Abstract
The associations between multiple sleep characteristics and smoking behavior are inconsistent, and it is unclear which sleep characteristics are most crucial for tobacco prevention. This study aimed to explore the associations between smoking status/intensity and multiple sleep characteristics and to identify the potential core domain of smoking-related sleep using network analysis. Data were obtained from a survey of cancer-related risk factors among Chinese adults. Logistic regression models were used to quantify the associations between sleep characteristics and smoking status/intensity. Network analyses were employed to identify the core sleep characteristics. A total of 5,228 participants with a median age of 44 years old were included in the study. Current smoking was significantly positively associated with long nap time, difficulty falling asleep, late bedtime, getting up after 7 am, and waking up earlier than expected. There was significant positive association between current smoking and short sleep duration in young adults under 45 years old. Late bedtime and getting up after 7 am were only associated with current heavy smoking, but not current light smoking. Network analyses showed that multiple smoking-related sleep characteristics were interconnected, with difficulty falling asleep and late bedtime as central characteristics in the network. The study found that the associations between sleep characteristics and smoking varied by age and smoking intensity and highlights the potential benefits of sleep health promotion in smoking cessation, with a particular focus on difficulty falling asleep and late bedtime.
Introduction
There were 1.14 billion current smokers worldwide in 2019, approximately 30% (341 million) of whom lived in China [Citation1]. A number of diseases, including chronic obstructive pulmonary disease, cardiovascular disease, and cancer, have been linked to smoking [Citation2–6]. More than one million people in China die each year from smoking-related diseases [Citation7]. Given the high prevalence and tremendous health impact of smoking, China has implemented policies to control tobacco use since 1979, such as raising the tobacco tax and advocating a smoke-free environment [Citation8]. These efforts have resulted in a 20.9% decline (from 62.8% in 1990 to 49.7% in 2019) in smoking prevalence among men and 18.2% (from 4.3% in 1990 to 3.5% in 2019) among women aged 15 years and older between 1990 and 2019 in China [Citation1]. However, the smoking reduction was still lower than the global average decline (27.5% among men and 37.7% among women) for the same period [Citation1], indicating the current tobacco prevention and control effort in China could be optimized. It is imperative to identify the influencing factors of smoking and establish more effective tobacco prevention and control strategies.
Sleep has been linked to smoking, however, the vast majority of the previous studies have focused on composite sleep scores [Citation9–12] or a single sleep duration [Citation13–20], with inconsistent results for specific sleep characteristics and smoking. Several studies demonstrated that self-reported sleep duration [Citation13–18], sleep latency [Citation19,Citation21,Citation22], difficulty maintaining sleep [Citation19,Citation21–23] and sleep disturbances [Citation24,Citation25] were associated with smoking, while other studies showed no significant differences between smokers and non-smokers in sleep duration [Citation14,Citation16,Citation18,Citation19], sleep latency [Citation11,Citation26] and sleep disturbances [Citation27,Citation28]. The inconsistent results could be due to differences in sleep assessment and smoking definition. Few previous studies have considered multiple sleep characteristics within the same study [Citation11,Citation22]. Additionally, some sleep characteristics such as getting up time and late bedtime, have not been well studied in relation to smoking.
Sleep is a complex multidimensional state, in which many sleep characteristics were interrelated [Citation29]. Network analysis can visually reveal intricate relationships among multiple interrelated characteristics by constructing pairwise conditional associations (edges) between sleep characteristics (network nodes) [Citation30–32]. It provides a strategy to identify the most influential ones within those interrelated sleep characteristics. Enhancing core characteristics has the potential to trigger a maximal cascading effect on other characteristics. As such, it presents a potential target for prevention, ultimately optimizing efficiency of sleep amelioration for potential smoking control.
In light of the above knowledge gaps, we analyzed data from a cross-sectional study conducted in China to further examine the associations between multiple sleep characteristics and smoking status/intensity, and to understand the interrelationships among the smoking-related sleep characteristics.
Methods
Data sources and sample
A cross-sectional study of cancer-related risk factors was conducted from April to October 2021 including 7,609 residents over 18 years old who mainly lived in Gansu and Henan provinces of China. A standardized electronic module-based questionnaire was administered to all participants after they signed electronic informed consent through the Smart Health Management Digital Platform for Primary Cancer Prevention (SmartHMDP-PCP) [Citation33]. Adults who attended a physical examination at a medical institution in Lanzhou or who were permanent residents at a community in Zhengzhou were recruited into the study. Individuals from other locations were recruited through a convenient sampling method. The study was approved by the Ethics Committee of the National Cancer Center/Cancer Hospital, Chinese Academy of Medical Sciences and Peking Union Medical College (Approval No. 21/460-3131). Participants with missing information on cigarette smoking (n = 2,048) and sleep (n = 333) were excluded from the study. A total of 5,228 participants were retained for final analysis.
Measures
Definition of smoking
The study collected detailed self-reported smoking information, including smoking status, daily cigarette consumption, and smoking duration. Smoking status was categorized into three groups: (1) non-smokers: individuals who had never smoked, (2) current smokers: individuals who had smoked at least 1 cigarette per day for over a month or had smoked more than 100 cigarettes in their lifetime and were still smoking at the time of interview, and (3) former smokers: individuals who had smoked at least 1 cigarette per day for over a month or had smoked more than 100 cigarettes in their lifetime but were no longer smoking at the time of interview [Citation34,Citation35]. Additionally, for sensitivity analysis, we further restricted former smokers to those who had quitted smoking for at least 1 year to avoid sleep-related withdrawal symptoms in short-term quitters [Citation12,Citation36]. Smoking intensity was quantified in pack-years, which was calculated as the average number of packs (20 cigarettes per pack) smoked per day multiplied by smoking duration in years [Citation37]. Participants were further categorized as light smokers (≤ 10 pack-years) or heavy smokers (> 10 pack-years) [Citation38,Citation39].
Assessment of sleep
Sleep information was collected for the past three months before the study. The sleep characteristics included short sleep duration (less than 7 h) [Citation40], long nap time (greater than 1 h), difficulty falling asleep (sleep latency greater than 30 min), late bedtime (after 11 pm), getting up after 7 am, difficulty maintaining sleep (waking up more than 30 min per night), waking up earlier than expected (more than 30 min), and use of sleep medication.
Pre-Sleep activities
We asked participants about their activities during the last hour before sleep, including mild entertainment activities (e.g. reading books, listening to audiobooks, watching TV, or using the computer for leisure purposes), intense entertainment activities (e.g., watching intense films or playing highly confrontational games), chatting over the phone, performing intellectual work, light physical activities (e.g., yoga or walking), vigorous physical activities (e.g., running or swimming), and eating (except for drinking milk).
Covariates
Covariates, including age, sex, marital status, highest education level, resident region, alcohol consumption, physical activity, depressive symptoms, and anxiety symptoms were also collected. Marital status was dichotomized as married and not in marriage (including divorced, widowed, or single). Education level was categorized as low (below bachelor’s degree) and high (bachelor’s degree and above). According to the Dietary Guidelines for Chinese Residents (2022), alcohol consumption was classified as within and without the healthy limit (15 g ethanol/day) [Citation41]. A total metabolic equivalent (MET) was used to measure the energy expenditure of physical activity, which was calculated by summing the products of physical activity frequency, duration, and intensity [Citation42]. Ratings of 1.25, 2.3, 4.5, and 7.5 METs were assigned for sedentary, light, moderate, and vigorous physical activity, respectively. Active physical activity was defined as the MET greater than the lowest tertile of total MET. Depressive symptoms and anxiety symptoms were assessed using the Self-rating Depression Scale (SDS) [Citation43] and the Self-rating Anxiety Scale (SAS) [Citation44], respectively. SDS score ≥ 53 was defined as having depressive symptoms, and SAS score ≥ 50 was defined as having anxiety symptoms.
Statistical analysis
The characteristics of participants by smoking status were expressed as the frequency with percentage for categorical variables and median with interquartile range (IQR) for continuous variables. Mann-Whitney U-test was used to compare continuous variables and the Chi-squared test for categorical variables. Multivariable binary logistic regression models were used to estimate adjusted odds ratios (AORs) and 95% confidence intervals (95% CIs) for the associations between sleep characteristics and smoking status/intensity.
Given the majority of the current/former smokers were men, we conducted sensitivity analyses among men. Additionally, recognizing that sleep characteristics of individuals with history of chronic diseases may differ from the general population [Citation45–49], we performed analyses by excluding those individuals. Stratified analyses by age were also conducted (< 45 versus ≥ 45 years). The equations of logistic models for primary analysis and stratified analyses were showed in Supplementary Materials. The percentage of missing covariates was less than 10% (Table S1), and missing values were handled by multiple imputations via chained equations using R-package mice [Citation50].
Network analysis was used to explore the relationships between smoking-related sleep characteristics, including those sleep characteristics that were significantly associated with smoking status/intensity in the logistic regression models. Using the R-package qgraph, we estimated the partial correlation network between binary variables based on Ising model [Citation51]. Additionally, we employed the least absolute shrinkage and selection operator combined with the extended Bayesian information criterion for model selection, to minimize the risk of spurious associations [Citation31,Citation52]. The partial correlation network displayed the relationship between variables with controlling for other variables. Node position in the network was determined by the Fruchterman–Reingold algorithm so that nodes with more connections and strong associations were close to each other [Citation53]. In the network, blue and red edges represent positive and negative correlations, respectively, and the strength of the relationship was illustrated by the thickness and density of the edges.
For the nodes in the network, we calculated three indicators to measure the core factors in the network, including betweenness, closeness, and strength. Betweenness measured how often a node lies on the shortest path of other pairs of nodes, a node with high betweenness acts as an important bridge between other nodes. Closeness represented the shortest distances from a node to other nodes. Strength was defined as the sum of the weights of all edges connected to the node [Citation54,Citation55].
The R-package bootnet was used to estimate the stability and accuracy of the network. A 95% nonparametric bootstrap confidence interval based on 1000 iterations was used to examine the accuracy of the network edge weights. An accurate network estimate exhibits narrow confidence intervals and bootstrap estimated means consistent with the original sample values [Citation56]. The accuracy of the centrality indices was evaluated using a case-dropping bootstrap with 1000 samples, and the correlation stability (CS) coefficient was calculated to quantify the correlation between the original sample estimate and the subsample estimate. The CS coefficient should optimally exceed 0.5 and must not be lower than 0.25 56. In addition, we conducted unconditional logistic regression models to evaluate pre-sleep activities between people with and without network central sleep characteristics.
All statistical analyses were completed using R (version 4.1.1). A two-sided p value < 0.05 was considered statistically significant.
Results
Characteristics of the study population
Of the 5,228 participants, the median age was 44 years (IQR: 34-52). More men (51.97%, n = 2,717) were included in the study, 14.23% of participants (n = 744) were current smokers, and 2.75% of participants (n = 144) were former smokers (). Compared with non-smokers, both current and former smokers were more likely to be men, married, live in Gansu province, have higher alcohol consumption, engage in active physical activity and have a history of chronic diseases. All of these differences were statistically significant (p < 0.05).
Table 1. Characteristics of participants by smoking status.
Associations between sleep characteristics and smoking status
As shown in , long nap time (AOR, 95% CI: 1.51, 1.03-2.23), difficulty falling asleep (1.66, 1.25-2.21), late bedtime (1.58, 1.24-2.00), getting up after 7 am (1.36, 1.11-1.68), and waking up earlier than expected (2.02, 1.22-3.34) were significantly positively associated with increasing current smoking. No statistically significant associations were found for short sleep duration, difficulty maintaining sleep, and use of sleep medication. In addition, only long nap time (2.11, 1.10-4.06) and waking up earlier than expected (2.97, 1.33-6.63) were significantly positively associated with former smoking compared to non-smokers. When former smokers were defined as having quit smoking for at least 1 year, the results of the sensitivity analysis (Table S3) were generally consistent with the main analysis. Analyses among men (Table S4) and participants without history of chronic diseases (Table S5) also reached consistent results with the overall population.
Table 2. Associations between sleep characteristics and smoking status.
In the age-stratified analysis (Figure S1), a significant interaction between age and short sleep duration was observed (Pinteraction=0.018). A significant positive association between short sleep duration and current smoking was found in adults younger than 45 years of age but not in older adults.
Associations between sleep characteristics and smoking intensity
We examined the associations between sleep characteristics and smoking intensity, using non-smokers as the reference group (). Overall, we found stronger positive associations among heavy smokers. Difficulty falling asleep and waking up earlier than expected were both significantly or marginally significantly positively associated with light and heavy smoking, respectively. However, late bedtime and getting up after 7 am are only statistically significantly associated with current heavy smoking but not current light smoking. Furthermore, former light smoking was only significantly positively associated with long nap time, and former heavy smoking was only significantly positively associated with late bedtime. Analyses conducted in men (Table S6) and participants without history of chronic diseases (Table S7) also yielded similar results to the overall population.
Table 3. Associations between sleep characteristics and smoking intensity.
The age-stratified analysis results showed that the association between short sleep duration and current heavy smoking varied across different age groups (Pinteraction=0.013), with a significant positive association observed in younger smokers but not in the elderly (Figure S2). No significant differences were observed in the association of other sleep characteristics with the smoking intensity between the two age groups.
Network analysis of smoking-related sleep characteristics
We conducted a network of smoking-related sleep characteristics among overall population (), incorporating all sleep characteristics that were statistically significantly associated with smoking status/intensity, including long nap time, difficulty falling asleep, late bedtime, getting up after 7 am, and waking up earlier than expected. Since short sleep duration was only significantly associated with smoking status in young adults and could be prevented by improving bedtime and getting up time, which have already been considered in the network, short sleep duration was not included.
Figure 1. Estimated network structure and centrality plot of smoking-related sleep characteristics among the overall population.
Abbreviations: Bed = late bedtime, Diff = difficulty falling asleep, Early = waking up earlier than expected, Getup = getting up after 7 am, Nap = long nap time.
Note. In the left panel displaying the estimated network structure, blue edges indicate positive effects and red edges indicate negative effects. In the right panel displaying the centrality plot, the y-axis shows the node name order by strength, the x-axis is the centrality value (standardized z-scores), the closeness of node’Nap’is missing because it is not connected to other nodes.
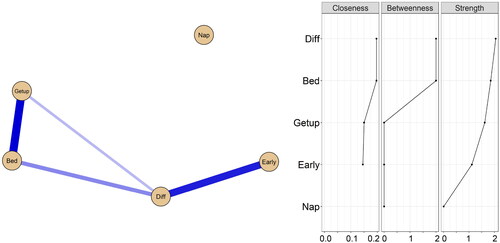
Long nap time was relatively isolated, while other smoking status-related sleep characteristics appeared close relationships. The results of the network centrality index showed that difficulty falling asleep and late bedtime were the central characteristics of the network. Difficulty falling asleep exhibited the greatest strength value, the betweenness and closeness values of the two sleep characteristics were similar. The edge-weight and case-dropping bootstrap results indicated that the network was approximately accurately estimated, and had high strength stability (Figure S3).
Pre-sleep behaviors of participants with network central sleep characteristics
In the smoking-related sleep characteristics network, we found that difficulty falling asleep and late bedtime were central characteristics, suggesting that effective intervention of these two sleep characteristics could improve other smoking-related sleep characteristics. To further explore potential causes of difficulty falling asleep and late bedtime, we analyzed the pre-sleep activities of participants with these two sleep characteristics (Tables S8–S9). We found that both people who had difficulty falling asleep and late bedtime were more likely to engage in intense entertainment activities and eating before sleep. Additionally, people who had late bedtime were more likely to engage in performing intellectual work and fewer light physical activities before going to bed.
Discussion
The study found multiple sleep characteristics including long nap time, difficulty falling asleep, late bedtime, getting up after 7 am, and waking up earlier than expected were associated with smoking. The observed associations varied by the intensity of smoking and the chronological age of the individuals. The study also for the first time identified that difficulty falling asleep and late bedtime were central factors among smoking-related sleep characteristics through network analysis.
The majority of previous studies have shown that short sleep duration was positively associated with smoking [Citation13–18,Citation57]. Although we did not find significant associations between short sleep duration and smoking status/intensity in our overall population, we found that short sleep duration was associated with current smoking and current heavy smoking in adults under the age of 45 years. This difference may be explained by the fact that older adults generally had shorter total sleep duration and better ability to cope with the adverse effects of short sleep than young adults [Citation58,Citation59]. Young adults with lower adaptability may be more inclined to rely on smoking as a coping mechanism for anxiety and fatigue arising from short sleep duration. Conversely, older adults, with more experience and effective strategies, may better manage the negative impact of short sleep duration. There were few epidemiological studies on nap time and smoking, and our findings were consistent with previous studies [Citation60,Citation61], which found a positive association between long nap duration and smoking status. Ensuring adequate nighttime sleep duration and avoiding long nap duration may be beneficial in reducing smoking.
Previous studies investigating the association of difficulty falling asleep, waking up earlier than expected and smoking have yielded inconsistent findings. Oftedal et al. suggested that smoking was associated with longer sleep onset latency, which was consistent with the method we measured for difficulty falling asleep [Citation62]. McNamara et al. observed that current smokers were more likely to report difficulty falling asleep and waking up early [Citation22]. Asghari et al. reported no difference in sleep latency between smokers and nonsmokers. However, they did not account for the confounding effects of other lifestyle factors, such as alcohol consumption, exercise, and mental health [Citation11]. Our study provided further evidence that, after adjusting for lifestyle and psychological factors, positive associations between difficulty falling asleep, waking up earlier than expected and current smoking were observed. Moreover, the observed association between difficulty falling asleep and current smoking became stronger among current heavy smokers.
Limited studies have investigated the associations of late bedtime and getting up time with smoking. Tobacco use was more prevalent in late chronotype [Citation13,Citation63,Citation64], while early chronotype was inversely associated with smoking [Citation13,Citation23,Citation65,Citation66], which were aligned with our findings. After stratification by light and heavy smoking, our study provided further information that late bedtime and getting up after 7 am were only associated with current heavy smoking but not current light smoking. This suggested that individualized sleep improvement measures should be taken for different smoking intensity.
Although the causal relationship between sleep characteristics and smoking could not be determined due to the cross-sectional nature of the study design, there are sociopsychological and biological mechanisms that could explain how sleep affects smoking behavior. People tend to smoke to relieve fatigue and self-medicate emotional dysfunctions when they have poor sleep states [Citation67]. Poor sleep states can lead to decreased attention and impaired decision-making and executive ability, which can reduce the ability to inhibit smoking behavior [Citation68]. Dopamine plays an important role in regulating positive emotions and the brain reward system [Citation69]. Poor sleep can down-regulate dopamine receptors in the ventral striatum of the brain, resulting in alterations in the reward nervous system due to decreased dopamine and leading to a compensatory increase in smoking desire [Citation69,Citation70].
To further shed light on the complex interactions between smoking-related sleep characteristics, we constructed a network analysis and found tight positive relationships among difficulty falling asleep, late bedtime, getting up after 7 am, and waking up earlier than expected. These sleep characteristics might formed a potentially positive feedback loop that facilitated smoking, in which difficulty falling asleep and late bedtime played central roles. Evidence from our study hinted that targeting core characteristics in prevention strategies might potentially influence the linkage changes of other factors, thereby suggesting an improvement in the efficiency of prevention. However, this inference was based on epidemiological associations and requires further researches for direct substantiation. Interestingly, we also found differences in pre-sleep behaviors between participants with and without difficulty falling asleep and late bedtime. Avoiding intense entertainment activities, eating, or intellectual work, and engaging in more light physical activities before sleep might be more specific and effective intervention measures.
Our study has several strengths and limitations. This study comprehensively explored the associations between multiple sleep characteristics and smoking. Network analysis provided novel insights into understanding the interrelationships between smoking-related sleep characteristics and identifying potential preventive features. As smoking and sleep characteristics were self-reported, which might lead to potential recall bias. Self-reported smoking often underestimates smoking rates, leading to a weakening of the true effect size [Citation71]. The participants in this study were mainly from Gansu and Henan provinces of China, and the prevalence of smoking in our study was 16.5%, which was lower than 26.6% in the previous surveys [Citation72], which might influence the generalizability of findings. Because there were only 34 female smokers, we were unable to explore sex differences in the associations between sleep characteristics and smoking in this study. The study provided preliminary insights into the associations between various sleep characteristics and smoking status, the results should be interpreted with caution due to the cross-sectional nature. Future longitudinal studies are warranted to determine the role of sleep improvement in the prevention of smoking. Given the complex exposure of individuals in the real world, it is also essential to assess the mixture effects of more risk factors on smoking to provide comprehensive information on tobacco prevention and control.
Conclusions
Our study showed that long nap time, difficulty falling asleep, late bedtime, getting up after 7 am and waking up earlier than expected were positively associated with smoking, where difficulty falling asleep and late bedtime were crucial factors in the smoking-related sleep characteristics network. These findings emphasize the potential benefits of sleep health promotion in smoking cessation, particularly addressing difficulty falling asleep and late bedtime. Further studies need to consider the potential effects of age and smoking intensity.
Author contributions
YWZ designed and conducted the research. YTX performed data analysis and drafted the paper. PYS, HH, JJW, YB, GYZ, FFY, DMZ, YQZ, and YWZ contributed to data collection. YTX, RRQ, ZLH, PYS, HH, KYZ, and YWZ revised the paper critically for important content. All authors read and approved the final version of the manuscript.
Supplemental Material
Download MS Word (1.5 MB)Acknowledgments
The authors thank all research staff members who participated in this work.
Disclosure statement
No potential conflict of interest was reported by the author(s).
Data availability statement
Due to the nature of this research, participants of this study did not agree for their data to be shared publicly, so supporting data is not available.
Additional information
Funding
References
- GBD 2019 Tobacco Collaborators. Spatial, temporal, and demographic patterns in prevalence of smoking tobacco use and attributable disease burden in 204 countries and territories, 1990-2019: a systematic analysis from the global burden of disease study 2019. Lancet. 2021;397(10292):1–11. doi: 10.1016/s0140-6736(21)01169-7.
- Jayes L, Haslam PL, Gratziou CG, et al. SmokeHaz: systematic reviews and meta-analyses of the effects of smoking on respiratory health. Chest. 2016;150(1):164–179. doi: 10.1016/j.chest.2016.03.060.
- Hackshaw A, Morris JK, Boniface S, et al. Low cigarette consumption and risk of coronary heart disease and stroke: meta-analysis of 141 cohort studies in 55 study reports. BMJ. 2018;360:j5855. doi: 10.1136/bmj.j5855.
- Chen ZM, Peto R, Iona A, et al. Emerging tobacco-related cancer risks in China: a nationwide, prospective study of 0.5 million adults. Cancer. 2015;121 (Suppl 17):3097–3106. doi: 10.1002/cncr.29560.
- Li TI, Chiang MT, Chiu KC, et al. The association of betel quid, alcohol, and cigarettes with salivary gland tumor-A case-control study. J Dent Sci. 2017;12(2):151–155. doi: 10.1016/j.jds.2016.12.002.
- Pang Y, Holmes MV, Guo Y, et al. Smoking, alcohol, and diet in relation to risk of pancreatic cancer in China: a prospective study of 0.5 million people. Cancer Med. 2018;7(1):229–239. doi: 10.1002/cam4.1261.
- Chen Z, Peto R, Zhou M, et al. Contrasting male and female trends in tobacco-attributed mortality in China: evidence from successive nationwide prospective cohort studies. Lancet. 2015;386(10002):1447–1456. doi: 10.1016/s0140-6736(15)00340-2.
- Hu TW. Tobacco control policy analysis in China: economics and health. Vol. 12. Singapore: World Scientific; 2008.
- Liao Y, Xie L, Chen X, et al. Sleep quality in cigarette smokers and nonsmokers: findings from the general population in Central China. BMC Public Health. 2019;19(1):808. doi: 10.1186/s12889-019-6929-4.
- Hwang JH, Park SW. The relationship between poor sleep quality measured by the pittsburgh sleep quality index and smoking status according to sex and age: an analysis of the 2018 korean community health survey. Epidemiol Health. 2022;44:e2022022. doi: 10.4178/epih.e2022022.
- Asghari A, Kamrava SK, Rezaee Hemami M, et al. Cigarette smoking habit and subjective quality of sleep. Scimetr. 2015;3(1):e18454. doi: 10.5812/scimetr.18454.
- Truong MK, Berger M, Haba-Rubio J, et al. Impact of smoking on sleep macro- and microstructure. Sleep Med. 2021;84:86–92. doi: 10.1016/j.sleep.2021.05.024.
- Patterson F, Malone SK, Lozano A, et al. Smoking, screen-based sedentary behavior, and diet associated with habitual sleep duration and chronotype: data from the UK biobank. Ann Behav Med. 2016;50(5):715–726. doi: 10.1007/s12160-016-9797-5.
- Yu J-G, Kim C-G, Jung S-P, et al. Associations between sleep duration and smoked cigarettes per day, heavy smoking in smokers. Korean J Health Promot. 2018;18(1):1. doi: 10.15384/kjhp.2018.18.1.1.
- Krueger PM, Friedman EM. Sleep duration in the United States: a cross-sectional population-based study. Am J Epidemiol. 2009;169(9):1052–1063. doi: 10.1093/aje/kwp023.
- Masood S, Cappelli C, Li Y, et al. Cigarette smoking is associated with unhealthy patterns of food consumption, physical activity, sleep impairment, and alcohol drinking in chinese male adults. Int J Public Health. 2015;60(8):891–899. doi: 10.1007/s00038-015-0730-7.
- Nuñez A, Rhee JU, Haynes P, et al. Smoke at night and sleep worse? The associations between cigarette smoking with insomnia severity and sleep duration. Sleep Health. 2021;7(2):177–182. doi: 10.1016/j.sleh.2020.10.006.
- You Y, Luo J, Elstgeest LEM, et al. Associations between health indicators and sleep duration of American adults: NHANES 2011-16. Eur J Public Health. 2021;31(6):1204–1210. doi: 10.1093/eurpub/ckab172.
- Nunez EC, Nunes S, Khan A, et al. Associations between major health behaviors and sleep problems: results from the 2015, 2016, 2017 Canadian community health survey. Behav Sleep Med. 2022;20(5):584–597. doi: 10.1080/15402002.2021.1968402.
- Patterson F, Grandner MA, Lozano A, et al. Transitioning from adequate to inadequate sleep duration associated with higher smoking rate and greater nicotine dependence in a population sample. Addict Behav. 2018;77:47–50. doi: 10.1016/j.addbeh.2017.09.011.
- Phillips BA, Danner FJ. Cigarette smoking and sleep disturbance. Arch Intern Med. 1995;155(7):734–737. doi: 10.1001/archinte.1995.00430070088011.
- McNamara JP, Wang J, Holiday DB, et al. Sleep disturbances associated with cigarette smoking. Psychol Health Med. 2014;19(4):410–419. doi: 10.1080/13548506.2013.832782.
- Gibson M, Munafò MR, Taylor AE, et al. Evidence for genetic correlations and bidirectional, causal effects between smoking and sleep behaviors. Nicotine Tob Res. 2019;21(6):731–738. doi: 10.1093/ntr/nty230.
- Wetter DW, Young TB. The relation between cigarette smoking and sleep disturbance. Prev Med. 1994;23(3):328–334. doi: 10.1006/pmed.1994.1046.
- Kashyap R, Hock LM, Bowman TJ. Higher prevalence of smoking in patients diagnosed as having obstructive sleep apnea. Sleep Breath. 2001;5(4):167–172. doi: 10.1007/s11325-001-0167-5.
- Conway SG, Roizenblatt SS, Palombini L, et al. Effect of smoking habits on sleep. Braz J Med Biol Res. 2008;41(8):722–727. doi: 10.1590/s0100-879x2008000800014.
- Hoflstein V. Relationship between smoking and sleep apnea in clinic population. Sleep. 2002;25(5):519–524.
- Casasola GG, Alvarez-Sala JL, Marques JA, et al. Cigarette smoking behavior and respiratory alterations during sleep in a healthy population. Sleep Breath. 2002;6(1):19–24. doi: 10.1007/s11325-002-0019-y.
- Zheng B, Lin LL, Yu CQ, et al. [Distributions and associations between duration of sleep, daytime naps and insomnia symptoms among chinese adults]. Zhonghua Liu Xing Bing Xue Za Zhi. 2017;38(4):452–456. doi: 10.3760/cma.j.issn.0254-6450.2017.04.008.
- Xu X, Xie T, Zhou N, et al. Network analysis of PGD, PTSD and insomnia symptoms in chinese shidu parents with PGD. Eur J Psychotraumatol. 2022;13(1):2057674. doi: 10.1080/20008198.2022.2057674.
- Lin Y, Bruner DW, Paul S, et al. A network analysis of self-reported psychoneurological symptoms in patients with head and neck cancer undergoing intensity-modulated radiotherapy. Cancer. 2022;128(20):3734–3743. doi: 10.1002/cncr.34424.
- Graziano RC, Aunon FM, LoSavio ST, et al. A network analysis of risk factors for suicide in Iraq/Afghanistan-era veterans. J Psychiatr Res. 2021;138:264–271. doi: 10.1016/j.jpsychires.2021.03.065.
- Huang H, Sun P, Zou K, et al. Current situation and prospect of primary prevention of malignant tumor in China. Zhonghua Zhong Liu Za Zhi. 2022;44(9):942–949. doi: 10.3760/cma.j.cn112152-20220209-00083.
- Shahab L, Beard E, Brown J. Association of initial e-cigarette and other tobacco product use with subsequent cigarette smoking in adolescents: a cross-sectional, matched control study. Tob Control. 2021;30(2):212–220. doi: 10.1136/tobaccocontrol-2019-055283.
- Qiu J, He X, Cui H, et al. Passive smoking and preterm birth in urban China. Am J Epidemiol. 2014;180(1):94–102. doi: 10.1093/aje/kwu092.
- Gallus S, Muttarak R, Franchi M, et al. Why do smokers quit? Eur J Cancer Prev. 2013;22(1):96–101. doi: 10.1097/CEJ.0b013e3283552da8.
- Ding N, Shah AM, Blaha MJ, et al. Cigarette smoking, cessation, and risk of heart failure with preserved and reduced ejection fraction. J Am Coll Cardiol. 2022;79(23):2298–2305. doi: 10.1016/j.jacc.2022.03.377.
- Karlson EW, Chang SC, Cui J, et al. Gene-environment interaction between HLA-DRB1 shared epitope and heavy cigarette smoking in predicting incident rheumatoid arthritis. Ann Rheum Dis. 2010;69(1):54–60. doi: 10.1136/ard.2008.102962.
- Barbers RG, Gong H, Jr., Tashkin DP, et al. Differential examination of bronchoalveolar lavage cells in tobacco cigarette and marijuana smokers. Am Rev Respir Dis. 1987;135(6):1271–1275. doi: 10.1164/arrd.1987.135.6.1271.
- Watson NF, Badr MS, Belenky G, et al. Joint consensus statement of the American academy of sleep medicine and sleep research society on the recommended amount of sleep for a healthy adult: methodology and discussion. J Clin Sleep Med. 2015;11(8):931–952. doi: 10.5664/jcsm.4950.
- Chinese Nutrition Society. The chinese dietary guidelines. Beijing: people’s Medical Publishing House. 2022.
- Haskell WL, Lee IM, Pate RR, et al. Physical activity and public health: updated recommendation for adults from the American college of sports medicine and the American heart association. Med Sci Sports Exerc. 2007;39(8):1423–1434. doi: 10.1249/mss.0b013e3180616b27.
- Zung WW. A self-rating depression scale. Arch Gen Psychiatry. 1965;12(1):63–70. doi: 10.1001/archpsyc.1965.01720310065008.
- Zung WW. A rating instrument for anxiety disorders. Psychosomatics. 1971;12(6):371–379. doi: 10.1016/s0033-3182(71)71479-0.
- Büttner-Teleagă A, Kim YT, Osel T, et al. Sleep disorders in cancer-a systematic review. Int J Environ Res Public Health. 2021;18(21):11696. doi: 10.3390/ijerph182111696.
- Surani S, Brito V, Surani A, et al. Effect of diabetes mellitus on sleep quality. World J Diabetes. 2015;6(6):868–873. doi: 10.4239/wjd.v6.i6.868.
- Sharafkhaneh A, Jayaraman G, Kaleekal T, et al. Sleep disorders and their management in patients with COPD. Ther Adv Respir Dis. 2009;3(6):309–318. doi: 10.1177/1753465809352198.
- Kavanagh J, Jackson DJ, Kent BD. Sleep and asthma. Curr Opin Pulm Med. 2018;24(6):569–573. doi: 10.1097/mcp.0000000000000526.
- Malhotra A, Loscalzo J. Sleep and cardiovascular disease: an overview. Prog Cardiovasc Dis. 2009;51(4):279–284. doi: 10.1016/j.pcad.2008.10.004.
- Van Buuren S, Groothuis-Oudshoorn K. MICE: multivariate imputation by chained equations in R. J Stat Soft. 2011;45(3):1–67. doi: 10.18637/jss.v045.i03.
- Epskamp S, Cramer AOJ, Waldorp LJ, et al. Qgraph: Network visualizations of relationships in psychometric data. J Stat Soft. 2012;48(4):1–18. doi: 10.18637/jss.v048.i04.
- Epskamp S, Fried EI. A tutorial on regularized partial correlation networks. Psychol Methods. 2018;23(4):617–634. doi: 10.1037/met0000167.
- Fruchterman TMJ, Reingold EM. Graph drawing by force-directed placement. Softw Pract Exp. 1991;21(11):1129–1164. doi: 10.1002/spe.4380211102.
- Borgatti SP. Centrality and network flow. Social Networks. 2005;27(1):55–71. doi: 10.1016/j.socnet.2004.11.008.
- Opsahl T, Agneessens F, Skvoretz J. Node centrality in weighted networks: generalizing degree and shortest paths. Social Networks. 2010;32(3):245–251. doi: 10.1016/j.socnet.2010.03.006.
- Hevey D. Network analysis: a brief overview and tutorial. Health Psychol Behav Med. 2018;6(1):301–328. doi: 10.1080/21642850.2018.1521283.
- Mehari A, Weir NA, Gillum RF. Gender and the association of smoking with sleep quantity and quality in American adults. Women Health. 2014;54(1):1–14. doi: 10.1080/03630242.2013.858097.
- Lavoie CJ, Zeidler MR, Martin JL. Sleep and aging. Sleep Sci Pract. 2018;2:1–8. doi: 10.1186/s41606-018-0021-3.
- Duffy JF, Willson HJ, Wang W, et al. Healthy older adults better tolerate sleep deprivation than young adults. J Am Geriatr Soc. 2009;57(7):1245–1251. doi: 10.1111/j.1532-5415.2009.02303.x.
- Furihata R, Kaneita Y, Jike M, et al. Napping and associated factors: a Japanese nationwide general population survey. Sleep Med. 2016;20:72–79. doi: 10.1016/j.sleep.2015.12.006.
- Metse AP, Clinton-McHarg T, Skinner E, et al. Associations between suboptimal sleep and smoking, poor nutrition, harmful alcohol consumption and inadequate physical activity (‘SNAP risks’): a comparison of people with and without a mental health condition in an Australian community survey. Int J Environ Res Public Health. 2021;18(11):5946. doi: 10.3390/ijerph18115946.
- Oftedal S, Kolt GS, Holliday EG, et al. Associations of health-behavior patterns, mental health and self-rated health. Prev Med. 2019;118:295–303. doi: 10.1016/j.ypmed.2018.11.017.
- Broms U, Pennanen M, Patja K, et al. Diurnal evening type is associated with current smoking, nicotine dependence and nicotine intake in the population based national FINRISK 2007 study. J Addict Res Ther. 2012;S2:002. doi: 10.4172/2155-6105.s2-002.
- Ishihara K, Miyasita A, Inugami M, et al. Differences in the time or frequency of meals, alcohol and caffeine ingestion, and smoking found between ‘morning’ and ‘evening’ types. Psychol Rep. 1985;57(2):391–396. doi: 10.2466/pr0.1985.57.2.391.
- Urbán R, Magyaródi T, Rigó A. Morningness-eveningness, chronotypes and health-impairing behaviors in adolescents. Chronobiol Int. 2011;28(3):238–247. doi: 10.3109/07420528.2010.549599.
- Randler C. Differences between smokers and nonsmokers in morningness-eveningness. Soc Behav Pers. 2008;36(5):673–680. doi: 10.2224/sbp.2008.36.5.673.
- Gehricke JG, Loughlin SE, Whalen CK, et al. Smoking to self-medicate attentional and emotional dysfunctions. Nicotine Tob Res. 2007;9 Suppl 4(Suppl 4):S523–S536. doi: 10.1080/14622200701685039.
- Hamidovic A, de Wit H. Sleep deprivation increases cigarette smoking. Pharmacol Biochem Behav. 2009;93(3):263–269. doi: 10.1016/j.pbb.2008.12.005.
- Perogamvros L, Schwartz S. The roles of the reward system in sleep and dreaming. Neurosci Biobehav Rev. 2012;36(8):1934–1951. doi: 10.1016/j.neubiorev.2012.05.010.
- Volkow ND, Tomasi D, Wang GJ, et al. Evidence that sleep deprivation downregulates dopamine D2R in ventral striatum in the human brain. J Neurosci. 2012;32(19):6711–6717. doi: 10.1523/jneurosci.0045-12.2012.
- Connor G,S, Schofield-Hurwitz S, Hardt J, et al. The accuracy of self-reported smoking: a systematic review of the relationship between self-reported and cotinine-assessed smoking status. Nicotine Tob Res. 2009;11(1):12–24. doi: 10.1093/ntr/ntn010.
- Chinese Center for Disease Control and Prevention. Global Adult Tobacco Survey 2018. Accessed 30 Mar, 2023. https://extranet.who.int/ncdsmicrodata/index.php/catalog/803/related-materials.