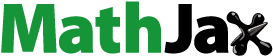
ABSTRACT
In the real world, decision-making process is related to alternative evaluation with respect to multiple conflicting criteria. In this paper, a hybrid group approach to solve the site selection problem is developed by integrating two methods which are the Best Worst Method (BWM) and Evaluation based on Distance from Average (EDAS). The multi-criteria group decision-making methods aggregate expert preferences and present the best agreement. BWM method is used to do pairwise comparisons in a structured way to determine the criteria weight. EDAS is a method used for alternative ranking which is useful for the decision issues that contain conflicting criteria. In order to show the procedures and the application of the developed method, a case study for site selection of plastic manufacturing company is developed. A comparison made between the proposed method and the AHP method is developed to ensure that the proposed method offers reliable results. Also, sensitivity analysis is conveyed for robustness validation.
Introduction
Most decision-making problems include alternatives which a decision-maker should determine the most suitable one based on defined criteria. In these problems, there are criteria and sub-criteria with different importance and alternatives need to be evaluated based on the pre-determined criteria. In this regard, the multi-criteria decision-making methods as the rational approach help to find the most appropriate alternative. Accordingly, multi-criteria group decision-making methods are used to aggregate individual expert preferences and present the most suitable alternative when more than one decision maker to take the decision (Chakraborty, Zavadskas, and Antucheviciene Citation2015).
Site selection is the process of finding the suitable sites for a project establishment depending on different criteria as the complexity of projects does not depend only on the industry but also on environmental, economic and social factors (Taibi and Atmani Citation2017). Previous studies have already applied different methods such as Analytic Hierarchy Process (AHP), Technique for Order Preference by Similarity to Ideal Solution (TOPSIS), and Elimination and Choice Expressing Reality (ELECTRE) to solve the site selection problems (Zavadskas, Turskis, and Kildienė Citation2014).
Another study for site selection is presented to find the best alternative for a plastic goods manufacturing company new site among four possible locations while all criteria are ranked and compared using AHP method and most convenient option was weighted using TOPSIS to be presented to the top managers of the company (Yaşlioğlu and Önder Citation2016). The problem with TOPSIS method is that it does not consider the correlation of attributes; difficult to weigh and keep consistency of judgment.
ELECTRE method is adopted to find optimum site which has several iterations and is also an outranking method (Uysal and Yavuz Citation2014). The disadvantage of ELECTRE method is that its process and outcomes can be hard to explain in simple terms. PROMETHE method also has been used but the problem that it does not provide a clear method to assign weights (Athawale and Chakraborty Citation2010).
The site selection problem has big concern over the last years and several methods have been recommended. The complexity of site selection problem is due to that it depends on variety of criteria such as environmental, economic, and industrial and social factors. Many criteria decision-making methods have grown-up concerned with computational tools for supporting decision-makers. The problem within the previously used methods is that they need a lot of pair-wise comparisons which is conflicting to decision makers and time consuming. Also, they are more suitable in conditions when the information is defined in numerical values and the impact analysis is implemented from the side where all weights of variables suggested a compromise choice which does not happen in site selection problem.
The main objective of this paper is to propose a multi-criteria group decision-making method based on Best Worst Method (BWM) and EDAS (Evaluation based on Distance from Average Solution) method for site selection problem. BWM needs little number of comparisons and maintains the consistency of pairwise comparisons. EDAS method is a new method which is useful for the decision issues that contain conflicting criteria easy to be applied without complex calculations. A numerical example for a new site selection of plastic manufacturing company is presented to illustrate the proposed method and show the efficiency of it. A comparison made between the proposed method and AHP method is developed to ensure that the proposed method offers reliable results. Then a sensitivity analysis is conducted for robustness validation. In sensitivity analysis, various scenarios are obtained where the weight of a criterion considered for examination is changed, and equal weights are given to the other criteria to examine the robustness of the results (Triantaphyllou, Citation2000) (Keshavarz Ghorabaee et al. Citation2015).
Literature Review
Best Worst Method (BWM) is a new method for solving multi-criteria decision-making problems introduced by Rezaei in 2015. In this method, the best (most important) and the worst (least important) criteria are chosen by the decision-maker. Afterward, pairwise comparisons are performed between each of the most and least important criteria and the other criteria. For calculating the decision-making criteria a maximum problem is formulated. In order to check the reliability of the comparisons a consistency ratio is proposed for the BWM (Rezaei Citation2015).
BWM is applied for assessing the social sustainability of supply chains (Ahmadi, Kusi-Sarpong, and Rezaei Citation2017). Another research used BWM to select suppliers on the basis of their green innovation ability (Gupta and Barua Citation2017). The selection of biomass thermochemical conversion technology using BWM in the Netherlands is presented at (Van De Kaa, Kamp, and Rezaei Citation2017). The analysis of the barriers to humanitarian supply chain management is presented in (Sahebi, Arab, and Moghadam Citation2017). In addition, a hybrid model including BWM and total area based on orthogonal vectors method to analyze the technology portfolio selection problem is presented (Mokhtarzadeh et al. Citation2018). From previous studies, it is concluded BWM method is achieving good results in weighting the decision-making criteria as it needs less comparison data than other methods and provides more reliable results.
In BWM a new structure of pairwise comparison is performed as experts only do reference comparisons. BWM has advantages over the AHP that is usually used for site selection as it reduces the complexity of comparisons and maintains the consistency of pairwise comparisons by disregarding redundant comparisons. Therefore, the results imitated by the BWM are more reliable than those by the AHP (Xiaomei et al., Citation2019).
EDAS (Evaluation based on Distance from Average Solution) method was firstly proposed by Keshavarz Ghorabaee et al. in 2015. The EDAS method is a multi-criteria decision-making method which is useful in decision problems that contain conflicting criteria. It depends on positive and negative distances from the average for appraising alternatives. The authors also presented a comparative analysis to indicate the validity of the proposed method and, compared EDAS method with Simple Additive Weighting (SAW) Technique for Preference by Similarity to the ideal Solution (TOPSIS), Complex Proportional Assessment (COPRAS), and VlseKriterijumska Optimizacija I Kompromisno Resenje (VIKOR) which means multi-criteria optimization and compromise solution methods (Keshavarz Ghorabaee et al. Citation2015). The problem with these methods is that they are appropriate when the information is numerical values and the results concern is suggesting a compromise solution.
Later, EDAS method was applied to different fields. For Example, EDAS method is used to solve air traffic problems in the Democratic Republic of Congo (Kikomba et al. Citation2016). Another research used integrated method based on AHP (Analytical Hierarchy Process) and EDAS methods to evaluate scenarios of city logistics in Doboj. The research used AHP method to determine criteria weights, and rank the alternatives with EDAS method (Stević et al. Citation2016). EDAS method is used to select the most suitable sewing machine for a textile firm (Ulutaş Citation2017).
Rahman et al. used Analytic Hierarchy Process (AHP) to select the most suitable site for plastic manufacturing industries in Bangladesh taken five commercial districts as location and ten criteria for deep consideration from all promising sites of Bangladesh (Rahman et al. Citation2018). AHP also used to study the conditions for installing a plant for jeans production in three possible regions where the most important textile companies in the country are located (Costa, Borges, and Dos Santos Machado Citation2016). But AHP has problems due to interdependence between criteria and alternatives; which leads to inconsistencies between judgment and ranking criteria.
A hybrid model based on AHP and EDAS methods was proposed to evaluate the heritage buildings intended for renovation based on their values (Turskis, Morkunaite, and Kutut Citation2017). A comparative study for quality assurance in different contractor contacts was presented with different multi-criteria decision-making methods including EDAS and other methods which are: SAW, TOPSIS, and COPRAS (Trinkūnienė et al. Citation2017). The evaluation of steam boiler alternatives is presented based on integrated method based on using Measuring Attractiveness by a Categorical Based Evaluation Technique (MACBETH) to determine the best steam for the dyehouse of the textile company (Kundakcı Citation2019). From previous studies, EDAS is useful for the alternatives evaluation as it has no complex calculations and can handle conflicting criteria. The advantage of EDAS method is that the best alternative is determined based on the distance from average, so decision makers do not need to determine ideal solutions (Keshavarz Ghorabaee et al. Citation2015).
Multi-criteria group decision-making methods are applied to take a decision for different problems of the various issues such as site selection (Devi and Yadav Citation2013; Dey et al. Citation2017), supplier selection (Lima-Junior and Carpinetti Citation2016; Qin, Liu, and Pedrycz Citation2017), project management (Heravi, Fathi, and Faeghi Citation2017) and performance evaluation (Uygun and Dede Citation2016).
In addition, some researchers discussed group-based decision-making methods applied by using a novel instrument to aggregate the preferences (Verma and Rajasankar Citation2016; Yan, Ma, and Huynh Citation2017). You et al. (Citation2015) extended a novel VIKOR method using linguistic information for the supplier selection problem that concludes the acceptable results. Also, Heravi, Fathi, and Faeghi (Citation2017) used group decision-making methods to evaluate some indicators related to the sustainability options in the construction industry.
Methodology
The proposed method is divided into three parts; the first part is to determine the criteria, sub-criteria and alternatives. The second part is to determine the criteria and sub-criteria weight using BWM method and the third part is to evaluate alternatives using EDAS method as shown in ():
The First part includes deriving the weights of the criteria by BWM method described as followis (Rezaei Citation2015; Rezaei Citation2016):
Step 1. Define the sets of decision-makers, criteria, and alternatives.
Step 2. The most important and the least important criteria and sub-criteria are with no comparison.
Step 3. The preference of the most important criterion over all the other using a number between 1 and 9 is determined as follows:
AB = (aB1,aB2, …, aBn)
Step 4. The preference of all criteria over the least important criterion using a number between 1 and 9 is determined as follows:
AB = (a1w,a2w, …, anw)
Step 5. At this step, the priority of the best sub-criterion over each of other sub-criterion is determined as a number between 1 and 9, which is expressed as AB = (ajB1; aj B2; …, ajBk), where ajBk is the priority of the best sub-criterion over k-th sub-criterion in j-th criterion.
Step 6. The priority of each sub-criterion over the worst sub-criterion is determined for each criterion as a number between 1 and 9, which is expressed as AB = (aj1W; aj 2W, …, aj kW), where ajkWis the priority of k-th sub-criterion over the worst sub-criterion for j-th criterion.
Step 7. Calculating the weights of the criteria and sub-criteria for each expert.
By solving the problem, we can obtain the optimal weight and ξL*. ξL* is an indicator of consistency, where the closer numbers to zero show better consistency.
Step 8. Calculating the aggregated weight for all experts, which is expressed as w is aggregated weight of criteria or sub-criteria, Wj is for the criteria and sub-criteria for each expert, and ne is number of experts.
The second part is to evaluate the alternatives by using EDAS method as following (Keshavarz Ghorabaee et al. Citation2015):
Step 9. The criteria and alternatives of the decision problem are defined.
Step 10. The second step includes the construction of the decision matrix X for each expert.
Step 11. This step includes the construction of aggregated decision matrix X for all experts.
Step 12. Calculate the average solution (AV) based on all criteria.
Step 13. This step includes the calculation of the Positive Distance from Average (PDA) and the Negative Distance from Average (NDA) matrices according to criteria type.
PDA = [PDAij]nxm, NDA = [NDAij]nxm
If jth criterion is benefited then:
If jth criterion is cost then:
Step 14. Weighted sum of PDA (SPi) and NDA (SNi) for all alternatives are calculated.
Step 15. For all alternatives, normalize SP and SN values
Step 16. Appraisal score (AS) for all alternatives is calculated, as follows:
Step 17. The alternative with the highest AS is the most suitable choice among the other alternatives.
Application
In this part, site selection problem of a plastic manufacture located in Egypt is solved with the proposed method. In this way, applicability of the integrated method is illustrated. A new firm decided to choose a location for the manufacture. After preliminary research, four locations (6th of October City, 10th of Ramadan City, Badr City, Delta Misr) are determined among various locations by Industrial Development Authority of Egypt.
Then, decision makers defined four main criteria for evaluating these sites which are C1 (Economic includes initial cost, raw materials cost, proximity to target market), C2 (Environment includes distance from residential areas, waste disposal area and health and safety), C3 (Production facilities include proximity to energy sources, proximity to raw materials sources, proximity to water sources, employment stability) and C4 (Physical facilities include proximity to fire response equipment, opportunities for possible site expansion, proximity to public transport) as shown in .
After determining the decision makers, alternatives and criteria, the decision-makers give the importance of criteria based on BWM, and then evaluate location alternatives by EDAS method. BWM is proposed to calculate the weights of the criteria. Then, the ranking of the alternatives is obtained with the help of EDAS method. Finally, the most suitable location is selected which has the highest appraisal score.
BWM questionnaire data obtained by experts referred at acknowledgment and the pairwise comparisons of criteria and sub-criteria including best-to-others (BO) and others-to-worst (OW) are represented in . The experts’ responses are collected by semi-structured questionnaire, as they are asked to compare their selected most important criterion with each of the other criteria and state their preference by using a value between 1 and 9. A score of 1 implies an equal importance and score of 9 implies that the most important criterion is extremely more preferred with respect to the other criteria. Then, by calculating arithmetic mean of the three expert’s questionnaires, aggregated weight is constructed, which is illustrated in .
Table 1. The comparisons for the criteria
Table 2. The comparisons for the sub-criteria
Table 3. The weights for the criteria and sub-criteria
The ranking of the site alternatives is obtained with EDAS method. In EDAS method, firstly decision matrix is constructed as seen in . This matrix consists of the data of the sites alternatives. Here, the data of C11, C12, which are quantitative but the data of other criteria, are qualitative and obtained as a percentage. Decision matrix is constructed then average based on all criteria is determined using EquationEquations (5)(5)
(5) . These average values are in the last column of . Later, Positive Distance Average matrix is formed by EquationEquation (6)
(6)
(6) for benefit criterion and for cost criterion by EquationEquation (8)
(8)
(8) . Then, Negative Distance Average matrix by EquationEquation (7)
(7)
(7) for benefit criterion and for cost criterion EquationEquation (9)
(9)
(9) is used. Then for all alternatives, weighted sum of Positive Distance Average and Negative Distance Average are determined by using EquationEquations 9
(9)
(9) and Equation10
(10)
(10) . Then, SPi and SNi values are calculated as shown in the first and second columns of .
Table 4. Data for plastic manufacture site alternatives
Table 5. Results of the EDAS method
For all alternatives, weighted sum of PDA (SP) and weighted sum of NDA (SN) are normalized by EquationEquations (12)(12)
(12) and (Equation13
(13)
(13) ) respectively to obtain NSPi and NSNi values as shown in . Finally, appraisal score for all alternatives is calculated with EquationEquation (14)
(14)
(14) as shown in .
Discussion and Results
In decision-making process, the criteria weights are to show the importance of the criteria. There are many criteria that cannot be expressed in quantitative values and it is difficult to determine the importance of these criteria. The logical idea is that the criterion with the highest weight is the most important. But in some cases, it is not true as lower weight criterion may be critical. It is needed to know how sensitive the order of alternatives is. In this section, a sensitive analysis to examine the effect of changing the criteria weights on ranking and a comparison between the proposed method, AHP method and VIKOR method is presented.
The results of sensitive analysis approach showed that the alternative 10th of Ramadan City is suggested as the most suitable site in the proposed method, has the highest score in 10 experiments from 12 experiments.
The comparison between that the proposed method, AHP and VIKOR methods as seen in () showed the following:
Table 6. A comparison of alternatives weights by the proposed method, AHP method and vikor method
The most used method in site selection in previous studies was AHP and VIKOR Methods. AHP needs a lot of pairwise comparisons. VIKOR method is suitable when information is in numerical values, as it has no way to determine the criteria weights.
The proposed method is compared with Group-AHP method and Group-VIKOR method where the obtained solutions are compared as shown in (). It was found that the ranking obtained by proposed method was the same and in agreement with the other methods. It is concluded from this comparison that the proposed method offers reliable results when collaboration takes place between decision makers and experts, and a good method is adopted.
BWM needs less time for comparisons than those in AHP, because the BWM needs reference comparisons regarding the best criterion and the worst criterion, while the AHP needs whole matrix of comparisons.
BWM depends on structured comparing process based on 1–9 scale integers, while in the AHP the 1/9–9 scale is used. This makes the complexity of comparisons in BWM is lower than as integral scale is much closer to human intuition.
EDAS method determines the best alternative based on the distance from average. So the decision maker does not need to determine ideal solutions.
Conclusion
In this paper, a hybrid method is proposed for the site selection problem. This method is based on BWM and EDAS which is more simple and time saving. BWM method is used for determining the weights of the criteria, whereas EDAS method is used for evaluating the site alternatives. In order to show the applicability of the proposed method, plastic manufacture site selection problem is solved. According to the results of the method final evaluating of site alternatives is obtained as 10th of Ramadan City > 6th of October City > Badr City > Delta Misr. As a result, the most appropriate site is found as 10th of Ramadan City as proved by using Group-VIKOR and Group-AHP method and sensitivity analysis.
One of the methods used in site selection in previous studies was AHP which needs a lot of pairwise comparisons. This makes AHP enormously complex and time-consuming method. Also VIKOR method is used for site selection but it is not suitable when information is not defined in numbers, and in the solution that is presented is compromised.
The proposed method is compared with other Group-AHP method and the obtained solutions are compared as shown in . It is concluded from this comparison that the proposed method offers reliable results when collaboration takes place between decision makers and experts, and a good method is adopted. The results of the study cannot be generalized, due to the fact that the proposed cannot evaluate products by itself.
In future studies, researchers can compare more methods with the proposed method. Also, researchers can expand the method by using fuzzy or neutrosophic numbers. Moreover, future research can integrate the proposed model into other multi-criteria decision-making methods.
Acknowledgments
Hany Mahdy, Owner of Plastic Factory.
Asmaa ashour zaid, Research Egypt State of the Environmental at eeaa
Prof. Ebtehal Ahmed Abd-AlMoity, Regional Development Department, Faculty of Urban and Regional Planning- Urban, Cairo University.
Industrial Development Authority of Egypt.
References
- Ahmadi, H. B., S. Kusi-Sarpong, and J. Rezaei. 2017. Assessing the social sustainability of supply chains using best worst method. Resources, Conservation and Recycling 126:99–106. doi:10.1016/j.resconrec.2017.07.020.
- Athawale, V. M., and S. Chakraborty (2010). Facility location selection using PROMETHEE II method. Proceedings of the 2010 international conference on industrial engineering and operations management, pp. 9–10.
- Chakraborty, S., E. K. Zavadskas, and J. Antucheviciene. 2015. Applications of WASPAS method as a multi-criteria decision-making tool. Economic Computation and Economic Cybernetics Studies and Research 49 (1):5–22.
- Costa, J. F. S., A. R. Borges, and T. Dos Santos Machado. 2016. Analytic hierarchy process applied to industrial location: A brazilian perspective on jeans manufacturing. International Journal of the Analytic Hierarchy Process 8 (1):77–91.
- Devi, K., and S. P. Yadav. 2013. A multicriteria intuitionistic fuzzy group decision making for plant location selection with ELECTRE method. The International Journal of Advanced Manufacturing Technology 66 (9–12):1219–29. doi:10.1007/s00170-012-4400-0.
- Dey, B., B. Bairagi, B. Sarkar, and S. K. Sanyal. 2017. Group heterogeneity in multi member decision making model with an application to warehouse location selection in a supply chain. Computers & Industrial Engineering 105 (SupplementC):101–22. doi:10.1016/j.cie.2016.12.025.
- Gupta, H., and M. K. Barua. 2017. Supplier selection among SMEs on the basis of their green innovation ability using BWM and fuzzy TOPSIS. Journal of Cleaner Production 152:242–58. doi:10.1016/j.jclepro.2017.03.125.
- Heravi, G., M. Fathi, and S. Faeghi. 2017. Multi-criteria group decision-making method for optimal selection of sustainable industrial building options focused on petrochemical projects. Journal of Cleaner Production 142:2999–3013. doi:10.1016/j.jclepro.2016.10.168.
- Keshavarz Ghorabaee, M., E. K. Zavadskas, L. Olfat, and Z. Turskis. 2015. EDAS. Multi‐criteria inventory classification using a new method of evaluation based on distance from average solution. Informatica 26(3):435–51. doi: 10.15388/Informatica.2015.57.
- Kikomba, M. K., Mabela, R. M., & Ntantu, D. I. (2016). Applying EDAS method to solve air traffic problems. International Journal of Scientific and Innovative Mathematical Research (IJSIMR), 4(8), 15–23.
- Kundakcı, N. 2019. An integrated method using MACBETH and EDAS methods for evaluating steam boiler alternatives. Journal of Multi-Criteria Decision Analysis 26 (1–2):27–34. doi:10.1002/mcda.1656.
- Lima-Junior, F. R., and L. C. R. Carpinetti. 2016. A multicriteria approach based on fuzzy QFD for choosing criteria for supplier selection. Computers & Industrial Engineering 101 (SupplementC):269–85. doi:10.1016/j.cie.2016.09.014.
- Mi, Xiaomei, Ming Tang, Huchang Liao, Wenjing Shen, and Benjamin Lev. “The state-of-the-art survey on integrations and applications of the best worst method in decision making: Why, what, what for and what's next?.” Omega 87 (2019): 205–225
- Mokhtarzadeh, N., H. Mahdiraji, M. Beheshti, and E. Zavadskas. 2018. A novel hybrid approach for technology selection in the information technology industry. Technologies 6 (1):34. doi:10.3390/technologies6010034.
- Qin, J., X. Liu, and W. Pedrycz. 2017. An extended TODIM multi-criteria group decision making method for green supplier selection in interval type-2 fuzzy environment. European Journal of Operational Research 258 (2):626–38. doi:10.1016/j.ejor.2016.09.059.
- Rahman, M. S., M. I. Ali, U. Hossain, and T. K. Mondal. 2018. Facility location selection for plastic manufacturing industry in Bangladesh by using AHP method. International Journal of Research in Industrial Engineering 7 (3):307–19.
- Rezaei, J. 2015. Best-worst multi-criteria decision-making method. Omega (53):49–57. doi:10.1016/j.omega.2014.11.009.
- Rezaei, J. 2016. Best-worst multi-criteria decision-making method: Some properties and a linear model. Omega 64:126–13. doi:10.1016/j.omega.2015.12.001.
- Sahebi, I. G., A. Arab, and M. R. S. Moghadam. 2017. Analyzing the barriers to humanitarian supply chain management: A case study of the tehran red crescent societies. International Journal of Disaster Risk Reduction 24:232–41. doi:10.1016/j.ijdrr.2017.05.017.
- Stević, Ž., I. Tanackov, M. Vasiljević, and S. Vesković (2016). Evaluation in logistics using combined AHP and EDAS method. International Symposium on Operational Research, XLIII, 309–13.
- Taibi, A., and B. Atmani. 2017. Combining fuzzy AHP with GIS and decision rules for industrial site selection. International Journal of Interactive Multimedia & Artificial Intelligence 4 (6):6. doi:10.9781/ijimai.2017.06.001.
- Triantaphyllou, E. (2000). Multi-criteria decision making methods. In Multi-criteria decision making methods: A comparative study (pp. 5–21). Springer, Boston, MA.
- Trinkūnienė, E., V. Podvezko, E. K. Zavadskas, I. Jokšienė, I. Vinogradova, and V. Trinkūnas. 2017. Evaluation of quality assurance in contractor contracts by multi-attribute decision making methods. Economic Research Ekonomska Istraživanja 30 (1):1152–80. doi:10.1080/1331677X.2017.1325616.
- Turskis, Z., Z. Morkunaite, and V. Kutut. 2017. A hybrid multiple criteria evaluation method of ranking of cultural heritage structures for renovation projects. International Journal of Strategic Property Management 21 (3):318–29. doi:10.3846/1648715X.2017.1325782.
- Ulutaş, A. 2017. Sewing machine selection for a textile workshop by using EDAS method. Journal of Business Research Turk 9 (2):169–83. doi:10.20491/isarder.2017.266.
- Uygun, Ö., and A. Dede. 2016. Performance evaluation of green supply chain management using integrated fuzzy multi-criteria decision making techniques. Computers & Industrial Engineering 102 (SupplementC):502–11. doi:10.1016/j.cie.2016.02.020.
- Uysal, H., and K. Yavuz. 2014. Selection of logistics centre location via ELECTRE method: A case study in turkey. International Journal of Business and Social Science 5:9.
- Van De Kaa, G., L. Kamp, and J. Rezaei. 2017. Selection of biomass thermochemical conversion technology in the Netherlands: A best worst method approach. Journal of Cleaner Production 166:32–39. doi:10.1016/j.jclepro.2017.07.052.
- Verma, M., and J. Rajasankar. 2016. A thermodynamical approach towards group multicriteria decision making (GMCDM) and its application to human resource selection. Applied Soft Computing, 52, 323–332..
- Yan, H.-B., T. Ma, and V.-N. Huynh. 2017. On qualitative multi-attribute group decision making and its consensus measure: A probability-based perspective. Omega 70:94–117. doi:10.1016/j.omega.2016.09.004.
- Yaşlioğlu, M. M., and E. Önder. 2016. Solving facility location problem for a plastic goods manufacturing company in turkey using AHP and TOPSIS methods. Journal of Administrative Sciences 14 (28):223–49.
- You, X.-Y., J.-X. You, H.-C. Liu, and L. Zhen. 2015. Group multi-criteria supplier selection using an extended VIKOR method with interval 2-tuple linguistic information. Expert Systems with Applications 42 (4):1906–16. doi:10.1016/j.eswa.2014.10.004.
- Zavadskas, E. K., Z. Turskis, and S. Kildienė. 2014. State of art surveys of overviews on MCDM/MADM methods. Technological and Economic Development of Economy 20 (1):165–79. doi:10.3846/20294913.2014.892037.