D241. doi: 10.1093/nar/gki059.
- Wang Y, Xiao J, Suzek TO, et al. PubChem’s BioAssay database. Nucleic Acids Res. 2012;40:D400–D412. doi: 10.1093/nar/gkr1132.
- Goodsell DS, Sanner MF, Olson AJ, et al. The AutoDock suite at 30. Protein Sci. 2021;30(1):31–43. doi: 10.1002/pro.3934.
- Seeliger D, de Groot BL. Ligand docking and binding site analysis with PyMOL and AutoDock/Vina. J Comput Aided Mol Des. 2010;24(5):417–422. doi: 10.1007/s10822-010-9352-6.
- Chen J. Observation of treatment efficacy for chronic atrophic gastritis using an integrated approach combining traditional Chinese medicine and Western medicine. J Pract Tradit Chin Med. 2018;34(2):214–215.
- Dai W. Clinical efficacy observation of modified Xiang Sha Liu Jun Zi Tang in the treatment of chronic atrophic gastritis. Res Tradit Chin Med. 2021;2021(48):81–83.
- Fu H. Observation of treatment efficacy for chronic atrophic gastritis using an integrated approach combining traditional Chinese medicine and Western medicine. Chin Manipul Rehabil Med. 2014;5(3):138–139.
- Gao Y. Clinical observation of the therapeutic efficacy of integrating traditional Chinese medicine and Western medicine in treating chronic atrophic gastritis. World Latest Med Inform. 2016;16(65):150–150.
- Lü J, Jia X. Clinical observation of integrative medicine approach in treating chronic atrophic gastritis. Chin Pract Med. 2013;8(13):188–189.
- Liu F. Analysis of the therapeutic effect of Yiwei decoction in the treatment of chronic atrophic gastritis. China Contin Med Educ. 2020;12(21):148–150.
- Liu W, Jiang Y, Long F, et al. Effect of spleen strengthening and gastric formula on the pathomorphology and EGF expression of gastric mucosa in 30 cases of chronic atrophic gastritis. Chin J Ethnomed Ethnopharm. 2015,24(09):95–96.
- Wang F, Wang Z, Zhang B, et al. Effect of Huazhuo Jiedu prescription on TCM syndrome scores, gastroscopic mucosal signs, and cytokines in patients with chronic atrophic gastritis. Hainan Med. 2021;32(23):3046–3049.
- Wang L. Effect of Yi Wei Huo Luo Jing Fang’s combined triple therapy on gastric mucosal glands and serum inflammatory factors in patients with chronic atrophic gastritis due to blood stasis in the stomach complex. Shaanxi Tradit Chin Med. 2016;37(01):48–50.
- Wang Q, Song Q, Chen Q. Hui Chun Jian Wei decoction in the treatment of chronic atrophic gastritis with deficiency of both Qi and Yin. Pract Clin J Integr Tradit Chin West Med. 2022;22(19):26–28,32.
- Yan Y. Effects of Banxia Xiexin decoction combined with triple therapy in treatment of patients with chronic atrophic gastritis. China Natl Health Med. 2022;34(13):96–99.
- Lu Y, Zhou Y, Xiao M, et al. Clinical effect of Wenzhong Fuxing Cuyu decoction on chronic atrophic gastritis and its effect on gastrointestinal hormones. Liaoning J Tradit Chin Med. 2022;49(11):98–101.
- Wang Y, Chu F, Lin J, et al. Erianin, the main active ingredient of Dendrobium chrysotoxum Lindl, inhibits precancerous lesions of gastric cancer (PLGC) through suppression of the HRAS-PI3K-AKT signaling pathway as revealed by network pharmacology and in vitro experimental verification. J Ethnopharmacol. 2021;279:114399. doi: 10.1016/j.jep.2021.114399.
- Wang B, Wang J, Zhao X-H. Bioactivity of two polyphenols quercetin and fisetin against human gastric adenocarcinoma AGS cells as affected by two coexisting proteins. Molecules. 2022;27(9):2877. doi: 10.3390/molecules27092877.
- Edgar R, Domrachev M, Lash AE. Gene Expression Omnibus: NCBI gene expression and hybridization array data repository. Nucleic Acids Res. 2002;30(1):207–210. doi: 10.1093/nar/30.1.207.
- Nookaew I, Thorell K, Worah K, et al. Transcriptome signatures in Helicobacter pylori-infected mucosa identifies acidic mammalian chitinase loss as a corpus atrophy marker. BMC Med Genomics. 2013;6(1):41. doi: 10.1186/1755-8794-6-41.
- Dowling L, Duseja A, Vilaca T, et al. MicroRNAs in obesity, sarcopenia, and commonalities for sarcopenic obesity: a systematic review. J Cachexia Sarcopenia Muscle. 2022;13(1):68–85. doi: 10.1002/jcsm.12878.
- Wang Y, Li M, Zeng J, et al. MiR-585-5p impedes gastric cancer proliferation and metastasis by orchestrating the interactions among CREB1, MAPK1 and MITF. Front Immunol. 2022;13:1008195. doi: 10.3389/fimmu.2022.1008195.
- Kim JG, Lee SJ, Chae YS, et al. Association between phosphorylated AMP-activated protein kinase and MAPK3/1 expression and prognosis for patients with gastric cancer. Oncology. 2013;85(2):78–85. doi: 10.1159/000351234.
- Jiang T, Xia Y, Lv J, et al. A novel protein encoded by circMAPK1 inhibits progression of gastric cancer by suppressing activation of MAPK signaling. Mol Cancer. 2021;20(1):66. doi: 10.1186/s12943-021-01358-y.
- Shen Y, Zhang N, Chai J, et al. CircPDIA4 induces gastric cancer progression by promoting ERK1/2 activation and enhancing biogenesis of oncogenic circRNAs. Cancer Res. 2023;83(4):538–552. doi: 10.1158/0008-5472.CAN-22-1923.
- Hsieh H-L, Yu M-C, Cheng L-C, et al. Quercetin exerts anti-inflammatory effects via inhibiting tumor necrosis factor-α-induced matrix metalloproteinase-9 expression in normal human gastric epithelial cells. World J Gastroenterol. 2022;28(11):1139–1158. doi: 10.3748/wjg.v28.i11.1139.
- Pu Y, Zhang T, Wang J, et al. Luteolin exerts an anticancer effect on gastric cancer cells through multiple signaling pathways and regulating miRNAs. J Cancer. 2018;9(20):3669–3675. doi: 10.7150/jca.27183.
- Wu B, Zhang Q, Shen W, et al. Anti-proliferative and chemosensitizing effects of luteolin on human gastric cancer AGS cell line. Mol Cell Biochem. 2008;313(1–2):125–132. doi: 10.1007/s11010-008-9749-x.
- Motallebi M, Bhia M, Rajani HF, et al. Naringenin: a potential flavonoid phytochemical for cancer therapy. Life Sci. 2022;305:120752. doi: 10.1016/j.lfs.2022.120752.