Abstract
This article presents a formal model of the knowledge representation scheme based on the fuzzy Petri net (FPN) theory. The model is represented as a 13-tuple consisting of the components of the FPN, two functions that give semantic meanings to the scheme and a set of contradictions. For the scheme, called the knowledge representation scheme based on the fuzzy Petri nets theory (KRFPN) the fuzzy inheritance and fuzzy recognition-inference procedures based on the dynamical properties of the FPN, are described in detail. The upper-time complexity of both the proposed inference algorithms is O(nm), where n is the number of places (concepts) and m is the number of transitions (relations) in the scheme. Illustrative examples of the fuzzy inheritance and the fuzzy recognition algorithms for the knowledge base, designed by the KRFPN, are given.
INTRODUCTION
The crucial component of an intelligent agent is its knowledge base. Informally, a knowledge base is an abstract representation of a working environment or a world in which the agent (or agents) has to solve tasks. It contains collections of (uncertain) facts and objects (in an abstract sense) and their relationships; vocabulary definitions; disjunctive facts, and constrains; descriptions of typical situations and the agent's behavior; the rules of the world; common-sense knowledge; decision rules; hypotheses; heuristics and problem-solving methods and procedures; knowledge about states, the actions, motivations, and goals of the agent; and knowledge about knowledge (meta-knowledge).
For more than 30 years one of the central problems of artificial intelligence is the development of a sufficiently precise and efficacious notation for the knowledge representation and reasoning, called a knowledge representation scheme (KRS) (Zeigler Citation1987; Garcia and Chien Citation1991; Aiello and Nardi Citation1991). The major classes of KRS, according to the taxonomy based on object-relationship, the true assertion about states and state-transformations criteria, are network (Quillian Citation1967; Findler Citation1979), logical (Israel and Beranek Citation1983; Dahl Citation1983; Bobrow Citation1985), and procedural schemes (Newell and Simon Citation1972), as well as schemes based on the frame theory (Minsky Citation1975).
In many real-world tasks, knowledge is based on imprecise, uncertain, incomplete, or even vague, and/or fuzzy information, and agents have to deal with the information in such a form. Therefore, in order to properly represent real-world knowledge and support vague, uncertain, and fuzzy-knowledge representation, reasoning, learning, and decision-making, different knowledge schemes were developed: scheme based on subjective Bayesian probabilities (so-called Prospector approach) (Duda, Gaschnig, and Hart Citation1979), rule-based system with certainty factors (MYCIN approach) (Buchanan and Shortliffe Citation1984), Bayesian or belief networks (Pearl Citation1988), schemes based on Dempster-Shafer calculus (Shafer Citation1976), and fuzzy logic-based schemes (Zadeh Citation1983). Among knowledge representation schemes that support uncertain and fuzzy knowledge representation and reasoning, there is a class of schemes based on the theory of fuzzy Petri nets (FPNs) (Cardoso and Camargo Citation1999): Looney (Citation1988) and Chen, Ke, and Chang (Citation1990) proposed FPNs for rule-based decision-making; Scarpelli, Gomide, and Yager (Citation1996) described a reasoning algorithm for a high-level FPN; Chen (Citation2002) introduced a weight FPN model for rule-based systems; Li and Lara-Rosano (Citation2000) proposed a model based on an adaptive FPN, which is implemented for knowledge inference, but it also has a learning ability; Looney and Liang (Citation2003) proposed the fuzzy belief Petri nets (PNs) as a combination of the bi-directional fuzzy propagation of the fuzzy belief network and modeling flexibility of the FPN; Lee, Liu, and Chaing (Citation2003) introduced a reasoning algorithm based on possibilistic Petri nets as a mechanism that mimics human inference; Canales, Liand, and Yu (Citation2006) described a method of fuzzy-knowledge learning based on an adaptive FPN; Ha, Li, Li, and Wang (2005) described knowledge representation by weighted fuzzy production rules and inference with generalized FPN; and Guo-Yan (Citation2006) proposed a hybrid of the PN and the fuzzy PN to support an inference procedure for the natural extension of fuzzy expert systems. Shen (Citation2006) presented the knowledge representation scheme based on a high-level FPN for modelling fuzzy IF-THEN and IF-THEN-ELSE rules. Based on the high-level FPN model, an efficient algorithm is proposed to automatically reason about imprecise and fuzzy information.
The main inference procedures, as the act of automatic reasoning from factual knowledge, in the network-based knowledge representation schemas are: inheritance, intersection search, and recognition. Inheritance is a form of reasoning that allows an agent to infer the properties of a concept on the basis of the properties of its ancestors in the network hierarchical structure (Touretzky Citation1986). An inference procedure, called the intersection search (Quillian Citation1967), allows relationships to be found among facts by “spreading activities” in semantic networks. The recognition (Shastri Citation1988) is the dual of the inheritance problem and it can be viewed as a general form of pattern-matching.
In this article, the original inference procedures—inheritance and recognition—for a network-based fuzzy knowledge representation scheme, called KRFPN, based on the fuzzy Petri net theory, are proposed.
A KNOWLEDGE REPRESENTATION SCHEME BASED ON FUZZY PETRI NETS
A network-based fuzzy knowledge representation scheme called KRFPN uses the concepts of fuzzy Petri net theory to represent vague and/or fuzzy information obtained from modelled real-word situations. In this section, we first define a marked FPN, describe the execution of an FPN, and then introduce the graphical representation of an FPN. After that, by using additional components and functions that provide semantic interpretations, we introduce the formal definition of the knowledge representation scheme based on the fuzzy Petri nets theory (KRFPN).
A Fuzzy Petri Net
A marked fuzzy Petri net structure can be defined as 10-tuple:
-
P = {p1, p2,…, pn} is a finite set of places,
-
T = {t1, t2,…, tm} is a finite set of transitions,
-
P ∩ T = Ø,
-
I: T → P∞ is an input function, a mapping from transitions to bags of places,
-
O: T → P∞ is an output function, a mapping from transitions to bags of places,
-
M = {m1, m2,…, mr}, 1 ≤ r < ∞, is a set of tokens,
-
Ω: P → 𝒫(M) is a mapping, from P to 𝒫(M), called a distribution of tokens, where 𝒫(M) denotes the power set of M. Using Ω0 we denote the initial distribution of tokens in the places of a FPN.
-
μ: P → N is a marking, a mapping from places to non-negative integers, N. A mapping μ can be represented as an n-component vector μ = (μ1, μ2,…, μn), where n is a cardinality of the set P. Obviously, μ(pi) = μi, and μ(pi) denotes the number of tokens in the place pi. An initial marking is denoted by the vector μ 0.
-
f: T → [0, 1] is an association function, a mapping from transitions to real values between zero and one.
-
c: M → [0, 1] is an association function, a mapping from tokens to real values between zero and one.
The complete information about the token mi ∊ M is given by a pair (pj, c(mi)), where the first component specifies the location of the token, and the second one, its value. λ ∊ [0, 1] is a threshold value related to the firing of an FPN.
Graphical Representation of an FPN
A marked FPN can be represented by a bipartite directed multi-graph containing two types of nodes: places and transitions. Graphically, the circles represent places while the bars are used for transitions. The relationships, based on input and output functions, from places to transitions and transitions to places, are represented by directed arcs. Each arc is directed from an element of one set (P or T) to the element of another set (T or P).
A generalized FPN allows multiple arcs, according to the definitions of the input and output functions, where their co-domains are bags of places (P∞). In the case when co-domains are sets, and for all transitions ti, i = 1, 2,…, m, is |I(ti)| = |O(ti)| = 1, where |.| denotes the cardinality of a set, a multi-graph is transformed into a graph called a state machine (Peterson, Citation1981). In our case, it is a fuzzy state machine.
The tokens in marked FPN graphs are represented by labelled dots c(mi), where c(mi) denotes the value of the token.
Dynamical Properties of an FPN
Tokens give dynamical properties to an FPN, and they are used to define its execution, i.e., by firing an enabled transition tj, tokens are removed from its input places (elements in I(tj)). Simultaneously, new tokens are created and distributed to its output places (elements of O(tj)). In an FPN, a transition tj is enabled if each of its input places has at least as many tokens in it as arcs from the place to the transition and if the values of the tokens c(m l ), l = 1, 2,…exceed a threshold value λ ∊ [0, 1]. The number of tokens at the input and output places of the fired transition is changed in accordance with the basic definition of the original PN (Peterson, Citation1981). The new token value in the output place is obtained as c(m l )f(t i ), where c(m l ) is the value of the token at the input place pj ∊ I(ti) and f(ti) is the degree of truth of the relation assigned to the transition ti ∊ T. Figure illustrates the firing of the enabled transition of an FPN.
FIGURE 1 Firing an enabled transition. (a) Before firing; c(m l ) > λ; (b) after firing; c(mp) = c(m l )f(ti).
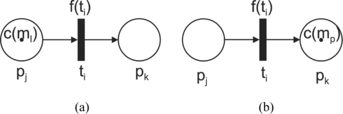
In general, if there are more tokens at an input place than arcs from the input place to the transition and if the value of each token exceeds the threshold value λ, then the selection of the token that takes the role in the firing is based on the maximum value c(mi). For example, in Figure (a), an input place pi has three tokens {c(m1) = 0.60, c(m2) = 0.20, c(m3) = 0.99}, and there is an arc directed to the transition tj with an associated value f(tj) = 0.80. The threshold value λ is 0.10. After firing the enabled transition tj, a new marking is shown in Figure b). The token (pj, c(m3)) takes place in firing the enabled transition tj and a new token c(m4) is generated at the output place pk. The value of the token m4 is 0.80·0.99 = 0.792. If there are two or more tokens with the same value c(mi); i = 1, 2,…, then the selection is based on random criteria.
Analogically, the generation of a new token at the output place pk in configurations where there is more than one arc connected to the transition tj with the output place, is based on the above-mentioned criteria, and it is illustrated in Figure . Figure (a) shows the situation before firing the enabled transition tj, while Figure (b) depicts the state after firing the transition tj. In this case, the tokens m4 and m5 are identical, i.e., the pair (pk, m4) is identical in terms of value to the pair (pk, m5).
FIGURE 3 Firing an enabled transition tj;(case O(tj) = {pk, pk}). (a) Before firing; (b) after firing the transition tj.
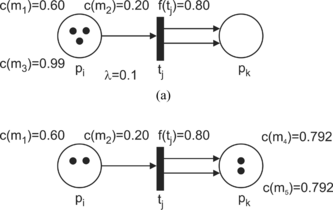
Note that the inheritance and recognition, as inference procedures defined for the proposed knowledge representation scheme, use the dynamical properties of a FPN.
Example 1
Let us illustrate an FPN graph and the execution of a fuzzy petri net, which is defined as a fuzzy state machine:
-
P = {p1, p2, p3, p4, p5, p6, p7},
-
T = {t1, t2, t3, t4, t5, t6},
-
I(t1) = {p1}, I(t2) = {p2}, I(t3) = {p4}, I(t4) = {p3}, I(t5) = {p3}, I(t6) = {p4},
-
O(t1) = {p3}, O(t2) = {p4}, O(t3) = {p5}, O(t4) = {p6}, O(t5) = {p7}, O(t6) = {p7},
-
M = {m1, m2, m3,…, mr},
-
Ω0 = {{m1, m2}, Ø, Ø, Ø, Ø, Ø, Ø},
-
μ 0 = (2, 0, 0, 0, 0, 0, 0},
-
f(t1) = 0.90, f(t2) = 0.99, f(t3) = 0.98, f(t4) = 1.0, f(t5) = 0.99, f(t6) = 1.0,
-
c(m1) = 0.90, c(m2) = 0.80,
-
λ = 0.02.
The FPN graph is shown in Figure .
According to the rule that defines enabled transitions, only the transition t1 is enabled because the number of tokens in its input place is two and there is only one arc connecting the place, and the values of the tokens exceed the threshold λ: c(m1), c(m2) > 0.02.
After firing the enabled transition t1, token m1 (based on the selection of the token that has the maximum value c(mi)), is removed from the input place p1 and simultaneously a new token m3 is generated at the place p3. The value of the token m3 is c(m3) = c(m1)·f(t1) = 0.90·0.90 = 0.81. The distribution of the tokens is now Ω 1 = ({m2}, Ø, {m3}, Ø, Ø, Ø, Ø, Ø} and the marking μ 1 = (1, 0, 1, 0, 0, 0, 0). Related to the new marking, there are now three enabled transitions in the FPN: t1, t4, and t5.
Formal Definition of the Knowledge Representation scheme KRFPN
The Knowledge Representation Scheme based on the Fuzzy Petri Nets theory is defined as 4-tuple:
-
α: P → D is a bijective function that maps a set of places onto a set of concepts D. The set of concepts D consists of the formal objects used for representing objects and facts from the agent's world. The elements from D = D1 ∪ D2 ∪ D3 are as follows: elements that denote classes or categories of objects and represent higher levels of abstraction (D1), elements corresponding to individual objects as instances of the classes (D2), and those elements representing the intrinsic properties of the concepts or values of these properties (D3).
-
β: T → Σ is a surjective function that associates a description of the relationship among facts and objects to every transition ti ∊ T;i = 1, 2,…, m, where m is a cardinality of a set T. The set Σ = Σ1 ∪ Σ2 ∪ Σ3 consists of elements corresponding to the relationships between the concepts used for partial ordering of the set of concepts (Σ1), the elements used to specify the types of properties to which values from subset D3 are assigned (Σ2), and the elements corresponding to the relationships between the concepts, but not used for hierarchical structuring (Σ3). For example, elements from Σ3 may be used to specify the spatial relations among the objects.
The functions α and β give a semantic interpretation to the scheme.
The semantic interpretation requires the introduction of a set of contradictions C. A set of contradictions C is a set of pairs of mutually contradictory relations and/or concepts: C = {(σi, σj),…, (σr, σs), (dk, dl),…, (dv, dz)}, were σu;u = i, j,…, r, s are from the set Σ, and dw;w = k, l,…, v, z are from the set D.
A set C contains elements corresponding to relations from Σ that mutually contradict each other, for example, is_a and is_not_a. Also, there are elements in the set D that are mutually contradictory if they are inherited for the same concept or object. For example, the object cannot simultaneously inherit properties such as “Quadruped” and “Biped.”
Both types of contradictions should be explicitly expressed in the KRFPN scheme.
The inverse function α−1: D → P, and the generalized inverse function β−1: Σ → τ; τ ⊆ T are defined in the KRFPN scheme.
Example 2
To illustrate the elementary KRFPN concepts, we use the FPN described in Example 1 as a component of the knowledge representation scheme, followed by the concepts needed for the semantic interpretation: functions α and β, and a set of contradictions C. The interpretation of the functions f and c is also needed. Example 2 is adopted from Touretzky (Citation1986).
For α: P → D we have:
-
α: p1 → Clyde,
-
α: p2 → Fred,
-
α: p3 → Elephant,
-
α: p4 → Human,
-
α: p5 → Two_legs,
-
α: p6 → Four_legs,
-
α: p7 → Mammal.
The function β is defined as follows:
-
β: t1 → is_a,
-
β: t2 → is_a,
-
β: t3 → has,
-
β: t4 → has,
-
β: t5 → is_a,
-
β: t6 → is_a.
A set of concepts D is D1 ∪ D2 ∪ D3, where a subset D1 = {Human, Elephant, Mammal} contains concepts that represent classes, D2 = {Clyde, Fred} is a subset consisting of concepts that correspond to individual objects as instances of the classes. A subset D3 = {Tw o − legs, Fou r − legs} defines properties of the concepts.
A set of relationships Σ = Σ1 ∪ Σ2 ∪ Σ3 consists of elements corresponding to the relationships between the concepts used for partial ordering of the set of concepts Σ1 = {i s − a}, the elements used to specify the types of properties to which values from subset D3 are assigned Σ2 = {has}, while the subset Σ3, that contains elements corresponding to the relationships between the concepts, but not used for hierarchical structuring, in our simple example is an empty set.
A set of contradictions, C = {(Tw o − legs, Fou r − legs)};Tw o − legs, Fou r − legs ∊ D, defines that an object cannot be simultaneously a biped and quadruped.
The values defined by the function f express our confidence or our belief in the truth of the relationships. For example, the value f(t4) = 1.0 defines our belief (or knowledge) that a human is always a mammal, while f(t1) = 0.90 expresses our belief that Clyde is an elephant. The value f(t1) = 0.90 can be interpreted as the statement that Clyde is a elephant is very true (see Table ).
TABLE 1 Truth Scales and the Corresponding Numerical Intervals
The values c(mi), i = 1, 2, may by interpreted as our assurance that we are really dealing with corresponding concepts. The threshold λ = 0.02 defines the relatively high sensitivity of the scheme related to the processes of inference. Figure shows the graphical representation of the simple knowledge base designed by the KRFPN.
Selection of Values f(t i ), c(m i ) and λ
A human's knowledge about facts from the real word is very often uncertain, ambiguous, and vague. The inference mechanism based on such facts, as well as the human interpretation of such facts and conclusions, generates uncertain and vague conclusions. There are many different approaches to representing knowledge in uncertain domains—from subjective Bayesian probabilities (Pearl Citation1988; Russel and Norvig Citation1995) through a rule-based system with certainty factors (Buchanan and Shortliffe Citation1984) to the fuzzy Petri net theory (Cardoso and Camargo Citation1999). In our approach we have used the last one, in which the uncertainty and confidence related to the facts, concepts, and the relationships between them are expressed by means of the values of f(ti), ti ∊ T, and c(mi), mi ∊ M, association functions. For example, the value of the function f, as well as the value of the function c, can be expressed by truth scales and by their corresponding numerical intervals depicted in Table (Chen, Ke and Chang 1990).
The threshold value λ ∊ [0, 1] defines the “sensitivity” of the scheme during the inference procedures. By using different values for λ, the user can specify different degrees of truth for inherited concept properties or the recognized concept. The value of λ has influence on a number of levels of generated inheritance or recognition trees. Usually, λ is chosen to be small enough, for example, 0.01 or 0.1.
Example 3
In order to illustrate the basic components of the KRFPN, a simple example (adopted from Touretzky (Citation1986)) of the agent's knowledge base for a scene (Figure ) is introduced. The knowledge base designed by the KRFPN = (FPN, α, β, C), where FPN is (P, T, I, O, M, Ω, μ, f, c, λ), has the following components (Figure ): P = {p1, p2,…, p10}; T = {t1, t2,…, t13}; I(t1) = {p1}; I(t2) = {p3},…;I(t13) = {p1};O(t1) = {p2}; O(t2) = {p4},…; and O(t13) = {p9}. The set of tokens is M = {m1, m2,…, mr}, the initial distribution of tokens is Ω0 = {{m1}, Ø,…Ø}, where c(m1) = 1.0, and Ø denotes an empty set. The vector μ 0 = (μ1, μ2,…, μ10) = (1, 0,…, 0) denotes that there is only one token in the place p1. The function f is specified as follows: f(t1) = 0.9; f(t2) = 0.9; f(t3) = 1.0; f(t4) = 1.0,…; f(t12) = 0.6; and f(t13) = 0.8 (Figure ). f(ti), i = 1, 2,…, m indicates the degree of our pursuance in the truth of the relation β(ti).
The set D = D1 ∪ D2 ∪ D3 is defined as follows: D1 = {Elephant, Human, Mammal, Biped, Quadruped}, D2 = {Fred, Clyde}, D3 = {White, Soul, Kin d − hearted}. The set Σ = Σ1 ∪ Σ2 ∪ Σ3is {i s − a, i s − no t − a} ∪ {ha s − colour, ha s − characteristic, has} ∪ {i s − i n − fron t − of, i s − behind}.
Functions α: P → D and β: T → Σ are (Figure ):
Let C be a set of contradictions defined as C = {(i s − a, i s − no t − a), (i s − i n − front, i s − behind), (Quadruped, Biped)}.
For the initial distribution of tokens, Ω0, the following transitions are enabled: t1, t9, t11, and t13.
Figure shows the ontological complexity of even a simple example. In large examples, a graph would be too cluttered to be of much use. Therefore, the hierarchical structures of graphs have to be used. Petri nets (Petri Citation1962), in general, are appropriate for hierarchical system modeling, i.e., the system can be represented at the different abstract levels. In a Petri net, a subnet may be replaced by a single place or transition (the process of abstraction), or places and transitions can be replaced by subnets (the process of refinement (Peterson Citation1977; Ribarić Citation1991)).
FUZZY INHERITANCE
Inheritance can be described as the process of determining the properties of a concept di ∊ D, by looking up the properties that are locally attached to the concept di. If such local information is not available (or is insufficient), the process will continue by looking up properties attached to the concepts that lie at higher levels in the conceptual hierarchy.
K-Level Inheritance Tree
The inheritance procedure in the KRFPN is based on its dynamical properties and the determination of the inheritance set of the KRFPN. The inheritance set for the KRFPN is based on concepts similar to the reachability set of the ordinary Petri nets (PNs), where the reachability relationship is the reflexive, transitive closure of the immediately reachable relationship (Peterson Citation1981). In the PN, for the marking μ, a marking μ' is immediately reachable from μ if there exists a transition tj ∊ T that can be fired, and by firing a new marking μ' is obtained. The reachability set is defined as the smallest set of all the reachable distributions of tokens stating for an initial distribution of tokens for the PN and recursively applying the firing of enabled transitions for immediately reachable distributions of tokens. The reachability set of the PN is graphically represented by a reachability tree.
The main differences between the inheritance set of the KRFPN and the reachability set of the PN (Peterson Citation1981) are as follows: (i) After firing all the enabled transitions for the distribution of tokens in the KRFPN, where the transitions are related to the elements in the subsets Σ2 and Σ3, the created tokens at the corresponding output places have to be frozen. Recall that the elements in Σ2 and Σ3 are used to specify the properties and the nonhierarchical structuring, respectively. A frozen token in the output place is fixed and it cannot enable a transition; (ii) An inheritance tree, as a graphical representation of the inheritance set, is bounded by k + 1 levels, where k is a predefined number of levels. Such an inheritance tree is called a k-level inheritance tree; (iii) A k-level inheritance tree has the following additional types of nodes: a k-terminal node, a frozen node, and an identical node.
A k-level inheritance tree consists of nodes (p j , c(mi)), j = 1, 2,…, n and i = 1, 2,…, v where n is a cardinality of the set of places P, and 0 ≤ v ≤ r, where r is a cardinality of the set of tokens M, and directed, labelled arcs. In order to simplify and make the notation uniform in the inheritance algorithm, the nodes in the tree are denoted by n-component vectors in the form π = (π1, π2,…π n ). Each component π i ; i = 1, 2,…, n of π is represented by an empty set Ø for μ(pi) = 0, i.e., there is no token(s) at the place pi , or by a set {c(mk),…, c(m l ),…, c(m s )}, where c(m l ) is the second component of the pair (pi, c(m l )) and represents the value of the token m l at the place p i. The number of elements in the above set is μ(pi) ≥ 1. The vector π is called the distribution vector, or simply the distribution.
The directed arcs in a k-level inheritance tree are labelled by tj ∊ T and f(tj), where tj denotes a fired transition and f(tj) the value of its association function f, respectively. A directed labelled arc leads from the node at the ith level of the inheritance tree to the corresponding node at the i + 1th level; i = 1,2,…, k-1, where k is (pre)defined as the final level of the inheritance tree.
During the creation process of the k-level inheritance tree, each node is classified either as a frontier node, a terminal node, a k-terminal node, a frozen node, an identical node, a duplicate node, or an interior node. Frontier nodes are nodes that have not yet been processed. The special frontier node is a node that represents the initial distribution and is a root of the inheritance tree. The root node is a node at the zero-level of the inheritance tree. The frontier nodes are converted by the inheritance-tree algorithm to terminal, k-terminal, internal, frozen, duplicate, or identical nodes. A terminal node is a node corresponding to the dead distribution for which there are no enabled transitions. A k-terminal node is a node at the kth level of the inheritance tree. A frozen node corresponds to the distribution that is created by firing an enabled transition, which is related to (by means of a function β) the elements from subsets Σ2 and Σ3. The distributions obtained by firing such transitions become the frozen distributions. (A frozen token is fixed at the input place and cannot enable the transition). Identical nodes correspond to the distributions that have previously appeared in the tree, and for them the following is valid: Two nodes π A and π B are identical if π Ai ≡ π Bi;i = 1, 2,…, n, where π Xi represents the ith component of π X , where x is A or B. Duplicate nodes are nodes that have also previously appeared in the tree; they are not identical in the sense of n-tuples π, but they have the same marking μ (see a formal definition of the KRFPN): Two nodes A and B are duplicates if π A = π B , where π X represents the marking; x is A or B. In other words, two nodes are duplicate nodes if they have an equal number of tokens in each corresponding place (regardless of the values of the tokens). An interior node is an already processed frontier node that is not classified as a terminal, a k-terminal, a frozen, an identical, or a duplicate node.
The inheritance-tree algorithm for the KRFPN is presented as follows:
Input: Initial distribution as a root of the k-level inheritance tree (a frontier node at the zero-level of the tree). The depth of the tree generation k; 1 ≤ k < ∞, and the threshold value λ ∊ [0,1], which defines the firing threshold for each transition.
Output: A k-level inheritance tree with all the nodes denoted as terminal, k-terminal, frozen, interior, identical, or duplicate.
Let π X be the frontier node to be processed.
Step 1 . If there exists another node π Y in the inheritance tree that is not a frontier node and π X ≡ π Y , the node π X is an identical node. Denote such a node by I.
If there exists another node π Z that is not a frontier node and π X = π Z , then the node π X is a duplicate node. Denote such a node by D.
Step 2 . If there are no enabled transitions for π X , then π X is a terminal node. Denote such a node by T.
If π X lies at the kth level of the inheritance tree, then denote such a node as the k-terminal node (k-T).
Step 3 . For all transitions tj that are enabled for π X , create a new node π W in the inheritance tree. The components of π W are defined according to the firing rule of the KRFPN. An arc, labelled by tj; f(tj), is directed from node π X to node π W . After that, the node π X is redefined as an interior node.
If π W is the result of firing a transition that corresponds to elements from Σ2 or Σ3, then π W is declared as a frozen node. Denote such a node by F.
Step 4 . If the node π W is not classified as a frozen node, then it becomes a frontier node.
When all the nodes have been classified as terminal, k-terminal, duplicate, identical, frozen, or interior nodes, the algorithm stops.
Example 4
Using this example, we illustrate the inheritance-tree algorithm. Let us suppose that the initial distribution of tokens for the KRFPN scheme depicted in Figure is given by Ω0 = π 0 = ({c(m1) = 1.0}, Ø,…, Ø), i.e., the token m1 is initially at the place p1 and has a value 1.0, all other places are without tokens. Let k be the depth of the inheritance tree k = 3 and the threshold level λ = 0.1.
Input: π 0 = ({c(m1) = 1.0}, Ø,…, Ø), k = 3, λ = 0.1.
Output: An l-level inheritance tree (0 ≤ l ≤ k) with all the nodes denoted as terminal, k-terminal, frozen, interior, duplicate, and/or identical nodes.
The 3-level inheritance tree generated by the algorithm is shown in Figure .
The initial distribution of tokens π 0 (node at the zero level) is a frontier node π x . There are enabled transitions as follows: t1, t9, t11, and t13. By firing the enabled transitions, the following nodes at the first level are created: π 11 , π 12 , π 13 , and π 14 . The nodes π 12 = (Ø, Ø, 0.9,…, Ø), π 13 = (Ø, Ø,…, 0.5), and π 14 = (Ø, Ø,…, 0.8, Ø) are frozen nodes. Note that the nodes π 13 and π 14 are also terminal nodes. The node π 11 becomes a frontier node and there are three enabled transitions: t4, t5, and t6. By firing the enabled transition t4, the node π 21 is generated. By firing the transitions t5 and t6, the nodes π 22 and π 23 are generated at the second level. Both these nodes are terminal nodes. The node π 21 = (Ø, Ø, Ø, Ø, 0.9, Ø,…, Ø) becomes a frontier node. The transition t7 is enabled, and after its firing, the node π 31 = (Ø, Ø, Ø, Ø, Ø, Ø, 0.72, Ø, Ø, Ø) is obtained. The node π 31 is a terminal node, and it is also a k-terminal node because the k = 3 level of the inheritance tree is reached. Note that π 31 is also a duplicate node because π 31 = π 23 .
Inheritance Assertion
By using the components of the k-level inheritance tree: a node at the level i − 1, labelled arc, a node at the level i (successor of the node at level i − 1), the functions α and β, a triplet named an inheritance assertion is formed.
For example, a node π 11 at the level 1, a labelled arc t4; f(t4) = 1.0, and the successor node π 21 at the level 2, and α(p2) = Human, β(t4) = i s − a, and α(p5) = Mammal, define the inheritance assertion: (Human is_a Mammal). Note that the tokens are at the places p2 (for π 11) and p5 (for π 21).
The strength of the assertion is defined as the value of the token at the successor node, i.e., as a product of the token value at the node at level i − 1 and the value of the association function of the corresponding transition.
For example, the strength of the inheritance assertion (Human is_a Mammal) is 0.90 × 1.0, where 0.90 is the value of the token at place p2(see the distribution π 11 ; Figure ).
The inheritance paths, starting from the root node of the inheritance tree and finishing at the leaves of the tree (terminal node, k-terminal node, frozen node, identical node, duplicate node) represent sequences of the inheritance assertions. An inheritance path is interpreted as the conjunction of the inheritance assertions in which the redundant concepts connected by AND are omitted. The strength of an inheritance path is defined by the value of the token at the node that is a leaf of the inheritance tree.
For example, the inheritance path defined from the root node π 0 to the leaf node of the tree π 22 (see Figure ) is: Fred is_a Human AND is_a Biped. The strength of the inheritance path is 0.72.
In network-based knowledge representation schemes, there is the well-known problem of the conflicting multiple inheritance (Touretzky Citation1986). The problem of the conflicting multiple inheritance in the KRFPN scheme is expressed as follows: Two inheritance paths, represented by sequences of the inheritance assertion, are in conflict if the same concept inherits the mutually contradictory elements from D, i.e., dk and dn, where (dk, dn) ∊ C,C is a set of contradictions. Also, two inheritance paths are in conflict if the same concept inherits the concept/property α(pk) ∊ D, but in one inheritance path it inherits the concept/property by means of β(tr) ∊ Σ, and in another by means of β(ts) ∊ Σ, where β(tr) = σr, β(ts) = σs, and(σr, σs) ∊ C.
To resolve the situations involving conflicting multiple inheritance in the KRFPN scheme, we used Touretzky's principle of inferential distance ordering PIDO (Touretzky Citation1986): In the situation of conflicting multiple inheritance, a concept inherits the property of the nearer individual (concept) or class. “Nearer” is defined as follows: Concept or class A is “nearer” to class B than to class C if A has an inheritance path through B to C. In many cases, the inferential distance ordering fails and reports an ambiguity (for example, see the well-known Quaker's problem) because it is based on a measure of “betweenness.” In such situations, in the KRFPN scheme, the concept inherits the property for which one can find the more direct inheritance path, i.e., the shorter path. If two or more inheritance paths have the same length the concept inherits property that corresponds to more strength in the inheritance path.
Inheritance Algorithm
The inheritance algorithm for the KRFPN is presented as follows:
Input: A concept of interest di ∊ D, the depth of the inheritance k; 0 ≤ k < ∞, λ ∊ [0,1].
Output: The properties of the concept di ∊ D, obtained by looking up the properties locally attached to the concept di, and the properties attached to the concepts that lie at higher levels in the conceptual hierarchy. The properties are expressed by means of semantic interpretations of the inheritance paths.
Step 1 . For a given concept of interest di ∊ D, by using the inverse function α−1, find the corresponding place pk:
Step 2. Define the initial marking μ 0 = (μ1, μ2,…, μ n ), where for j = 1, 2,…, n
Step 3. Define the initial distribution of tokens Ω 0 = π 0 = (Ø Ø Ø…{(pj, c(m1)},…, Ø, Ø) and set c(m1) = 1.0.
Step 4. For the initial distribution of tokens Ω0 = π 0 construct k levels of the inheritance tree.
Step 5. By using the elements of the inheritance tree: a node at level i − 1, a labelled arc, a corresponding node at level i, and the functions α and β, form the inheritance assertions.
Step 6. Find the inheritance paths, starting from the root node of the inheritance tree and finishing at the leaves of the inheritance tree. Determine the strengths of each inheritance path.
Step 7. If there are situations involving conflict due to the conflicting multiple inheritance, use the inferential distance ordering concept PIDO or (if that fails) make a decision on the basis of the more direct inheritance path. If two or more inheritance paths have the same length the concept inherits the property that corresponds to the stronger path. On the basis of the above criteria, remove the inheritance assertion that is the source of conflict and all the inheritance assertions that follow it.
Example 5
For the knowledge base represented in Figure infer the properties of the concept Fred (with the depth of inheritance k = 3), λ = 0.1. A set of contradictions C = {(is_a, is_not_a), (is_in_front, is_behind_of), (Biped, Quadruped)}.
Input: Fred ∊ D, k = 3, λ = 0.1.
Step 1 . α−1: Fred → p1.
Step 2. The initial marking is μ 0 = (1, 0, 0, 0, 0, 0, 0, 0, 0, 0).
Step 3. The initial distribution of tokens is Ω 0 = π 0 = ({(p1, c(m1)}, Ø, Ø, Ø,…, Ø, Ø) and c(m1) = 1.0.
Step 4. The inheritance tree is shown in Figure .
Step 5. The inheritance assertions are (see Figure ):
Level 1:
Fred is_a Human; (α(p1) β(t1) α(p2)); (strength = 0.90)
Fred is_in_front Clyde; (α(p1) β(t9) α(p3)); (strength = 0.90)
Fred has_characteristic Kind_hearted; (α(p1) β(t11) α(p10)); (strength = 0.50)
Fred has Soul; (α(p1) β(t13) α(p9)); (strength = 0.80)
Level 2:
Human is_a Mammal; (α(p2) β(t4) α(p5)); (strength = 0.90)
Human is_a Biped; (α(p2) β(t5) α(p6)); (strength = 0.72)
Human is_not_a Quadruped; (α(p2) β(t6) α(p7)); (strength = 0.90)
Level 3:
Mammal is_a Quadruped; (α(p5) β(t7) α(p7)); (strength = 0.72)
Step 6. The inheritance paths and their interpretations are:
-
Fred is_a Human AND is_a Mammal AND is_a Quadruped; (0.72)
-
Fred is_a Human AND is_a Biped; (0.72)
-
Fred is_a Human AND is_not_a Quadruped; (0.90)
-
Fred is_in_front Clyde; (0.90)
-
Fred has a Soul; (0.80)
-
Fred has_characteristic Kind_hearted; (0.50).
Step 7. According to the elements of the set of contradictions C and the definition of the contradiction, the inheritance paths (i) and (ii) generate contradictions because (Biped, Quadruped) ∊ C. The paths (i) and (iii) introduce a conflicting multiple inheritance due to (is_a, is_not_a) ∊ C. There is an ambiguity about the above inheritance paths: Is Fred a quadruped or a not?
To resolve the ambiguity caused by the inheritance paths (i) and (iii), the PIDO concept is used. Conflict due to the inheritance paths (i) and (ii) is solved on the basis of the more direct inheritance path: The inheritance path (i) is a path from the concept Fred through the concepts Human and Mammal to the concept Quadruped, and simultaneously there is the inheritance subpath Human is_a_not Quadruped.
The result of resolving the conflict situation on the basis of the PIDO and a more direct inheritance path is:
-
Fred is_a Human AND is_a Mammal; (0.90)
-
Fred is_a Human AND is_a Biped; (0.72)
-
Fred is_a Human AND is_not_a Quadruped; (0.90)
-
Fred is_in_front Clyde; (0.90)
-
Fred has Soul; (0.80)
-
Fred has_characteristic Kind_hearted; (0.50).
The strength attached to each inheritance path has to be interpreted as the confidence of the statement. With reference to Table , for instance, the statement: Fred is_a Human AND is_a Mammal; (0.90) is interpreted as very true, while the statement Fred has_characteristic Kind_hearted; (0.50) is interpreted as moderately true.
FUZZY RECOGNITION
The fuzzy recognition in the KRFPN can be described as follows:
Initialization : A set of the properties S of an unknown concept X is given, where it is not necessary that X ∊ D. S = S1 ∪ S2, where S1 is a set consisting of pairs (si, ai), where si can be an element of a set of concepts D and ai ∊ [1, 0] is the degree of a user's assurance that the unknown concept X has the property si. In this case the function α−1 is defined for si, but if si ∉ D, then the function α−1 is not defined for si. The elements in S2 have the form (relationship, (si, ai)). Usually the relationship is from Σ3 and allows recognition based on the relative spatial position of concepts, but in general it is possible that relationship is not an element of Σ, because we deal with unknown concepts. Specify the threshold value λ ∊ [0, 1].
Action : Search for the concept in the KRFPN that best matches the properties in the set S. The search is based on local properties and the properties that are inherited from the higher levels of the knowledge base.
The recognition-inference procedure in the scheme KRFPN is based on an inverse scheme −KRFPN and a slightly modified definition of the enabled transition, as well as a modification of the association function c. The inverse −KRFPN = (−FPN, α, β, C) is obtained by interchanging the positions of the input I and the output O functions in the 10-tuple that defines an FPN:
The main reason for the modification of the association function c is the existence of elements in Σ that have the forms of an exception or a negation of a property (for example, is_not_a). The modification of the association function c is also reflected in the execution of the −KRFPN. Firing an enabled transition ti in the −KRFPN (where ti corresponds to an exception in the original scheme) results in a new value of the token at the output place (Figure ):
FIGURE 9 Firing an enabled transition that corresponds to an exception. (a) Before firing; (b) after firing: cr(m2) = −cr(ml)f(ti) = −0.8.
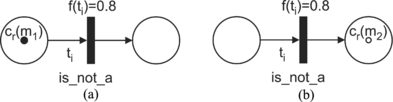
cr(mk) = −cr(mj)f(ti), where cr(mj) is the value of the token at the input place pj ∊ I(ti) and f(ti) is the degree of truth of the relation assigned to the transition ti ∊ T. Such a token is graphically represented by the symbol ○ (see Figure ).
Figure illustrates the firing of such a transition and applying this modification of the association function. The initial marking is μ 0 = (1, 0) and the initial distribution of the tokens is Ω 0 = {{m1}, ⊘}; cr(m1) = 1.0; f(ti) = 0.8; λ = 0.1; and the transition ti is enabled. After firing the enabled transition ti a new marking, μ′ = (0, 1), is obtained. The token in the output place has the value cr(m2) = − cr(m1)f(ti) = − 0.8.
The existence of the tokens with negative values in the −KRFPN also requires a redefinition of the enable transition:
-
(a) if the values of the tokens, cr(mj) > 0, j = 1, 2,…, k ≤ m, exceed the threshold value λ ∊ [0, 1], the corresponding transition is enabled.
-
(b) if the values of the tokens cr(mj) < 0, j = 1, 2,…,k ≤ m, the corresponding transition is enabled when |c(mj)| exceeds the threshold value λ ∊ [0, 1]; |x| denotes the absolute value of x.
The reachability set of the −KRFPN, called the recognition set, with an initial marking μ 0 and initial distributions of the tokens Ω0, is defined in a similar way to the inheritance set of the KRFPN, except for two important differences:
-
(c) the modification of the firing rule mentioned above is used;
-
(d) the transitions corresponding to relations in the subset Σ3 cannot be fired regardless of the states of their input places.
A graphical representation of a recognition set is called the recognition tree.
For properties having the form (relationship, (si, ai)), by means of selective firing (i.e., firing of the enabled transition corresponding to the specific relationship), the recognition subtree is obtained. Note that after firing the selected transition, the token at the output place is frozen and all the subsequent firings are disabled. A subtree consists of two nodes: the initial and the terminal. There are two exceptions in the construction of a recognition sub-tree in relation to the construction of a recognition tree:
-
(e) if the selected transition has the form of an exception or a negation of a property, then the value of the token in the output place is positive, i.e., cr(mk) = |cr(mj)·f(ti)|;
-
(f) the restriction expressed in (d) does not hold.
Recognition Algorithm
The recognition-inference algorithm for the KRFPN is presented as follows:
Input : A set of properties S of an unknown concept and a depth of search L, (1 ≤ L ≤ Deep < ∞; where Deep is the maximum depth of the search), expressed by levels of the recognition tree are given. The threshold value λ is selected (usually λ is chosen to be small enough, for example, 0.1).
Output : A concept from D that best matches the unknown concept X described by the set of properties S.
Step 1 . For the scheme KRFPN find the inverse scheme −KRFPN.
Step 2. For all (si, ai) ∊ S1; si ∊ D and ai ∊ [0,1], i = 1, 2,…, length ≤ Card(S1), where Card denotes a cardinality of a set, by means of the inverse function α−1: si → pj, determine the places pj, 0 < j = b ≤ n. Each such place pj becomes a place with a token (pj, cr(mi)), where the token value cr(mi) is ai. It defines b initial markings and initial token distributions. The initial markings are the root nodes of the recognition trees (nodes at level l = 0). The initial token values c(mi); i = 1, 2,…, b are determined by the degrees of assurance ai; i = 1, 2,…, b.
Step 3. For all the elements in S2 that have the form (relationship, (si, ai)), using the inverse functions, determine the initial markings and selective transitions for the construction of the recognition subtrees: α−1(si) = pj and β−1(relationship) = τ ⊂ T.
From the set τ select such a ti for which pj ∊ I(ti) in the −KRFPN. Put the token into a place pj – this is the initial marking of the subtree. The initial token value is determined on the basis of the user's specification of a degree of assurance for the concept ai: cr(mi) = ai. If there is no such a ti for which pj ∊ I(ti), the selective transition does not exist.
Step 4. Find L levels of all the recognition trees for b initial markings and initial token distributions.
Step 5. Find the recognition subtrees defined in Step 3.
Step 6. For each recognition tree, for levels l = 1, 2,…, L, compute the sum of the nodes (represented as vectors π):
Step 7. Compute the total sum of all the nodes for all the recognition trees:
Step 8. Compute the sum of the terminal nodes for all the recognition subtrees:
Step 9. Compute the sum E = Z + A , where E = (e1, e2,…, e n ).
Step 10. Find:
Step 11 . Select pi, for every i k ∗, k = 1, 2,…, |I∗|, k = 1,2,…, |I∗| where i = i k ∗, from the set P, and find all dk ∊ D using the function α: pi → dk. The concept(s) dk, where k = 1, or k = 1, 2,…, |I∗|, is(are) the best match(es) to the unknown concept X described by the set of properties S.
Example 6
Let us suppose that the unknown concept X is described by the following set of properties: S = S1 ∪ S2, where S1 = {(Quadruped, 0.9),(White, 0.6),(Kind_hearted, 0.5),(Royal_pet, 1.0)}, and S2 = {(is_on, (Earth, 1.0)), (is_behind, (Fred, 0.8))}. Find the concept in the KRFPN knowledge base (Figure ) that best matches the unknown concept X, for L = 3 levels of the recognitions trees and λ = 0.1.
Step 1. The inverse graph −KRFPN is shown in Figure (b). (For easy reference the graph of the original scheme is repeated in Figure (a)).
FIGURE 10 The KRFPN and −KRFPN inverse scheme (Example 3). (a) The KRFPN scheme; (b) the −KRFPN inverse scheme.
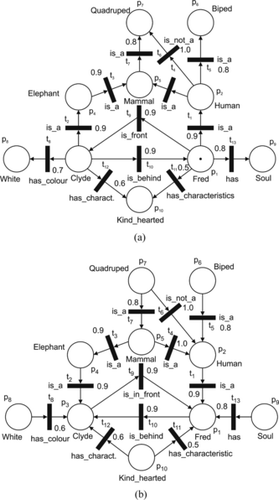
Step 2.
Step 3. For (is_behind, (Fred, 0.8)), β−1(is_behind) = {t10} and α−1(Fred) = p1, and p1 ∊ I(t10) in the −KRFPN, the initial marking π 0s for a subtree is π 0s = (0.8, 0, 0, 0, 0, 0, 0, 0, 0, 0). The selected transition that will be fired is t10. For (is_on; (Earth, 1.0)) the functions α−1 and β−1 are not defined.
Step 4. Recognition trees k = 1, 2, 3; (b = 3) for the depth of the search L = 3 are shown in Figure (a).
FIGURE 11 The recognition trees and the recognition subtree. (a) Recognition trees; (b) the recognition subtree.
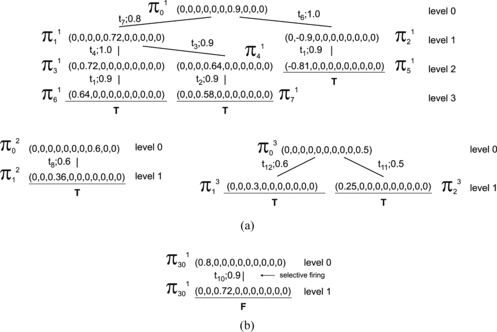
Step 5. The recognition subtree is shown in Figure (b).
Step 6.
Compute
For recognition tree 1, the nodes are (Figure (a)):
For recognition tree 3, the nodes are: ;
, and their sum is z
3 = (0.25, 0, 0.30, 0, 0, 0, 0, 0, 0, 0).
Step 7. Compute the total sum of all the nodes for all the recognition trees:
Step 8.
There is only one subtree (Figure (b)): .
Step 9. Compute the sum E = Z + A :
Step 10. Find: i∗ = arg maxi=1,…,10 {ei} = 3.
Step 11 . Select pi, where i = i∗, from the set P, and find drec ∊ D using the function α: pi → drec: α: p3 → Clyde.
According to the result of the recognition-inference procedure, the concept Clyde is the best match to the unknown concept X. Clyde is a quadruped; he is white and kind-hearted, and he is behind Fred (Figure ). Is he a royal pet (probably yes!) or is he on the Earth (probably yes!)? We explicitly don't know.
CONCLUSIONS
Original fuzzy inference procedures for the knowledge representation scheme KRFPN based on the FPN theory are proposed. The fuzzy inheritance uses a k-level inheritance tree, which is generated on the basis of the dynamical properties of the FPN, i.e., on firing enabled transitions and changing the values of the tokens according to the association function f that specifies the degree of assurance for the relation assigned to the transitions, and according to the association function c. Recognition, as a dual of the inheritance problem, uses the inverse –KRFPN that is obtained by interchanging the positions of the output and input functions in the 13-tuple specification of the KRFPN.
The very important properties of both inference algorithms are that the inheritance and recognition trees are finite and that the upper bound of the time complexity of the fuzzy inference algorithms is O(nm), where n is the number of places (concepts) and m is the number of transitions (a total number of relations in a knowledge base designed by the KRFPN). These allow the efficient execution of both, the inheritance and recognition procedures. The KRFPN was tested on numerous examples of the agent's knowledge bases of block world scenes as well as outdoor scenes, as well as in the knowledge base in a system for determination of a mental state of a driver.
Adaptation of the knowledge representation scheme KRFPN for time-varying fuzzy knowledge and spatio-temporal reasoning are our future research directions.
REFERENCES
- Aiello , L. C. and D. Nardi . 1991 . Perspectives in knowledge representation . Applied Artificial Intelligence 5 ( 1 ): 29 – 44 .
- Bobrow , D. G. 1985 . If prolog is the answer, what is the question? Or what it takes to support al programming paradigms . IEEE Software Eng. 11 ( 11 ): 1401 – 1408 .
- Buchanan , B. G. and E. H. Shortliffe . 1984 . Rule-Based Expert Systems: The MYCIN Experiments of the Stanford Heuristic Programming Project . Reading , MA : Addison–Wesley .
- Canales , J. C. , X. Liand , and W. Yu . 2006 . Fuzzy knowledge learning via adaptive fuzzy petri net with triangular function model . Intelligent Control and Automation. The Sixth World Congress on WCICA 1 : 4249 – 4253 .
- Cardoso , J. and H. Camargo (eds.). 1999 . Fuzziness in Petri Nets . Heidelberg : Physica-Verlag .
- Chen , S.-M. , J.-S. Ke , and J.-F. Chang . 1990 . Knowledge representation using fuzzy petri nets . IEEE Trans. on Knowledge and Data Engineering 2 ( 3 ): 311 – 319 .
- Chen , S.-M. 2002 . Weighted fuzzy reasoning using weighted fuzzy petri nets . IEEE Trans. on Knowledge and Data Engineering 14 ( 2 ): 386 – 397 .
- Dahl , V. 1983 . Logic programming as a representation of knowledge . Computer 16 ( 10 ): 106 – 111 .
- Duda , R. , J. G. Gaschnig , and P. E. Hart . 1979 . Model design in the PROSPECTOR consultant system for mineral exploration . In: Expert Systems in the Microelectronic Age , ed. D. Michie , 153 – 167 , Edinburgh : Edinburgh Univ. Press .
- Findler , N. V. (ed.). 1979 . Associative Networks , New York : Academic Press .
- Garcia , O. N. and Y.-T. Chien (ed.). 1991 . Knowledge-Based Systems: Fundaments and Tools . Los Alamitos : IEEE Computer Society Press .
- Guo-Yan , H. 2006 . Analysis of artificial intelligence based petri net approach to intelligent integration of design . Proc. of the International Conference on Machine Learning and Cybernetics : 1691 – 1695 .
- Ha , M.-H. , Y. Li , H.-J. Li , and P. Wang . 2005 . A new form of knowledge representation and reasoning . Proc. of the Fourth Int. Conf. on Machine Learning and Cybernetics , vol. 4 pp. 2577 – 2582 , Guangzhou .
- Israel , D. J. and B. Beranek . 1983 . The role of logic in knowledge representation . Computer 16 ( 10 ): 37 – 41 .
- Lee , J. , K. F. R. Liu , and W. Chaing . 2003 . Model uncertainty reasoning with possibilistic petri nets . IEEE Trans. on Systems, Man and Cybernetics – Part B: Cybernetics 33 ( 2 ): 214 – 224 .
- Li , X. and F. Lara-Rosano . 2000 . Adaptive fuzzy petri nets for dynamic knowledge representation and inference . Expert Systems with Applications 19 : 235 – 241 .
- Looney , C. G. 1988 . Fuzzy petri nets for rule-based decision making . IEEE Trans. on the System, Man and Cybernetics 18 ( 1 ): 178 – 183 .
- Looney , C. G. and L. R. Liang . 2003 . Inference via fuzzy belief petri nets . Proceed. of the 15th Int. Conf. on Tools with Artificial Intelligence (ICTAI '03) 510 – 514 .
- Minsky , M. L. 1975 . A framework for representing knowledge . In: The Psychology of Computer Vision ed. P. H. Winston , New York : McGraw-Hill .
- Newell , A. and H. A. Simon . 1972. Human Problem Solving . Englewood Cliffs , NJ : Prentice-Hall.
- Pearl , J. 1988 . Probabilistic Reasoning in Intelligent Systems: Network of Plausible Inference . San Mateo : Morgan Kaufmann .
- Peterson , J. L. 1977 . Petri nets , ACM Computing Surveys 3 ( 9 ): 223 – 253 .
- Peterson , J L. 1981 . Petri net theory and Modeling of Systems . Englewood Cliffs , NJ : Prentice-Hall .
- Petri , C. A. 1962 . Kommunikation mit Automaten . Bonn : Institut für Instrumentelle Mathematik, Schriften des IIM Nr. 2 . Second Edition: New York: Griffiss Air Force Base, Technical Report RADC-TR-65-377, 1, 1966: Suppl. 1 .
- Quillian , M. R. 1967 . Word concepts: A theory and simulation of some basic semantic capabilities . Behavioral Science 12 ( 5 ): 410 – 430 .
- Ribarić , S. 1991 . An original knowledge representation scheme . Automatika 1–2 ( 32 ): 13 – 24 .
- Russel , S. and P. Norvig . 1995 . Artificial Intelligence, A Modern Approach . Upper Saddle River , NJ : Prentice Hall .
- Scarpelli , H. , F. Gomide , and R. R. Yager . 1996 . A reasoning algorithm for high level fuzzy petri nets . IEEE Trans. on Fuzzy Systems 4 ( 3 ): 282 – 294 .
- Shafer , G. 1976 . Mathematical Theory of Evidence . Princeton , NJ : Princeton Univ. Press .
- Shastri , L. 1988 . Semantic Networks: An Evidential Formalization and Its Connectionist Realization . London : Pitman .
- Shen , V. R. L. 2006 . Knowledge representation using high-level fuzzy petri nets . IEEE Trans. on Systems, Man, and Cybernetics-Part A 36 ( 6 ): 1220 – 1227 .
- Touretzky , D. S. 1986 . The Mathematics of Inheritance Systems . London : Pitman .
- Zadeh , L. A. 1983 . Role of fuzzy logic in the management of uncertainty in expert systems . In: Fuzzy Sets and Systems . Pp. 199 – 227 . New York : Elsevier-North Holland .
- Zeigler , B. P. 1987 . Knowledge representation from Newton to Minsky and beyond . Applied Artificial Intelligence 1 : 87 – 107 .