Abstract
Despite its exploration immaturity, the Central Lapland Greenstone Belt has geodynamic, geological and gold-endowment characteristics to indicate that it is potentially the premier orogenic-gold province in Finland. Previous GIS-based prospectivity mapping of the Central Lapland Greenstone Belt used only empirical geophysical and geochemical datasets based on the weights-of-evidence integration method. In this study, such empirical data are combined with conceptual geological parameters derived from a 1:200 000 scale bedrock map of northern Finland and use the weights-of-evidence, logistic regression and fuzzy-logic methods for integration. A targeting model is developed based both on the empirical data and conceptual parameters defined from understanding of the processes essential to produce large deposits in an orogenic-gold mineral system. Key spatially referenced layers are derived from the empirical data and from critical proxies for essential processes in the targeting model, and the spatial association between these key layers and a training set of known gold deposits and prospects is quantified. Key parameters are then reclassified into binary layers according to maximum spatial association with the training set, and these layers are then integrated into prospectivity maps using both the weights-of-evidence and logistic regression methods. The highest probability class in all models defines <1% of the Central Lapland Greenstone Belt, a remarkable reduction in highest-priority target zones. The resulting maps are evaluated using a set of gold prospects excluded from the original training set as validation sites. The resultant prospectivity maps provide subtle, but significant, improvement on the maps derived solely from empirical data, especially where logistic regression is applied. Proxies for strain gradients appear particularly important. The analysis demonstrates that homogeneous, high-quality, small-scale geological maps, integrated using a robust targeting model, can enhance the predictive capacity of prospectivity maps derived from GIS-based modelling.
Introduction
Orogenic-gold deposits, as defined by Groves et al. (Citation1998), are the most common type of gold mineralisation in Finland, representing about 90% of the drilled gold occurrences in the country (Eilu et al. Citation2003). These deposits occur either in Late Archean greenstone belts or in Paleoproterozoic greenstone and schist belts (Eilu & Weihed Citation2005). The current paper focuses on finding gold deposits in the Paleoproterozoic Central Lapland Greenstone Belt in the Northern Fennoscandian Shield, northern Finland (, ).
Figure 2 Geological map of the study area based on the 1:200 000 scale mapping by Lehtonen et al. (Citation1998). Gold occurrences based on the FinGold database (Eilu Citation1999).
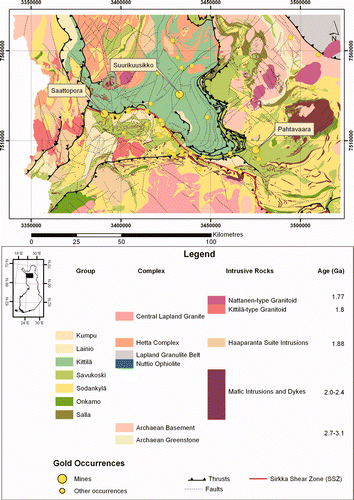
Prospectivity mapping in its various forms incorporates spatially referenced data from a range of geoscientific or other sources into a single map that highlights the most prospective areas for the mineral resource of interest. Terminology used in this paper is based on Bonham-Carter (Citation1994) and Raines (Citation1999), as summarised in . Bonham-Carter (Citation1994) divided the quantitative approaches of mineral prospectivity mapping into two main categories: empirical (data driven) and conceptual (knowledge driven) approaches. There are a number of recent examples in which the empirical weights-of-evidence method is used for mineral prospectivity mapping or other analogous assessments (Carranza & Hale Citation2000; Harris et al. Citation2000, Citation2001; Venkataraman et al. Citation2000; Paganelli et al. Citation2002; Raines & Mihalasky Citation2002; Carranza Citation2004; Cheng Citation2004; Harris & Sanborn-Barrie Citation2006; Kapo & Burton Citation2006; Raines & Bonham-Carter Citation2006). Nykänen & Salmirinne (Citation2007) demonstrated a straightforward, largely empirical, approach to conduct prospectivity mapping for orogenic gold by using only geophysical and geochemical data as inputs and ignoring the geological interpretations. In addition to simplicity, their reasoning for not using geological input was that the weights-of-evidence method (Bonham-Carter Citation1994) is based on Bayes' rule, which requires conditional independence of the primary data layers used in the predictive calculations. The geometrical features in bedrock geological maps of Finland are mainly derived from multi-component high-resolution airborne geophysical surveys (Airo Citation2005), and hence these data are conditionally dependent on each other to some extent.
Table 1 Terminology, based largely on Bonham-Carter (Citation1994), Raines (Citation1999) and Raines & Bonham-Carter (Citation2006).
To avoid this problem, there are analytical methods available that do not have this requirement of conditional independency, and thus enable the use of more variable data sources for prediction models. Alternatively, a certain amount of uncertainty could be tolerated and thus the consequences of the conditional dependencies accepted in the weights-of-evidence methodology (Agterberg & Cheng Citation2002). There are a number of examples in which regression and logistic regression have been applied to mineral exploration or prospectivity mapping (Agterberg Citation1974; Chung & Agterberg Citation1980; Reddy et al. Citation1991; Harris & Pan Citation1999; Sahoo & Pandalai Citation1999; Carranza & Hale Citation2001; Robinson et al. Citation2004). This paper uses logistic regression in combination with the weights-of-evidence method for prospectivity mapping as a compromise between the two approaches to avoid the conditional dependency issue. Artificial neural networks would be another applicable and increasingly popular approach to complete the task (Harris & Pan Citation1999; Koike et al. Citation2002; Bougrain et al. Citation2003; Brown et al. Citation2000, Citation2003; Porwal et al. Citation2003, Citation2004), but was not used here to keep the modelling process as close to that published by Nykänen & Salmirinne (Citation2007) for comparison.
Although the purely empirical approach of Nykänen & Salmirinne (Citation2007) was able to indicate prospective areas, the question of how the geological input data might improve the models was raised. As has been described in several papers on orogenic-gold deposits (Groves et al. Citation1998, Citation2000; Eilu et al. Citation2003; Goldfarb et al. Citation2005), there are certain geological factors derived from interpretations of geological maps that are useful inputs in predictive gold exploration. Therefore, this paper incorporates several derivatives of the digital 1:200 000 scale bedrock map of Northern Finland (Lehtonen et al. Citation1998) into the spatial modelling using a combination of the weights-of-evidence (Bonham-Carter Citation1994) and logistic regression (Agterberg et al. Citation1993) methods. The aim is to show how the empirical spatial modelling is enhanced using both empirical and conceptual input data.
Study area
The study area is a roughly 20 000 km2 area in the Northern Fennoscandian Shield (, ) covering the middle parts of the Central Lapland Greenstone Belt. The area, located some 100 km north of the Arctic Circle, is mainly composed of Paleoproterozoic mafic to ultramafic volcanic sequences and related sedimentary units (Lehtonen et al. Citation1998) surrounded by younger granitic intrusions. The sedimentary and volcanic units are also intruded by mafic dykes and sills of various ages. The area has been under sporadic gold exploration since the mid-1980s, but is nevertheless considered as a greenfields exploration terrain. Most gold exploration in the Central Lapland Greenstone Belt has concentrated on the Sirkka Shear Zone, which extends approximately east – west across the entire greenstone belt. However, there are several gold prospects a considerable distance from the Sirkka Shear Zone, suggesting that there are other controlling geological factors. The FinGOLD database (Eilu Citation1999) includes over 30 drilled gold occurrences with at least 1 m of 1 ppm Au in the area. These occurrences provide a convenient way of quantitatively estimating the relative importance of geological, geophysical and geochemical parameters in controlling gold mineralisation. Importantly, Weihed et al. (Citation2005) summarised the indirect age constraints as 1.9 – 1.86 Ga and 1.85 – 1.79 Ga for two Paleoproterozoic orogenic-gold mineralising events in the Fennoscandian Shield. Based on geophysical and field evidence (Airo & Mertanen Citation2001; Korkalo Citation2006), gold mineralisation cuts the overlying quartzitic sedimentary units (Kumpu Group: ). It is assumed that the timing of gold mineralisation is late- or post-orogenic, thus being among the latest events and making it possible to use the current geometries on the geological map as one of the controlling factors in the spatial modelling.
Targeting model
lists the data used to define a targeting model (see Hronsky & Groves Citation2008) to be used to produce the prospectivity map of the Central Lapland Greenstone Belt. The first column in lists the parameters, which are described in terms of available datasets in the second column. The first four data layers that define the empirical parameters are low magnetic intensity and low resistivity from the airborne geophysical survey, gravity gradient from the regional gravity survey, and anomalous till geochemistry (Nykänen & Salmirinne Citation2007). The remainder of the parameters are derived from the digital 1:200 000 scale bedrock map of the Central Lapland Greenstone Belt (Lehtonen et al. Citation1998). The selection of the primary data layers was based both on expert opinions and recent exploration success. The derivatives of the bedrock map were generated by using common GIS tools and functions as discussed below. According to Weihed et al. (Citation2005), all orogenic-gold deposits in the Fennoscandian Shield are structurally controlled, being located in second- to lower-order shear or fault zones within local compressional to transpressional structures at the time of mineralisation. Furthermore, they described the favourable sites for orogenic gold on a local scale as: (i) pre-gold albitised units; (ii) competent units enveloped by less-competent rocks; and (iii) contact zones between chemically reactive rocks with a significant competency contrast with adjacent rocks. Since these features are not commonly shown on regional-scale geological maps, derivatives of the geological map are used together with geophysical data as proxies for them.
Table 2 Evidence for the exploration model and the datasets that define them.
Training sites
The empirical spatial modelling (e.g. weights-of-evidence or logistic regression) requires a set of known gold prospects or deposits to be used as training sites in the calculations. The selection of the training sites is critical, and as far as possible, they need to represent a coherent group. For this study, 24 known orogenic-gold prospects or deposits () in the study area were selected from the FinGOLD database (Eilu Citation1999). Because these prospects or deposits tend to be narrow (generally a few tens of metres) but relatively long in strike (hundreds of metres up to several kilometres), some of the training sites are represented by a set of points along the strike. Thus, the total number of training points used in the modelling was 35. Most of the training sites are active exploration targets or prospects, and one of them is currently in production. The study area also contains a few other orogenic-gold occurrences, which were used not for training but as a validation of the final exploration models ().
Table 3 Training sites (gold deposits and prospects) used in the modelling, ordered by their discovery year.
Table 4 Gold prospects used as validation sites.
Neither weights-of-evidence nor logistic regression methods can use a training site attribute (e.g. tonnage or other measure of deposit size) to give weights to individual sites in the training set. This could be achieved by using only deposits with significantly large tonnage as training sites (like the past and present producers of gold), but this would result in high uncertainties in areas with only a few real deposits. As only one of the deposits is world class in the Central Lapland Greenstone Belt, it is difficult to bias the analysis towards larger deposit sizes.
Primary datasets for empirical parameters
The datasets used to define the empirical parameters were high-resolution airborne geophysics and regional till geochemistry and their derivatives. In the following sections, the primary datasets are described, followed by descriptions of the methodologies to generate the input parameters for modelling.
Before data integration into prospectivity maps, several tasks need to be completed in GIS to extract the relevant information from the datasets. There are routine GIS tools to apply spatial analytical calculations to the vector GIS layers, such as proximity analysis, density analysis and visibility analysis, or various interpolation methods such as inverse distance weighting, spline or kriging. Then, the resultant grid data can be reclassified, generalised or integrated in several ways. Focal functions may be needed to calculate statistical values over a certain neighbourhood around each cell across the study area. There are various filters to apply. In general, the pre-processing of the primary datasets (i.e. the input parameter generation) is, in many cases, the most time-consuming and perhaps the most critical part after development of the targeting model using the expertise of exploration geologists, geophysicists and geochemists. The input data layers, and how they were derived from the primary datasets based on the targeting model, are described below.
Airborne geophysics and alteration zones
Almost the entire land surface of Finland is covered by high-resolution multicomponent airborne geophysical surveys flown at 40 m altitude and 200 m line spacing (Airo Citation2005). In the current study area, the airborne surveys were carried out between 1975 and 2003. Airborne magnetic measurements were derived from a cesium magnetometer on the rear boom of the aircraft. Secular and other magnetic field variations were corrected according to data from a base-station registration. The line data were levelled using an in-house program, and final levelling was done using tie lines. Airborne electromagnetic (EM) surveys were carried out to map conductivity using the dual-frequency fixed-wing EM equipment. The measured parameters are in-phase and quadrature components of both frequencies 3 kHz and 14 kHz. The lower frequency 3 kHz, with better depth penetration (Peltoniemi Citation1998), has been widely used for mineral exploration in Finland, and also elsewhere in Europe and in Africa. The higher frequency 14 kHz is more suitable for engineering and environmental applications (Poikonen et al. Citation1998).
The orogenic-gold deposits in the greenstone belt tend to be spatially related to multi-stage alteration zones, which causes variable but detectable effects in the host-rocks. The alteration styles reported are sericitisation and carbonitisation in lower to middle greenschist facies domains, biotitisation and carbonation in upper greenschist to lower amphibolite facies domains, and biotitisation and formation of K-feldspar and calc-silicate minerals in higher metamorphic-grade domains (Eilu & Weihed Citation2005), Most commonly, the breakdown of magnetite due to hydrothermal alteration is the most prominent and detectable feature in measured magnetic-field total intensity (Airo Citation2002). The high-resolution low-altitude airborne survey of the Geological Survey of Finland has resulted in excellent-quality magnetic data, and the application of a median filter significantly enhances the magnetic lows associated with the alteration zones hosting the deposits (Nykänen & Salmirinne Citation2007). The median filter was calculated by subtracting the median value of a 4 km radius circular neighbourhood from the raw aeromagnetic data around each of the cells in the grid to produce a magnetic residual (). This was then used as an input in the modelling.
Figure 3 Filtering of the aeromagnetic data using a median filter. A moving window of 4000 m radius was applied to calculate the median value around each 50×50 m cell of the original magnetic-field total-intensity grid. The resulting median value in the 250×250 m grid was then subtracted from the original magnetic-field total-intensity grid, resulting in the residual grid, which is used as the input in the spatial modelling.
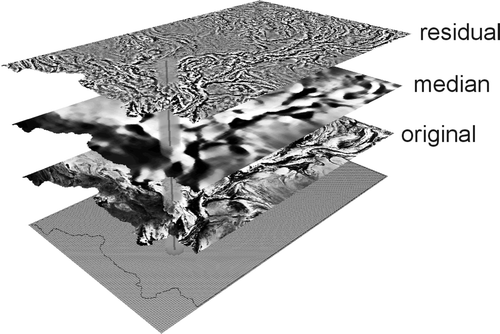
Gravity data and large crustal structures
The regional gravimetric survey in the study area was carried out with an average density of one point every 1 km2 by the Geological Survey of Finland and the Finnish Geodetic Institute (Kääriäinen & Mäkinen Citation1997).
Gravity reflects large crustal-scale structures, lithological units, faults, and shear zones, particularly those that cause the thickening of the greenstone lithologies, commonly associated with gold deposits (Jamieson et al. Citation1998). Bouguer anomaly data were interpolated to a grid cell size of 500×500 m, and the horizontal gradient was calculated for the analysis to represent significant rock boundaries, including zone of significant thickening of supracrustal sequences ().
Figure 4 (a) Gravity gradient calculated from the regional gravimetric survey with a point density of 1 point/km2. Missing data in the gravity gradient are dealt with as zero weight in weights-of-evidence and area weighted mean value in logistic regression. (b) Combined till geochemistry (Au, As, Cu, Fe, Ni and Te) indicating the favourable areas for gold mineralisation. (c) Density of the contacts between geological units.
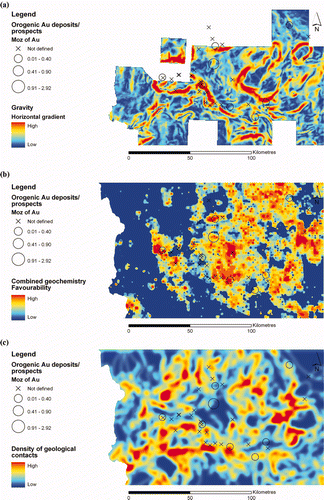
Till geochemistry
The countrywide regional till-geochemical survey was conducted in the 1980s (Salminen Citation1995) with a sampling density of one sample per 4 km2. Most of the samples were collected as a composite of three to five subsamples, from an average depth of 1.5 m, taken with a portable percussion drill equipped with a through-flow bit. In the Central Lapland Greenstone Belt study area, part of the sampling was conducted by combining five to 10 line samples. The consequence is that some artefacts have been introduced, which are shown as level differences between the map sheets with single samples. The sampled material was chemically unaltered parent till. The samples were dried and the <0.06 mm fraction sieved for analysis. Hot aqua regia digest was used, and Al, Ba, Ca, Co, Cr, Cu, Fe, K, La, Li, Mg, Mn, Mo, Ni, P, Pb, Sc, Sr, Th, Ti, V, Y, Zn and Zr were determined by ICP-AES. In addition, Au, Pd and Te were analysed with graphite-furnace AAS (Kontas Citation1981; Kontas et al. Citation1990).
The orogenic-gold deposits are associated with several indicative geochemical anomalies, and the regional till-geochemical survey of the Geological Survey of Finland has been widely used as a reconnaissance-scale exploration tool. For modelling, the selected element data were interpolated into grids with 200×200 m cell size using inverse distance weighting. The elements used were As, Au, Cu, Fe, Ni and Te, reflecting a sulfide source and a gold occurrence. The inverse distance weighting (IDW) algorithm, is based on the following equation
where w(d) is the weighting factor applied to a known value, d is the distance from this value, and p is a power factor. The d and p factors are commonly defined by user in a GIS software. Alternatively, the user may define the number of samples included in interpolation together with the maximum distance used, and the software would compute the variable distance factor when calculating a value for each interpolated cell. On the other hand, when applying a fixed search radius, the user specifies the distance of the radius of the circle used to search for points around each interpolated cell and also a minimum number of points that must be found. The nature of the IDW interpolation method is that the weights are decreasing by the increasing distance from the interpolated points (e.g. geochemical sampling points). The IDW method implements the spatial autocorrelation theory that objects that are close to one another are more alike than those that are farther apart. It is especially suitable if sample points are evenly distributed throughout the study area and if they are not clustered. The IDW method thus fits well for a regional till-geochemical data used here.
To avoid the effect of conditional dependency, a single anomaly map was generated from the six separate interpolated colour surface maps of the individual elements (As, Au, Cu, Fe, Ni and Te) by rescaling them first to a common scale from 0 to 1 and then combining the maps by using the operators in the GIS in the following way (Nykänen & Salmirinne Citation2007): the anomaly maps of As, Fe, Ni and Te were combined first by using the ‘fuzzy or’ operator resulting in a combination that was integrated together with the Cu anomaly map by using the ‘fuzzy and’ operator. Finally, Au anomaly map was combined with this combination by using the ‘fuzzy gamma’ operator ().
Bedrock geological map and derived structural interpretations
The derivatives of the digital 1:200 000 bedrock map of northern Finland (Lehtonen et al. Citation1998) were used also as conceptual inputs in the modelling. The map has been mainly digitised in 1:50 000 scale using existing airborne geophysics, and then generalised into 1:200 000 scale. The map has been updated as new airborne data became available. Most of the area was covered by the low-altitude airborne survey at the initial stage. The map defines the broader scale stratigraphic units, such as groups and formations, for the sedimentary and volcanic sequences, not the actual lithologies within the formations. This is a limiting factor on its usefulness, because the deposits are predicted to be sited at rheological and geochemical boundaries not shown at the scale used in the analysis. A simplified and somewhat stylised version of the geological map was also used as an input into the geomechanical paleostress modelling, as discussed below. For the prospectivity analysis, several spatial analysis functions in the GIS, including proximity analysis and density analysis, were applied to generate the input map parameters.
Geological complexity
Most orogenic-gold deposits occur in domains of geologic complexity or complexity gradients (Hodkiewicz et al. Citation2005). The concept of lithodiversity or geologic diversity defines the frequency of rock types in a given neighbourhood or pixel in a geological map. As shown by Mihalasky & Bonham-Carter (Citation2001), there is a spatial association between mineral deposits and increased lithodiversity, which could therefore be used as a potential predictor of deposits. Lithodiversity can be calculated by counting the number of unique geological map units in a moving window of desired shape and size, the spatial neighbourhood. However, for the current paper, the density of the contacts of the individual geological map units using a 4 km radius search area () was calculated instead and then used as a proxy for lithodiversity.
First-order structural control
According to Groves et al. (Citation2000) orogenic-gold deposits are formed over a large crustal-depth range from deep-seated low-salinity H2O – CO2 ± CH4 ± N2 ore fluids and with Au transported as thio-complexes. Structure is the first-order control on the location of orogenic-gold deposits (Hodgson Citation1993; Groves et al. Citation2000; Goldfarb et al. Citation2005). In particular, the gold deposits are commonly aligned along crustal-scale shear zones (Goldfarb et al. Citation2005) and/or are sited at the boundary between convergent and divergent stress regimes caused by complexities in the geometries of granitoid domes (Groves et al. Citation2000). Proximity to the Sirkka Shear Zone is an obvious parameter, since many of the gold occurrences in the Central Lapland Greenstone Belt are closely associated with this major structure. This proximity grid was combined with a grid of a parameter that measures divergent/convergent stress regimes. The latter was calculated by first completing a proximity analysis to granitoids in the Kittilä, Savukoski and Sodankylä Groups (). The mean distance to granitoids within a 2500 m neighbourhood was then subtracted from the original proximity grid, resulting in a grid illustrating the midpoint between the granitoids in the greenstone belt. The midpoint is interpreted as a vector of favourable fluid flow from convergent stress regimes of pure shear between closely spaced granitoids to divergent stress regimes of simple shear in the greenstone belt ().
Figure 5 (a) Proximity to Sirkka Shear Zone (SSZ) combined with the distance to granitoid midpoints in the greenstone belt, as a measure of strain gradients. (b) Paleostress modelling. (c) Proximity to greenstone/sedimentary rock contacts, as used to define the regional zones of rheology contrast and hydrothermal fluid seals. The coarseness of the pattern probably relates to erosion of most of the sedimentary rocks post-gold mineralisation.
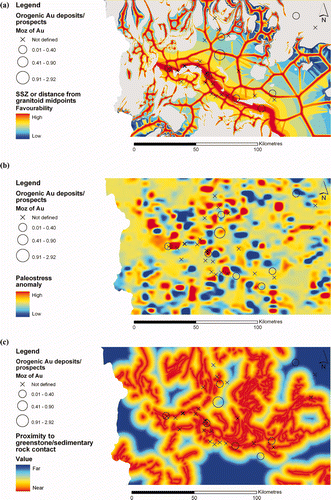
Geomechanical paleostress modelling
Geomechanical interpretation for the paleostress modelling was done at 1:100 000 scale following the principles described by Holyland & Ojala (Citation1997). Faults and lithological contacts were interpreted from 1:100 000 scale geophysical and 1:200 000 scale geological maps. Faults were further divided into first- and second-order faults and early thrust faults using their length, continuity and timing as the main criteria, and rock contacts to greenstone and granitoid contacts. Dominant rock type was used to define the material properties for the rock blocks between the faults and contacts. The rheologies of the rocks were approximated from the published geomechanical database (Clark Citation1966). Rock types were simplified in seven different rheologies in the order of competency: gabbro, granitoid, mafic volcanic rock, quartzite, ultramafic rocks, mica schist and fine-grained sedimentary rocks. Discontinuities were modelled in order of increasing stiffness: first-order faults, second-order faults, thrusts, greenstone contacts and granitoid contacts. The greenstone and granitoid contact stiffness were modelled a magnitude higher than the fault stiffness. The far-field stress orientation was interpreted to be broadly northeast – southwest during the gold mineralisation based on the broad geometry of greenstone belt and shear sense of mineralised faults and orientations veins. Stress boundaries were applied, and the vectors at maximum and minimum principal stresses were 100 and 70 MPa, respectively. The modelling was done using a distinct element code UDEC (Itasca Citation2004). Rocks and discontinuities were modelled as Mohr – Coulomb materials, and brittle – ductile material properties were used as the metamorphic grade of the rocks is greenschist to low amphibolite facies. The Au mineralisation was interpreted to be late in the structural history and to be spatially related to brittle and brittle – ductile faults. The results of the modelling are shown in .
Fluid seals
Proximity to the contact zone lithologies between greenstone belt and overlying quartzitic sedimentary units () was used as a proxy for a regional rheology contrast, which could have acted as a regional seal on the orogenic hydrothermal system (Groves et al. Citation2000). Such seals are evident in the Abitibi Belt, Canada, and Eastern Goldfields Province, Western Australia (Phillips et al. Citation1996). They are required to maintain high fluid pressures in potential trap sites.
Spatial association between parameter layers and training sites
The spatial association between training sites and the data layers described above was quantified by using the weights-of-evidence method (Bonham-Carter Citation1994; Raines et al. Citation2000). Aggregating features into classes enables us to spot patterns in the spatial data more easily. Thus, the data layers were all reclassified into an appropriate number of classes in each case using either standard classification schemes of GIS software or a manual classification based on expert knowledge. The class breaks are defined in , . A pair of weights was calculated for each map class of the input layers. A positive weight (W+) was calculated for the presence of each parameter at the training site, and a negative weight (W−) for absence of that parameter. The weights are a function of density of the training sites within a map class and the area of the corresponding map class. The magnitude of the weights depends on this measured spatial association between the parameter layers and the training sites. A weight of zero indicates no spatial association between the training sites and a particular parameter. The layers were reclassified into binary patterns for the model integration by using the maximum contrast value, which is the arithmetic difference between the weights in the cumulative weights calculation. The confidence of the weights calculation was estimated by calculating the Studentised contrast (the ratio and standard deviation of the contrast), which is the measure of the certainty that the contrast is not zero (Bonham-Carter Citation1994). Studentised contrast values above 1.96, which is about a 97.5% confidence level, were considered acceptable (Raines Citation1999). The lowest confidence was detected for the density of geological contacts. The results of weights calculations are reported in , for empirical and conceptual datasets, respectively, and the data layers are discussed in this order below.
Table 5 Spatial association between empirical data map classes and training sites.
Table 6 Spatial association between the conceptual data map classes and the training sites.
Aeromagnetic residual grid
The aeromagnetic residual floating-point grid was reclassified in GIS into an integer grid with 15 classes using the quantile method, where each class contains an equal number of features, and cumulative ascending weights were calculated for these classes (). The grid cell size was 50×50 m, and the grid covers 99.9% of the study area. The 0.1% missing data are due to minor differences between the study area grid and the flight area on the border between Finland and Sweden. The maximum contrast value falls into the first map class (<−243 nT), which covers only 6.9% of the study area and includes nine of the training sites. This makes the pattern defining the magnetic lows very small and thus highly significant.
Apparent resistivity
The apparent resistivity grid was classified into nine classes manually, and cumulative ascending weights were calculated for these classes (). The grid cell size is 50×50 m, and the grid covers 99.4% of the study area. The maximum contrast value is also in the first map class (<−110 Ωm), and the confidence values are relatively high. The optimal contour of the resistivity covers 11% of the total area and captures 19 training sites, and hence is highly significant.
Gravity gradient
Cumulative descending weights for the 20 classes were calculated for the gravity gradient grid (). The grid cell size is 500×500 m. Unfortunately, the gravity dataset had missing data covering 34% of the total study area. The maximum contrast break is in class 5 (gradient > 1.19 mgal/km2). The relatively large enclosing pattern size of 64% of the total area captures altogether 21 training sites, and so this parameter defines the less significant areas well but the significant areas only moderately well.
Till geochemistry
The combined geochemical evidence layer summarises the geochemical anomalies of As, Au, Cu, Fe, Ni and Te on a common scale from 0 to 1, classified into 10 classes (Nykänen & Salmirinne Citation2007). The grid cell size for the combined grid as well as for the original interpolations was 200×200 m. A cumulative descending weight calculation results in the maximum contrast in class 5 (Fuzzy membership value > 0.4) covering 44% of the total area and including 27 training sites (). This parameter is again less significant than the residual aeromagnetic and resistivity layers.
Density of contacts—geological complexity
The first layer derived from the geological map is the density of contacts as a proxy for geological complexity. The grid cell size is 50×50 m. This layer is the poorest predictor of all the used evidence layers, as it does not pass the requirement of confidence. Classes from 6 to 16 (density >0.4 m/km2) are classified as enclosing 71% of the total study area and capturing 28 training sites (). The failure of this layer as a predictor of gold mineralisation must be, at least in part, due to the poor definition of geological contacts at the scale of deposit control on the 1:200 000 scale map. In other words, the complexity defined by the GIS analysis is at a scale poorer than that controlling the siting of the gold deposits. Thus, this data layer was rejected from the integration.
Divergent/convergent regimes and Sirkka Shear Zone
This evidence layer is a fuzzy combination of two proximity layers: divergent/convergent regimes and Sirkka Shear Zone in a 100×100 m grid cell size. The resulting combination was reclassified into 10 classes in equal intervals. Cumulative descending weight calculation results in a maximum contrast in class 7 (Fuzzy membership value >0.5) (). This encloses 40% of the total area and captures 30 training sites. As such, it is the most effective evidence layer, suggesting that regional-scale stress and resulting strain heterogeneities were the main control in siting of orogenic-gold deposits in the Central Lapland Greenstone Belt.
Geomechanical paleostress anomalies
The paleostress modelling produced spotty and spatially limited anomalies on a map with 500×500 m grid cell size. This was reclassified into 13 classes by the standard deviation method and maximum contrast after the cumulative descending weights calculation is found in class 11 (modelled minimum principal stress values <70.05 MPa), resulting in an extremely small anomaly size of only 3.3% of the total study area (). This captures only four training sites. However, the layer is relatively significant, since the enclosing area is so small. In other words, the paleostress anomaly map is a good positive indicator but does not give a good definition of the areas that are not prospective for orogenic gold.
Regional seal/rheology contrast
Another derivative of the 1:200 000 scale bedrock map was proximity to greenstone/sedimentary rock contacts being used as a proxy to indicate the regional zones of rheology contrast and hydrothermal fluid seals. The proximity grid with 50×50 m cell size was reclassified into 16 classes by using the quantile method prior to quantifying the spatial association between the training sites and the evidence layers. Classes 1 – 10 (distance <6299 m) enclose 72% of the total area and capture 32 training sites (). This parameter is not very effective due to the relatively coarse enclosing pattern size, which probably relates to erosion of the sedimentary rock units after the gold mineralisation event.
Data integration into the prospectivity map
As described above, the spatial association between the training sites and the evidential data layers was quantified by using the weights-of-evidence method (Bonham-Carter Citation1994; Raines et al. Citation2000). The layers were reclassified into binary patterns using the maximum contrast value in the cumulative weights calculation, and then the logistic regression method was used to combine the evidence layers (Agterberg Citation1989; Agterberg et al. Citation1990, Citation1993) into a single prospectivity map for orogenic-gold deposits. The weights-of-evidence method was used in a comparative analysis. To ensure that the total areas of all the input layers were equal, the missing data area was dealt with as zero weight in weights-of-evidence modelling and area-weighted mean value in logistic regression modelling. The use of logistic regression is more robust due to strong conditional dependencies among the evidential data layers, as shown by the Agterberg & Cheng (Citation2002) conditional independence test, which indicates that the probability of this model including seven evidence layers not being conditionally independent is 99.3% (with T − n / STD = 2.438: where n is the observed number of training points, T is the expected number of training points, and STD is the standard deviation of T). For the empirical weights-of-evidence model of Nykänen & Salmirinne (Citation2007), the above test indicates that the probability that this model is not conditionally independent is 83.5% (with T − n / STD = 0.972), which is a slightly lower conditional dependency problem compared with the model described in the present paper. The consequences of the conditional dependencies are evident on the graph in , where the posterior probabilities from both models are plotted against the cumulative area. When using all seven data layers, the weights-of-evidence model tends to result in higher posterior probability values than the logistic regression model, in the range where posterior probability is above prior probability. On the contrary, below the prior probability, the posterior probabilities of the weights-of-evidence model are lower than the logistic regression model. This phenomenon is assumed to be due to the effects of conditional dependencies. The values appear to be similar in the middle section, the crossover of the values being somewhat close to prior probability. For comparison, the same graph is shown for purely empirical models with only four evidence layers. In this case, the weights-of-evidence and logistic regression models result in almost identical posterior probability values, since the effects of conditional dependencies are only minor. It is noticeable that the highest probability class in all models occupies a relatively small area, <1% of the total study area, giving a remarkable reduction in the area of interest for exploration.
Figure 6 Posterior probabilities of weights-of-evidence and logistic regression models on logarithmic scale plotted against cumulative area explaining the classification, which is based on natural breaks in the data. These class breaks correspond to the classes Very Low, Low, Moderate, High and Very High, reported in the final prospectivity maps. (a) Combined empirical/conceptual model with added geological evidence. The graph illustrates the subtle differences between the posterior probability values of weights-of-evidence and logistic regression models. (b) Pure empirical model from Nykänen & Salmirinne (Citation2007) using only geophysical and geochemical evidence. The graph clearly demonstrates that the weights-of-evidence and logistic regression models are almost identical.
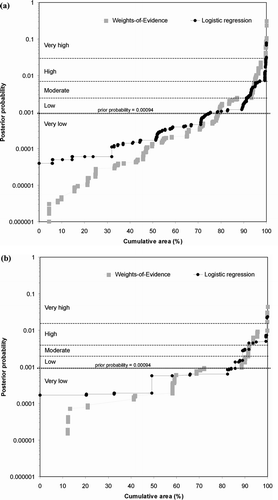
The final prospectivity maps are shown in for both the weights-of-evidence model and the logistic regression model. The posterior probabilities are classified into five classes using the natural breaks method, in which classes are based on natural groupings inherent in the data. The GIS software identifies break points by picking the class breaks that best group similar values and maximise the differences between classes. The class breaks were then manually adjusted by using the graphs in , where posterior probability is plotted against cumulative area. These graphs can thus also be used to divide the data into groups. The features are divided into classes whose boundaries are set where there are relatively large variations in the data values. The locations of the training sites and the validation sites are also shown on the maps. Agterberg & Bonham-Carter (Citation2005) discussed and summarised several validation procedures to measure the performance of mineral potential maps. In the current study, the validation sites are other known orogenic-gold prospects, which were not used in training, since they were not included in the FinGOLD database (Eilu Citation1999) when this project was started. Validation of the modelling was accomplished by quantifying the spatial association between the 11 validation sites () and the final posterior probability maps (). The results are summarised in , where the mean posterior probability within a 200 m radius area around each validation site is shown as a histogram. Most of the validation sites occupy areas within the posterior probability value higher than the prior probability of the model. In another validation process described by Nykänen & Salmirinne (Citation2007), the validity of the modelling was tested by calculating 23 successive models and leaving out one of the training sites at each iteration, and then comparing the resulting posterior probabilities at the location of the training sites between the original model and the subsequent validation models. This test suggests that excluding any deposit from the model, except the Kutuvuoma deposit would not give any significant deviation from the model that included all the training sites. Nykänen & Salmirinne (Citation2007) explained the different behaviour of Kutuvuoma as an artefact driven by the generalised gravity pattern including all the other training sites except Kutuvuoma.
Figure 7 Final prospectivity maps for orogenic gold in the Central Lapland Greenstone Belt using combined empirical and conceptual data. (a) Logistic regression model and (b) weights-of-evidence model. The class breaks are explained in . The numbers refer to the validation sites in .
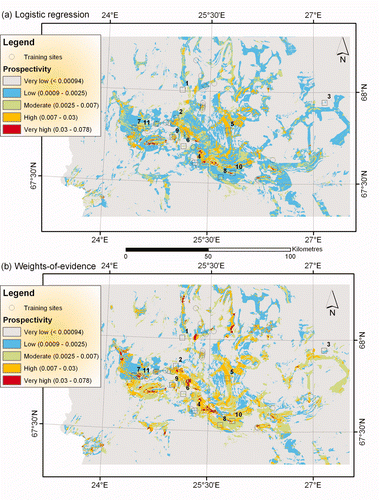
Figure 8 Posterior probabilities from the three models associated together with the validation sites from and . Prior probability = 0.00094.
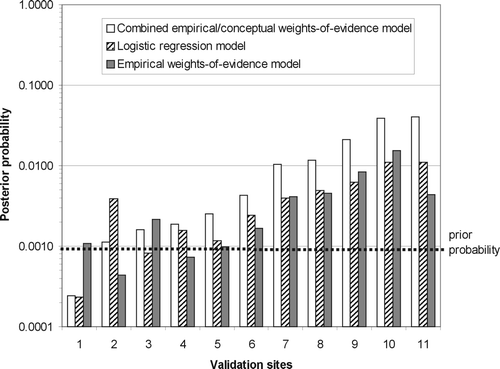
The spatial association between the training sites and the final prospectivity maps was also calculated using both cumulative descending and categorical methods. The results of the cumulative descending weights are given together with the evidential data layers in . As expected, the contrast and confidence values for the model grids are remarkably higher than those of the evidential data layers. The empirical weights-of-evidence model (Nykänen & Salmirinne Citation2007) and combined empirical/conceptual weights-of-evidence model presented in this paper are close to each other in terms of weights and contrast values. In addition, the categorical weights for each individual map class in the resulting prospectivity map were calculated separately (, ). The logistic regression model is less effective in terms of capturing training sites within the two highest probability classes (9 of 35), compared with 19 out of the 35 training sites in the two weights-of-evidence models. The area under the curve in , where the relative cumulative area is plotted against the relative cumulative number of training sites, was also calculated to test the efficiency of classification of the models following the method of Chung & Fabbri (Citation2003). This test demonstrates that the addition of the new conceptual data layers improved the efficiency so that more points are captured within a smaller area when compared with the empirical weights-of-evidence model with fewer data layers. The difference is not large but noticeable. The logistic regression model and the combined empirical/conceptual weights-of-evidence model do not differ from each other remarkably.
Figure 9 Efficiency curves according to Chung & Fabbri (Citation2003). The area under curve defines the efficiency of classification. The efficiency in percentage of area is 85% for the conceptual weights-of-evidence model, 84% for the logistic regression model and 82% for the empirical weights-of-evidence model of Nykänen & Salmirinne (Citation2007).
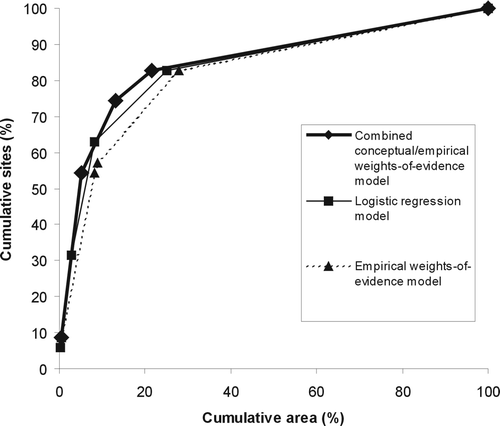
Table 7 Weights (W+ and W−), contrast and confidence for the evidential data layers used in the weights-of-evidence model and for the final prospectivity maps.
Table 8 Categorical weights calculated for the five prospectivity classes in the resulting prospectivity maps in Figure 12 using the training sites.
Table 9 Categorical weights calculated for the five prospectivity classes in the resulting prospectivity maps in Figure 12 using the validation sites.
Summary and conclusions
The aim of this research was to test the effect of combining empirical geophysical and geochemical evidence with conceptual derivatives of a geological map in creating a prospectivity analysis based on a targeting model for orogenic-gold deposits in a Paleoproterozoic greenstone belt in the Fennoscandian Shield. Two empirical methods, weights-of-evidence and logistic regression, were used to complete the data integration. The task was conducted in four main steps. In step 1, evidence layers were selected based on the targeting model, and the key input layers (the anomaly maps, etc.) were created using expert knowledge. In step 2, spatial association between the evidence layers and the training sites was quantified, and the evidence was reclassified into binary patterns according to maximum spatial association. In step 3, binary evidence layers were integrated into the single prospectivity map using weights-of-evidence and logistic regression methods, and model results evaluated using another set of known deposits as validation sites in step 4.
Comparison between results for the original empirical model (Nykänen & Salmirinne Citation2007) and the conceptually enhanced models described here indicates a subtle improvement in the predictive capacity of the latter prospectivity analysis in terms of model effectiveness and confidence, especially when the logistic regression technique was used. It is concluded that, given good-quality bedrock geology maps, spatial modelling techniques are capable of incorporating and integrating geological interpretations with empirical geophysical and geochemical datasets in order to improve the predictive capacity of prospectivity maps resulting from the GIS-based modelling. The study also suggests that higher-quality geological and gravity data at a higher scale would further enhance the prospectivity analysis by increasing the number of critical parameters that could be incorporated into the modelling.
Acknowledgements
The authors would like to thank Gary Raines from the US Geological Survey, Jeff Harris from the Geological Survey of Canada and an anonymous reviewer for their thorough reviews that considerably improved the paper.
References
- Agterberg , F. P. 1974 . Automatic contouring of geological maps to detect target areas for mineral exploration . Mathematical Geology , 6 : 373 – 395 .
- Agterberg , F. P. 1989 . LOGDIA; Fortran 77 program for logistic regression with diagnostics: statistical methods for resource appraisal . Computers & Geosciences , 15 : 599 – 614 .
- Agterberg , F. P. and Bonham-Carter , G. F. 2005 . Measuring the performance of mineral-potential maps . Natural Resources Research , 14 : 1 – 17 .
- Agterberg , F. P. , Bonham-Carter , G. F. , Cheng , Q. and Wright , D. F. 1993 . “ Weights of evidence modeling and weighted logistic regression for mineral potential mapping ” . In Computers in Geology, 25 Years of Progress , Edited by: Davis , J. C. and Herzfeld , U. C. 13 – 32 . Oxford : Oxford University Press .
- Agterberg , F. P. , Bonham-Carter , G. F. and Wright , D. F. 1990 . “ Statistical pattern integration for mineral exploration ” . In Computer Applications in Resource Estimation. Prediction and Assessment for Metals and Petroleum , Edited by: Gaal , G. and Merriam , D. 1 – 21 . Oxford : Pergamon Press .
- Agterberg , F. P. and Cheng , Q. 2002 . Conditional independence test for weights-of-evidence modelling . Natural Resources Research , 11 : 249 – 255 .
- Airo , M.-L. 2002 . Aeromagnetic and aeroradiometric response to hydrothermal alteration . Surveys in Geophysics , 23 : 273 – 302 .
- Airo M-L. (Editor) 2005. Aerogeophysics in Finland 1972 – 2004: methods, system characteristics and applications. Geological Survey of Finland Special Paper 39
- Airo , M.-L. and Mertanen , S. 2001 . “ Magnetic signatures related to Precambrian greenstone-hosted Au mineralizations, northern Fennoscandia ” . In IAGA-IASPEI Joint Scientific Assembly, 19 – 31 August 2001, Hanoi, Vietnam, Abstracts 263
- Bonham-Carter , G. F. 1994 . Geographic Information Systems for Geoscientists—Modelling with GIS , New York : Pergamon Press . (Computer Methods in the Geosciences 13).
- Bougrain , L. , Gonzalez , M. , Bouchot , V. , Cassard , D. , Lips , A. L. W. , Alexandre , F. , Stein , G. and Kouda , R. 2003 . Knowledge recovery for continental-scale mineral exploration by neural networks . Natural Resources Research , 12 : 173 – 181 .
- Brown , W. , Groves , D. , Gedeon , T. and Kouda , R. 2003 . Use of fuzzy membership input layers to combine subjective geological knowledge and empirical data in a neural network method for mineral-potential mapping . Natural Resources Research , 12 : 183 – 200 .
- Brown , W. M. , Gedeon , T. D. , Groves , D. I. and Barnes , R. G. 2000 . Artificial neural networks: a new method for mineral prospectivity mapping . Australian Journal of Earth Sciences , 47 : 757 – 770 .
- Carranza , E. J. M. 2004 . Weights of evidence modeling of mineral potential: a case study using small number of prospects, Abra, Philippines . Natural Resources Research , 13 : 173 – 187 .
- Carranza , E. J. M. and Hale , M. 2000 . Geologically constrained probabilistic mapping of gold potential, Bauio District, Philippines . Natural Resources Research , 9 : 237 – 253 .
- Carranza , E. J. M. and Hale , M. 2001 . Logistic regression for geologically constrained mapping of gold potential, Baguio district, Philippines . Exploration and Mining Geology , 10 : 165 – 175 .
- Cheng , Q. 2004 . Application of weights of evidence method for assessment of flowing wells in the greater Toronto Area, Canada . Natural Resources Research , 13 : 77 – 86 .
- Chung , C. F. and Agterberg , F. P. 1980 . Regression models for estimating mineral resources from geological map data . Mathematical Geology , 12 : 472 – 488 .
- Chung , C. F. and Fabbri , A. G. 2003 . Validation of spatial prediction models for landslide hazard mapping . Natural Hazards , 30 : 451 – 472 .
- Clark , S. P. 1966 . Handbook of Physical Constants . Geological Society of America Memoir , 97
- Eilu , P. 1999 . FinGOLD—a public database on gold deposits in Finland . Geological Survey of Finland Report of Investigation , 146 Online <http://en.gtk.fi/ExplorationFinland/Commodities/Gold/gtk_gold_map>
- Eilu , P. , Sorjonen-Ward , P. , Nurmi , P. and Niiranen , T. 2003 . A review of gold mineralization styles in Finland . Economic Geology , 98 : 1329 – 1353 .
- Eilu , P. and Weihed , P. 2005 . Fennoscandian Shield—orogenic gold deposits . Ore Geology Reviews , 27 : 326 – 327 .
- Goldfarb , R. J. , Baker , T. , Dubé , B. , Groves , D. I. , Hart , C. J. R. and Gosselin , P. Distribution, character, and genesis of gold deposits in metamorphic terranes . Economic Geology 100th Anniversary Volume . pp. 407 – 450 .
- Groves , D. I. , Goldfarb , R. J. , Gebre-Mariam , M. , Hagemann , S. G. , Robert , F. and Arne , D. C. 1998 . Orogenic gold deposits; a proposed classification in the context of their crustal distribution and relationship to other gold deposit types: mesothermal gold mineralization in space and time . Ore Geology Reviews , 13 : 7 – 27 .
- Groves , D. I. , Goldfarb , R. J. , Knox-Robinson , C. M. , Ojala , J. , Gardoll , S. , Yun , G. Y. and Holyland , P. 2000 . Late kinematic timing of orogenic gold deposits and significance for computer-based exploration techniques with emphasis on the Yilgarn block, Western Australia . Ore Geology Reviews , 17 : 1 – 38 .
- Harris , D. and Pan , G. 1999 . Mineral favorability mapping; a comparison of artificial neural networks, logistic regression, and discriminant analysis . Natural Resources Research , 8 : 9 – 109 .
- Harris , J. R. and Sanborn-Barrie , M. 2006 . “ Mineral potential mapping: examples from the Red Lake Greenstone Belt, Northwest Ontario ” . In GIS for the Earth Sciences Edited by: Harris , J. R. 1 – 21 . Geological Association of Canada Special Publication 44
- Harris , J. R. , Wilkinson , L. and Grunsky , E. C. 2000 . Effective use and interpretation of lithogeochemical data in regional mineral exploration programs: application of Geographic Information Systems (GIS) technology . Ore Geology Reviews , 16 : 107 – 143 .
- Harris , J. R. , Wilkinson , L. , Heather , K. , Fumerton , S. , Bernier , M. A. , Ayer , J. and Dahn , R. 2001 . Application of GIS processing techniques for producing mineral prospectivity maps; a case study: mesothermal au in the Swayze greenstone belt, Ontario, Canada . Natural Resources Research , 10 : 91 – 124 .
- Hodgson , C. J. 1993 . “ Mesothermal lode-gold deposits ” . In Mineral Deposit Modeling Edited by: Kirkham , R. V. , Sinclair , W. D. , Thorpe , R. I. and Duke , J. M. 635 – 678 . Geological Association of Canada Special Paper 40
- Hodkiewicz , P. F. , Weinberg , R. F. , Gardoll , S. J. and Groves , D. I. 2005 . Complexity gradients in the Yilgarn Craton: fundamental controls on crustal-scale fluid flow and the formation of world-class orogenic gold deposits . Australian Journal of Earth Sciences , 52 : 831 – 841 .
- Holyland , P. W. and Ojala , V. J. 1997 . Computer aided structural targeting: two and three-dimensional stress mapping . Australian Journal of Earth Sciences , 44 : 421 – 432 .
- Hronsky , J. M. A. and Groves , D. I. 2008 . Science of targeting: definition, strategies, targeting and performance measurement . Australian Journal of Earth Sciences , 55 : 3 – 12 .
- Itasca . 2004 . UDEC—Universal Distinct Element Code User's Guide , Minneapolis : Itasca Consulting Group .
- Jamieson , R. A. , Beaumont , C. , Fullsack , P. and Lee , B. 1998 . “ Barrovian regional metamorphism: where's the heat? ” . In What Drives Metamorphism and Metamorphic Reactions? Edited by: Treloar , P. J. and O'Brien , P. J. 23 – 51 . Geological Society of London Special Publication 138, 23 – 51
- Kääriäinen , J. and Mäkinen , J. 1997 . The 1979 – 1996 gravity survey and results of the gravity survey of Finland 1945 – 1996 . Publications of the Finnish Geodetic Institute , 125
- Kapo , K. E. and Burton , G. A. Jr . 2006 . A geographic information systems-based, weights-of-evidence approach for diagnosing aquatic ecosystem impairment . Environmental Toxicology and Chemistry , 25 : 2237 – 2249 .
- Koike , K. , Matsuda , S. , Suzuki , T. and Ohmi , M. 2002 . Neural-network-based estimation of principal metal contents in the Hokuroku District, Northern Japan, for exploring Kuroko-type deposits . Natural Resources Research , 11 : 135 – 156 .
- Kontas , E. 1981 . Rapid determination of gold by flameless atomic absorption spectrometry in the ppb and ppm ranges without organic solvent extraction . Atomic Spectroscopy , 2 : 59 – 61 .
- Kontas , E. , Niskavaara , H. and Virtasalo , J. 1990 . Gold, palladium and tellurium in South African, Chinese and Japanese geological reference samples . Geostandards Newsletter , 14 : 477 – 478 .
- Korkalo , T. 2006 . Gold and copper deposits in Central Lapland, Northern Finland, with special reference to their exploration and exploitation . Acta Universitatis Ouluensis. A Scientiaererum Naturalium , 461
- Lehtonen , M. , Airo , M.-L. , Eilu , P. , Hanski , E. , Kortelainen , V. , Lanne , E. , Manninen , T. , Rastas , P. , Räsänen , J. and Virransalo , P. 1998 . The stratigraphy, petrology and geochemistry of the Kittilä greenstone area, northern Finland. A report of the Lapland Volcanite Project . Geological Survey of Finland Report of Investigation , 140 (in Finnish with English summary)
- Mihalasky , M. J. and Bonham-Carter , G. F. 2001 . Lithodiversity and its spatial association with metallic mineral sites, Great Basin of Nevada . Natural Resources Research , 10 : 209 – 226 .
- Nykänen , V. and Salmirinne , H. 2007 . “ Prospectivity analysis of gold using regional geophysical and geochemical data from the Central Lapland Greenstone Belt, Finland ” . In Gold in the Central Lapland Greenstone Belt, Finland Edited by: Ojala , V. J. 235 – 253 . Geological Survey of Finland Special Paper 44
- Paganelli , F. , Richards , J. P. and Grunsky , E. C. 2002 . Integration of structural, gravity, and magnetic data using the weights of evidence method as a tool for kimberlite exploration in the Buffalo Head Hills, Northern Central Alberta, Canada . Natural Resources Research , 11 : 219 – 236 .
- Peltoniemi , M. 1998 . Depth penetration of frequency-domain airborne electromagnetics in resistive terrains . Exploration Geophysics , 29 : 12 – 15 .
- Phillips , G. N. , Groves , D. I. and Kerrich , R. 1996 . Factors in the formation of the giant Kalgoorlie gold deposit . Ore Geology Reviews , 10 : 297 – 317 .
- Poikonen , A. , Sulkanen , K. , Oksama , M. and Suppala , I. 1998 . Novel dual frequency fixed-wing airborne EM system of Geological Survey of Finland (GTK) . Exploration Geophysics , 29 : 46 – 51 .
- Porwal , A. , Carranza , E. J. M. and Hale , M. 2004 . A hybrid neuro-fuzzy model for mineral potential mapping . Mathematical Geology , 36 : 803 – 826 .
- Porwal , A. , Carranza , E. J. M. , Hale , M. and Kouda , R. 2003 . Artificial neural networks for mineral-potential mapping; a case study from Aravalli Province, Western India . Natural Resources Research , 12 : 155 – 171 .
- Raines , G. L. 1999 . Evaluation of weights of evidence to predict epithermal-gold deposits in the Great Basin of the Western United States . Natural Resources Research , 8 : 257 – 276 .
- Raines , G. L. and Bonham-Carter , G. F. 2006 . “ Exploratory spatial modelling: demonstration for Carlin-type deposits, central Nevada, USA, using Arc-SDM ” . In GIS for the Earth Sciences Edited by: Harris , J. R. 23 – 52 . Geological Association of Canada Special Publication 44
- Raines , G. L. , Bonham-Carter , G. F. and Kemp , L. D. 2000 . Predictive probabilistic modelling using Arcview GIS . ArcUser , 3 : 45 – 48 .
- Raines , G. L. and Mihalasky , M. J. 2002 . A reconnaissance method for delineation of tracts for regional-scale mineral-resource assessment based on geologic-map data . Natural Resources Research , 11 : 241 – 248 .
- Reddy , R. K. T. , Agterberg , F. P. and Bonham-Carter , G. F. Application of GIS-based logistic models to base-metal potential mapping in Snow Lake area, Manitoba . Proceedings, 3rd International Conference on Geographic Information Systems . Ottawa : Canadian Institute of Surveying and Mapping . pp. 607 – 619
- Robinson , G. R. , Kapo , K. E. and Raines , G. L. 2004 . A GIS analysis to evaluate areas suitable for crushed stone aggregate quarries in New England, USA . Natural Resources Research , 13 : 143 – 159 .
- Sahoo , N. R. and Pandalai , H. S. 1999 . Integration of sparse geologic information in gold targeting using logistic regression analysis in the Hutti-Maski schist belt, Raichur, Karnataka, India—a case study . Natural Resources Research , 8 : 233 – 250 .
- Salminen R. ed. 1995. Geochemical mapping in Finland in 1982 – 1994. Geological Survey of Finland Report of Investigation 130 (in Finnish with English summary)
- Venkataraman , G. , Babu Madhavan , B. , Ratha , D. S. , Antony , J. P. , Goyal , R. S. , Banglani , S. and Sinha Roy , S. 2000 . Spatial modelling for base-metal mineral exploration through integration of geological data sets . Natural Resources Research , 9 : 27 – 42 .
- Weihed , P. , Arndt , N. , Billström , K. , Duchesne , J.-C. , Eilu , P. , Martinsson , O. , Papunen , H. and Lahtinen , R. 2005 . Precambrian geodynamics and ore formation: the Fennoscandian Shield . Ore Geology Reviews , 27 : 273 – 322 .