Abstract
We discuss issues in designing virtual humans for applications that require long-term voluntary use and the problem of maintaining engagement with users over time. Concepts and theories related to engagement from a variety of disciplines are reviewed. We describe a platform for conducting studies into long-term interactions between humans and virtual agents and present the results of two longitudinal, randomized, controlled experiments in which the effect of manipulations of agent behavior on user engagement was assessed.
INTRODUCTION
Many applications in health care, education, entertainment, and other fields require designing voluntary-use systems for long-term interaction. For example, an automated weight loss counseling intervention may require a series of conversations with a user spanning months or years in duration, and intelligent tutoring systems may ultimately be designed to lead students through semester-long classes or even become life-long learning companions. Designing such systems requires approaches to maintaining user engagement over dozens, if not thousands, of interactions. Engagement is crucial, because it is typically a prerequisite for other system objectives: If a user stops interacting with a system, then it cannot have any further impact.
The most substantial body of work to date in the design and evaluation of technologies for discretionary long-term use lies within the wellness domain. These systems have fundamentally different requirements from prescribed medical monitoring applications in which adherence can be enforced by clinicians. In the last few years several such systems and longitudinal field studies have been developed for exercise promotion (Bickmore and Picard, Citation2005; Bickmore et al., Citation2005; Consolvo et al., Citation2006, 4), medication adherence (Bickmore and Pfeifer, Citation2008), diabetes self-care management (Mamykina et al., Citation2008), and other areas. The development of technologies to promote such healthy behavior (“behavioral informatics”) has also become a burgeoning area of research in the behavioral medicine community (Revere and Dunbar, Citation2001; Bickmore and Giorgino, Citation2006). Many companies are also now developing products in this space, such as the recently released Wii Fit and the Nike +iPod Sport Kit, but for the most part the impact of these devices on health outcomes has yet to be proven.
Maintaining long-term engagement is important in other application domains as well. Computer game developers are concerned with designing challenge hierarchies that maintain both short- and long-term engagement in their users over many sessions of play (Febretti and Garzotto, Citation2009). Website designers are concerned with visitor engagement, both within and between sessions (website “stickiness”) (Eytan et al., Citation2008). Finally, businesses care about fostering long-term customers, leading to developments in “customer relationship management” and “brand loyalty” (Dick and Basu, Citation1994).
Relational agents may represent a particularly compelling interface for such applications. Relational agents are conversational virtual humans designed to build and maintain long-term social–emotional relationships with their users (Bickmore and Picard, Citation2005). The use of social and relational behaviors by these agents, such as empathy and social chat, can serve to establish a social bond with users that in turn serves to maintain engagement over time and keep users returning again and again. In the wellness and healthcare context, these social bonds can also serve to increase adherence to health regimens being promoted by the agents.
Engagement: Concepts and Theories
There are many concepts of “engagement” in the literature, as well as several useful theoretical frameworks for studying and promoting engagement with users. Although many of these concepts have to do with short-term cognitive engagement in performing a task or in working with another person or thing (e.g., Csikszentmihályi's “flow” Csíkszentmihályi, Citation1990, Tickle-Degnen's “rapport” Tickle-Degnen and Rosenthal, Citation1990, or Sidner's “engagement” Sidner et al., Citation2005), we are more interested in conceptualizations of engagement that span much longer periods of time. We define long-term engagement as the degree of involvement a user chooses to have with a system over time. A related concept in longitudinal studies is “retention,” which is the number of individuals who complete a longitudinal intervention. Another measure of long-term engagement is the length of time a user adheres to an intervention protocol, or the length of time from when they start using a system until they indicate they no longer wish to use it. If a user can interact with a system as often as they like, another measure of long-term engagement is the number of interactions—or the percent of recommended interactions—they conduct within a given time span. A predictive self-report measure of long-term engagement is to simply ask users the degree to which they want to continue interacting with an agent, as an analogue to measures of commitment in research on romantic human relationships (Revere et al., Citation1994). Of course, there are negative extremes: “Addiction” is defined as a recurring compulsion by an individual to engage in a specific activity, despite harmful consequences to the individual's health, mental state, or social life.
Although improvements in short-term engagement with a relational agent should generally lead to improvements in long-term engagement through increased liking of and social bonding with the agent, Bickmore demonstrated one context in which these two are at odds with each other. In agents that must interrupt a user and “demand” their engagement, more polite interruption strategies tend to decrease short-term engagement (adherence to the interrupt request) but increase long-term retention, whereas less polite (and more annoying) interruption strategies tend to have the opposite effect (Bickmore et al., Citation2007).
Although several theoretical models can be used to predict long-term engagement and thus guide the actions of a relational agent, the most mature models are from the field of personal relationship research (Bickmore and Picard, Citation2005). Of these, the investment model of personal relationships has received the most empirical support and provides an economic framework in which engagement can be both assessed and promoted (Revere et al., Citation1994). This theory indicates that the factors that may positively influence relationship commitment to an agent include (1) increases in a user's ongoing perceived benefit of interacting with the agent (e.g., by providing useful information or entertainment), (2) decreases in their perceived costs, (3) increases in their perceived investment in the system, and (4) and decreases in their perceptions of viable alternatives to using the system. According to the theory, these all tend to increase user commitment to continuing with the agent and thereby their long-term engagement.
Overview
In the remainder of this article we briefly review related work in virtual human agents that attempt to maintain long-term engagement with users, then present the research platform we have been using in studies of long-term human-agent engagement. We then present the results of two empirical studies in which we manipulated different aspects of agent behavior and assessed their impact on engagement before closing and discussing future work.
RELATED WORK ON PROMOTING LONG-TERM ENGAGEMENT
The FitTrack study was one of the first longitudinal studies of engagement between users and virtual humans. In this study an animated exercise counselor (“Laura”) talked with sedentary users every day for a month about their exercise behavior in an attempt to motivate them to do more walking. This system was evaluated in a three-condition randomized trial with 101 mostly young adults to test the efficacy of the agent's relational behavior (Bickmore et al., Citation2005). One group of study participants (relational) interacted with a version of Laura in which all of her relational behavior (social dialogue, empathy, nonverbal liking behavior, etc.) was enabled, whereas a second group interacted with the same agent in which these relational behaviors were removed (nonrelational). A third group acted as a nonintervention control and simply recorded their daily physical activity (control). The Working Alliance Inventory—used to assess the quality of counselor–patient relationships in clinical psychotherapy (Horvath and Greenberg, 1989)—was used as the primary relational outcome measure. Participants in the relational condition reported significantly higher Working Alliance scores compared with those in the nonrelational condition, at both 1 week and the end of the 4-week intervention. Several other self-report and behavioral measures indicated that relational bonding with the agent was significantly greater in the relational group compared with the nonrelational group. There were no significant differences in the number of times users talked to Laura or retention between groups, likely due to the short duration of the study.
The Autom robotic weight loss coach was evaluated in a longitudinal diet intervention to promote dietary tracking among overweight adults. Autom consists of a desktop touch screen computer with an anthropomorphic robotic head on top, capable of tracking users with its head and eyes. Study participants were asked to record their diets using the system every day for 4 weeks, with an option to continue for another 2 weeks. The study compared Autom with an equivalent touch screen computer without the “robot” functionality (humanoid head) and with paper-based diet logs (15 participants in each group). Participants randomized to the Autom group used their system significantly longer compared with the other two groups (50.6 days compared with 36.2 for computer users and 26.7 days for paper logs) and scored significantly higher on the Working Alliance Inventory compared with the computer group (Kidd, Citation2008).
Another approach to promoting engagement is to treat engagement as a behavioral variable and use theories and techniques from persuasion (Petty and Cacioppo, Citation1996) and health behavior change (Glanz et al., Citation1997) to motivate users to continue their interactions with a system. This approach was taken in a relational agent designed to promote medication adherence among patients with schizophrenia (Bickmore and Pfeifer, Citation2008). From the very first conversation the agent reminds the user of the importance of continuing use of the system every day, provides feedback on system use adherence (via dialogue and self-monitoring charts), helps the user resolve barriers to system use (e.g., forgetting or not having the time), and obtains a behavioral commitment at the end of every conversation to talk to the agent again at a specific time in the future. In a 30-day quasi-experimental evaluation study, the 20 participants talked to the agent an average of 65.8% of the available days, with 9 participants talking to the agent at least 25 times during the 31-day intervention.
VIRTUAL LABORATORY SYSTEM
To answer empirical questions about the effects of relational agent behavior on long-term engagement, we developed a “virtual laboratory” system (Bickmore and Schulman, Citation2009). This system provides a framework for running longitudinal studies of ongoing interactions between humans and relational agents, in which a standing group of study participants interacts periodically with an agent that is remotely manipulated to effect different study conditions, with outcome measures also collected remotely. This architecture allows new experiments to be dynamically defined and immediately implemented in the continuously running system without delays due to recruitment and system reconfiguration. In the current instantiation, up to 30 older adults interact daily with a relational agent who plays the role of an exercise counselor to promote walking behavior. Older adults were selected as the target population because of their particular need for physical activity and their lower levels of computer literacy (Bickmore et al., Citation2005).
The virtual laboratory has been running continuously over the last 24 months, with a total of 51 study participants aged 55 or older conducting a total of over 10,000 conversations with the animated exercise counselor (Figure ). The subject pool has had 24 participants on average, with participants staying in the intervention between 18 and 572 days. Participants are on average 60 years old (range, 55–75), 73% female, and 54% married.
Common Study Methods
The following studies share a common set of procedures and measures. All participants were required to be 55 years or older, to have access to an Internet-connected personal computer, and were compensated $1 per day for each day they completed a conversation with the agent. The sample is generally well educated (92% have some college education), computer literate (12% self-identified as computer experts, the other 88% say they use computers regularly), and has a positive attitude toward computers overall (64% said they enjoyed working with computers).
Steps walked per day by study participants were measured with Omron HJ-720ITC pedometers. Participants were prompted once per day to connect their pedometer to the computer so the step count could be automatically downloaded. The pedometers store up to 6 weeks of step counts, so that information was not lost if a participant did not interact with the system on a particular day.
Participants underwent a short intake procedure, which took place in our laboratory, at which time they were randomly assigned to one of the study conditions. Participants received brief instruction in the use of the pedometer and participated in a sample interaction with the agent. After this, participants had up-to-daily interactions with the agent at home. Participants were told they can stay in the virtual laboratory system up to 4 years or until they withdrew or missed 14 consecutive daily interactions, at which time they would be dropped. Participants were contacted after missing 5 days and again after 10 and 12 days in an attempt to keep them in the virtual laboratory. With these exceptions, the researchers did not contact participants unless they were experiencing technical or other problems.
To examine the trends in participant behavior over time, we analyzed the data using mixed-effect modeling. All analysis was performed using R 2.9.0 (R Development Core Team 2008) with the “nlme” and “lme4” packages. Quantitative outcomes such as self-report scores were analyzed by fitting linear mixed-effect regression models to the data, whereas binary outcomes such as daily system usage were analyzed with a logistic mixed-effect regression model.
EMPIRICAL STUDY ON THE EFFECT OF AGENT BEHAVIOR VARIABILITY ON LONG-TERM ENGAGEMENT
One surprising finding in the longitudinal studies of the FitTrack system was that, even though dialogue scripts had been authored to provide significant variability in each day's interaction, most participants found the conversations repetitive at some point during the intervention, and because of this many lost motivation to follow the agent's advice (Bickmore et al., Citation2005; Bickmore and Picard, Citation2005). As one participant put it, “It would be great if Laura could just change her clothes sometimes.” This repetitiveness was more than an annoyance; some subjects indicated that it negatively impacted their motivation to exercise. (e.g., “In the beginning I was extremely motivated to do whatever Laura asked of me, because I thought that every response was a new response.”)
Our first longitudinal study using the virtual laboratory was thus to evaluate the impact of perceived agent repetitiveness on retention and adherence to a health behavior change intervention. The study had a between-subjects design with two treatments: variable and nonvariable. Participants were randomized into one treatment initially; after an initial intervention period, each participant was switched to the other treatment for an additional intervention period. We designed two parallel sets of dialogue scripts to promote walking as a form of exercise, based on work on prior projects (Bickmore et al., Citation2005). The scripts were functionally identical, except that in the nonvariable condition the agent used the exact same dialogue structure and language in every situation (e.g., contingent positive reinforcement was always given as “Congratulations. Looks like mission accomplished on the exercise.”) and the agent's appearance and setting were never changed. In contrast, in the variable condition one of five different dialogue structures were randomly selected each interaction to guide the overall topic sequence in the conversation. For example, one topic sequence may cause the agent to greet the user, then conduct some social chat, and then review the user's exercise behavior, whereas a different topic sequence may cause the agent to greet the user, review the user's exercise behavior, and then conduct social chat. In addition, in the variable condition every agent utterance within a topic had multiple surface forms, of which one was selected randomly during each conversation (e.g., “Looks like you met your exercise goal of 5,000 steps. Great job!”, “Looks like you got your walking in and met your goal of 5,000 steps!”, etc.). In addition, one of five different background scene images was randomly selected and displayed behind the agent at the start of each conversation.
Variability Study Participants
Twenty-four participants (17 woman and 7 men, aged 55 to 75) enrolled in the virtual laboratory system and took part in the study.
Variability Study Measures
At the end of each daily interaction, participants completed two single-item questionnaires, which measured their desire to continue using the system (“How much would you like to continue working with Karen?”) and the perceived repetitiveness of the interactions (manipulation check: “How repetitive are your conversations with Karen?”). Both used a 5-point Likert scale, ranging from “not at all” to “very much.”
Variability Study Results
Of the 24 participants, 10 were randomized to the variable condition and 14 to nonvariable. Participants initially interacted with the system between 62 and 141 days (mean, 102.32), and 3 from each group (6 in total) dropped out during the initial intervention period. All remaining participants then interacted with the system in the opposite condition for an additional 126 days. There were no additional dropouts during this period.
Figure shows plots of key measures over the duration of the study, by study group, and Table presents descriptive statistics for key weeks in the study. Table shows the results of fitting mixed-effect regression models (linear for steps and perceived repetitiveness, and logistic models for desire to continue and system usage). For all outcomes two models were considered: one with effects of study day and study condition and one with an additional interaction effect. The best-fitting model, according to AIC, was used for inference. All models include random effects of intercept and study day. Note that Table summarizes a small subset of the data, whereas the regression model in Table is fit to all longitudinal data points, providing greater accuracy and statistical power for hypothesis testing.
FIGURE 2 Plots of measures over time by study group for variability study. Left plot: Repetitiveness. Middle plot: Desire to continue. Right plot: Sessions.
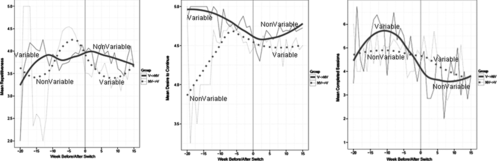
TABLE 1 Descriptive Statistics for Key Weeks in Variability Study
TABLE 2 Longitudinal Model Fit and Hypothesis Tests for the Variability Study
Perceived Repetitiveness
Participants reported significantly more perceived repetitiveness in the nonvariable condition. No significant change over time was observed.
Desire to Continue
There was a large ceiling effect on this measure; on a 5 item scale, participants answered 5 on most responses (74.1%). Therefore, we dichotomized this outcome, treating a response of 5 as “high desire to continue” and any other response as “low desire to continue.”
Participants were significantly more likely to report a high desire to continue when in the variable condition. There was also a significant, but far smaller in magnitude, interaction effect: Participants tended to report high desire to continue more often over time when in the nonvariable condition.
System Usage
System usage was analyzed as a binary outcome; that is, whether participants had a conversation with the agent on a particular day. Participants were significantly more likely to have a conversation when in the variable condition (Figure ). There was also a significant effect of study day: Participants tended to have fewer conversations over time.
Steps
Participants walked a significantly greater number of steps per day in the nonvariable condition. However, participants also walked significantly fewer steps over time, and there was a significant interaction effect: Participants'; step counts decreased more quickly in the nonvariable condition.
Variability Study Discussion
We found a positive effect of variability in agent behavior on long-term engagement: Participants interacted with the agent significantly more when it exhibited variability in its behavior over time and were more likely to report a high desire to continue interacting. However, this increased engagement did not translate into more exercise—in fact, participants walked less when the agent exhibited more variability. There are several possible reasons for this. First, it could be that phrasing feedback in exactly the same way every conversation leads to better habit formation, in this case walking. It could also be that the intervention is less effective when used more frequently (a negative dose–response relationship), or that subjects took the agent or the intervention less seriously when it appeared to be more fun to interact with. Exactly which factors—dialogue, utterance, or visual variability—were responsible for all these results will need to be teased apart in future studies.
EMPIRICAL STUDY ON THE EFFECT OF BACK STORIES ON LONG-TERM ENGAGEMENT
One design issue faced by all developers of conversational virtual human agents that interact with users in nonentertainment domains is to what extent the agents should present themselves as actually being human. The decision as to whether the agents should be presented as humans at all is moot, because fidelity to human appearance and behavior is the overarching objective of this field of research. However, many researchers believe they are somehow crossing an ethical boundary if their agents start discussing their childhood home or the fight they just had with their (presumably human) spouse. Just as Deckard in the movie Blade Runner was shocked when he learned that replicants (bioengineered anthropomorphic beings) were being created with autobiographical memories, many people seem to recoil at the thought of a computer being designed to actually present itself as human, without any fictional or “as if” framing. However, there has been no systematic exploration of this topic from an empirical perspective. How would users actually react to agents that present themselves with human autobiographical memories compared with the same agents that make no such pretense? Would they feel cheated and deceived, as many researchers contend, or would the use of such stories actually increase long-term engagement? Social chat by agents in applications designed for voluntary long-term use provides a mechanism for maintaining user engagement over arbitrary lengths of time, provided that the stories the agent tells are, in fact, entertaining and engaging. Within this context, first-person stories may provide the additional engagement required to make a longitudinal application successful.
A number of empirical studies suggest that users actually want agents to be more like them, implying they may also want them to be more human-like, whether they are conscious of this desire or not. For example, in the Media Equation studies, Reeves and Nass (Citation1996) demonstrated that users prefer computers that match them in personality (along the introversion/extroversion dimension based on text messages displayed) compared with computers that do not. van Vugt et al. (Citation2006) demonstrated that users prefer characters that match them in body shape.
In addition, in a prior study involving animated exercise coaches, Bickmore (Citation2003) related anecdotes from study participants in which they stated their desire for the animated coach they had worked with for the prior month to have a more human back story. An example follows:
“I wish she could imitate a real person's life in her answers rather than sticking to the reality and saying things like she is limited to that box. Maybe this has something to do with trainees wanting to have role model to achieve their own physical fitness roles by taking the trainer as a role model. Or maybe it is just about having a richer conversation helping getting connected to the other person.”
To investigate reactions of users to agents that relate personal human (“first person”) back stories, we conducted a randomized longitudinal experiment in which users conducted daily conversations with an agent that related such stories (Bickmore et al., Citation2009).
To compare the effects of the use of first-person and third-person narrative dialogue by an agent on long-term engagement, we conducted a longitudinal study using participants enrolled in the virtual laboratory system. The agent conducted daily conversations about exercise, with the addition of narrative dialogue generated using a social story generation system (Bickmore et al., Citation2009). Participants were randomized into one of two conditions: In the first (first-person), the agent presented the narrative as its own life story, whereas in the second (third-person), the agent presented the narrative as stories about a friend (Figure ).
FIGURE 4 Example narrative dialogue showing the same story fragments in first-person and third-person conditions.
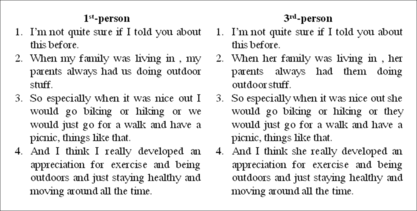
We expected that the use of first-person narrative would promote greater engagement with the agent due to a perception of self-disclosure by the agent and the perception of more direct involvement in the stories, leading to more consistent usage of the system. However, we were also concerned that users would perceive the agent as dishonest when it presented a life story for itself that was not plausibly true for a computer character. Participants were administered daily questionnaires to assess their enjoyment of the stories, their engagement with the system, and their belief that the agent was dishonest.
Back Story Study Participants
A total of 26 participants (21 women and 5 men, aged 54–67, 80% White, 20% African American) took part in this study. Fifteen had previously interacted with the system at the start of the study, whereas 11 were newly recruited. Exactly half of the participants were randomized into each arm of the study (first-person and third-person). Participants were exposed to these study conditions for varying periods of time, ranging from 5 to 37 days (mean, 28.8 days).
Back Story Study Measures
After each complete conversation with the agent, participants were given three single-item measures in randomized order, asking how much they (1) “enjoy the stories that the counselor tells,” (2) “look forward to talking to the counselor,” and (3) “feel that the counselor is dishonest.” Each item was assessed on a 5-point rating scale ranging from “not at all” to “very much.”
Narrative Dialogue
Narrative social dialogue was generated using the dynamic social story generation described above. In the first-person condition, the narratives were initially introduced as being part of the agent's own life story (“I'd like to tell you some stories about myself”). In the third-person condition, the narratives were introduced as being from the life story of a human friend of the agent with a similar role and occupation (“I'd like to tell you some stories about a friend of mine. She's an exercise counselor too.”).
The differences between the first- and third-person variants of the dialogue were minimal and consisted mainly of replacing pronouns. Figure shows an example of the narrative dialogue in both variants.
Back Story Study Results
Table presents descriptive statistics for key weeks in the study. Table shows the results of fitting mixed-effect regression models through all data points. For all outcomes, models were used that included fixed effects of study day and study condition. Model selection procedures (as in the earlier study; see Section 4.3) indicated that a model including an interaction of day and condition was not preferable. All models included random effects of intercept and study day.
TABLE 3 Descriptive Statistics for Key Weeks in Back Story Study
TABLE 4 Longitudinal Model Fit and Hypothesis Tests for Back Story Study
System Usage
Participants in the first-person condition had a significantly greater probability of talking to the agent on any given day, compared with those in the third-person group (Figure ). There was also a significant effect of study day; for the average participant, the probability of completing a session on any given day decreased over time.
Self-Report of Engagement
There were no significant differences between conditions on degree to which participants said they “looked forward” to working with the agent. However, the average participant (both groups) reported significantly decreasing levels of engagement over time (approximately 0.017 per day).
Enjoyment of the Stories
Participants in the first-person condition reported significantly greater enjoyment of the stories compared with those in the third-person group. There was also a significant effect of study day; participants reported decreasing enjoyment of the stories over time (approximately 0.035 per day).
Perceived Dishonesty
Participants, overall, did not perceive the agent as very dishonest. Average perceived dishonesty (both groups) after the first conversation was 1.69 (on a 1 = “not at all” to 5 = “very much” scale). There was no significant effect of study day or of study condition on this measure.
Steps
Participants in the third-person condition walked significantly more steps compared with those in the first-person condition. There was no significant effect of study day on steps.
Continuing Versus New Participants
The 11 participants who were newly recruited for this study did use the system significantly more compared with participants who had already been interacting with the system at the start of the study (p = .01). Including old versus new participant as a covariate in the regression analysis does not change the significance status of any of the results above.
Back Story Study Discussion
As hypothesized, participants who interacted with an agent that used first-person stories reported greater enjoyment of the stories and were more likely to use the system. Therefore, we can conclude that the first-person stories led to greater engagement with the system, at least during the short duration of time studied here. Both measures had significant decreases over time (in both conditions). This is likely due to increasing repetitiveness, as the agent had only a small set of story fragments to draw from in generating each day's story.
However, participants were not significantly more likely to report that they looked forward to working with the agent in the first-person condition. We consider two possible explanations. First, scores on this measure were all quite high (mean 4.22 on a 5-point scale), so ceiling effects may be hiding any difference caused by study conditions. Second, this result may indicate that our self-report measure of engagement does not reflect actual behavior; this raises methodological issues for future studies.
Participants were not significantly more likely to report that the agent was dishonest when it used first-person narrative, despite the fact that these stories could not possibly be true stories about a virtual character. This result suggests that users are willing to accept a fictional narrative that would be plausible for the character if the character were human.
CONCLUSION
The longitudinal studies described demonstrate that increases in user engagement with an interface agent—measured by actual frequency of voluntary system use—can be manipulated using relatively simple techniques that make the agent more life-like and human. The first study showed that increased variability in agent behavior leads to increased engagement and self-reported desire to continue interacting with the agent. The second study showed that giving the agent a human back story also led to increased engagement.
Neither study demonstrated that increased engagement led to increased exercise behavior—in fact, both studies showed the opposite. This may be evidence that for this behavior (exercise) and this counseling format (daily check-ins) there is a negative dose–response relationship between frequency of system use and adherence. Another possible explanation is that the agent behaviors we manipulated—variability and personification—actually made subjects take the agent and the intervention less seriously, leading to lower adherence.
The studies begin to illuminate how perceptions and attitudes of users change over time as they interact with conversational virtual humans. Seemingly superficial behavior, such as subtle changes in language and visual representation, have a cumulative impact on user perceptions and attitudes that eventually translate into user behavior we care about, such as the decision to continue using a system or not. Strategies for increasing user involvement, such as the telling of fictitious autobiographical back stories also have an ultimate impact on long-term engagement. Studies such as these are essential as we begin to design agents and robots intended to live and work with people over very long periods of time.
In light of the investment model of personal relationships presented in Section 1.1, variability and personal stories can be seen as increasing the perceived rewards of interacting with an agent. Personal stories may also increase investment when “cliffhanger” techniques are used to tell stories of conflict whose resolution is intentionally withheld until a future interaction.
These results are significant for designers of “serious” virtual humans that engage users in counseling, pedagogical, or health care conversations over long periods of time. Maintaining user engagement with these systems is a prerequisite for achieving any intervention outcomes, because users who stop using such a system or use it at a suboptimal frequency do not receive the therapeutic and informational messages required to achieve the desired results. The manipulations to affect engagement perform what Jakobson (Citation1960) defined as the “phatic” function of dialogue, which keeps the communication channel open so that the primary functional messages can be conveyed.
Future Work
In our ongoing work we are developing virtual human-based health counseling interventions that span a year or more of daily conversations with a user. In addition to procedural dialogue content generation (e.g., based on weather data or sports scores from the Internet) and approaches to generating random variability in behavior, we see autobiographical conversational storytelling by the agent as one of the most important methods available for maintaining user engagement in the intervention over time. We are working on many enhancements to our storytelling system to make it scalable for telling large numbers of stories over time with little or no manual authoring and to allow users to play more of an interactive role in the storytelling dialogue.
ACKNOWLEDGMENTS
We thank Thomas Brown, Jenna Zaffini, and the rest of the Relational Agents Group at Northeastern for their help and Jennifer Smith and Laura Pfeifer for their helpful comments on this article. This work was supported by NSF CAREER IIS-0545932.
Notes
Values are means, with standard deviations in parentheses.
Values are means with standard deviations in parentheses. Condition: 0 = variable, 1 = nonvariable. Example interpretation: For steps, the significant fixed effect on condition indicates the average participant walked an estimated 847.97 more steps per day when in the nonvariable condition. The fixed effect on Day indicates an estimated decrease in walking of 13.63 steps per day, and the significant interaction on Day and Condition indicates that this decrease was greater when in the nonvariable condition.
*p ≤ 0.05, **p ≤ 0.01, ***p ≤0.001.
Values are means with standard deviations in parentheses.
Values are means with standard deviations in parentheses. Condition: 0 = first-person, 1 = third-person.
*p < 0.5, **p < 0.01, ***p < 0.001.
REFERENCES
- Bickmore , T. 2003 . Relational agents: Effecting change through human-computer relationships. Ph.D. Dissertation, Media Arts & Sciences. Massachusetts Institute of Technology, Cambridge, MA.
- Bickmore , T. , and T. Giorgino . 2006 . Health dialog systems for patients and consumers . J. Biomedical Informatics , 39 ( 5 ): 556 – 571 .
- Bickmore , T. , and L. Pfeifer . 2008 . Relational agents for antipsychotic medication adherence. Proceedings of the Conference on Human Factors in Computer Systems, CHI'08 Workshop on Technology in Mental Health, Florence, Italy, 1–7.
- Bickmore , T. , and R. Picard . 2005 . Establishing and maintaining long-term human-computer relationships . ACM Transactions on Computer Human Interaction , 12 ( 2 ): 293 – 327 .
- Bickmore , T. , and D. Schulman . 2009 . A virtual laboratory for studying long-term relationships between humans and virtual agents. Proceedings from the Conference on Autonomous Agents and Multi-Agent Systems (AAMAS), Budapest, Hungary, 297–304.
- Bickmore , T. , L. Caruso , K. Clough-Gorr , and T. Heeren . 2005. “It's just like you talk to a friend”—Relational agents for older adults. Interacting with Computers , 17(6):711–735.
- Bickmore , T. , A. Gruber , and R. Picard . 2005 . Establishing the computer-patient working alliance in automated health behavior change interventions . Patient Education and Counseling , 59 ( 1 ): 21 – 30 .
- Bickmore , T. , D. Mauer , F. Crespo , and T. Brown . 2007 . Persuasion, task interruption and health regimen adherence. Proceedings of the Persuasive Technology Conference. Stanford, CA, USA, 1–11.
- Bickmore , T. , D. Schulman , and L. Yin . 2009 . Engagement vs. deceit: Virtual humans with human autobiographies. Proceedings of the Intelligent Virtual Agents (IVA) Conference, Amsterdam, 6–19.
- Consolvo , S. , K. Everitt , I. Smith , and J. A. Landay . 2006 . Design requirements for technologies that encourage physical activity. Proceedings of Conference on Human Factors in Computer Systems, CHI'06, Quebec, Canada, 457–466.
- Consolvo , S. , D. McDonald , T. Toscos , M. Y. Chen , J. Froehlich , B. Harrison , P. Klasnja , A. LaMarca , L. LeGrand , R. Libby , I. Smith , and J. A. Landay . 2008 . Activity sensing in the wild: A field trial of UbiFit Garden. Proceedings of Conference on Human Factors in Computer Systems, CHI '08, Florence, Italy, 1797–1806.
- Csíkszentmihályi , M. 1990 . Flow: The Psychology of Optimal Experience . New York : Harper and Row
- Dick , A. , and K. Basu . 1994 . Customer loyalty: Toward an integrated conceptual framework . Journal of the Academy of Marketing Science , 22 ( 9 ): 99 – 113 .
- Eytan , A. , J. Teevan , and S. Dumais . 2008 . Large scale analysis of web revisitation patterns. Proceedings of Conference on Human Factors in Computer Systems, CHI'08, Florence, Italy, 1197–1206.
- Febretti , A. , and F. Garzotto . 2009 . Usability, playability, and long-term engagement in computer games. Proceedings of Conference on Human Factors in Computer Systems, CHI'09 Extended Abstracts, Boston, MA, USA, 4063–4068.
- Glanz , K. , F. M. Lewis , and B. K. Rimer . 1997 . Health Behavior and Health Education: Theory, Research, and Practice . San Francisco : Jossey-Bass
- Jakobson , R. 1960 . Linguistics and poetics . In Style in Language , ed. T. A. Sebeok. Cambridge , MA : MIT Press .
- Jefferson , G. 1978 . Sequential aspects of storytelling in conversation . In Studies in the Organization of Conversational Interaction , ed. J. Schenkein , 219 – 248 . New York : Academic Press .
- Kidd , C. D. 2008 . Designing long-term human-robot interaction and application to weight loss. Ph.D. Dissertation, Media Arts & Sciences, MIT, Cambridge, MA, USA.
- Mamykina , L. , E. Mynatt , P. Davidson , and D. Greenblatt . 2008 . MAHI: Investigation of social scaffolding for reflective thinking in diabetes management. Proceedings of CHI'08, Florence, Italy, 477–486, 219–248.
- Petty , R. , and J. Cacioppo . 1996 . Attitudes and Persuasion: Classic and Contemporary Approaches . Boulder , CO : Westview Press
- R: A Language and Environment for Statistical Computing. http://www.R-project.org
- Reeves , B. , and C. Nass . 1996 . The Media Equation . Cambridge : Cambridge University Press
- Revere , D. , and P. J. Dunbar . 2001 . Review of computer-generated outpatient health behavior interventions: Clinical encounters “in absentia” . Journal of the American Medical Informatics Association , 8 : 62 – 79 .
- Rusbult , C. , S. Drigotas , and J. Verette . 1994 . The investment model: An interdependence analysis of commitment processes and relationship maintenance phenomena . In Communication and relational maintenance , eds. D. Canary and L. Stafford , 115 – 140 . San Diego , CA : Academic Press .
- Sidner , C. L. , C. Lee , C. D. Kidd , N. Lesh , and C. Rich . 2005 . Explorations in engagement for humans and robots . Artificial Intelligence , 166 ( 1–2 ): 140 – 164 .
- Tickle-Degnen , L. , and R. Rosenthal . 1990 . The nature of rapport and its nonverbal correlates . Psychological Inquiry , 1 ( 4 ): 285 – 293 .
- van Vugt , H. , E. Konijn , J. Hoorn , and J. Veldhuis . 2006 . Why fat interface characters are better e-health advisors. Proceedings of Intelligent Virtual Agents (IVA) Conference, Marina Del Rey, CA, USA, 1–13.