Abstract
Observational studies have contributed in a major way to understanding modifiable determinants of cardiovascular disease risk, but several examples exist of factors that were identified in observational studies as potentially protecting against coronary heart disease, that in randomized controlled trials had no such effect. The likely reason for misleading findings from observational epidemiological studies is that associations are influenced by confounding, bias, and reverse causation—where disease influences a risk factor, rather than vice versa. Mendelian randomization utilizes genetic variants that serve as proxy measures for modifiable risk factors to allow estimation of the causal influence of the modifiable risk factor in question. We present examples of the use of the Mendelian randomization approach and discuss both the limitations and potentials of this strategy.
Introduction: Limits of observational epidemiological studies of cardiovascular disease
Observational epidemiology has had a long and distinguished history of causal discovery—smoking and lung cancer, asbestos and mesothelioma, blood pressure and stroke, cholesterol and coronary heart disease, to mention a few. Despite these successes, debate continues on the credibility of observational studies Citation1, Citation2. While better approaches to control for confounding and selection bias have begun to be explored in observational approaches to evaluate treatment effects Citation3, causal observational epidemiology has lagged behind. Consequently, to investigators interested in cardiovascular disease (CVD) and the consequences of a modifiable environmental exposure—say, a particular aspect of diet—the obvious approach would be to directly study dietary intake and how this relates to the risk of CVD. Why, then, should an alternative approach be advanced? The impetus for thinking of new approaches is that conventional observational study designs have yielded findings that have failed to be confirmed by randomized controlled trials Citation4.
Observational studies suggesting that beta carotene Citation5, vitamin E supplements Citation6, Citation7, vitamin C supplements Citation8, and hormone replacement therapy (HRT) Citation9 were cardioprotective were followed by large trials showing no such protection Citation10–14. In each case special pleading was advanced to explain the discrepancy—were the doses of vitamins given in the trials too high or too low to be comparable with the observational studies? Did HRT use start too late in the trials? Were differences explained by duration of follow-up or other design aspects? Were interactions with other factors such as smoking or alcohol consumption key? Rather than such particular explanations being true (with the happy consequence that both the observational studies and the trials had got the right answers, but to different questions) it is likely that a general problem of confounding—by life-style and socio-economic factors, or by base-line health status and prescription policies—was responsible. Indeed in the example of vitamin E supplements, the observational studies and the trials tested precisely the same thing. presents the findings from observational studies of taking vitamin E supplements Citation6, Citation7 and a meta-analysis of trials of supplements Citation15. The point here is that in the observational studies specifically investigating the effect of taking supplements, an apparent large protective effect—about a halving of risk—was seen even when taken only for a short period (2–4 years), as well as when taken for longer (5–9 years and 10 years or more), even after adjustment for confounders. The trials tested randomization to essentially the same supplements for the same length of time, and found no protective effect. Importantly, the trial findings cannot be attributed to confounding or self-selection of healthier people into a vitamin-taking group, as taking or not taking vitamin E was determined randomly, which, providing it is done properly, avoids these sources of bias.
Key message
Genetic variants can serve as instrumental variables for estimating the causal effects of modifiable risk factors in cardiovascular epidemiology.
Figure 1. A: Vitamin E supplement use and risk of CHD in two observational studies Citation6, Citation7 and in a meta-analysis of RCTs Citation15. B: Observed effect of duration of vitamin E use compared to no use on CHD events in the Health Professional Follow-up Study Citation6. RR = relative risk.

Circulating vitamin C levels are inversely associated with incident coronary heart disease Citation16. Summary data are shown in which displays the relative risk of coronary heart disease (CHD) for 15.7 µmol/L higher plasma vitamin C level, assuming a log-linear association. As can be seen, adjustment for confounders had little impact on this association. However, a large-scale randomized controlled trial, the Heart Protection Study, examined the effect of a supplement that increased average plasma vitamin C levels by 15.7 µmol/L. In this study randomization to the supplement was associated with no decrement in coronary heart disease risk Citation13.
Figure 2. Estimates of the effects of an increase of 15.7 µmol/L plasma vitamin C on CHD 5-year mortality estimated from observational epidemiological EPIC Citation16 and randomized controlled Heart Protection Study Citation13. (EPIC m = men, age-adjusted; EPIC m* = men, adjusted for systolic blood pressure, cholesterol, body mass index (BMI), smoking, diabetes, and vitamin supplement use; EPIC w = women, age-adjusted; EPIC w* = women, adjusted for systolic blood pressure, cholesterol, BMI, smoking, diabetes, and vitamin supplement use).

What underlies the discrepancy between these findings? One possibility is that there is considerable confounding between vitamin C levels and other exposures that could increase the risk of coronary heart disease. A demonstration of such effects can be seen in the British Women's Heart and Health Study (BWHHS). In this study, women with higher plasma vitamin C levels were found to be less likely to be in a manual social class, have no car access, be a smoker, or be obese, and more likely to exercise, be on a low-fat diet, have a daily alcoholic drink, and be tall Citation17. Furthermore for these women in their 60s and 70s those with higher plasma vitamin C levels were less likely to have come from a home 50 years or more previously in which their father was in a manual job, or had no bathroom or hot water, or within which they had to share a bedroom. They were also less likely to have limited educational attainment. In short, a substantial amount of confounding by factors from across the life-course that predict elevated risk of coronary heart disease was seen Citation17.
Moreover, in the BWHHS a 15.7 µmol higher plasma vitamin C level was associated with a relative risk of incident coronary heart disease of 0.88 (95% confidence interval (CI) 0.80–0.97), in the same direction as the estimates seen in the observational study summarized in . Adjustment for the same confounders as were used in the observational study reported in changed the estimate very little—to 0.90 (95% CI 0.82–0.99). When additional adjustment was made for confounders acting across the life-course, considerable attenuation was seen, with a residual relative risk of 0.95 (95% CI 0.85–1.05) Citation18. It is obvious that given inevitable amounts of measurement imprecision in the confounders, or a limited number of missing unmeasured confounders, the residual association is essentially null and close to the finding of the randomized controlled trial. Most studies have more limited information on potential confounders than is available in the BWHHS, and in other fields we may be even more ignorant of the confounding factors we should measure. In these cases inferences drawn from observational epidemiological studies can be seriously misleading. As the major and compelling rationale for doing these observational studies is to underpin public health prevention strategies, their repeated failures are a major concern for public health policy makers, researchers, and funding bodies.
Other processes in addition to confounding can generate robust, but non-causal, associations in observational studies. Reverse causation—where the disease influences the apparent exposure, rather than vice versa—may generate strong and replicable associations. For example, many studies have found that people with low circulating cholesterol levels are at increased risk of several cancers, including colon cancer. If causal, this is an important association as it might mean that efforts to lower cholesterol levels would increase the risk of cancer. However, it is possible that the early stages of cancer may, many years before diagnosis or death, lead to a lowering in cholesterol levels, rather than low cholesterol levels increasing the risk of cancer. Similarly in studies of inflammatory markers such as C-reactive protein and cardiovascular disease risk it is possible that early stages of atherosclerosis—which is an inflammatory processes—lead to elevation in circulating inflammatory markers, and since people with atherosclerosis are more likely to experience cardiovascular events a robust, but non-causal, association between levels of inflammatory markers and incident cardiovascular disease is generated. Reverse causation can also occur through behavioural processes—for example, people with early stages and symptoms of cardiovascular disease may reduce their consumption of alcohol, which would generate a situation in which alcohol intake appears to protect against cardiovascular disease. A form of reverse causation can also occur through reporting bias, with the presence of disease influencing reporting disposition. In case-control studies people with the disease under investigation may report on their prior exposure history in a different way than do controls—perhaps because the former will think harder about potential reasons that account for why they have developed the disease.
The problems of confounding and reverse causation discussed above relate to the production of associations in observational studies that are not reliable indicators of the true direction of causal associations. A separate issue is that the strength of associations between causal risk factors and disease in observational studies will generally be under-estimated due to random measurement imprecision in indexing the exposure. A century ago Charles Spearman demonstrated mathematically how such measurement imprecision would lead to what he termed the ‘attenuation by errors’ of associations Citation19, Citation20. This has more latterly been renamed ‘regression dilution bias’ Citation21.
Observational studies can and do produce findings that either spuriously enhance or downgrade estimates of causal associations between modifiable exposures and disease. This has serious consequences for the appropriateness of interventions that aim to reduce disease risk in populations. It is for these reasons that alternative approaches—including those within the Mendelian randomization framework—need to be applied.
Mendelian randomization
The basic principle utilized in the Mendelian randomization approach is that if genetic variants either alter the level of, or mirror the biological effects of, an modifiable environmentally exposure that itself alters disease risk, then these genetic variants should be related to disease risk to the extent predicted by their influence on exposure to the risk factor. Common genetic polymorphisms that have a well characterized biological function (or are proxies for such variants) can therefore be utilized to study the effect of a suspected environmental exposure on disease risk Citation4, Citation22–25. The exploitation of situations in which genotypic differences produce effects similar to environmental factors (and vice versa) clearly resonates with the concepts of phenocopy and genocopy in developmental genetics. The term phenocopy is attributed to Goldschmidt Citation26 and describes the situation where an environmental effect produces the same effect as a genetic mutation. For example the niacin-deficiency disease pellagra is clinically similar to the autosomal recessive condition Hartnup disease Citation27, and pellagra has been referred to as a phenocopy of the genetic disorder Citation28, Citation29. Genocopy is a less widely utilized term, attributed to Schmalhausen (cited by Gause) Citation30 and has generally been considered to be the reverse of phenocopy—i.e. when genetic variation generates an outcome that could be produced by an environmental stimulus Citation31. The two concepts are mirror images reflecting differently motivated accounts of how both genetic and environmental factors influence physical state. For example, Hartnup disease can be called a genocopy of pellagra, while pellagra can be considered a phenocopy of Hartnup disease. Mendelian randomization can, therefore, be viewed as an appreciation of the phenocopy-genocopy nexus that allows causation to be separated from association.
It may seem illogical to study genetic variants as surrogate measures for environmental exposures rather than measure the exposures themselves. However, there are several crucial advantages of utilizing functional genetic variants (or their markers) in this manner, that relate to the problems with observational studies outlined above. First, unlike environmental exposures, genetic variants are not generally associated with the wide range of behavioural, social, and physiological factors that, for example, confound the association between vitamin C and coronary heart disease. This means that if a genetic variant is used to act as a marker of an environmentally modifiable exposure, it is unlikely to be confounded in the way that direct measures of the exposure will be.
Further, aside from the effects of population structure Citation32, such variants will not be associated with other genetic variants, excepting those with which they are in linkage disequilibrium. This latter assumption follows from a contemporary appreciation of the law of independent assortment (sometimes referred to as Mendel's second law, hence the term ‘Mendelian randomization’). This powerful aspect of Mendelian randomization can be seen in A, showing the strong associations between a range of variables and blood fibrinogen levels but no association of the same factors (with the exception of apolipoprotein B/A1 ratio) with genetic variants in the beta-fibrinogen 148C/T genotype Citation33. As expected, genotype is related to plasma fibrinogen levels but not to other risk factors or diseases. The association of genotype with apolipoprotein B/A2 ratio was not expected and may simply reflect the play of chance due to multiple comparisons made, or may represent a pleiotropic effect of the beta-fibrinogen genotype. This example demonstrates the importance of checking that the Mendelian randomization has resulted in removing the effects of measured confounders—in this case it may not have fully done this, potentially limiting interpretation of the findings.
Table I. A: Means or proportions of various risk factors and potential confounders by quintiles of plasma fibrinogen n=2,932 (from Keavney et al. 2006) Citation33.
Table I. B: Means or proportions of various risk factors and potential confounders by beta-fibrinogen -148C/T genotype.
Second, we have seen how inferences drawn from observational studies may be subject to bias due to reverse causation. Disease processes may influence exposure levels such as alcohol intake, or measures of intermediate phenotypes such as cholesterol levels and plasma fibrinogen. However germ-line genetic variants associated with average alcohol intake or circulating levels of intermediate phenotypes will not be influenced by the onset of disease. This will be equally true with respect to reporting bias generated by knowledge of disease status in case-control studies, or of differential reporting bias in any study design.
Third, associative selection bias, in which selection into a study is related to both exposure level and disease risk and can generate spurious associations, is unlikely to occur with respect to genetic variants. For example, empirical evidence supports a lack of association between a wide range of genetic variants and participation rates in three separate case-control studies: breast cancer, non-Hodgkin's lymphoma, and lung cancer Citation34. As these investigators noted, it is important that researchers test this assumption in their own data, as it is possible that other genotypes than those tested in this study, particularly those associated with health-relevant behaviours (e.g. alcohol consumption), may show associations.
Finally, in most instances, a genetic variant will indicate long-term levels of exposure, and if the variant is taken as a proxy for such exposure it will not suffer from the measurement error inherent in phenotypes that have high levels of variability. For example, groups defined by cholesterol level-related genotype will, over a long period, experience the cholesterol difference seen between the groups. For individuals, blood cholesterol is variable over time, and the use of single measures of cholesterol will under-estimate the true strength of association between cholesterol and, say, coronary heart disease. Indeed use of the Mendelian randomization approach predicts a strength of association that is in line with randomized controlled trial findings of effects of cholesterol-lowering when the increasing benefits seen over the relatively short trial period are projected to the expectation for differences over a lifetime Citation22, as discussed further below.
Categories of Mendelian randomization
There are several categories of inference that can be drawn from studies utilizing the Mendelian randomization paradigm. In the most direct forms genetic variants can be related to the probability or level of exposure (‘exposure propensity’) or to intermediate phenotypes believed to influence disease risk. Less direct evidence can come from genetic variant-disease associations that indicate that a particular biological pathway may be of importance, perhaps because the variants modify the effects of environmental exposures. Several examples from of these categories have been given elsewhere Citation4, Citation22, Citation24; here a few illustrative cases are briefly outlined.
Exposure propensity
Alcohol intake and cardiovascular disease
The possible protective effect of moderate alcohol consumption on CHD risk remains controversial Citation35–37. Non-drinkers may be at a higher risk of CHD because health problems (perhaps induced by previous alcohol abuse) dissuade them from drinking Citation38. As well as this form of reverse causation, confounding could play a role, with non-drinkers being more likely to display an adverse profile of socio-economic or other behavioural risk factors for CHD Citation39. Alternatively, alcohol may have a direct biological effect that lessens the risk of CHD—for example, by increasing the levels of protective high-density lipoprotein (HDL) cholesterol Citation40. It is, however, unlikely that a randomized controlled trial (RCT) of alcohol intake, able to test whether there is a protective effect of alcohol on CHD events, will be carried out.
Alcohol is oxidized to acetaldehyde, which in turn is oxidized by aldehyde dehydrogenases (ALDHs) to acetate. Half of Japanese people are heterozygotes or homozygotes for a null variant of ALDH2 and peak blood acetaldehyde concentrations post alcohol challenge are 18 times and 5 times higher, respectively, among homozygous null variant and heterozygous individuals compared with homozygous wild-type individuals Citation41. This renders the consumption of alcohol unpleasant through inducing facial flushing, palpitations, drowsiness, and other symptoms. As A shows, there are very considerable differences in alcohol consumption according to genotype Citation42. The principles of Mendelian randomization are seen to apply—two factors that would be expected to be associated with alcohol consumption, age and cigarette smoking, which would confound conventional observational associations between alcohol and disease, are not related to genotype despite the strong association of genotype with alcohol consumption (B).
Figure 3. A: Relationship between alcohol intake and ALDH2 genotype. B: Relationship between characteristics and ALDH2 genotype. C: Relationship between HDL cholesterol and ALDH2 genotype (data from Tagaki et al., 2002 Citation42).

It would be expected that ALDH2 genotype influences diseases known to be related to alcohol consumption, and as proof of principle it has been shown that ALDH2 null variant homozygosity—associated with low alcohol consumption—is indeed related to a lower risk of liver cirrhosis Citation43. Considerable evidence, including data from randomized controlled trials, suggests that alcohol increases HDL cholesterol levels Citation44, Citation45 (which should protect against CHD). In line with this, ALDH2 genotype is strongly associated with HDL cholesterol in the expected direction (C). Given the apparent protective effect of alcohol against CHD risk seen in observational studies, possession of the ALDH2 allele—associated with lower alcohol consumption—should be associated with a greater risk of myocardial infarction, and this is what was seen in a case-control study Citation42. Men either homozygous or heterozygous for null ALDH2 were at twice the risk of myocardial infarction. In support of reasoning that the HDL cholesterol-elevating effects of alcohol are what renders it protective against coronary heart disease, statistical adjustment for HDL cholesterol greatly attenuated the association between ALDH2 genotype and CHD. Counterbalancing any protective effect of alcohol on CHD risk mediated through higher HDL cholesterol is an adverse effect of alcohol intake on blood pressure. This has been demonstrated through showing that ALDH2 genotype is associated with blood pressure to the extent predicted by the joint effects of genotype on alcohol intake and alcohol intake on blood pressure Citation45.
Intermediate phenotypes
Genetic variants can influence circulating biochemical factors such as cholesterol, homocysteine, or fibrinogen levels. This provides a method for assessing causality in associations observed between these measures (intermediate phenotypes) and disease, and thus whether interventions to modify the intermediate phenotype could be expected to influence disease risk.
Cholesterol and coronary heart disease
Familial hypercholesterolaemia is a dominantly inherited condition in which many rare mutations (over 700 DNA sequence variations Citation46 of the low-density lipoprotein receptor gene, about 10 million people affected world-wide, a prevalence of around 0.2%), lead to high circulating cholesterol levels Citation47. The high risk of premature CHD in people with this condition was readily appreciated, with an early UK report demonstrating that by age 50 half of men and 12% of women had suffered from CHD Citation48. Compared with the population of England and Wales (mean total cholesterol 6.0 mmol/L), people with familial hypercholesterolaemia (mean total cholesterol 9 mmol/L) suffered a 3.9-fold increased risk of CHD mortality, although very high relative risks among those aged less than 40 years have been observed Citation49. These observations, regarding genetically determined variation in risk, provide strong evidence that the associations between blood cholesterol and CHD seen in general populations reflecte a causal relationship. The causal nature of the association between blood cholesterol levels and coronary heart disease has historically been controversial Citation50, Citation51. As both Daniel Steinberg Citation52 and Ole Færgeman discuss Citation53, many clinicians and public health practitioners rejected the notion of a causal link for a range of reasons. However, from the late 1930s onwards evidence that people with genetically high levels of cholesterol had high risk for coronary heart disease should have been powerful and convincing evidence of the causal influence of elevated blood cholesterol in the general population.
The efficacy of cholesterol-lowering treatment with statins has removed any residual doubt that that the cholesterol-CHD relationship is causal. Among people without CHD, reducing total cholesterol levels with statins by around 1–1.5 mmol/L reduces CHD mortality by around 25% over 5 years Citation54. People with familial hypercholesterolaemia have an almost 4-fold increased risk of CHD compared with the general population, whereas extrapolating the randomized trial evidence to their cholesterol levels would suggest that only a 2-fold increase should be expected. People with familial hypercholesterolaemia will have had high total cholesterol levels throughout their lives, and this would be expected to generate a greater risk than that predicted by the results of lowering cholesterol levels for only 5 years. Furthermore, ecological studies relating cholesterol levels to CHD demonstrate that the strength of association increases as the lag period between cholesterol level assessment and CHD mortality increases Citation55, again suggesting that long-term differences in cholesterol level are the important aetiological factor in CHD Citation22.
More recently, mutations in the gene coding for apolipoprotein B (apoB) have been found to produce a syndrome phenotypically indistinguishable from familial hypercholesterolaemia—familial defective apoB Citation56–58. In a recent study of the Arg3500Gln mutation of the APOB gene, the basic principle behind Mendelian randomization can be demonstrated, in that Arg3500Gln heterozygotes had higher levels of total cholesterol but other CHD risk factors (including triglycerides, fibrinogen, glucose, body mass index, and waist-hip ratio) did not differ from non-heterozygotes in the general population Citation59. The Arg3500Gln heterozygotes had a median 2.6 mmol/L higher blood cholesterol level and a high (but imprecise) odds ratio for CHD of 7.0 (95% CI 2.2–22) compared with the general population. As in the case of familial hypercholesterolaemia this is greater than that predicted by the randomized controlled trial data, but again the differences in cholesterol by genotype will have been life-long, and the elevated CHD risk probably reflects the effects of long-term differences in cholesterol level (22).
Genetic variants in PCSK9 are associated with levels of low-density lipoprotein (LDL) cholesterol between 15%–23% lower than levels in people without the mutant variants in the Atherosclerosis Risk in Communities Study (ARIC), and considerably lower risks of CHD—between 47% and 88% lower—have been observed, depending on the level of LDL cholesterol associated with each sequence variant Citation60. Despite participants in ARIC having substantial burdens of other cardiovascular risk factors, these data indicate that life-long exposure to low levels of LDL cholesterol (consistent with those achieved by statin treatment) is associated with markedly reduced risks of CHD, greater than the reductions observed for short-term cholesterol-lowering in the statin trials. As other commentators have observed this is not surprising as atherosclerosis begins early in life, whereas statin treatment in later life would not be expected to achieve the same benefit Citation61.
C-reactive protein (CRP) and coronary heart disease
Strong associations of C-reactive protein (CRP), an acute phase inflammatory marker, with hypertension, insulin resistance, and coronary heart disease have been repeatedly observed Citation62–68, with the obvious inference that CRP is a cause of these conditions Citation69–71. These apparently convincing observational analyses have been accompanied by meta-analyses of the relationship between circulating CRP concentrations and CHD risk which have also reported the consistent relationship between this biomarker and disease risk Citation72, Citation73. However, a growing body of evidence has emerged suggesting that this relationship is artefactual as opposed to causal. As sample sizes have grown, effect sizes have fallen, from the region of 4 times elevated odds of disease for CHD, for the top to bottom quartile of the CRP distribution Citation74, to recent reports presenting an odds ratio for CHD risk from top to bottom tertile of the CRP distribution of around 1.4 Citation42. Furthermore, evidence as to the potential mechanistic links between CRP elevation and CVD risk has been increasingly shown to be inconsistent Citation75, Citation76. Thus, whilst evidence as to the potential modulating effects of CRP after acute coronary events may be available Citation77, that concerning the causal role of chronic elevations of CRP on end-point CVD risk is unclear.
Given the availability of a series of genetic markers surrounding the CRP locus which are consistently, and apparently simply, related to long-term differences in the circulating levels of CRP, Mendelian randomization is a suitable approach for establishing causality in this framework. A simple form of such a study has examined polymorphisms of the CRP gene and demonstrated that while serum CRP differences were highly predictive of blood pressure and hypertension, the CRP variants—which are related to sizeable serum CRP differences—were not associated with these same outcomes Citation78. In the light of these data it was suggested that the original observational findings may be explained by the extensive confounding between serum CRP and outcomes, or by the existence of reverse causation, in which existing hypertension leads to elevation of CRP levels. Indeed the findings from the Mendelian randomization approach, of a best-estimate of no causal effect of CRP on hypertension, is similar to the observational association once full adjustment for life-course confounding factors has taken place ().
Figure 4. Triangulation with observational data: odds ratio for hypertension for a doubling in C-reactive protein, with and without full adjustment (from Davey Smith et al., 2005, 78).
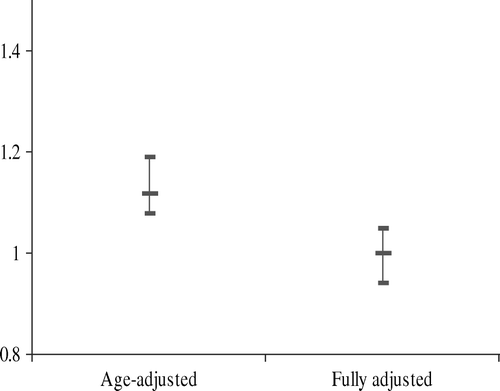
Current evidence on this issue also suggests that CRP levels do not lead to elevated risk of insulin resistance Citation79, or coronary heart disease, or extent of atherosclerosis indexed by measures of intima media thickness Citation80. Confounding and reverse causation—where existing coronary disease or insulin resistance or increased adiposity may influence CRP levels—could account for this discrepancy. The studies to date of this issue are, however, statistically underpowered, and there are relatively wide confidence intervals around the null causal effect estimates. Similar findings have been reported for serum fibrinogen, variants in the beta-fibrinogen gene and CHD Citation33, Citation81. The CRP and fibrinogen examples demonstrate that Mendelian randomization can both increase evidence for a causal effect of an environmentally modifiable factor (as in the cases of alcohol and cholesterol levels discussed earlier) and also provide evidence against causal effects, that can help direct efforts away from targets of no preventative or therapeutic relevance.
Progress in explicating the role of intermediate phenotypes through Mendelian randomization
The expanding scope of cardiovascular disease epidemiology is leading to challenges that cannot be successfully tackled by conventional approaches. Cardiovascular epidemiologists are increasingly investigating circulating factors that have complex sets of interrelationships—e.g. interleukins, tumour necrosis factors, lipoprotein metabolism components, insulin and glucose homeostasis markers, coagulation factors and hormones, and adipokines and cytokines generally. Literally hundreds of molecules are under active consideration as possible components of pathways influencing cardiovascular disease risk and therefore as potential targets for therapeutic manipulation. These factors have myriad sets of associations: indeed it is probably more unusual to find pairs of factors that are not related than ones that are related.
Understanding the causal relationships is key to identifying potential points of intervention, but observational approaches cannot deal well with the strongly intercorrelated networks of factors that are measured with varying degrees of error and vary over time to a greater or lesser degree. Animal studies and human interventional studies can help here but have their obvious limitations. As genetic variants that are associated with different on-average levels of these factors are uncovered, however, they allow for node-by-node investigation of direction of effect of each factor on the others, and ultimately the structure of the networks may be elucidated Citation82. Through analysing the broad phenotypic effects of variants associated with natural perturbations at each node both the beneficial and potentially detrimental effects of therapeutic modification can be estimated.
Broad mechanisms of causation
The role of chronic infection in cardiovascular disease has been a subject of considerable interest. For example, Chlamydia pneumoniae was thought to be a potentially causal agent in the aetiology of cardiovascular disease, but meta-analysis of all the data showed disappointingly little evidence of association Citation83. Similarly, chronic hepatitis B infection seems to be associated with increased risk of cardiovascular disease Citation84. Moreover, the disappointing results from trials of long-term antibiotics in secondary prevention of coronary heart disease Citation85 suggest that our understanding of the role of infection in CHD aetiology is insufficient. However, evidence that acute infection is involved in triggering vascular events is more compelling Citation86, and the increased risk of cardiovascular disease in people with auto-immune diseases makes exploration of general roles of infection and immunity in the aetiology of cardiovascular disease of continued interest.
It has been suggested that genetic variants in the innate immune response system that modify risk of infection and inflammatory responsiveness Citation87 may be used to explore the role of infection in the aetiology of cardiovascular disease Citation88. Briefly, this would be done by using a Mendelian randomization design; first checking that confounders such as smoking and socio-economic position were similar between those difference alleles of the relevant genetic variant, second demonstrating the expected associations with response to acute infections (if feasible) of different risk alleles, and then comparing the rates of cardiovascular disease between those with treatment or alleles. If cardiovascular disease was found to be more common in those possessing risk alleles this would provide evidence that either reducing infection load or pursuing biological pathways influenced by these genetic variants would be worthwhile as a means of identifying targets for prevention. If genetic variants related to inflammatory response are found to be related to CVD risk then this would indicate that infections—by known or as yet unidentified infectious agents—that activate this response could increase cardiovascular risk.
Implications of Mendelian randomization study findings
Establishing the causal influence of environmentally modifiable risk factors from Mendelian randomization designs informs policies for improving population health through population level interventions. They do not imply that the appropriate strategy is genetic screening to identify those at high risk and application of selective exposure reduction policies. For example, establishing the association between genetic variants (such as familial defective apoB) associated with elevated cholesterol level and CHD risk strengthens causal evidence that elevated cholesterol is a modifiable risk factor for CHD for the whole population. Thus even though the population-attributable risk for CHD of this variant is small, it usefully informs public health approaches to improving population health. It is this aspect of Mendelian randomization that illustrates its distinction from conventional risk identification and genetic screening purposes of genetic epidemiology.
The determination of observational associations for which appropriate resource allocation for an RCT is justified represents another important application of the paradigm of Mendelian randomization. Mendelian randomization suffers from several limitations (which will be discussed in more detail) and cannot provide definitive answers as to the nature of observational relationships. However, this approach has the ability to cast a considerable opinion as to the likely existence of causal relationships and hence which pathways may benefit from extensive follow-up analyses. Such a method would prove an important contribution to the weight of evidence suggesting which biological pathways or environmentally modifiable disease risk factors merit examination using RCTs.
There are similarities in the logical structure of RCTs and Mendelian randomization studies. illustrates this, drawing attention to the unconfounded nature of exposures proxied for by genetic variants (analogous to the unconfounded nature of a randomized intervention), the lack of possibility of reverse causation as an influence on exposure-outcome associations in both Mendelian randomization and randomized controlled trial settings, and the importance of intention to treat analyses—i.e. analysis by group defined by genetic variant, irrespective of associations between the genetic variant and the proxied for exposure within any particular individual.
Figure 5. Mendelian randomization and randomized controlled trial designs compared Citation23.

The analogy with randomized controlled trials is also useful with respect to one objection that has been raised in relation to Mendelian randomization studies. This is that the environmentally modifiable exposure (such as alcohol intake or circulating CRP levels) proxied by the genetic variant is influenced by many other factors in addition to the genetic variant Citation89. This is of course true. However, consider a randomized controlled trial of blood pressure-lowering medication. Blood pressure is mainly influenced by factors other than taking blood pressure-lowering medication—obesity, alcohol intake, salt consumption and other dietary factors, smoking, exercise, physical fitness, genetic factors, and early-life developmental influences are all of importance. However the randomization that occurs in trials ensures that these factors are balanced between the groups that receive the blood pressure-lowering medication and those that do not. Thus the fact that many other factors are related to the modifiable exposure does not vitiate the power of RCTs; neither does it vitiate the strength of Mendelian randomization designs.
Effect sizes and the application of instrumental variables approaches to the formal analysis of Mendelian randomization frameworks
A further common objection is that the genetic variants often explain only a trivial proportion of the variance in the environmentally modifiable risk factor that is being proxied for Citation90. Again consider a randomized controlled trial of blood pressure-lowering medication, where 50% of participants receive the medication and 50% receive a placebo. If the antihypertensive therapy reduced blood pressure by a quarter of a standard deviation, which is approximately the situation for such pharmacotherapy, then within the whole study group treatment assignment (i.e. antihypertensive use versus placebo) will explain about 1.5% of the variance in blood pressure. In the example of CRP haplotypes used as instruments for CRP levels Citation79, these haplotypes explain 1.66% of the variance in CRP levels in the population. As can be seen the quantitative association of genetic variants as instruments can be similar to that of randomized treatments with respect to biological processes that such treatments modify. Both logic and quantification fail to support criticisms of the Mendelian randomization approach based on either the obvious fact that many factors influence most phenotypes of interest or that particular genetic variants only account for a small proportion of variance in the phenotype.
The notion of genetic variation acting as an instrument for the re-assessment of observational relationships draws upon the ability of Mendelian randomization frameworks to be assessed formally through the application of instrumental variables methodology. In an instrumental variable approach the instrument is a variable that is only related to the outcome through its association with the modifiable exposure of interest. The instrument is not related to confounding factors, nor is its assessment biased in a manner that would generate a spurious association with the outcome. Furthermore the instrument will not be influenced by the development of the outcome (i.e. there will be no reverse causation). presents this basic schema, where the dotted line between genotype and the outcome provides an unconfounded and unbiased estimate of the causal association between the exposure that the genotype is acting as a proxy for and the outcome.
The development of instrumental variable methods within econometrics, in particular, has led to a sophisticated suite of statistical methods for estimating causal effects, and these have now been applied within Mendelian randomization studies (Citation45, Citation78, Citation79, Citation81. The parallels between Mendelian randomization and instrumental variable approaches are discussed in more detail elsewhere Citation91–93.
Limitations to the Mendelian randomization paradigm
Mendelian randomization employs the specific characteristics of genetic variation in efforts to circumvent the problems associated with the use of more conventional observational techniques. As it depends upon robust association between genotype and the modifiable exposure of interest, the method is susceptible to all of the problems that have led genetic association studies to generate non-reproducible findings (see ). These have been discussed at length elsewhere Citation94 and are probably becoming less important as the understanding of appropriate sample size in genetic association studies has improved. Furthermore the recent successes of genome-wide association studies Citation95, Citation96 demonstrate that very robust associations between genotype and many phenotypes will be revealed. Therefore in this section we only discuss problems with Mendelian randomization that arise after the establishment of a robust relationship between the genotype and the modifiable factor for which it is acting as a surrogate measure.
Table II. Reasons for inconsistent genotype-phenotype associations.
In removing the complications of naïve observational analyses, Mendelian randomization takes on a series of complications relating to the nature of this new analytical framework, as we have discussed at length in an earlier paper Citation4. These, principally, relate to the use of genetic variation as a proxy for the measurement of potentially modifiable risk and can be summarized under the six headings below.
Canalization
A potential problem for Mendelian randomization arises from the developmental compensation that may occur through a polymorphic genotype being expressed during fetal or early postnatal development and thus influencing development in such a way as to buffer against the effect of the polymorphism. Such compensatory processes have been discussed since C. H. Waddington introduced the notion of canalization in the 1940s Citation97. Canalization refers to the buffering of the effects of either environmental or genetic forces attempting to perturb development, and Waddington's ideas have been well developed both empirically and theoretically Citation98–105. Such buffering can be achieved either through genetic redundancy (more than one gene having the same or similar function) or through alternative metabolic routes, where the complexity of metabolic pathways allows recruitment of different pathways to reach the same phenotypic end-point. In effect a functional polymorphism expressed during fetal development or postnatal growth may influence the expression of a wide range of other genes, leading to changes that may compensate for the influence of the polymorphism. Put crudely, if a person has developed and grown from the intra-uterine period onwards within an environment in which one factor is perturbed (e.g. there is elevated CRP due to genotype) then they may be rendered resistant to the influence of lifelong elevated circulating CRP, through permanent changes in tissue structure and function that counterbalance its effects.
In intervention trials—for example, RCTs of cholesterol-lowering drugs—the intervention is generally randomized to participants during their middle age; similarly in observational studies of this issue, cholesterol levels are ascertained during adulthood. In Mendelian randomization, on the other hand, randomization occurs before birth. This leads to important caveats when attempting to relate the findings of conventional observational epidemiological studies to the findings of studies carried out within the Mendelian randomization paradigm.
In some Mendelian randomization designs developmental compensation is not an issue. For example, when maternal genotype is utilized as an indicator of the intra-uterine environment then the response of the fetus will not differ whether the effect is induced by maternal genotype or by environmental perturbation, and the effect on the fetus can be taken to indicate the effect of environmental influences during the intra-uterine period. Also in cases where a variant influences an adulthood environmental exposure—e.g. ALDH2 variation and alcohol intake—developmental compensation to genotype will not be an issue. In many cases of gene by environment interaction interpreted with respect to causality of the environmental factor Citation25 the same applies.
Acute disease predisposition
One limitation to the application of Mendelian randomization approaches is their difficulty in effectively modelling the presence or action of acute disease events or risk effects. The Mendelian randomization paradigm is well suited to the analysis of long-term alterations in the exposure to potentially modifiable risk factors, as for example in our discussion of long-term CRP levels and CHD risk. The approach cannot easily be applied to the study of whether short-term extreme differences in CRP level—such as those following a myocardial infarction—influence prognosis. Thus the findings of Mendelian randomization studies with respect to CRP do not address the potential beneficial effects of CRP inhibition following myocardial infarction, demonstrated in a recent animal study Citation77. To address the issue of whether a more marked CRP response following infarction has a detrimental effect on prognosis it would be necessary to identify variants reliably related to CRP response to myocardial infarction that can be studied in large numbers of myocardial infarction (MI) patients who are undergoing follow-up. While theoretically possible, this would be very difficult to achieve in practice.
Power
For Mendelian randomization approaches to contribute to the information that can already be obtained from available associations, adequate analytical power must be available for all elements of the Mendelian randomization triangle. This can prove to be a considerable limiting factor to the undertaking of such analyses. Rather than the requirement of power for a single association (possibly corrected for the undertaking of multiple tests), Mendelian randomization analyses require (even at their basic triangulation level) power to detect relationships between 1) intermediate phenotype/risk factor and outcome, 2) genotype/proxy marker and intermediate phenotype/risk factor, and 3) between genotype and outcome. If present, the existence of detectable associations in the three arms of the Mendelian randomization framework will allow for qualitative assessment of the causal effect of the intermediate phenotype. Moving to formal quantitative assessment within the Mendelian randomization framework for the assessment of a particular risk factor, all of these estimates are used in conjunction to assess the quantitative causal strength of the association. Within instrumental variable regression, all three individual relationships are incorporated, along with their respective degrees of error. Consequently the importance of statistical power is heightened Citation93.
Furthermore, whilst associations between quantiles of a given intermediate phenotype may yield test groups of equal and sufficient size, genotype frequencies do not conform to such specifications. Minor allele frequencies can be variable and lead to the presence of small exposure groups even within the bounds of relatively large cohorts. Furthermore, whilst the effect sizes of intermediate phenotypes on outcomes can be marked, the impact of genotypic variation is often small and of a composite nature (especially in the case of common, complex phenotypes). Therefore, with potentially small exposure groups (be they for the assessment of continuous variables or otherwise) and potentially small effect sizes, the imprecision in effect estimates derived from the instrumental variable regression analyses can be large.
The consequence of these factors for the application of Mendelian randomization is ultimately the widening of confidence intervals for the estimates of intermediate phenotype/risk factor causal effects when performing instrumental variable analyses. Thus, in ideal situations, one would study proxy marker candidates that exhibit marked genetic effects and genotypes that are common in the population under study. However, this is often not the case, and very large sample sizes are required to obtain robust estimates.
Heterogeneous effects: pleiotropy and linkage disequilibrium
Commonly termed pleiotropy, heterogeneity in this contest refers to the involvement of a particular gene in a series of biological pathways (or phenotypic effects). Ultimately, this implicates the presence of variation in the gene in question (or related to it) with effects in a variety of phenotypes rather than a specific pathology (perhaps through the action of transcription factor activation or alternative splicing effects). Involvement in a series of pathways is increasingly thought to be present for a wide range of genes and as such may be fundamental to the make-up of complex gene/phenotype relationships.
With reference to the successful application of Mendelian randomization, pleiotropy stands as a potential problem for analyses as a result of reintroduced confounding. Whilst there may be a valid association between intermediate phenotype/risk factor and the genotype chosen to be its proxy marker, the presence of associations between this proxy marker and other phenotypes could vitiate the use of this marker to assess the non-confounded and unbiased casual effect of the intermediate risk factor.
This situation may be derived conceptually from two sources. In the case of the classical interpretation of pleiotropy a single genetic variant may have multiple biological impacts. Consequently, whilst one relationship may appear to allow the application of the Mendelian randomization approach, the presence of a second (possibly counteracting) phenotypic effect would complicate this situation. Alternatively, linkage disequilibrium may reintroduce confounding through the genetic variant of interest being associated with another variant acting via a different phenotypic pathway, effectively presenting a situation analogous to that of classical pleiotropy.
Importantly, these derivations of apparent pleiotropy have a major difference. In classical pleiotropy, one is faced with an unsurpassable problem for the straightforward application of Mendelian randomization. However, in the case of linkage disequilibrium (LD)-derived heterogeneity of effect, one may theoretically avoid this problem through the exploitation of different population-based samples with differing population histories and hence LD structures.
Since pleiotropy and linkage disequilibrium can reintroduce confounding and vitiate the power of the Mendelian randomization approach it is important to consider ways of ameliorating this. Genomic knowledge may help in estimating the degree to which these are likely to be problems in any particular Mendelian randomization study, through, for instance, explication of genetic variants that may be in linkage disequilibrium with the variant under study, or the function of a particular variant and its known pleiotropic effects. Furthermore, genetic variation can be related to measures of potential confounding factors in each study, and the magnitude of such confounding estimated. Empirical studies to date suggest that common genetic variants are largely unrelated to the behavioural and socio-economic factors considered to be important confounders in conventional observational studies Citation106. However, relying on measurement of confounders does, of course, remove the central purpose of Mendelian randomization, which is to balance unmeasured as well as measured confounders (as randomization does in RCTs).
It may be possible to identify two separate genetic variants, that are not in linkage disequilibrium with each other, but which both serve as proxies for the environmentally modifiable risk factor of interest. If both variants are related to the outcome of interest and point to the same underlying association then it becomes much less plausible that reintroduced confounding explains the association, since it would have to be acting in the same way for these two unlinked variants. This can be likened to RCTs of different blood pressure-lowering agents, which work through different mechanisms and have different potential side-effects, but which lower blood pressure to the same degree. If the different agents produce the same reductions in cardiovascular disease risk then it is unlikely that this is through agent-specific effects of the drugs; rather it points to blood pressure-lowering as being key. The use of multiple genetic variants working through different pathways has not been explicitly applied in Mendelian randomization to date, but represents an important potential development in the methodology. Indeed the multiple genetic variants that have now been related to both cholesterol level and CHD risk constitute an informal demonstration of this multiple instrument approach.Citation107
Complexity of associations and interpretations
The interpretation of findings from studies that appear to fall within the Mendelian randomization remit can often be complex, as has been previously discussed with respect to MTHFR and folate intake Citation4. As a second example, consider the association of extracellular superoxide dismutase (EC-SOD) and CHD. EC-SOD is an extracellular scavenger of superoxide anions, and thus genetic variants associated with higher circulating EC-SOD levels might be considered to mimic higher levels of antioxidants. However, findings are dramatically opposite to this—bearers of such variants have an increased risk of CHD Citation108. The explanation of this apparent paradox could be that the higher circulating EC-SOD levels associated with the variant may arise from movement of EC-SOD from arterial walls; thus the in-situ anti-oxidative properties of these arterial walls is lower in individuals with the variant associated with higher circulating EC-SOD. The complexity of these interpretations—together with their sometimes speculative nature—detracts from the transparency that otherwise makes Mendelian randomization attractive.
Lack of suitable genetic variants to proxy for exposure of interest
An obvious limitation of Mendelian randomization is that it can only examine areas for which there are functional polymorphisms (or genetic markers linked to such functional polymorphisms) that are relevant to the modifiable exposure of interest. In the context of genetic association studies more generally it has been pointed out that in many cases even if a locus is involved in a disease-related metabolic process, there may be no suitable marker or functional polymorphism to allow study of this process Citation109. In an earlier paper on Mendelian randomization Citation4 we discussed the example of vitamin C, since one of our examples of how observational epidemiology appeared to have got the wrong answer related to vitamin C. We considered whether the association between vitamin C and coronary heart disease could have been studied utilizing the principles of Mendelian randomization. We stated that polymorphisms exist that are related to lower circulating vitamin C levels—for example, the haptoglobin polymorphism Citation110, Citation111—but in this case the effect on vitamin C is at some distance from the polymorphic protein and the other phenotypic differences could have an influence on CHD risk that would distort examination of the influence of vitamin C levels through relating genotype to disease. SLC23A1—a gene encoding for the vitamin C transporter SVCT1, involved in vitamin C transport by intestinal cells—would be an attractive candidate for Mendelian randomization studies. However, by 2003 (the date of the earlier paper) a search for variants had failed to find any common single nucleotide polymorphism (SNP) that could be used in such a way Citation112. We therefore used this as an example of a situation where suitable polymorphisms for studying the modifiable risk factor of interest—in this case vitamin C—could not be located. However, since the earlier paper was written, variation in SLC23A1 has been identified that is related to circulating vitamin C levels (Timpson et al., personal communication). We use this example not to suggest that the obstacle of locating relevant genetic variation for particular problems in observational epidemiology will always be overcome, but to point out that rapidly developing knowledge of human genomics will identify more variants that can serve as instruments for Mendelian randomization studies.
Summary
Mendelian randomization presents the epidemiologist with a potential strategy to overcome the limitations of observational epidemiology. Confounding, reverse causation, and bias have impeded the application of conventional epidemiology in several areas. Investigation within population-based association analyses may be helped by Mendelian randomization as a result of the fundamental properties of genetic variation which lend themselves to the direct exploration of causal pathways. It is undoubtedly important to note the challenges to the application of Mendelian randomization, and also to consider its use in examining the complex causal pathways in common diseases. The expanding scope of cardiovascular disease epidemiology is leading to a series of challenges that cannot be addressed by basic tests of association. Cardiovascular epidemiologists are increasingly investigating the contribution of a swathe of inflammatory and regulatory molecules to a range of cardiovascular disease phenotypes. While it is often clear that an association exists, the nature and direction of these relationships remain elusive. The application of non-confounded, directional, and unbiased assessment of such relationships offers new insights, possibility also a route to, now commonly advocated, translational research objectives. The results of Mendelian randomization analyses, where performed appropriately, present the opportunity to identify potentially modifiable exposure-disease relationships which may then be tested in further in-vivo, in-vitro, and intervention studies. Importantly, Mendelian randomization may contribute to decisions about the application of resources to continued investigation of biological pathways, and have the potential to determine where the use of RCTs might be expected to deliver decisive results.
References
- Vandenbroucke JP. When are observational studies as credible as randomised trials?. Lancet. 2004; 363: 1728–31
- Concato J, Horwitz RI. Beyond randomised versus observational studies. Lancet. 2004; 363: 1660–1
- Hernán MA, Brumback B, Robins JM. Estimating the causal effect of zidovudine on CD4 count with a marginal structural model for repeated measures. Statistics in Medicine. 2002; 21: 1689–709
- Davey Smith G, Ebrahim S. ‘Mendelian Randomisation’: can genetic epidemiology contribute to understanding environmental determinants of disease?. Int J Epidemiol. 2003; 32: 1–22
- Manson J Stampfer MJ Willett WC Colditz G Rosner B Speizer FE , et al. A prospective study of antioxidant vitamins and incidence of coronary heart disease in women. Circulation. 1991;84 suppl II:II–546.
- Rimm EB, Stampfer MJ, Ascherio A, Giovannucci E, Colditz GA, Willett WC. Vitamin E Consumption and the Risk of Coronary Heart Disease in Men. New Engl J Med. 1993; 328: 1450–6
- Stampfer MJ, Hennekens CH, Manson JE, Colditz GA, Rosner B, Willett WC. Vitamin E Consumption and the Risk of Coronary Disease in Women. New Engl J Med. 1993; 328: 1444–9
- Osganian SK, Stampfer MJ, Rimm E, Spiegelman D, Hu FB, Manson JE, et al. Vitamin C and risk of coronary heart disease in women. J Am Coll Cardiol. 2003; 42: 246–52
- Stampfer MJ, Colditz GA. Estrogen replacement therapy and coronary heart disease: a quantitative assessment of the epidemiologic evidence. Prev Med. 1991;20:47–63 ( reprinted Int J Epidemiol. 2004;33:445–53).
- Omenn GS, Goodman GE, Thornquist MD, Balmes J, Cullen MR, Glass A. Effects of a combination of beta carotene and vitamin A on lung cancer and cardiovascular disease. N Engl J Med. 1996; 334: 1150–5
- The effect of vitamin E and beta carotene on the incidence of lung cancer and other cancers in male smokers. The Alpha-Tocopherol, Beta Carotene Cancer Prevention Study Group. N Engl J Med. 1994;330:1029–35.
- Dietary supplementation with n-3 polyunsaturated fatty acids and vitamin E after myocardial infarction: results of the GISSI-Prevenzione trial. Gruppo Italiano per lo Studio della Sopravvivenza nell'Infarto miocardico. Lancet. 1999;354:447–55.
- Heart Protection Study Collaborative Group. MRC/BHF Heart Protection Study of antioxidant vitamin supplementation in 20536 high-risk individuals: a randomised placebo-controlled trial. Lancet. 2002;360:23–33.
- Beral V, Banks E, Reeves G. Evidence from randomized trials of the long-term effects of hormone replacement therapy. Lancet. 2002; 360: 942–4
- Eidelman RS, Hollar D, Hebert PR, Lamas GA, Hennekens CH. Randomized trials of vitamin E in the treatment and prevention of cardiovascular disease. Arch Internal Med. 2004; 164: 1552–6
- Khaw K-T, Bingham S, Welch A, Luben R, Wareham N, Oakes S, et al. Relation between plasma ascorbic acid and mortality in men and women in EPIC-Norfolk prospective study: a prospective population study. Lancet. 2001; 357: 657–63
- Lawlor DA, Davey Smith G, Kundu D, Bruckdorfer KR, Ebrahim S. Those confounded vitamins: what can we learn from the differences between observational versus randomised trial evidence?. Lancet. 2004; 363: 1724–7
- Lawlor DA, Ebrahim S, Kundu D, Bruckdorfer KR, Whincup PH, Davey Smith G. Vitamin C is not associated with coronary heart disease risk once life course socioeconomic position is taken into account: Prospective findings from the British Women's Heart and Health Study. Heart. 2005; 91: 1086–7
- Spearman C. The proof and measurement of association between two things. Am J Psychol. 1904; 15: 72–101
- Davey Smith G, Phillips AN. Inflation in epidemiology: ‘The proof and measurement of association between two things’ revisited. Br Med J. 1996; 312: 1659–61
- Peto R. Two properties of multiple regression analysis and regression to the mean (and regression from the mean). The natural history of chronic bronchitis and emphysema: an eight year study of early chronic obstructive lung disease in working men in London, CM Fletcher, R Peto, CM Tinker, FE Speizer. Oxford University Press, Oxford 1976; 218–23
- Davey Smith G, Ebrahim S. Mendelian randomization: prospects, potentials, and limitations. Int J Epidemiol. 2004; 33: 30–42
- Davey Smith G, Ebrahim S. What can mendelian randomization tell us about modifiable behavioural and environmental exposures. BMJ. 2005; 330: 1076–9
- Davey Smith G. Randomised by (your) god: robust inference from an observational study design. J Epidemiol Community Health. 2006; 60: 382–8
- Davey Smith G, Ebrahim S. Mendelian randomization: Genetic variants as instruments for strengthening causal inference in observational studies. Biosocial surveys: Current insight and future promise. National Research Council, JW Vaupel, M Weinstein, KW Wachter. The National Academies Press, Washington, DC 2008; 336–66
- Goldschmidt RB. Physiological Genetics. McGraw Hill, New York 1938
- Baron DN, Dent CE, Harris H, Hart EW, Jepson JB. Hereditary pellagra-like skin rash with temporary cerebellar ataxia, constant renal amino-aciduria, and other bizarre biochemical features. Lancet. 1956; 271: 421–8
- Snyder LH. Fifty years of medical genetics. Science. 1959; 129: 7–13
- Guy JT. Oral manifestations of systematic disease. Otolaryngology—Head and Neck Surgery, CW Cummings. Mosby Year Book, Inc, St Louis 1993; 2
- Gause GF. The relation of adaptability to adaption. Q Rev Biol. 1942; 17: 99–114
- Jablonka-Tavory E. Genocopies and the evolution of interdependence. Evolutionary Theory. 1982; 6: 167–70
- Palmer L, Cardon L. Shaking the tree: mapping complex disease genes with linkage disequilibrium. Lancet. 2005; 366: 1223–34
- Keavney B, Danesh J, Parish S, Palmer A, Clark S, Youngman L, et al. Fibrinogen and coronary heart disease: test of causality by ‘Mendelian randomization’. Int J Epidemiol. 2006; 35: 935–43
- Bhatti P, Sigurdson AJ, Wang SS, Chen J, Rothman N, Hartge P, et al. Genetic variation and willingness to participate in epidemiological research: data from three studies. Cancer Epidemiol Biomarkers Prev. 2005; 14: 2449–53
- Marmot M. Reflections on alcohol and coronary heart disease. Int J Epidemiol. 2001; 30: 729–34
- Bovet P, Paccaud F. Alcohol, coronary heart disease and public health: which evidence-based policy?. Int J Epidemiol. 2001; 30: 734–7
- Klatsky AL. Commentary: Could abstinence from alcohol be hazardous to your health?. Int J Epidemiol. 2001; 30: 739–42
- Shaper AG. Editorial: alcohol, the heart, and health. Am J Public Health. 1993; 83: 799–801
- Hart C, Davey Smith G, Hole D, Hawthorne V. Alcohol consumption and mortality from all causes, coronary heart disease, and stroke: results from a prospective cohort study of Scottish men with 21 years of follow up. BMJ. 1999; 318: 1725–9
- Rimm E. Commentary: Alcohol and coronary heart disease—laying the foundation for future work. Int J Epidemiol. 2001; 30: 738–9
- Enomoto N, Takase S, Yasuhara M, Takada A. Acetaldehyde metabolism in different aldehyde dehydrogenase-2 genotypes. Alcohol Clin Exp Res. 1991; 15: 141–4
- Takagi S, Iwai N, Yamauchi R, Kojima S, Yasuno S, Baba T, et al. Aldehyde dehydrogenase 2 gene is a risk factor for myocardial infarction in Japanese Men. Hypertens Res. 2002; 25: 677–81
- Chao Y-C, Liou S-R, Chung Y-Y, Tang H-S, Hsu C-T, Li T-K, et al. Polymorphism of alcohol and aldehyde dehydrogenase genes and alcoholic cirrhosis in Chinese patients. Hepatology. 1994; 19: 360–6
- Burr ML, Fehily AM, Butland BK, Bolton CH, Eastham RD. Alcohol and high-density-lipoprotein cholesterol: a randomized controlled trial. Br J Nutr. 1986; 56: 81–6
- Chen L, Davey Smith G, Harbord R, Lewis S. Alcohol intake and blood pressure: a systematic review implementing a Mendelian Randomization approach. PLoS Medicine 2008; 5: 461–471
- LDL receptor mutation catalogue. Available at: http://www.ucl.ac.uk/fh (accessed 16 December 2003).
- Marks D, Thorogood M, Neil HAW, Humphries SE. A review on diagnosis, natural history and treatment of familial hypercholesterolaemia. Atherosclerosis. 2003; 168: 1–14
- Slack J. Risks of ischaemic heart disease in familial hyperlipoproteinaemic states. Lancet. 1969; 2: 1380–2
- Risk of fatal coronary heart disease in familial hypercholesterolaemia. Scientific Steering Committee on behalf of the Simon Broome Register Group. BMJ. 1991;303:893–6.
- Cholesterol sceptics website. Available at: http://www.thincs.org/ (accessed 16 August 2007).
- Steinberg D. Thematic review series: The Pathogenesis of Atherosclerosis. An interpretive history of the cholesterol controversy. Part 1. J Lipid Res. 2004; 45: 1583–93
- Steinberg D. Thematic review series: The Pathogenesis of Atherosclerosis. An interpretive history of the cholesterol controversy. Part II. The early evidence linking hypercholestrolemia to coronary disease in humans. J Lipid Res. 2005; 46: 179–90
- Færgeman O. Coronary Artery Disease: Genes Drugs and the Agricultural Connection. Elsevier, AmsterdamNetherlands 2003
- Baigent C, Keech A, Kearney P, Blackwell L, Buck G, Pollicino C, et al. Cholesterol Lowering Treatments Collaboration. Efficacy and safety of cholesterol-lowering treatment: prospective meta-analysis of data from 90,056 participants in 14 randomised trials of statins. Lancet. 2005; 366: 1267–78
- Rose G. Incubation period of coronary heart disease. BMJ. 1982; 284: 1600–1
- Soria LF, Ludwig EH, Clarke HRG, Vega GL, Grundy SM, McCarthy BJ. Association between a specific apolipoprotein B mutation and familial defective apolipoprotein B-100. Proc Natl Acad Sci U S A. 1989; 86: 587–91
- Tybjaerg-Hansen A, Humphries SE. Familial defective apolipoprotein B-100: a single mutation that causes hypercholesterolemia and premature coronary artery disease. Atherosclerosis. 1992; 96: 91–107
- Myant NB. Familial defective apolipoprotein B-100: a review, including some comparisons with familial hypercholesterolaemia. Atherosclerosis. 1993; 104: 1–18
- Tybjærg-Hansen A, Steffenson R, Meinertz H, Schnohr P, Nordestgaard BG. Association of mutations in the apolipoprotein B gene with hypercholesterolemia and the risk of ischemic heart disease. New Engl J Med. 1998; 338: 1577–84
- Cohen JC, Boerwinkle E, Mosely TH, Hobbs HH. Sequence variations in PSCK9, low LDL, and protection against coronary heart disease. New Eng J Med. 2006; 354: 1264–72
- Brown MS, Goldstein JL. Lowering LDL—not only how low, but how long?. Science. 2006; 311: 1721–3
- Danesh J, Wheller JB, Hirschfield GM, Eda S, Eriksdottir G, Rumley A, et al. C-reactive protein and other circulating markers of inflammation. In the prediction of coronary heart disease. New Engl J Med. 2004; 350: 1387–97
- Wu T, Dorn JP, Donahue RP, Sempos CT, Trevisan M. Associations of serum C-reactive protein with fasting insulin, glucose, and glycosylated hemoglobin: the Third National Health and Nutrition Examination Survey, 1988–1994. Am J Epidemiol. 2002; 155: 65–71
- Pradhan AD, Manson JE, Rifai N, Buring JE, Ridker PM. C-reactive protein, interleukin 6, and risk of developing type 2 diabetes mellitus. JAMA. 2001; 286: 327–34
- Han TS, Sattar N, Williams K, Gonzalez-Villalpando C, Lean ME, Haffner SM. Prospective study of C-reactive protein in relation to the development of diabetes and metabolic syndrome in the Mexico City Diabetes Study. Diabetes Care. 2002; 25: 2016–21
- Sesso D, Buring JE, Rifai N, Blake GJ, Gaziano JM, Ridker PM. C-reactive protein and the risk of developing hypertension. JAMA. 2003; 290: 2945–51
- Hirschfield GM, Pepys MB. C-reactive protein and cardiovascular disease: new insights from an old molecule. QJM. 2003; 9: 793–807
- Hu FB, Meigs JB, Li TY, Rifai N, Manson JE. Inflammatory Markers and Risk of Developing Type 2 Diabetes in Women. Diabetes. 2004; 53: 693–700
- Ridker PM, Cannon CP, Morrow D, Rifai N, Rose LM, McCabe CH, et al. C-reactive protein levels and outcomes after statin therapy. New Engl J Med. 2005; 352: 20–8
- Sjöholm A, Nyström T. Endothelial inflammation in insulin resistance. Lancet. 2005; 365: 610–2
- Verma S, Szmitko PE, Ridker PM. C-reactive protein comes of age. Nat Clin Pract Cardiovasc Med. 2005; 2: 29–36
- Ridker PM, Rifai N, Rose L, Buring JE, Cook NR. Comparison of C-reactive protein and low-density lipoprotein cholesterol levels in the prediction of first cardiovascular events. N Engl J Med. 2002; 347: 1557–65
- Danesh J, Whincup P, Walker M, Lennon L, Thomson A, Appleby P, et al. Low-grade inflammation and coronary heart disease: prospective study and updated meta-analyses. BMJ. 2000; 321: 199–204
- Ridker P. Role of inflammatory biomarkers in prediction of coronary heart disease. Lancet. 2001; 358: 946–8
- Taylor KE, Giddings JC, van den Berg CW. C-Reactive protein induced in vitro endothelial cell activation is an artefact caused by azide and lipopolysaccharide. Arterioscler Thromb Vasc Biol. 2005; 25: 1225–30
- Hirschfield GM, Gallimore JR, Kahan MC, Hutchinson WL, Sabin CA, Benson GM, et al. Transgenic human C-reactive protein is not proatherogenic in apolipoprotein E-deficient mice. Proc Natl Acad Sci U S A. 2005; 102: 8309–14
- Pepys MB, Hirschfield GM, Tennent GA, Gallimore JR, Kahan MC, Bellotti V, et al. Targeting C-reactive protein for the treatment of cardiovascular disease. Nature. 2006; 440: 1217–21
- Davey Smith G, Lawlor D, Harbord R, Timpson N, Rumley A, Lowe G, et al. Association of C-reactive protein with blood pressure and hypertension: life course confounding and Mendelian randomization tests of causality. Arterioscler Thromb Vasc Biol. 2005; 25: 1051–6
- Timpson NJ, Lawlor DA, Harbord RM, Gaunt TR, Day INM, Palmer LJ, et al. C-reactive protein and its role in metabolic syndrome: mendelian randomization study. Lancet. 2005; 366: 1954–9
- Casas JP, Shah T, Cooper J, Hawe E, McMahon AD, Gaffney D, et al. Insight into the nature of the CRP-coronary event association using Mendelian randomization. Int J Epidemiol. 2006; 35: 922–31
- Davey Smith G, Harbord R, Milton J, Ebrahim S, Sterne JAC. Does Elevated Plasma Fibrinogen Increase the Risk of Coronary Heart Disease? Evidence from a Meta-Analysis of Genetic Association Studies. Arterioscler Thromb Vasc Biol. 2005; 25: 2228–33
- Tegner J, Bjorkegren J. Perturbations to uncover gene networks. Trends Genet. 2007; 23: 34–41
- Danesh J, Whincup P, Walker M, Lennon L, Thomson A, Appleby P, et al. Chlamydia pneumoniae IgG titres and coronary heart disease: prospective study and meta-analysis. BMJ. 2000; 321: 208–13
- Sung J, Song Y-M, Choi Y-H, Ebrahim S, Davey Smith G. Hepatitis B Virus Seropositivity and the Risk of Stroke and Myocardial Infarction. Stroke. 2007; 38: 1436
- Andraws R, Berger JS, Brown DL. Effects of antibiotic therapy on outcomes of patients with coronary artery disease: a meta-analysis of randomized controlled trials. JAMA. 2005; 293: 2641–7
- Smeeth L, Thomas SL, Hall AJ, Hubbard R, Farrington P, Vallance P. Risk of myocardial infarction and stroke after acute infection or vaccination. N Engl J Med. 2004; 351: 2611–18
- Kiechl S, Lorenz E, Reindl M, Wiedermann CJ, Oberhollenzer F, Bonora E, et al. Toll-like receptor 4 polymorphisms and atherogenesis. N Engl J Med. 2002; 347: 185–92
- Smeeth L, Casas JP, Hingorani AD. The role of infection in cardiovascular disease: more support but many questions remain. Eur Heart J. 2007; 28: 1178–9
- Jousilahti P, Salomaa V. Fibrinogen, social position, and Mendelian randomisation. J Epidemiol Community Health. 2004; 58: 883
- Glynn RK. Commentary: Genes as instruments for evaluation of markers and causes. Int J Epidemiol. 2006; 35: 932–4
- Thomas DC, Conti DV. Commentary on the concept of ‘Mendelian Randomization’. Int J Epidemiol. 2004; 33: 17–21
- Didelez V Sheehan NA Mendelian randomization: Why Epidemiology needs a formal language for causality. Stat Methods Med Res. 2007 ( in press).
- Lawlor DA, Harbord RM, Sterne JA, Timpson N, Davey Smith G. Mendelian randomization: using genes as instruments for making causal inferences in epidemiology. Stat Med. 2008; 27: 1133–63
- Colhoun H, McKeigue PM, Davey Smith G. Problems of reporting genetic associations with complex outcomes. Lancet. 2003; 361: 865–72
- Wellcome Trust Case Control Consortium. Genome-wide association study of 14,000 cases of seven common diseases and 3,000 shared controls. Nature. 2007;447:661–78.
- Frayling T, Timpson NJ, Weedon N, Zeggini E, Freathy RM, Lindgren CM. A Common Variant in the FTO Gene Is Associated with Body Mass Index and Predisposes to Childhood and Adult Obesity. Science. 2007; 316: 889–94
- Waddington CH. Canalization of development and the inheritance of acquired characteristics. Nature. 1942; 150: 563–5
- Wilkins AS. Canalization: a molecular genetic perspective. Bioessays. 1997; 19: 257–62
- Rutherford SL. From genotype to phenotype: buffering mechanisms and the storage of genetic information. BioEssays. 2000; 22: 1095–105
- Gibson G, Wagner G. Canalisation in evolutionary genetics: a stabilising theory?. BioEssays. 2000; 22: 372–80
- Hartman JLT, Garvik B, Hartwell L. Principles for the buffering of genetic variation. Science. 2001; 291: 1001–4
- Debat V, David P. Mendelian randomization as an instrumental variable approach to caausal inference. Trends Ecol Evol. 2001; 16: 555–61
- Kitami T, Nadeau JH. Biochemical networking contributes more to genetic buffering in human and mouse metabolic pathways than does gene duplication. [erratum appears in Nat Genet. 2002;32:681]. Nat Genet. 2002; 32: 191–4
- Gu Z, Steinmetz LM, Gu X, Scharfe C, Davis RW, Li W-H. Role of duplicate genes in genetic robustness against null mutations. Nature. 2003; 421: 63–6
- Hornstein E, Shomron N. Canalization of development by microRNAs. Nat Genet. 2006; 38: S20–4
- Davey Smith G, Lawlor DA, Harbord R, Timpson NJ, Day I, Ebrahim S. Clustered Environments and Randomized Genes: a fundamental distinction between conventional and genetic epidemiology. PLoS-Med. 2007; 4: e352
- Kathiresan S, Melander O, Anevski D, Guiducci C, Burtt NP, Roos C, et al. Polymorphisms associated with cholesterol and risk of cardiovascular events. N Engl J Med 2008; 358;12: 1240–1249
- Juul K, Tybjaerg-Hansen A, Marklund S, Heegaard NHH, Steffensen R, Sillesen H, et al. Genetically reduced antioxidative protection and increased ischaemic heart disease risk: the Copenhagen city heart study. Circulation. 2004; 109: 59–65
- Weiss K, Terwilliger J. How many diseases does it take to map a gene with SNPs?. Nat Genet. 2000; 26: 151–7
- Langlois MR, Delanghe JR, De Buyzere ML, Bernard DR. Ouyang J. Effect of haptoglobin on the metabolism of vitamin C. Am J Clin Nutr. 1997; 66: 606–10
- Delanghe J, Langlois M, Duprez D, De Buyzere M, Clement D. Haptoglobin polymorphism and peripheral arterial occlusive disease. Atherosclerosis. 1999; 145: 287–92
- Erichsen HC, Eck P, Levine M, Chanock S. Characterization of the genomic structure of the human vitamin C transporter SVCT1 (SLC23A2). J Nutr. 2001; 131: 2623–7
- Cardon LR, Bell JI. Association study designs for complex diseases. Nat Rev Genet. 2001; 2: 91–9